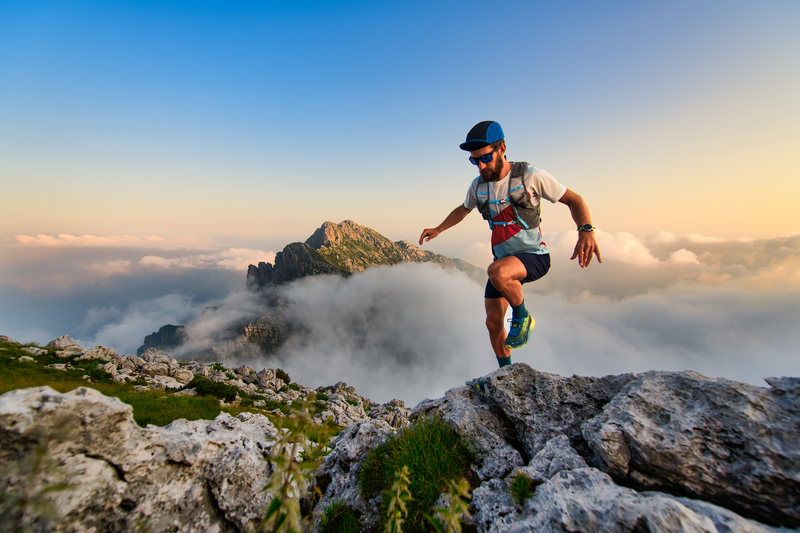
95% of researchers rate our articles as excellent or good
Learn more about the work of our research integrity team to safeguard the quality of each article we publish.
Find out more
METHODS article
Front. Water , 07 January 2025
Sec. Water and Built Environment
Volume 6 - 2024 | https://doi.org/10.3389/frwa.2024.1489315
This article is part of the Research Topic Blue-Green Infrastructure: Key to Sustainable Urban Development View all 3 articles
Kochi city in southern India periodically experiences waterlogging or urban floods due to unabated urban growth and extreme rainfall events. This study aims to mitigate urban flood hazards through green infrastructure (GI) and its effective management. Assessment of storm water runoff (SWR) modeling is carried out in four scenarios, viz., baseline, past, severe, and green, using urban growth and GI driven simulations. Urban growth modeling and GI suitability analysis are carried out using Cellular Automata Markov (CA-Markov) and urban planning guidelines, respectively. The study provides insights into how GI influences SWR reduction and urban environment conservation, with 16% SWR reduction as compared to the baseline scenario and 18% when compared to the severe scenario.
Urban flooding is triggered by undesirable modification in land use/ land cover (LULC), which is an outcome of rising population, industrialization and encroachment into urban drainage systems. It brings life to a standstill while causing enormous damage to urban infrastructure and environment. It is intensified by the loss of green spaces and conversion of wetlands into buildings, parking lots, roads, etc. Due to these alterations from permeable to impermeable surfaces, the infiltration potential of soil decreases and runoff rises at two to six times (Ramachandra and Kumar, 2008). The situation gets exacerbated after an extreme rainfall event (ERE) overwhelming the capacity of urban drainage system. According to König et al. (2002), the urban flooding has following consequences: direct, indirect and social impacts. Direct damages are primarily caused by water or water velocity and largely affect buildings and their interiors. These damages can range from major structural issues like cracks in foundations, etc. to damage to perishable goods. Indirect damages are caused due to irregularities and their repercussions, while social consequences contain all the associated long-term negative effects.
Green Infrastructure (GI) is an environment-friendly approach to manage storm water runoff (SWR) (Zhang et al., 2015) and defined as an effective system of naturally occurring vegetative structures that provides numerous environmental, social, and economic benefits to the society (Zhang et al., 2012). It aims to mimic natural surfaces, allowing rainwater to infiltrate the ground where it falls and be naturally filtered by soil and vegetation (Goldstein et al., 2004; Harlan, 2021). Urban green spaces (UGS) are crucial components of urban environment, which are generally emphasized by urban planners based on their abundance and quality (Gupta et al., 2012). GI utilizes flora, soils, and other elements and practices to restore natural processes which are essential to manage water effectively and create healthier cities. They provide flood resilience, improved air and water quality, and enhanced aesthetic and recreational values for communities (USEPA, 2014). Cities can have a variety of GIs including parks and gardens, roadside greens, railway corridors, derelict sites, etc. These green infrastructures significantly impact human well-being by offering valuable ecosystem services (Brzoska and SpāĢe, 2020). Sanchez and Govindarajulu (2023) observed that widespread urbanization has changed the network of GIs that connects existing waterways and water bodies in India’s coastal cities. LULC transition in Kochi during the past 15 years was examined by Thekkeyil et al. (2023). They discovered that while urban grids are getting close to saturation, taking up almost two-thirds of the land with urban elements at the expense of green space; the change rates are higher in rural regions. Using geospatial data and a multi-criteria decision approach, Nandakrishnan and Prasad (2024) conducted risk assessment and classified flood risk zones in a low-lying deltaic region. Thirteen flood conditioning criteria were utilized to determine the flood risk.
