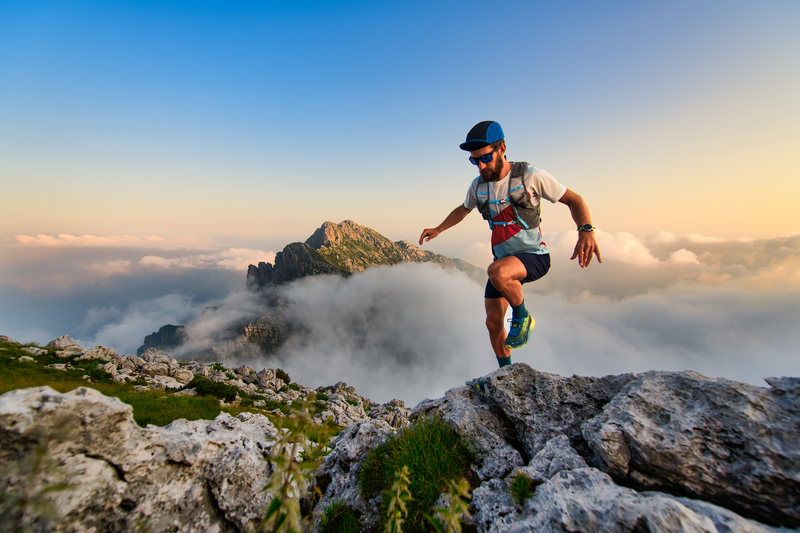
94% of researchers rate our articles as excellent or good
Learn more about the work of our research integrity team to safeguard the quality of each article we publish.
Find out more
ORIGINAL RESEARCH article
Front. Water , 29 November 2024
Sec. Water Resource Management
Volume 6 - 2024 | https://doi.org/10.3389/frwa.2024.1451648
The Paraguay River Basin forms part of the La Plata River Basin in South America. Its streamflow is significantly attenuated by a high evapotranspiration rate, very gentle slopes and the presence of a vast wetland known as the Pantanal. Modeling the hydrology of watersheds in which the flood pulse is affected by the presence of large floodplains can pose issues for hydrological models that do not account for spatial complexity and simplify water routing using linear assumptions. The new version of the Soil and Water Assessment Tool, known as SWAT+, routes water using variations of the kinematic wave model. However, with the inclusion of connectivity and Landscape Units, SWAT+ provides more flexibility in terms of representing the hydrologic fluxes in the watershed. The main objective of this study is to use the concept of Landscape Units and connectivity to represent the water exchanges between uplands, floodplains and channels. We developed code routines to (1) temporally retain surface and subsurface water coming from the upland into the floodplain, by assuming a reservoir-like floodplain behavior, and (2) represent overbank flow, aiming to fully simulate the interactions between channels and floodplains. The model was calibrated based on monthly discharge for the period 1990 to 2020. The simulated average annual water storage in the floodplains of the Paraguay River is ~108.81 mm accounting for 56.5% of the total annual discharge at the outlet. Furthermore, ~61% of the total annual surface runoff in the Paraguay River Basin flows through the floodplains. Results indicate that the model is able to capture the hydrologic regime in the Paraguay River representing an improvement of SWAT+.
When expansive floodplains are present in river basins, they exert a profound influence on hydrological dynamics (Bates et al., 2000). Typically found in gently sloping terrains, floodplain zones serve as conduits for flood waves emanating from the main channel, facilitating water storage within their area. This storage engenders significant temporal disparities between downstream and upstream hydrographs (Fleischmann et al., 2016). Consequently, floodplains offer a multitude of ecosystem services, including flood mitigation, sediment deposition, and preservation of water quality (Alsdorf et al., 2007). Moreover, they impact the carbon and nitrogen cycles, thus exerting an important role in regulating greenhouse gas emissions (dos Santos Pinto et al., 2020).
Modeling the hydrology of watersheds in which the flood pulse is greatly affected by topography and floodplains can pose several issues for hydrological models that do not account for spatial complexity and simplify water routing using linear assumptions (Fenton, 2019). In watersheds with extensive floodplains, the flow paths of water become very complex. Water can flow not only through the main channel but also across the floodplain and through various interconnected channels (Fleischmann et al., 2016), which substantially impacts flow velocities in the river network (Wong and Laurenson, 1983; Fenton, 2019).
