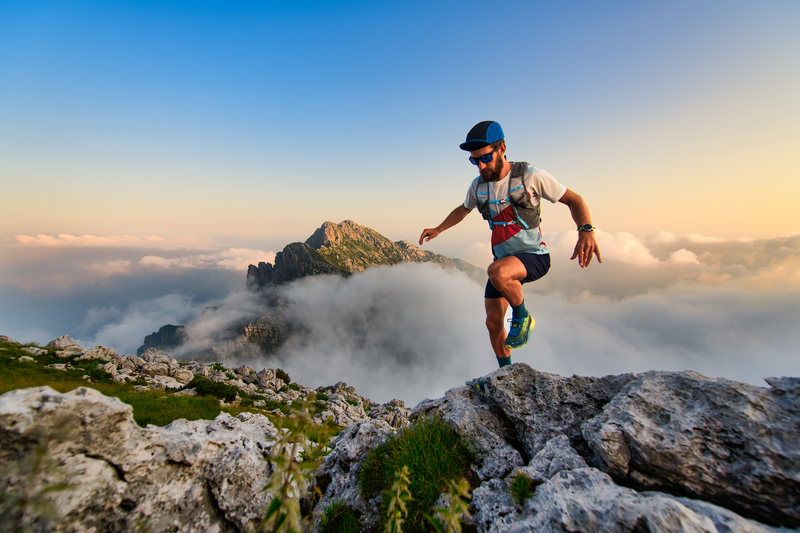
94% of researchers rate our articles as excellent or good
Learn more about the work of our research integrity team to safeguard the quality of each article we publish.
Find out more
EDITORIAL article
Front. Water
Sec. Water and Climate
Volume 7 - 2025 | doi: 10.3389/frwa.2025.1579106
This article is part of the Research Topic Spatiotemporal Modelling and Assessment of Water-related Multi-hazards View all 8 articles
The final, formatted version of the article will be published soon.
You have multiple emails registered with Frontiers:
Please enter your email address:
If you already have an account, please login
You don't have a Frontiers account ? You can register here
artificial intelligence-based approaches, such as long short-term memory (LSTM) and sequential data assimilation (SDA) techniques as tools to forecast such unprecedented climate hazards at lead times, necessary for operational perspectives, and finally one paper dealt with investigating likelihood of wet-dry and dry-wet transitions across Meuse River basin in the Netherlands, highlighting the preconditioning role of meteorological scenarios in triggering catchment-specific hydrologic responses.Compound flooding (CF) is often driven by a combination of physical processes, such as hurricanes, storm surges, heavy rainfall, and high tides. However, current flood modelling and mitigation strategies typically only focus on individual mechanisms, neglecting the co-occurrence of these sources and their compounded effects (Bevacqua et al., 2020;Radfar et al., 2024). Using statistically grounded methods, such as encapsulating trend analysis with a bivariate probabilistic framework, Lewis et al. showed existence of non-stationarity (i.e., temporal changes in hydrological and meteorological variables), non-linearity (i.e., complex interactions between flood drivers), and multi-dimensionality (i.e., various factors influencing flood risk) among compound flood drivers, focusing on two coastal areas on the Gulf Coast of the United States. Combined with statistical tests, their study also includes a comprehensive review of relevant CF policy documents. They find clear evidence of compounding and shifts in the first-order statistical moment (i.e., mean shift) of flood drivers in a warming climate, but there remains a significant gap between science and practice, as most current policies fail to adequately consider the complex and dynamic nature of CF. This highlights the critical need for an integrated approach that accounts for multi-mechanism and non-stationarity in CF assessments.To bridge the gap between theory and practice, Wang etal. developed a simple conceptual framework, 'c-HAND' (Coastal-Height Above Nearest Drainage) to map compound fluvial and coastal inundation. Subsequently, c-HAND was coupled with a fluvial flood forecasting model to create a compound fluvial-coastal inundation mapping workflow that can be run in near realtime. The framework's fast wall-clock time and low CPU requirements add value towards near real-time flood inundation mapping of compound coastal ─ fluvial floods in low-lying marine fluvial transition regime. The method was validated against results from a state-of-the-art numerical ocean circulation model ADCIRC for a massive coastal flooding, resulting from Hurricane Ike (September 11 ─ 13, 2008) at Southeast Texas region, along the Gulf of Mexico. One of the practical challenges in CF mitigation is either joint or back-to-back impacts of several mutually interdependent climatic and weather stressors within a short time window, leaving little time for recovery, which can propagate through a network of infrastructure systems, causing cascading failures (Najafi et al., 2021). The study by Preisser et al. attempted to prioritize the critical resources (such as hospitals and groceries), when storm occurs in a near-real time. Based on open ─ source data and a network-based approach, they propose a model to solve the 'user equilibrium traffic assignment problem' by calculating how an individual's access to critical resources changes during and immediately the post ─ flood event. In a case-study for Austin, Texas they found that the most vulnerable households are the least resilient to the impacts of floods and experience the most volatile shifts in redundancy, reliability, and recoverability. The open-source framework developed in the paper may benefit emergency planning stakeholders by helping identify households that require specific resources during and immediately after hazard events.Due to climate change consequences, in some regions, not only are the frequency and magnitude of similar or dissimilar kinds of hydrological extremes (e.g., floods and droughts) increasing, but transitions between these events are also becoming more frequent and abrupt, accelerating economic and societal impacts (Chen and Wang, 2022;Bai et al., 2023;Xi et al., 2023;Banfi et al., 2024;Bowers et al., 2024). Based on observations of over seven decades (1951─2022), Sudha et al. developed a statistical framework to define meteorological extreme -wet (i.e., abundance of water) and dry (i.e., lack of water) events -and investigate the transitions between them using a case study of Meuse River basin in the Netherlands. Their analysis indicates a statistically significant increase in water deficit due to evapotranspiration in Spring and Summer, along with increased lengths of dry spells due to warming temperature. They also identify abrupt transitions between wet and dry phases of extremes that challenge water management, with such conditions occurring in 6% of wet-dry and 20% of dry-wet transitions. These findings provide new insights into nonstationary trends of contrasting compound climate stressors in hydrological risk assessment.Modeling and quantification of catastrophic risk require interaction of several correlated hazards ranging from natural and anthropogenic