Kim et al. (2017) investigated the impacts of flood on GI health using Normalized Difference Vegetation Index (NDVI) derived from Moderate Resolution Imaging Spectroradiometer (MODIS) data. The results highlighted the importance of GIs in reducing flood risk and emphasized the requirement of a greenery index with local and regional GI-based land use plans for decision makers. Mobilia et al. (2014) carried out a study to analyze the impact of green roofs using rational method to assess the adequacy of storm water facility. They reported that the installation of green roofs has reduced the SWR by 40–98%. Nagarajan and Basil (2014) used geospatial methods in conjunction with the Soil Conservation Service Curve Number (SCS-CN) approach to evaluate runoff in urbanized zones within the Cochin Corporation area. A research by Zimmermann et al. (2016) found that GIs like parks, green spaces, and green roofs significantly enhance the essential ecosystem services in urban environment. Through a comprehensive review of recent studies, Khodadad et al. (2023) reported the importance of GIs to increase urban flood resistance. Chen et al. (2024) developed a multi-objective optimization tool for GIs planning and their hydrological linkages. Song et al. (2023) quantified the impacts of GIs and building resilience in coastal regions that are prone to disasters. The runoff reduction impact was highest when the maximum biotope area ratio of 30% was applied to artificial ground.
Although the impact of GIs on SWR has been studied previously, a comprehensive analysis of the effects of both GIs and urban expansion on SWR is still needed. Hence, the present study aims to analyze the impact of GIs and urban expansion on SWR based on urban growth modeling and GI suitability analysis in parts of Kochi city, India. The city frequently experiences the rapid recurrence of waterlogging and/ or urban flooding. These are very likely to increase, if nothing is done to address the unplanned growth of the city and poor management of urban drainage. This study focuses on finding the solution for flood risk reduction through increasing GI and management strategies. The objectives of the study are to: (a) generate a geodatabase of factors influencing the SWR, (b) analyze future urban growth using Cellular Automata Markov (CA-Markov) based technique, (c) carry out multi-criteria based GI suitability analysis as per urban planning guidelines and (d) estimate peak runoff for baseline, past, severe and green scenarios.
This study is carried out for an urban watershed with a geographical area of 400 ha within Kochi city, a major port city in southern India. The geographic coordinates of the city fall within 9°49’N to 10°14’N and 76°10′ E to 76°31′E. Kochi or Cochin is a major commercial centre in Kerala province of southern India. It is situated on India’s Malabar coast and often referred to as the ‘queen of the Arabian sea’. It has long been a preferred hub for South Asian trade due to its strategic location between the Arabian sea, the western Ghats, and the Alappuzha lagoons. With 6,340 inhabitants per square kilometre, Kochi is the most populous city and largest urban agglomeration in Kerala (Chandramouli, 2011).
Kochi and the surrounding areas have a tropical climate, with average daily temperature ranging from 23°C to 34°C all year round. The annual average rainfall is 3,015 mm with an annual average of 124 rainy days. Kochi city frequently gets affected by the flood as reported in the recent years. In 2018, Kerala received heavy rainfall which is 116 percent severe than the normal monsoon, and has also resulted into landslides and the worst floods in a century, killing over 480 people and incurring property damage to the tune of Indian Rupees 4 lakh crore (Kerala: Three years and the ravages of climate change, 2021). Strong depression systems during the 2019 monsoon also produced heavy rains, which led to catastrophic flooding that killed 121 people, directly impacted over 200,000 people, completely destroyed 1,789 dwellings, and partially damaged 14,524 homes (Kerala Floods 2019: 121 dead, 1,789 Houses Collapsed, 2019). Similarly, in November 2021, the Kerala state received 394.1 mm rainfall against the normal of 153.3 mm, i.e., an excess of 157% according to India Meteorological Department (IMD) (Record rainfall for November in Kerala this year, 2021).
The average elevation in city varies from less than 1 m above mean sea level (MSL) toward west and 7 m above MSL toward the eastern fringes (Sowmya et al., 2015). The soils of this region is broadly categorized into two categories as alluvial and lateritic. The central city, with flat terrain and low altitude, is interspersed with a network of canals that links to backwater. The city’s secondary canals serve as natural floodwater drainages, but are now severely clogged due to excessive silt and waste dumping. Several water bodies in the city are dwindling because of increasing urbanization and inefficient waste disposal system. Untreated sewage from industries and households is polluting the Periyar River, leading to water scarcity in the summer season (Shrivastava, 2016). Figure 1 shows the location map of study area.
The following datasets have been used in this study: (a) High-resolution Google Earth, (b) Moderate resolution Landsat-8, (c) Digital Elevation Model (DEM), (d) Three-hourly precipitation, and (e) Population data. The list of data, utilized in the present study is given in Table 1.
In this study, four scenarios, i.e., baseline, past, severe, and green, are developed to assess their impact on peak runoff. The baseline, past, and severe scenarios refer to runoff characteristics that are predicted through LULC and urban growth modeling for the years 2020, 2005 and 2035, respectively. The severe scenario is modeled using CA-Markov-based urban growth modeling for the year 2035. The green scenario is developed using suitability analysis and urban planning guidelines for Cochin City (Model Building Bye-Laws, 2016). Initially, a micro-watershed within Kochi city is delineated and the LULC maps are prepared for the years 2005 and 2020 using Object-based Image Analysis (OBIA) and contextual classification technique. Image segmentation method was followed with eCognition software for OBIA based image classification. Using the Gumbel probability distribution model of Generalized Extreme Value (GEV), the rainfall intensities for 3-h, 6-h, 12-h, and 24-h duration are estimated from intensity–duration–frequency (IDF) curves and peak runoff for all the four scenarios are derived by applying the rational method. Figure 2 shows the overall methodology followed for analyzing the effects of urban growth and GI on urban SWR.
a. LULC classification: OBIA involves categorization of pixels into objects or segments based on their spatial relationship to the surrounding pixels, and with spatial contiguity of pixels of similar texture, color, and tone (Walsh et al., 2008). LULC characteristics of the study area were identified using the OBIA approach. Initially, the image was segmented into vector objects using multiresolution segmentation. Next, the representative segments for each LULC class were selected, and the image was classified into 13 LULC classes using the nearest neighborhood technique.