The Soil and Water Assessment Tool model (SWAT; Arnold et al., 1998) is not exempt from this limitation. First, SWAT is rigid in terms of connectivity and spatial discretization of the watershed (Bieger et al., 2017). Secondly, although SWAT provides to the modeler two routing methods (Variable Storage Capacity and Muskingum) that are suitable for various river basins worldwide (Fenton, 2019; Abbaspour et al., 2015), they often fail to accurately estimate flow discharge in watersheds where hydrology is influenced by extensive floodplains. Some research work have detailed these limitations, proposing new routing modules that are able to represent the floodplain effect on a river basin (Rahman et al., 2016; Liu et al., 2008). Another example is the work by Guilhen et al. (2022), who introduced a different flow routing method for SWAT based on the findings of Santini (2020).
The SWAT+ model, which is the result of revising and restructuring the SWAT code (Bieger et al., 2017), was released in 2017. It uses the same routing methodologies (Variable Storage and Muskingum), channel geometry, and flow velocity equations as SWAT. However, the inclusion of connectivity between spatial objects and Landscape Units (LSUs) provides far more flexibility than SWAT when representing the hydrologic processes of the study area (Bieger et al., 2017). This is because the delineation of landscape units enables a more accurate depiction of spatio-temporal dynamics of the studied watershed (Wagner et al., 2022). When defining the uplands and floodplains, the default SWAT+ configuration routes water from the upland to the floodplain and from the floodplain to the channel (Bieger et al., 2019). However, water flowing from the channel to the floodplain is not yet represented, posing problems for the current SWAT+ to deal with watersheds in which the hydrology is floodplain dominated.
The Paraguay River Basin, located in South America, is one example of a river system in which the hydrology is highly affected by floodplains (do Nascimento et al., 2023). This is because of the Pantanal, the largest wetland in the world (Paz et al., 2011), and the Chaco biome region (Bravo et al., 2012). The Paraguay River hydrology has been studied before due to its importance on biodiversity (Garcia, 2021) and its role in the hydrology of La Plata River Basin (Berbery and Barros, 2002). Examples of previous research include: the dynamics of inundation in the Pantanal (Pereira et al., 2021), river-floodplain interactions (do Nascimento et al., 2023; Paz et al., 2011; Bravo et al., 2012), and model development (Schrapffer et al., 2020; Pontes et al., 2017; Collischonn et al., 2007).
In addition, current and future human activities in the Paraguay River Basin, such as dam construction (Jardim et al., 2020), land use change (de Oliveira Roque et al., 2021; Ferreira Barbosa et al., 2022), water pollution (Camargo et al., 2022; Viana et al., 2022), and climate change (Marengo et al., 2021; Marques et al., 2021; Findlay, 2022), threaten the biodiversity and the hydrologic cycle of the region suggesting severe consequences not only for the conservation of this natural area but for societies benefiting from the Paraguay River ecosystem (Metcalfe and Menone, 2020).
To respond to these challenges, and to enhance the capabilities of the SWAT+ model, we developed code routines to (1) temporally retain surface and subsurface water coming from the uplands into the floodplain by assuming a reservoir-like floodplain behavior and (2) represent overbank flow, aiming to fully simulate the interactions between channels and floodplains. Overbank flow occurs when the volume of water entering the stream exceeds the channel's bankfull capacity, resulting in overflow onto the floodplain. We utilized SWAT+ with its new Landscape Unit concept to assess whether the model can satisfactorily represent discharge, and the physical processes in the Paraguay River Basin. Additionally, we provide new model outputs to offer insights into the hydrology of the Paraguay River.
The Paraguay River Basin is an international watershed that covers Brazil (33.4%), Paraguay (33.3%), Argentina (16.7%), and Bolivia (16.6%) (Krepper et al., 2006). It has a surface area close to 1,100,000 km2. The Paraguay River originates in the Brazilian plateau and flows southwards across very flat areas until it reaches the Parana River. The total length of the river is ~2,700 km (Figure 1). The annual mean rainfall and discharge at the outlet are respectively 1,120 mm/year (Su and Lettenmaier, 2009) and 3,210 m3/s corresponding to 160.4 mm/year.