stressors. In particular, coincidence and/or lagged occurrences between different hazard categories, responsible for extreme impact are dictated by causality or interconnectedness of different stressors, which in turn influenced by climate change, urbanization ─ leading to changes in land use and land cover (LULC) pattern, and technological advancement. Siddique et al. quantified changes in land use and land cover for an ecologically critical area, a freshwater wetland system, Hakaluki Haor in Sylhet region of Bangladesh. Leveraging Landsat satellite data in a cloud-based platform (Google Earth Engine Database), coupled with state-of-the-art machine learning models, this study analyses LULC dynamics from 2000 to 2023. While one of the striking findings of the study is overall more than half of the areas show reduction in water bodies in the wet-land ecosystem, other notable changes include over 350% increase in settlements with substantial decline (> 70%) in vegetation cover. Similar shifts in wet-land extents have been observed in other locations across the globe. A prominent example includes, Lake Urmia in northwestern Iran, which has experienced a drastic shrinkage due to multiple anthropogenic stressors, such as aggressive water resources development plans, intensive agricultural practices, competition for water resources in upstream reaches (AghaKouchak et al., 2015). The lake has not managed to recover to its designated ecological threshold, even during the normal water year after the persistent 5-year drought of 1998─2002 (AghaKouchak et al., 2015;Alborzi et al., 2018). Although anthropogenic stressors are major drivers, the natural drivers responsible for wetland changes could be shifted precipitation patterns ─ more drying in arid environments and increased infiltration in the nival regime due to warmer temperatures, reducing soil ice, as well as substantial drying of the wetland ecosystem when biotic processes peak (Xu et al., 2024).In a complex landscape, runoff mechanisms and sub-surface flows are dictated by varying geological formations influencing baseflows, soil types, and vegetation, which could be challenging to model given the paucity of data. Merizalde et al highlights the importance of feature engineering to improve the performance of deep learning models in ungauged hydrological systems. The authors combined satellite-derived rainfall products, soil and land cover maps, digital elevation models, and empirical rainfall-runoff methods to feed specialized runoff forecasting models in the Andes of Ecuador. Results of this research are promising with lead times up to 11 h; enabling near real-time forecasting and paving the way for more advanced techniques focused on mountainous areas characterized by geographic complexity and data availability constraints. While there are few efforts on applications of statistical learning in predicting runoff in ungauged catchments (Razavi and Coulibaly, 2013;Pugliese et al., 2018;Prieto et al., 2019;Herath et al., 2021), such approaches in modelling groundwater flow and contaminant transport have recently started gaining popularity (McConnell et al., 2022;Rad et al., 2024). Béraud et al.introduce an innovative data assimilation method that combines Ensemble Smoother with Multiple Data Assimilation, updating parameters locally around each observation well through successive assimilations, thus enabling credible calibration of large, complex groundwater models with limited observations. The model is further validated with a synthetic 3D model and a real regional groundwater flow model, showing significant improvements in calibration and predictions in heterogeneous areas, proving the model's skills in predicting changes in groundwater flow patterns and contaminant transport.Finally, a few aspects of this collection are worth highlighting: (1) Water resources modellers and stakeholders need to be aware of limitations of existing practices, such as assumptions of independence for modelling multi-hazard events in space and time. Proper coordination between scientists and the stakeholder community is required to develop societal resilience to mitigate such hazards. (2) Climate change consequences have led to shifts in timing and trend in hydroclimatic extremes, which in turn mediated the frequency of compound hazards (Blöschl et al., 2017;Kemter et al., 2020;Raut and Ganguli, 2024). Through the application of 'process-based advanced machine learning' tools, development and implementation of credible multi-hazard warning systems can be one of the most effective ways to reduce mortality associated with such natural hazards and protect natural and built environment systems in multihazard risk hotspots in a changing climate (ESCAP, 2023).
Keywords: extreme weather, Compound events, risk, Nonstationality, Interdepedence
Received: 18 Feb 2025; Accepted: 24 Feb 2025.
Copyright: © 2025 Ganguli, Han, Muñoz and Pal. This is an open-access article distributed under the terms of the Creative Commons Attribution License (CC BY). The use, distribution or reproduction in other forums is permitted, provided the original author(s) or licensor are credited and that the original publication in this journal is cited, in accordance with accepted academic practice. No use, distribution or reproduction is permitted which does not comply with these terms.
* Correspondence:
Poulomi Ganguli, Indian Institute of Technology Kharagpur, Kharagpur, India
Disclaimer: All claims expressed in this article are solely those of the authors and do not necessarily represent those of their affiliated organizations, or those of the publisher, the editors and the reviewers. Any product that may be evaluated in this article or claim that may be made by its manufacturer is not guaranteed or endorsed by the publisher.
Research integrity at Frontiers
Learn more about the work of our research integrity team to safeguard the quality of each article we publish.