b. Rainfall analysis: IDF curve represents the mathematical relationship between return period, rainfall duration, and intensity. It predicts the likelihood that a specific average rainfall will occur during the given timeframe (Sun et al., 2019). Maximum rainfall and floods are the extreme random hydrological variables, which are often described using the Extreme Value (EV) distribution model. Gumbel probability distribution model is a popular, asymmetric Type-1 EV model (Equation 1), which models maximum and minimum rainfall, and has been used in this study. The 3-hourly precipitation data for the past 20 years were sourced from Goddard Earth Sciences Data and Information Services Centre and near real-time (3B42RT) data of Multi-Satellite Precipitation Analysis (TMPA) from Tropical Rainfall Measuring Mission (TRMM) (NASA EOSDIS Distributed Active Archive Centers (DAACs), 2024) for IDF curve generation. Equation 1 defines the value of design rainfall corresponding to ‘T’ years return period.
Where, is rainfall for return period T, is mean of observations, S denotes the standard deviation of observations, and (Equation 2) is frequency factor associated with return period T. factor is further defined as given in Equation 2.
The 3-hourly rainfall data from TRMM 3B42 RT satellite for the past 20 years were aggregated as 6-hourly, 12-hourly, and 24-hourly rainfall data. Using the Gumbel probability distribution model, the IDF curve was generated to analyze the rainfall intensities.
c. Peak runoff Analysis: The rational method, which is initially proposed by Kuichling (1889) for peak runoff estimation has been used in this study. The rational method-based runoff coefficient for various LULC in the study area was drawn from the literature (Thanapura et al., 2007). The method is defined as Equation 3:
Where, Q is peak runoff (m3/s), C is runoff coefficient, ‘i’ denotes rainfall intensity (mm/h.), and A is area of watershed (hectare). The runoff coefficient (C) is 0 for impervious surfaces and 1 for pervious areas (Kuichling, 1889), which signifies that percentage of rainfall becoming runoff is proportional to the impervious surface percentage within watershed. The composite runoff coefficient is computed as follows (Equation 4):
Where, C is composite runoff coefficient, ‘i’ is sub-area (with particular LULC), is runoff coefficient for the given LULC, and is area of watershed.
Visual Basic (VB) language in the ArcGIS platform was used for the generation of CN map using thematic layers of hydrologic soil group and LULC maps.
a. Urban growth modeling: Markov chain method is one of the popular approaches for LULC computation that uses the past trends to predict the probability of a LULC change. It predicts the likelihood of land use changes for a future “t + 1” time using the progression from “t-1” to “t” (Wang et al., 2021). The probabilities obtained from past changes are utilized to forecast future changes. Markov chain method (Boerner et al., 1996) is described as follows (Equation 5):
Where, is status of cell at a time t + 1, is transitional probability matrix, and is status of cell at the time t.
Cellular Automata (CA) incorporates the spatial component, while adding direction to the spatial modeling using a combination of methods such as Markov Chain, Multi-Criteria Evaluation (MCE), Multi-Objective Land Allocation (MOLA), etc. (Mondal et al., 2020). In the present study, CA-Markov analysis was carried out as a three-step process. The first step includes the determination of transition through spatial overlay analysis of LULC images from 2005 and 2020, wherein the transition probability matrix and transfer areas are estimated. The second step includes the determination of CA filters based on standard kernel size. The standard 5*5 kernel size used in the study allowed for gains in individual categories to occur in close proximity to pre-existing categories. The third step is determining the starting point and the number of CA iterations required, where the LULC image of 2020 is taken as a starting point and 15 iterations were carried out to simulate the LULC for the year 2035. IDRISI software was used for predicting urban growth.
Five parameters were used as driving factors in the urban growth modeling process: (a) Distance from roads, (b) Distance from high-density residential areas, (c) Distance from medium-density residential areas, (d) Distance from low-density residential areas, and (e) Slope. The distance from road and residential areas were estimated using Euclidian distance tool, while the slope percentage was estimated from DEM. In the distance from road and residential layers, the 0–500 m, 500 m - 1000 m and 1,000 m - 1500 m buffers were considered as highly suitable, moderately suitable and marginally suitable, respectively for LULC conversion. In the slope layer, 0–3, 3–9% and 9–15% classes were considered as highly suitable, moderately suitable and marginally suitable areas, respectively for LULC conversion. Above criteria were built based on ground evidence and interactions with field officials. The underlying assumption for urban growth within residential areas is that the density of built-up areas follows a steady transition, where low-density built-up gradually converts to medium-density and medium-density gets converted into high-density based on various urban growth indicators. However, any change in Government policy or interventions by an individuals or institutions could either interrupt or accelerate the ongoing urbanization processes.
a. LULC scenarios: LULC change is one among the key indicators used to assess how urbanization affects runoff in the planning region. In the present study, initially, a ‘Baseline Scenario’ (Scenario-1) is generated which considers the LULC of the year 2020. LULC classes were defined by OBIA classification and then runoff coefficients were assigned to each LULC class. Similarly, a ‘Past Scenario’ (Scenario-2) was defined based on LULC classes for the year 2005. Scenario-3, the ‘Severe Scenario’, which considers the future LULC for the year 2035, has also been analyzed where the LULC classes were predicted by CA-Markov modeling. The Scenario-4, the ‘Green Scenario’ was developed by identifying the suitable areas for GI according to the “Model Building Bye-Laws, 2016” by the Greater Cochin Development Authority (Model Building Bye-Laws, 2016), which recommends that 30% of the residential areas and 20% of vacant land can be converted into GI to maintain the city’s green environment. Five criteria were considered for GI suitability analysis, namely (i) Modified Normalized Difference Impervious Surface Index (MNDISI), (ii) Distance from waterbody, (iii) Slope, (iv) Distance from road, and (v) Distance from residential areas. MNDISI represents the percentage of impervious surface present within study area.
