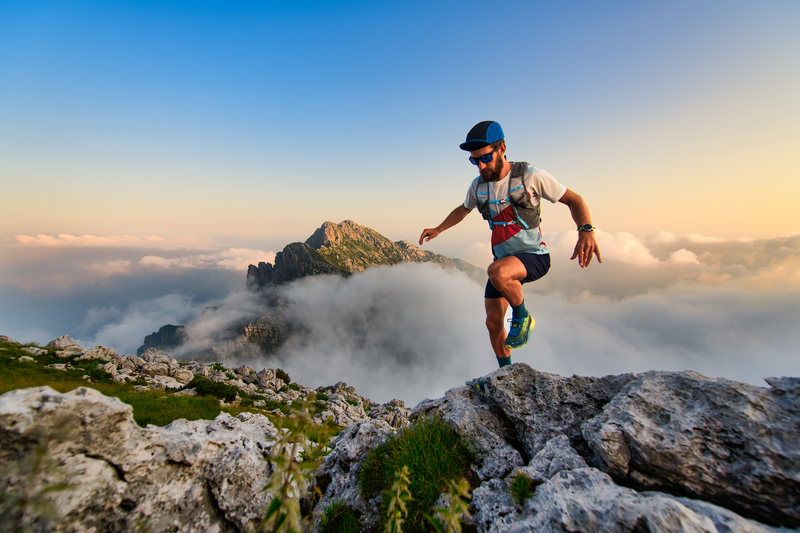
94% of researchers rate our articles as excellent or good
Learn more about the work of our research integrity team to safeguard the quality of each article we publish.
Find out more
ORIGINAL RESEARCH article
Front. Water
Sec. Water and Climate
Volume 7 - 2025 | doi: 10.3389/frwa.2025.1559046
This article is part of the Research Topic Understanding Drought Dynamics: Causes, Attribution, and Forecasting for Early Warning Systems View all articles
The final, formatted version of the article will be published soon.
You have multiple emails registered with Frontiers:
Please enter your email address:
If you already have an account, please login
You don't have a Frontiers account ? You can register here
Understanding the probability of drought occurrence in agricultural areas is important for designing effective adaptation strategies to drought impacts on agriculture and food security. This knowledge is critical, especially in arid and semi-arid areas of Morocco, which are prone and vulnerable to droughts.This study examines the linkage between meteorological drought (MD) and agricultural drought (AD) in a critical agricultural region in Morocco. Different agricultural drought indexes (NDVI anomaly, vegetation condition index (VCI), temperature condition index (TCI), vegetation health index (VHI)), and a meteorological drought index (Standardized Precipitation Evapotranspiration Index (SPEI) in different time scales (3, 6, 9, 12 months)) are assessed for the period 2000-2022. Statistical measures such as Spearman correlation (R), root mean square error (RMSE), and mean absolute error (MAE), are utilized to assess the performance of the meteorological drought index to detect the agricultural drought. The propagation time from meteorological drought to agricultural drought was identified, and probabilistic linkages between the two types of droughts were investigated using the copula function and Bayesian network. Results show that a combination of SPEI3 as meteorological drought index and VHI as agricultural drought index has the highest correlation coefficient of 0.65 and the lowest RMSE and MAE of 1.5 and 1.5, respectively. The propagation time from meteorological to agricultural drought was 39 days on a scale of 12 months, and seasonally, it was 29 days, 32 days, and 82 days, for autumn, winter, and spring, respectively. Bayesian network results show that agricultural droughts have the high probability to occur whenever there is severe and extreme meteorological drought, with the highest probabilities for mild and moderate agricultural drought. The findings have significant applications in water resource management and agricultural planning, for water usage and food security based on likelihood of agricultural drought occurence.
Keywords: Agriculture, drought, Early warning system, Food security, Morocco
Received: 11 Jan 2025; Accepted: 17 Mar 2025.
Copyright: © 2025 Dahhane, Ongoma, Hadri, Kharrou, Hakam and CHEHBOUNI. This is an open-access article distributed under the terms of the Creative Commons Attribution License (CC BY). The use, distribution or reproduction in other forums is permitted, provided the original author(s) or licensor are credited and that the original publication in this journal is cited, in accordance with accepted academic practice. No use, distribution or reproduction is permitted which does not comply with these terms.
* Correspondence:
Younes Dahhane, international water research insitute, Mohammmed VI polytechnic university, Benguerir, Morocco
Disclaimer: All claims expressed in this article are solely those of the authors and do not necessarily represent those of their affiliated organizations, or those of the publisher, the editors and the reviewers. Any product that may be evaluated in this article or claim that may be made by its manufacturer is not guaranteed or endorsed by the publisher.
Research integrity at Frontiers
Learn more about the work of our research integrity team to safeguard the quality of each article we publish.