ORIGINAL RESEARCH
Published on 30 Nov 2021
Nrf2 Promotes Inflammation in Early Myocardial Ischemia-Reperfusion via Recruitment and Activation of Macrophages
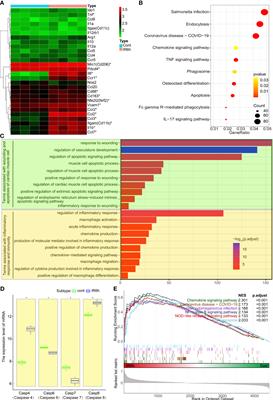
doi 10.3389/fimmu.2021.763760
- 5,600 views
- 25 citations
6,957
Total downloads
19k
Total views and downloads
Select the journal/section where you want your idea to be submitted:
ORIGINAL RESEARCH
Published on 30 Nov 2021
ORIGINAL RESEARCH
Published on 23 Nov 2021
ORIGINAL RESEARCH
Published on 03 Jun 2021
ORIGINAL RESEARCH
Published on 14 May 2021
Frontiers in Immunology