Figure 1. (A) The Paraguay River Basin location in South America; (B) The modeled area with its main rivers and hydrologic gauges.
Rainfall patterns show a clear seasonality: wet periods occur mostly during October to March and dry seasons from April to September due to the South America monsoon system (Su and Lettenmaier, 2009). Nearly all of the rainfall contribution comes from the northeast area of the basin as the west area is mostly dry throughout the year.
Around 13% of the Paraguay River Basin is covered by a vast swamp known as the Pantanal. The presence of this extensive wetland, combined with its unique topographical features, yields a heightened rate of evapotranspiration, resulting in a notably diminished rainfall-runoff relationship (Berbery and Barros, 2002). These hydrological processes significantly influence streamflow characteristics particularly the timing of flooding events.
The streamflow exhibits pronounced seasonality, with peak values occurring during the austral winter (Figure 2). This suggests a lag of ~4–6 months between peak rainfall and discharge values (Collischonn et al., 2001).
Figure 2. Monthly mean rainfall and discharge values. The plot shows the effect of the Pantanal wetland on streamflow values. Discharge values correspond to the Porto Murtinho gauge. Rainfall values correspond to the monthly aggregation for the entire basin.
With an area of ~140,000 km2, the Pantanal is the largest tropical wetland (do Nascimento et al., 2023). The hydrological processes in the Pantanal are very complex as it is characterized by dynamic rivers, featuring both active and abandoned alluvial fans, which contribute to its diverse landscape (Jardim et al., 2020). As a result, the Pantanal represents a mosaic of fluctuating flooded habitats, such as permanent lakes, water-filled depressions, and seasonal small lakes that periodically dry out (Paz et al., 2011).
The Pantanal provides many ecosystem services as its lagging effect on streamflow balances the water level of the Paraguay River, and thus affecting its navigability (Hamilton, 1999; Assine et al., 2016), protecting important cities from flooding (Hamilton and McClain, 2002), and regulates biogeochemical processes (Ivory et al., 2019). Moreover, activities such as fishing (Shrestha et al., 2002), and tourism (Tortato and Izzo, 2017) are dependent on the hydrologic functions of the Pantanal.
The new version of the Soil and Water Assessment Tool (SWAT+) is a completely revised version of SWAT. The code is now object oriented which facilitates the management, maintenance and future developments within the SWAT+ community (Bieger et al., 2017). One example is the new groundwater flow module developed by Bailey et al. 2020, which aims to improve the representation of groundwater processes. SWAT+ has been applied in different watersheds at different scales, proving that the model is capable to correctly represent the hydrologic cycle (Barresi Armoa et al., 2023; Wagner et al., 2022; Leone et al., 2024).
SWAT+ uses the concept of Hydrologic Response Units (HRUs) as the smallest unit of discretization of the watershed, where all model calculations are done. However, one major improvement of SWAT+ is the possibility to combine the floodplain delineation with the HRUs in order to built Landscape Units (LSUs). Landscape Units consists of a collection of HRUs. It can represent a sub-basin, a floodplain, upland or a grid cell containing multiples HRUs. To sub-divide the sub-basins into uplands and floodplains, the modeler needs to input the digital elevation model. After the calculation of flow direction, flow accumulation and the channel delineation, the algorithm estimates the flooding areas based on the calculation of a coefficient that takes into account the topography and zones that may experience flooding during periods of high streamflow (Rathjens et al., 2016). Nevertheless, the delineation of LSUs is flexible allowing the modeler to define them based on their specific requirements (Schürz et al., 2022; White et al., 2022).
Another considerable difference between SWAT+ and SWAT are decision tables (Arnold et al., 2018). They allow to represent complex rule sets to determine its corresponding action. For example, if in a crop field the water stress is above a certain value, the action will be to irrigate a specific amount. Following the same logic, if a reservoir volume surpasses the emergency spillway volume, the action will be to release the excess of water until the reservoir volume returns to its normal operating value. SWAT+ is capable of handling different operations and actions, all of which are explained in Arnold et al. 2018. In this study, a decision table was built in order to model the volume of water returning from the floodplain to the channel.