GI suitability analysis was carried out using the Analytic Hierarchy Process (AHP) (Saaty, 1994) to identify suitable areas for UGS. Initially, a GIS database was developed with five parameters, namely, distance from roads, distance from water bodies, distance from residential areas, slope and MNDISI layers. The distance from roads, distance from water bodies, distance from residential areas were prepared from high-resolution satellite images and using buffer distance tool; the slope and MNDISI layer were generated from ALOS PALSAR DEM and Landsat-8 satellite image, respectively. The criteria maps were classified into five suitability classes, namely, (i) Suitability class-1: highly suitable, (ii) Suitability class-2: moderately suitable, (iii) Suitability class-3: marginally suitable, (iv) Unsuitable class-1: marginally not suitable and (v) Unsuitable class-2: least suitable. AHP table was prepared and suitable areas for GI were identified for the assessment of green scenario using ArcGIS software. Figure 3 shows the overall methodology for GI suitability analysis.
Normalized Difference Impervious Surface Index (NDISI) method proposed by Xu (2010) uses Visible, Near-Infrared (NIR), Thermal Infrared (TIR), and Shortwave Infrared (SWIR) bands (Equation 6):
Where Landsat Enhanced Thematic Mapper (ETM) bands 4, 5, and 6 are NIR, SWIR1, and TIR bands, respectively; while the visible band (VIS1) is one of the ETM bands 1, 2, or 3. NDISI can range between −1 and + 1. The above equation is adjusted to generate MNDISI for defining impervious surfaces from background characteristics (Sun et al., 2017) as given in Equation 7. MNDISI is utilized in this study to assess the imperviousness of built-up surfaces and UGS suitability analysis.
Where, Modified Normalized Difference Water Index (MNDWI) is computed as given in Equation 8.
AHP method was used for the multi-criteria evaluation (MCE) of green scenarios. In the AHP method, the consistency ratio (CR) aids to determine whether the evaluation is sufficiently consistent. The maximum acceptable threshold of CR is 10% and if it is not met, the adjustments are made to ensure consistency. In the present study, the CR of AHP table was estimated as 0.003, which is within the acceptable range as defined by Saaty and Tran (2007).
In the present study, the LULC maps were prepared for the years 2005 and 2020 with thirteen classes as per Atal Mission for Rejuvenation and Urban Transformation (AMRUT) Design and Standards (MoUD, 2016) using the OBIA method and followed by contextual refinement. From this analysis, it is deduced that percentage of UGS within the city have reduced from 50% in 2005 to 36% in 2020. The impervious areas had a significant increase during this period as the area under apartments have increased from 0.65% (2005) to 2.8% (2020), roads from 3.77% (2005) to 4.68% in (2020), high density residential from 21.46% (2005) to 30.96% (2020), and medium density residential from 9.22% (2005) to 13.94% (2020). Figure 4 shows the LULC for years 2005 and 2020.
‘Severe Scenario’ was developed based on urban growth modeling principles with an understanding that if ‘business as usual’ continues then unregulated urbanization will further affect the UGS, vacant lands, water bodies, etc. Hence, CA-Markov based urban growth modeling was carried out with LULC data of year 2005 and 2020 as past trends and projections have been made for the year 2035. Figure 5 shows various parameters used for urban growth modeling (Figures 5A–6E) and the projected LULC for the year 2035 (Figure 5F). The comparison between LULC-2020 and LULC-2035 shows a further decrease in UGS to 34% of the study area. The built-up classes have shown further increase such as apartments from 2.83 to 3.57%, high-density residential areas from 21.46 to 30.96%, and medium-density residential from 13.94 to 14.1%. Similarly, the grassland and tree-clad area, vacant land and water bodies are predicted to decrease further in subsequent years. During year 2020 to 2035, the UGS (grass and tree-clad area) has further decreased to 34%; high-density residential area has increased from 33.7 to 35.4%, while medium-density residential area has increased from 13.9 to 14.1%. Table 2 shows the geographic area under various LULC classes for the years 2005 and 2020 and the projected LULC for the year 2035.
Figure 5. Parameters used for urban growth modelling and the projected LULC: (a) density from low density residential, (b) distance from high density residential, (c) distance from medium density residential, (d) distance from road, (e) slope, and (f) projected LULC.
Figure 6. Various parameters used for UGI suitability analysis and UGS suitability map: (a) NDISI, (b) distance from road, (c) slope, (d) distance from high density residential, (e) distance from waterbody and (f) UGS suitability map.
The green scenario was built based on LULC characteristics of the study area. MCE-based evaluation of various parameters, namely, (i) MNDISI, (ii) distance from water bodies, (iii) slope, (iv) distance from roads, and (v) distance from residential areas were considered for green scenario prediction. These GIS layers were used to prepare GI suitability map. The weights for each criterion were determined based on MCE evaluation. Table 3 shows the results obtained from AHP-based MCE analysis and UGS suitability analysis. The weights are assigned to each criteria layer based on MCE analysis viz., MNDISI (48%), distance from road (29%), distance from residential areas (11%), distance from water body (7%), and slope (5%). To derive the UGS suitability map, a weighted overlay of the input parameters was carried out. The final suitability map is classified into five classes and is assigned the ranking as (i) Suitability class-1: highly suitable, (ii) Suitability class-2: moderately suitable, (iii) Suitability class-3: marginally suitable, (iv) Unsuitable class-1: marginally not suitable and (v) Unsuitable class-2: least suitable.
