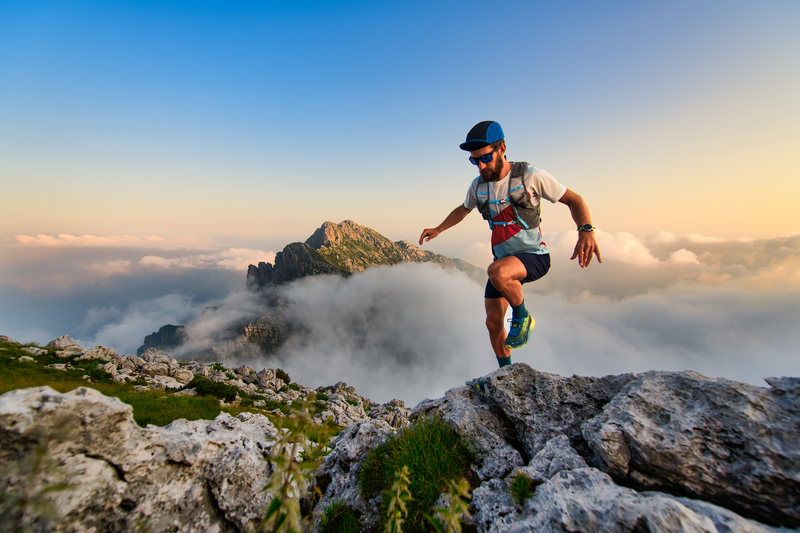
94% of researchers rate our articles as excellent or good
Learn more about the work of our research integrity team to safeguard the quality of each article we publish.
Find out more
ORIGINAL RESEARCH article
Front. Water
Sec. Water and Climate
Volume 7 - 2025 | doi: 10.3389/frwa.2025.1541052
This article is part of the Research Topic Droughts in a Changing Climate: Advances in Modeling, Forecasting and Strategies for Adaptation View all 6 articles
The final, formatted version of the article will be published soon.
You have multiple emails registered with Frontiers:
Please enter your email address:
If you already have an account, please login
You don't have a Frontiers account ? You can register here
General Circulation Models (GCM) have comprised ubiquitous tools for supporting water resources planning and decision-making under changing climate conditions. However, GCMs are often highly biased, which may limit their utilization for representing future trajectories of the hydroclimatic processes of interest. In addition, assessing the predictive uncertainty of climate models, which is paramount for simulation purposes, is not straightforward. For tackling these problems, in this paper we resort to the expanded Bluecat framework, which utilizes empirical conditional distributions for providing a complete stochastic representation of GCM outputs simultaneously to bias correction. The stochastic model was employed for assessing future trajectories of monthly rainfall and temperatures, under three Shared Socioeconomic Pathways, namely, SSP1-2.6, SSP2-4.5, and SSP5-8.5, in the Metropolitan Region of Belo Horizonte, Brazil. Our results indicated that e-Bluecat properly corrected bias for both variables and provided coverage probabilities close to the theoretical ones. Nonetheless, the resulting uncertainty, as materialized by confidence intervals, was deemed too large, which implicitly reflects the inability of the GCMs in describing the observed processes. In addition, in median terms, the bias-corrected estimates suggest considerably smaller increases in temperatures ( 1ºC), as compared to the climate models (up to 5ºC), in all future scenarios. These findings suggest that deterministic outputs of GCMs may present limitations in effectively informing adaptation strategies, necessitating complementary approaches . Moreover, in view of the large uncertainty 1 Santos et al.
Keywords: Anthropogenic climate change, Bias Correction, stochastic models, expanded Bluecat framework, meteorological drought assessment
Received: 06 Dec 2024; Accepted: 28 Feb 2025.
Copyright: © 2025 Santos, Barbosa, Oliveira, Silva, Mendiondo and Costa. This is an open-access article distributed under the terms of the Creative Commons Attribution License (CC BY). The use, distribution or reproduction in other forums is permitted, provided the original author(s) or licensor are credited and that the original publication in this journal is cited, in accordance with accepted academic practice. No use, distribution or reproduction is permitted which does not comply with these terms.
* Correspondence:
Veber Afonso Figueiredo Costa, Federal University of Minas Gerais, Belo Horizonte, 31270-901, Minas Gerais, Brazil
Disclaimer: All claims expressed in this article are solely those of the authors and do not necessarily represent those of their affiliated organizations, or those of the publisher, the editors and the reviewers. Any product that may be evaluated in this article or claim that may be made by its manufacturer is not guaranteed or endorsed by the publisher.
Research integrity at Frontiers
Learn more about the work of our research integrity team to safeguard the quality of each article we publish.