To delineate the floodplain, we used a digital elevation model (DEM) with a spatial resolution of 500 m from the Shuttle Radar Topography Mission STRM database (Farr et al., 2007). The Slope Position method (Rathjens et al., 2016), is one of the algorithms used in SWAT+ to delimit uplands and floodplains. As Rathjens et al. 2016 demonstrated, the slope position provided good results when delineating the alluvial floodplains. Moreover, Guilhen et al. 2022 compared the slope position delineation method with the Soil Water Fraction at High Resolution (SWAF-HR; Parrens et al., 2017) to delimit the floodplain, and concluded that the slope position approach can result in a good spatial representation of the alluvial areas.
The slope position value σi is a relationship between the valley bottom elevation vi, which is the landscape where flow paths converge; ei the elevation of the grid cell i; and the ridge top elevation ri. The floodplain is then all the area adjacent to the valley bottom. Slope position values are between 0 (valley bottom) and 1 (ridge top). The complete description of the methodology can be found in Rathjens et al. (2016).
In SWAT+, the concept of floodplain and wetland are similar in terms of hydrologic conditions, such as high evapotranspiration rate, high soil water content, and low surface runoff.
Wetlands are modeled similar to reservoirs (Figure 3), thus, water can flow back to the channel depending on the wetland capacity to store water and the actual volume of water on the wetland. If the wetland is flooded, water can move back to the channel faster. If the wetland is not filled to capacity, it will move slower. To model this behavior, SWAT+ sets a principal and emergency volume using the respective depths and the wetland surface area as input values. In addition, the wetland's volume can vary through losses such as seepage and evapotranspiration. Volume gain occurs from rainfall, inflow coming as surface runoff and lateral flow from uplands, and overbank flow from the channel. The total volume stored in the wetland can be expressed as:
where Vstored is the total volume of water stored in the wetland; Vin is the volume of water coming into the wetland; Vout is the volume of water released from the wetland; Vflooded is the volume of water released from the wetland when it is flooded; and Vreturn is the overbank volume of water coming from the channel. Values are expressed in m3.
Figure 3. Wetland representation in SWAT+. Parameters dp_ps and dp_es correspond to depth to the principal spillway and depth to the emergency spillway respectively.
When the volume of water reaches the principal spillway volume, the release of water is calculated using the following equation:
When the current water volume exceeds the emergency volume, the excess of water above the emergency volume is released from the wetland, and the total release water flow rate is increased accordingly.
where ΔVtr is the total released volume of water; Vi is the actual volume of water; Vps is the volume to the principal spillway; Ves is the volume to the emergency spillway. Values are expressed in m3.
SWAT+ offers different options to control the wetland release. In this study, we chose to lag the flow according to the “days” option. The model will first calculate a threshold value according to the volume of water to the principal spillway or the volume of water to the emergency spillway.
When the option days is used, the release is based on drawdawn days, meaning that the flow above a modeler-defined threshold will take the n number of days to return to the channel. There are different cases on how SWAT+ will calculate the released volume of water depending on the file pointer (fp) option.
1. If case null is selected, the threshold value for the reservoir release calculation is zero.
2. If case “pvol” is selected the threshold value for the reservoir release calculation is:
where blo represents the threshold value; Vps represent the total volume to the principal spillway and C2 represent a release constant.
3. If case “evol” is selected, the threshold value for the reservoir release calculation is:
where blo represents the threshold value; Ves represent the total volume to the emergency spillway, and C2 represent a release constant, referred as const2 in SWAT+ decision tables.
The released flow is then calculated as:
where Vtr is the total released flow; Vs is the stored volume of water; blo is the threshold; and C represents the release constant referred as const in SWAT+ decision tables.
The equations used in this study belong to the “days” release option, all equations for different cases were coded in the res_hydro.f90 subroutine. SWAT+ code is open access and can be found in: https://github.com/swat-model/swatplus. More detail on decision tables can be found in Arnold et al. 2018.