Three green scenarios were developed to enhance the green spaces according to “Model Building Bye-Laws” (Model Building Bye-Laws, 2016). Figure 6 shows various parameters used for GI suitability analysis and UGS suitability map. Figure 7 shows the MCE based GI suitability analysis and the result obtained are as follows: (a) Green scenario-1: vacant land falling in the suitability class-1 is selected as most suitable area for the creation of GI. Accordingly, 16% of vacant land falls in the suitability class-1, (b) Green scenario-2: vacant land falling in suitability class-2 is considered as suitable places for GI. The result showed that 33% of vacant land are falling under suitability class-2, and (c) Green scenario-3: It is created with LULC classes of vacant land falling in the suitability class-3. The result showed that 36.4% of vacant land falls under green scenario-3.
Figure 7. MCE based GI suitability analysis: (A) Green scenario-1, (B) Green scenario-2, (C) Green scenario-3.
The peak runoff for various scenarios was estimated using the rational method (Table 4). The composite runoff coefficient for various scenario was estimated and multiplied with rainfall intensities and area of the watershed as per rational method.
• Past scenario: Based on the LULC map for 2005, the composite runoff coefficient computed for the past scenario is 0.320 and the peak runoff is computed for the return period of 2-year, 5-year, 10-year, 30-year, 50-year, and 100-year return periods and the rainfall durations of 3-h, 6-h, 12-h, and 24-h.
• Baseline scenario: LULC map of the year 2020 served as baseline year and the composite runoff coefficient is computed as 0.407. ‘Baseline scenario’ driven peak runoff is computed for 3-h, 6-h, 12-h, and 24-h storm events for the 2-year, 5-year, 10-year, 30-year, 50-year, and 100-year return periods. In comparison to the ‘Past scenario’, the peak runoff computed for the baseline scenario showed a significant increase due to increased impervious surfaces and decreased water bodies and green spaces, and consequently, the composite runoff coefficient has increased from 0.320 to 0.407. Under this scenario, the peak runoff has significantly increased, e.g., peak runoff has increased from 6.15 cumecs to 7.82 cumecs for a 3-h rainfall event and for the return period of 2-year, which has increased the flood risk in the baseline scenario by 27% as compared to the ‘Past Scenario’.
• Severe Scenario: Peak runoff is computed to predict the SWR for the ‘severe scenario’ based on projected LULC for the year 2035 prepared through CA-Markov modeling. The estimated runoff coefficient for the severe scenario is 0.414. The composite runoff coefficient computed has increased from 0.407 (baseline scenario) to 0.414. In comparison to the peak runoff estimated for the baseline scenario for 3-h rainfall events with a 2-year return period, the peak runoff has increased from 7.82 cumecs to 7.93 cumecs, which increases the risk of flooding by 29% for 3-hourly rainfall event.
• Green Scenario: The green scenario is generated based on LULC and green spaces suitability maps. Model Building Bye-Laws (2016) states that 30% of the residential area and 20% of vacant land can be transformed into UGS in the city. It is observed that by increasing the green spaces according to above guidelines can reduce the peak runoff. The computed composite runoff coefficient for the ‘Green scenario-1’ is 0.352. In comparison to the baseline and severe scenarios, green scenario has shown a significant decrease in the peak runoff. In the green scenario, the computed peak runoff using the rational method has reduced from 7.82 cumecs to 6.81 cumecs, while in comparison to baseline scenario, it has decreased from 7.93 cumecs to 6.81 cumecs.
Rapid urbanization processes have altered the urban hydrological characteristics of Cochi city alike to other coastal cities in India and other parts of the world. In this study, the LULC transition in Kochi city during 15 years (2005–2020) was examined using high-resolution satellite data to assess the impact of urbanization on peak runoff. In this process, the LULC characteristics of study area were examined using OBIA approach. The growth prediction of urban surfaces was analyzed using CA-Markov based technique. Verburg et al. (2004) classified the probable driving factors for urban expansion into five broad categories viz., environmental characteristics, social factors, spatial neighborhood variables, economic factors, and spatial policies. In this study, urban growth modeling principles were followed with an understanding that if ‘business as usual’ continues then unregulated urbanization will further affect the UGS, vacant lands, water bodies, etc. Accordingly, five parameters were considered as driving factors in the urban growth modeling process: (a) Distance from roads, (b) Distance from high-density residential areas, (c) Distance from medium-density residential areas, (d) Distance from low-density residential areas, and (e) Slope. The underlying assumption for urban growth modeling as followed in this study was also that the density of built-up areas follows a steady transition, where the low-density built-up gradually converts to medium-density and medium-density gets converted into high-density based on various urban growth indicators. However, any change in Government policies or interventions by an individuals or institutions could either interrupt or accelerate the ongoing urbanization processes.
Assessment of storm water runoff (SWR) was carried out in four scenarios, i.e., baseline, past, severe, and green, using urban growth and GI driven simulations. The ‘baseline scenario’ considered the LULC of the year 2020, ‘past Scenario’ was defined based on LULC classes for the year 2005. Scenario-3, the ‘severe scenario’, which considered future LULC for the year 2035, has also been analyzed where the LULC classes were predicted by CA-Markov modeling. Scenario-4, the ‘Green Scenario’ was developed by identifying the suitable areas for GI according to “Model Building Bye-Laws” (Model Building Bye-Laws, 2016), which recommends that 30% of residential areas and 20% of vacant land can be converted into GI to maintain the city’s green environment.
