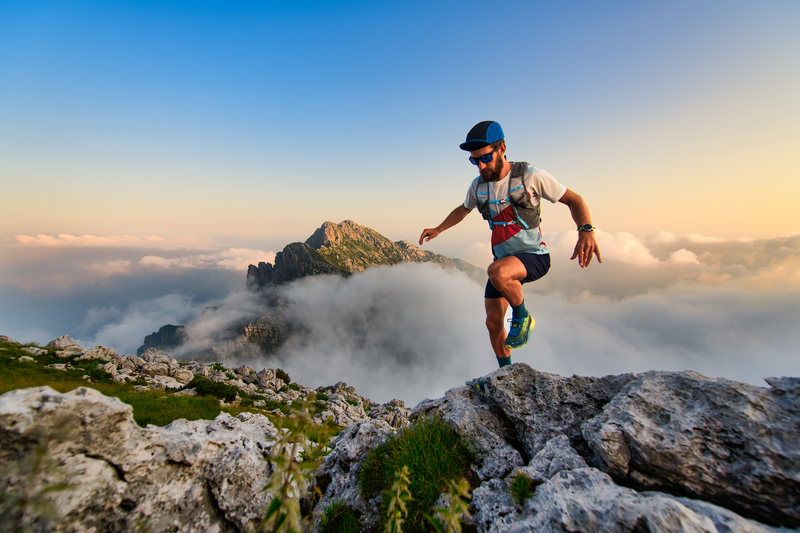
94% of researchers rate our articles as excellent or good
Learn more about the work of our research integrity team to safeguard the quality of each article we publish.
Find out more
ORIGINAL RESEARCH article
Front. Water
Sec. Water and Critical Zone
Volume 7 - 2025 | doi: 10.3389/frwa.2025.1465505
This article is part of the Research Topic Groundwater Vulnerability Assessments: Integrating Experimental, Modeling, and Mapping Techniques View all 4 articles
The final, formatted version of the article will be published soon.
You have multiple emails registered with Frontiers:
Please enter your email address:
If you already have an account, please login
You don't have a Frontiers account ? You can register here
Flood is the most frequent and destructive natural disaster, causing significant negative impacts on humans and built and natural ecosystems. While it is extremely challenging to prevent floods, their associated hazards can be mitigated through well-planned and appropriate measures. The present study combined the analytical hierarchy process (AHP) analysis and an ArcGIS-based multi-criteria decision-making (MCDM) approach to assess, categorize, quantify, and map the flood-prone areas in Khyber Pakhtunkhwa, Pakistan, a region particularly vulnerable to recurrent flooding. Eight key factors including precipitation, rivers/streams, slope, elevation, soil, normalized difference vegetation index, and land use were used for flood susceptibility modeling. The weighted sum overlay tool in global positioning system ArcGIS was utilized to give weightage to each raster layer, based on the AHP ranking to produce a flood susceptibility map for the study area. According to the AHP analysis, the most impactful factors defining the flood susceptibility in our study area were streams (0.29%), precipitation (0.23%), slope of the area (14%), and LST (10%). Our flood model achieved excellent accuracy, with Area Under the Curve (AUC) value of 0.911. The model predicted that 9% of the total area is classified as very high risk, while 14% is identified as high risk, covering approximately 923,257 hectares and 1,419,480 hectares, respectively. These high-risk zones are predominantly concentrated in the central and lower northern, densely populated districts of the province. Our flood susceptibility results would assist policymakers, concerned departments, and local communities in assessing flood risk in a timely manner and designing effective mitigation and response strategies.
Keywords: Flood susceptibility, AHP, MCDA, risk zones, Management, Khyber pakhtunkhwa, Pakistan
Received: 16 Jul 2024; Accepted: 14 Feb 2025.
Copyright: © 2025 Khan, Omifolaji and Iqbal. This is an open-access article distributed under the terms of the Creative Commons Attribution License (CC BY). The use, distribution or reproduction in other forums is permitted, provided the original author(s) or licensor are credited and that the original publication in this journal is cited, in accordance with accepted academic practice. No use, distribution or reproduction is permitted which does not comply with these terms.
* Correspondence:
Arshad Iqbal, Centre for Biotechnology and Microbiology, University of Swat, Mingora, Khyber Pakhtunkhwa, Pakistan
Disclaimer: All claims expressed in this article are solely those of the authors and do not necessarily represent those of their affiliated organizations, or those of the publisher, the editors and the reviewers. Any product that may be evaluated in this article or claim that may be made by its manufacturer is not guaranteed or endorsed by the publisher.
Research integrity at Frontiers
Learn more about the work of our research integrity team to safeguard the quality of each article we publish.