SWAT+ assumes a trapezoidal channel shape as shown in Figure 4 (Neitsch and Arnold, 2009). The bottom width is calculated using the top width or bankfull width and depth as input. SWAT+ assumes a slope of 0.5 as it considers that the run to rise ratio zch equals 2. Assuming zch = 2 then,
Figure 4. Geometric shape of a channel including hydrologic processes in wetlands. ΔV corresponds to the exchanges of flood volumes from the channel to the floodplain and from the floodplain to the channel.
If Wbtm ≤ 0 then,
and,
Given a certain water level the width is calculated by:
The cross sectional area of flow Ach, wetted perimeter of the channel Pch, and the volume of water in the channel Vch are:
where Wbtm corresponds to the bottom width of the channel (m); Lch is the channel length (km); depth is the depth of the water filling the channel (m); depthbnkfull corresponds to the depth of water when filled to the top of the bank (m).
The model assumes a floodplain trapezoidal shape with the bottom width of the floodplain Wbtm,fld to be 5 times the channel's bankfull width Wbnkfull. The model sets the run to rise ratio of the floodplain zfld to 4, so the slope is 0.25.
When the water volume in the channel surpasses its capacity, additional water will flow out onto the floodplain area, this process is known as overbank flow. When water is flowing across the floodplain, the calculation of depth, cross sectional area, wetted perimeter, and total volume is the sum of channel and floodplain parameters:
If the peak rate is greater than the flow rate when the channel is at bankfull depth, overbank flow occurs. Assuming a triangular hydrograph, overbank volume can be calculated as the top of the triangle above bankfull.
Where Vob is the overbank flow in m3; Vi corresponds to the daily volume inflow in m3; velbf is the flow rate when the channel is at bankfull depth in m3/s; and tbase is the hydrograph base time in seconds.
The SWAT+ model precipitation data was acquired from the Climate Hazards group Infrared Precipitation with Stations CHIRPSv2, with a spatial resolution of 0.25° (Funk et al., 2015). CHIRPSv2 has been applied to study different hydrologic conditions, such as droughts (Marengo et al., 2021), and floods (Pereira et al., 2021). Cerón et al. 2020 assessed CHIRPSv2 dataset to study the spatio-temporal rainfall variability in the La Plata Region where our study area is located, and concluded that the dataset is useful on a large-scale basis. In addition Beck et al. 2017 evaluated 22 precipitation products indicating the good performance of CHIRPS to reproduce observed data across the Paraguay Basin. Maximum and minimum temperature and relative humidity data where obtained from the ERA5 dataset (Hersbach et al., 2020).
Monthly discharge in situ data was obtained from the HIDROWEB website managed by the National Water Agency of Brazil (ANA). The data is available at: https://www.snirh.gov.br/hidroweb/apresentacao for each gauge station.
The QSWAT+ interface was used to set up the model and run it at a daily time step, with a simulation period of 1987 to 2020 and three years of warm-up. The Paraguay River Basin was discretized into 305 sub-basins, 607 LSUs, and 6,225 HRUs. The total simulated area was ~631,300 km2. The Hargreaves method was used to calculate the evapotranspiration. Parameterization of the model was carried out using the hard calibration technique (Chawanda et al., 2020), keeping a correct representation of the physical processes in the watershed. We used the SPOTPY open source Python library (Houska et al., 2019) selecting the Dream algorithm and 3,000 model runs. For the present results, we show only the best model run based on the calculation of the following objective functions: (1) Nash-Sutcliffe Efficiency (Equation 20); (2) Percent Bias (Equation 21); (3) Correlation Coefficient (Equation 22); (4) and Kling-Gupta Efficiency (Equation 23). The performance of the model was evaluated based on the thresholds suggested by Moriasi et al. 2007.
where Qi,o and Qi,s correspond the observed and simulated values respectively; and represent the average observed and simulated values respectively; n is the total number of observations; α correspond to relationship between the standard deviations for simulated and observed data and β correspond to relationship between and .
The GRACE (Gravity Recovery and Climate Experiment) satellite mission, was designed to measure changes in Earth's gravity field by tracking variation in gravitational pull (Schmidt et al., 2008). GRACE provides information about water mass anomalies and its changes over time. Although GRACE data is coarse, it can offer a general overview and a qualitative assessment of how the hydrologic cycle is represented in the model (Ngo-Duc et al., 2007).