The decline in UGS surfaces is observed in this study from 50% in 2005 to 36% in 2020 and to 34% as per predicted LULC for the year 2035. The importance of GIS-based analyses is emphasized in several studies which encompasses the multi-scalar aspects of GIs and as the nature-based solutions are still not mainstreamed in planning and governing practices of global cities (Yazar et al., 2023). Hence, in order to promote the UGS and improve the hydrological regime of urbanized surfaces, multi-criteria based GI suitability analyses was carried out in this study. Five criteria were considered for GI suitability analysis, namely (i) Modified Normalized Difference Impervious Surface Index (MNDISI), (ii) Distance from waterbody, (iii) Slope, (iv) Distance from road, and (v) Distance from residential areas. MNDISI represents the percentage of impervious surface present within study area. The study indicates that 16% (7.82 cumecs to 6.81 cumecs) reduction in peak runoff is estimated as compared to baseline scenario and 18% (7.93 cumecs to 6.81 cumecs) reduction in peak runoff is estimated as compared to severe scenario.
Li et al. (2020) evaluated the impact of existing GIs in Ghent, Belgium to reduce SWR. The results showed forests had the least impact on SWR reduction, whereas grasslands have the highest. The implementation potential of GIs in urban neighborhoods was evaluated by Uribe et al. (2022). They emphasized that the implementation of permeable pavement, infiltration trenches, and street planters reduced SWR the most in residential areas, whereas only permeable pavements had the capacity to significantly reduce runoff in industrial regions. Our study however, has not considered the impact of different types of GIs on SWR reduction. While city managers and environmentalists stress the importance of GIs in a typical urban setting; the population growth and the growing needs of local population lead to “infill,” which raises the built environment’s density and “extension” to the nearby countryside. The hydrological impact of increasing imperviousness is mostly disregarded by such urban expansion. Therefore, this study aimed to analyze the impact of both GIs and urban expansion on SWR based on urban growth modeling and GI suitability analysis.
This study utilized remote sensing data to derive runoff coefficients for various scenarios to assess the impact of improving UGS in Cochin City to mitigate the urban flood risks. The green scenario is developed based on “Model Building Bye-Laws (2016)” recommended by the Greater Cochin Development Authority. The past scenario and baseline scenarios, generated from LULC maps of years 2005 and 2020, respectively showed the reduction of UGS from 50 to 36%. Further, the severe scenario generated from the projected LULC for the year 2035 predicts a further decrease in the UGS to 34%. Rational method-based estimation of peak runoff showed the increase of peak runoff in baseline and severe scenarios. In comparison to the past scenario, the peak runoff has increased by 27% in baseline scenario and 29% in the severe scenario. To mitigate the urban flood risk, the study proposed a green scenario, which focuses on increasing the GI in the study area to reduce the peak runoff and consequently the urban flood risk. The findings show a 16% reduction in peak runoff compared to the baseline and 18% reduction when compared to the severe scenario.
The human beings and the nature are together responsible for the flood tribulations in urban surfaces. The recent floods in Kochi City and other neighboring districts of Kerala, India have accelerated the need for its scientific understanding to improve physical and social resilience. Thorough knowledge of LULC and flood duration is essential for effective planning and management of flood-prone areas. The findings of this study depict the relationship of peak runoff and LULC characteristics and also suggest for reducing the urban flood risk through GIs which can be a valuable tool to planners, water managers, and policymakers to take appropriate measures. The implementation of GI through government plans and policies, and people’s participation for effective urban SWR management shall help in improved resilience of the city toward urban flood risks. Further studies can be carried out to find the type of GIs which can be promoted within study area. The immediate need is to identify the vulnerable zones based on the location of water bodies, natural drains, etc. and it has to be made as “no building zones.” Moreover, awareness has to be created through campaigns at the local level about the causative factors for the flood disasters. The wide range of management will help the Cochin city to be relieved from the risk of flood at every monsoon.
Publicly available datasets were analyzed in this study. This data can be found at: https://earthexplorer.usgs.gov/, https://search.asf.alaska.edu/#/, https://www.earthdata.nasa.gov/centers/gesdisc-daac.
AG: Conceptualization, Data curation, Formal analysis, Investigation, Methodology, Project administration, Resources, Software, Validation, Visualization, Writing – original draft, Writing – review & editing. PK: Conceptualization, Methodology, Project administration, Resources, Supervision, Writing – original draft, Writing – review & editing. KG: Conceptualization, Writing – original draft, Writing – review & editing.
The author(s) declare that no financial support was received for the research, authorship, and/or publication of this article.
The authors extend their gratitude to the Director, Indian Institute of Remote Sensing (IIRS) for constant encouragements towards exploring the applications of geospatial technology in understanding our environment. We also express our gratitude to United States Geological Survey, Google Earth, and Alaska Satellite facility for providing satellite data and DEM for the study area; and Goddard Earth Sciences Data and Information Services Centre for providing rainfall data. We deeply acknowledge the support rendered by the Kochi Municipal Corporation for providing information about the city. We acknowledge the efforts of Ms. Kakali Deka in formatting the manuscript at its early stage.
The authors declare that the research was conducted in the absence of any commercial or financial relationships that could be construed as a potential conflict of interest.
All claims expressed in this article are solely those of the authors and do not necessarily represent those of their affiliated organizations, or those of the publisher, the editors and the reviewers. Any product that may be evaluated in this article, or claim that may be made by its manufacturer, is not guaranteed or endorsed by the publisher.
Alaska Data Search Vertex (2024). Available at: https://search.asf.alaska.edu/#// (Accessed July 31, 2024).
Boerner, R. E., DeMers, M. N., Simpson, J. W., Artigas, F. J., Silva, A., and Berns, L. A. (1996). Markov models of inertia and dynamism on two contiguous Ohio landscapes. Geogr. Anal. 28, 56–66. doi: 10.1111/j.1538-4632.1996.tb00921.x
Brzoska, P., and Spāģe, A. (2020). From city-to site-dimension: assessing the urban ecosystem services of different types of green infrastructure. Land 9:150. doi: 10.3390/land9050150
Chandramouli, C. M. (2011). Provisional population totals. India: Office of the Registrar General & Census Commissioner.
Chen, W., Wang, W., Mei, C., Chen, Y., Zhang, P., and Cong, P. (2024). Multi-objective decision-making for green infrastructure planning: impacts of rainfall characteristics and infrastructure configuration. J. Hydrol. 628:130572. doi: 10.1016/j.jhydrol.2023.130572
Explorer, E. (2024). Available at: https://earthexplorer.usgs.gov. (Accessed July 31, 2024).
Goldstein, N. C., Candau, J. T., and Clarke, K. C. (2004). Approaches to simulating the “march of bricks and mortar”. Comput. Environ. Urban. Syst. 28, 125–147. doi: 10.1016/S0198-9715(02)00046-7
Gupta, K., Kumar, P., Pathan, S. K., and Sharma, K. P. (2012). Urban Neighbourhood green index–a measure of green spaces in urban areas. Landsc. Urban Plan. 105, 325–335. doi: 10.1016/j.landurbplan.2012.01.003
Harlan, T. (2021). Green development or greenwashing? A political ecology perspective on China’s green belt and road. Eurasian Geogr. Econ. 62, 202–226. doi: 10.1080/15387216.2020.1795700
Kerala: Three years and the ravages of climate change (2021). Available at: https://indianexpress.com/article/india/kerala/kerala-climate-change-floods-landslide-mining-land-use-7586423/ (Accessed July 31, 2024).
Kerala Floods 2019: 121 dead, 1,789 Houses Collapsed (2019). Available at: https://www.newsclick.in/kerala-floods-2019-121-dead-1789-houses-collapsed (Accessed July 31, 2024).
Khodadad, M., Aguilar-Barajas, I., and Khan, A. Z. (2023). Green infrastructure for urban flood resilience: a review of recent literature on bibliometrics, methodologies, and typologies. Water 15:523.
Kim, H. W., Kim, J. H., Li, W., Yang, P., and Cao, Y. (2017). Exploring the impact of green space health on runoff reduction using NDVI. Urban For. Urban Green. 28, 81–87. doi: 10.1016/j.ufug.2017.10.010
König, A., Sægrov, S., and Schilling, W. (2002). “Damage assessment for urban flooding,” in Proceedings of the Ninth International Conference on Urban Drainage. Portland, Oregon, USA.
Kuichling, E. (1889). The relation between the rainfall and the discharge of sewers in populous districts. Trans. Am. Soc. Civ. Eng. 20, 1–56. doi: 10.1061/TACEAT.0000694
Li, L., Van Eetvelde, V., Cheng, X., and Uyttenhove, P. (2020). Assessing stormwater runoff reduction capacity of existing green infrastructure in the city of Ghent. Int. J. Sustain. Dev. World Ecol. 27, 749–761. doi: 10.1080/13504509.2020.1739166
Mobilia, M., Longobardi, A., and Sartor, J. (2014). “Impact of green roofs on stormwater runoff coefficients in a Mediterranean urban environment,” in Recent Advances in Urban Planning, Sustainable Development and Green Energy. Florence, Italy. 100–106.
Model Building Bye-Laws (2016). Town and country planning organization. Ministry of Urban Development, government of India. New Delhi, India, 111–119.
Mondal, M. S., Sharma, N., Kappas, M., and Garg, P. K. (2020). CA Markov modelling of land use land cover change predictions and effect of numerical iterations, image interval (time steps) on prediction results. Int. Arch. Photogrammetry Remote Sensing Spatial Inform. Sci. XLIII-B3-2020, 713–720. doi: 10.5194/isprs-archives-XLIII-B3-2020-713-2020
MoUD (2016). Formulation of GIS based master plans for AMRUT cities design and standards. Town and Country Planning Organization, Ministry of Housing and Urban Affairs, Government of India. Available at: https://amrut.gov.in/writereaddata/designandStandards_AMRUT.pdf (Accessed August 10, 2024).
Nagarajan, M., and Basil, G. (2014). Remote sensing-and GIS-based runoff modelling with the effect of land-use changes (a case study of Cochin corporation). Nat. Hazards 73, 2023–2039. doi: 10.1007/s11069-014-1173-9
Nandakrishnan,, and Prasad, P. R. C. (2024). Geoscape characterization of Ashtamudi, Sasthamkotta, and Vembanad Ramsar sites in Kerala, India. Water Res. 51, 462–474. doi: 10.1134/S009780782360273X
NASA EOSDIS Distributed Active Archive Centers (DAACs) (2024). Available at: https://earthdata.nasa.gov/eosdis/daacs (Accessed July 31, 2024).
Ramachandra, T. V., and Kumar, U. (2008). Wetlands of greater Bangalore, India: automatic delineation through pattern classifiers. Electronic Green J. 1:2. doi: 10.5070/G312610729
Record rainfall for November in Kerala this year (2021). Available at: https://timesofindia.indiatimes.com/city/kochi/record-rainfall-for-november-in-state-this-year/articleshow/88097105.cms (Accessed July 31, 2024).
Saaty, T. L. (1994). Fundamentals of decision making and priority theory with the analytic hierarchy process, Pittsburgh, PA: RWS Publications.
Saaty, T. L., and Tran, L. T. (2007). On the invalidity of fuzzifying numerical judgments in the analytic hierarchy process. Math. Comput. Model. 46, 962–975. doi: 10.1016/j.mcm.2007.03.022
Sanchez, F. G., and Govindarajulu, D. (2023). Integrating blue-green infrastructure in urban planning for climate adaptation: lessons from Chennai and Kochi, India. Land Use Policy 124:106455. doi: 10.1016/j.landusepol.2022.106455
Song, K., Seok, Y., and Chon, J. (2023). Nature-based restoration simulation for disaster-prone coastal area using green infrastructure effect. Int. J. Environ. Res. Public Health 20:3096. doi: 10.3390/ijerph20043096
Sowmya, K., John, C. M., and Shrivasthava, N. K. (2015). Urban flood vulnerability zoning of Cochin City, southwest coast of India, using remote sensing and GIS. Nat. Hazards 75, 1271–1286. doi: 10.1007/s11069-014-1372-4
Sun, Y., Wendi, D., Kim, D. E., and Liong, S. Y. (2019). Deriving intensity–duration–frequency (IDF) curves using downscaled in situ rainfall assimilated with remote sensing data. Geosci. Lett. 6:17. doi: 10.1186/s40562-019-0147-x
Sun, Z., Wang, C., Guo, H., and Shang, R. (2017). A modified normalized difference impervious surface index (MNDISI) for automatic urban mapping from Landsat imagery. Remote Sens. 9:942. doi: 10.3390/rs9090942
Thanapura, P., Helder, D. L., Burckhard, S., Warmath, E., O’Neill, M., and Galster, D. (2007). Mapping urban land cover using QuickBird NDVI and GIS spatial modelling for runoff coefficient determination. Photogramm. Eng. Remote Sens. 73, 57–65. doi: 10.14358/pers.73.1.57
Thekkeyil, A., George, A., Abdurazak, F., Kuriakose, G., Nameer, P. O., Abhilash, P. C., et al. (2023). Land use change in rapidly developing economies—a case study on land use intensification and land fallowing in Kochi, Kerala, India. Environ. Monitor. Assess. 195:1089. doi: 10.1007/s10661-023-11731-7
Uribe, C. H. A., Brenes, R. B., and Hack, J. (2022). Potential of retrofitted urban green infrastructure to reduce runoff-a model implementation with site-specific constraints at neighborhood scale. Urban For. Urban Green. 69:127499. doi: 10.1016/j.ufug.2022.127499
USEPA (2014). Enhancing sustainable communities with green infrastructure; a guide to help communities better manage storm water while achieving other environmental, public health, social, and economic benefits; USEPA: Washington. USA: DC.
Verburg, P. H., Van Eck, J. R. R., de Nijs, T. C., Dijst, M. J., and Schot, P. (2004). Determinants of land-use change patterns in the Netherlands. Environ. Plan. B 31, 125–150. doi: 10.1068/b307
Walsh, S. J., McCleary, A. L., Mena, C. F., Shao, Y., Tuttle, J. P., González, A., et al. (2008). QuickBird and Hyperion data analysis of an invasive plant species in the Galapagos Islands of Ecuador: implications for control and land use management. Remote Sens. Environ. 112, 1927–1941. doi: 10.1016/j.rse.2007.06.028
Wang, S. W., Munkhnasan, L., and Lee, W. K. (2021). Land use and land cover change detection and prediction in Bhutan's high altitude city of Thimphu, using cellular automata and Markov chain. Environ. Challenges 2:100017. doi: 10.1016/j.envc.2020.100017
Xu, H. (2010). Analysis of impervious surface and its impact on urban heat environment using the normalized difference impervious surface index (NDISI). Photogramm. Eng. Remote Sens. 76, 557–565. doi: 10.14358/pers.76.5.557
Yazar, M., Daloglu Cetinkaya, I., Iban, M. C., and Bilgilioglu, S. S. (2023). The green divide and heat exposure: urban transformation projects in Istanbul. Front. Environ. Sci. 11:1265332. doi: 10.3389/fenvs.2023.1265332
Zhang, B., Li, N., and Wang, S. (2015). Effect of urban green space changes on the role of rainwater runoff reduction in Beijing, China. Landsc. Urban Plan. 140, 8–16. doi: 10.1016/j.landurbplan.2015.03.014
Zhang, B., Xie, G., Zhang, C., and Zhang, J. (2012). The economic benefits of rainwater-runoff reduction by urban green spaces: a case study in Beijing, China. J. Environ. Manag. 100, 65–71. doi: 10.1016/j.jenvman.2012.01.015
Keywords: green infrastructure, urban growth, storm water runoff, rational method, CA-Markov
Citation: George AL, Kumar P and Gupta K (2025) Impact assessment of green infrastructure and urban growth on stormwater runoff through geospatial modeling. Front. Water. 6:1489315. doi: 10.3389/frwa.2024.1489315
Received: 31 August 2024; Accepted: 09 December 2024;
Published: 07 January 2025.
Edited by:
Abbas Roozbahani, Norwegian University of Life Sciences, NorwayReviewed by:
G. N. Tanjina Hasnat, University of Chittagong, BangladeshCopyright © 2025 George, Kumar and Gupta. This is an open-access article distributed under the terms of the Creative Commons Attribution License (CC BY). The use, distribution or reproduction in other forums is permitted, provided the original author(s) and the copyright owner(s) are credited and that the original publication in this journal is cited, in accordance with accepted academic practice. No use, distribution or reproduction is permitted which does not comply with these terms.
*Correspondence: Pramod Kumar, cHJhbW9kdmFpc2hAZ21haWwuY29t
Disclaimer: All claims expressed in this article are solely those of the authors and do not necessarily represent those of their affiliated organizations, or those of the publisher, the editors and the reviewers. Any product that may be evaluated in this article or claim that may be made by its manufacturer is not guaranteed or endorsed by the publisher.
Research integrity at Frontiers
Learn more about the work of our research integrity team to safeguard the quality of each article we publish.