To obtain the TWSt from GRACE, we defined the coordinates of the Paraguay River Basin (-16.32 North; -58.94 West; -54.70 East; and -23.06 South). GRACE data is available from 2002, therefore we compared from 2002 to 2020 (final year of SWAT+ simulation period) and calculated the coefficient of determination (R2), a similar approach was done by Ngo-Duc et al. 2007 and Schrapffer et al. 2023.
The Total Water Storage (TWS) anomaly can be calculated in order to be compared to the data obtained from GRACE satellite mission. The anomaly of the total water storage is expressed as:
where TWSt is the total water storage value of the month t of the current year; corresponds to the long term average TWS; and δ is the standard deviation.
Figure 5 displays the distribution of the floodplain within each sub-basin in the Paraguay River Basin, obtained after running the slope position algorithm (Rathjens et al., 2016). The total floodplain area in the watershed is ~113,000 km2, with an average of 371 km2 and a standard deviation of 355 km2 per sub-basin. The DEM-based method delineates a theoretical floodplain, it provides the maximum floodable regions per sub-basin (Guilhen et al., 2022). Therefore, all sub-basins in the watershed have a floodable area. The resulting floodplain area constitutes 18% of the total simulated watershed area.
Figure 5. Spatial representation of the alluvial floodplains obtained by using the Slope Position Method. The brown zone corresponds to the Pantanal wetland. X and Y coordinates are in degrees.
We performed a hard calibration of the model against monthly streamflow observations at three gauges. The first step was to calibrate cn3_swf (soil water factor for the curve number condition III), latq_co (lateral flow coefficient) and perco (soil percolation coefficient) according to the Soil Vulnerability Index proposed by Thompson et al. 2020. Since the presence of the floodplains highly influences the hydrology of the basin, the parameters: (1) wetland depth to the principal (dp_ps) and (2) to the emergency (dp_es) spillway; (3) floodplain roughness coefficient number (fpn); (4) wetland evapotranspiration (evap); and (5) wetland soil infiltration (k), became very sensitive. Final calibrated parameters are shown in Table 1.
Table 2 lists the objective function values for the three hydrologic gauges. Figure 6 shows the monthly simulated and observed discharge for the three gauges. The SWAT+ model with the floodplain effect represents discharge considerable better than without the floodplain effect. Figure 7 displays a comparison between SWAT+ with and without the floodplain effect at Porto Murtinho hydrological station. Indeed, objective functions values for the SWAT+ setup without the floodplain effect were unsatisfactory. For example, in Porto Murtinho gauge the NSE value was -8.33, PBIAS 77.01, COR 0.12 and the KGE -0.58 for the entire simulation period. In SWAT+ with the floodplain effect, the time of concentration increases, providing a better estimation of discharge. The SWAT+ model without the floodplain systematically overestimates discharge, reaching values close to 12,000 m3/s during wet periods as shown in Figure 7.
Figure 6. Monthly simulated and observed discharge at (A) Caceres, (B) Porto Murtinho, and (C) Asuncion from 1990 to 2020. Calibration period: 1990–2010; Validation period: 2011–2020. Final parameter and objective function values are shown in Tables 1, 2, respectively.
Figure 7. Monthly discharge comparison between a SWAT+ model without floodplains and the SWAT+ model with floodplains from 1990 to 2020 at Porto Murtinho gauge. All parameters were set to the same values in both setups.
Calibration of the Paraguay River Basin, or any floodplain-dominated watershed, is not feasible without accounting for the floodplains. For instance, the surface runoff coefficient (surlag) parameter is adapted to watersheds where the time of concentration is reached in hours, causing discharge delays in terms of days. However, this approach is inadequate for basins similar to the Paraguay River, where the flood wave can take several months to reach the outlet. Moreover, forcing parameter optimization with unrealistic values can distort physical processes and lead to an inaccurate representation of hydrological dynamics across the watershed (Arnold et al., 2012).
Figure 8 shows the volume of water storage in the Paraguay River Basin. According to our results, maximum storage occurs in April and minimum storage in October. A similar pattern was reported by Schrapffer et al. 2023 after applying a high-resolution floodplain scheme adapted to the ORCHIDEE land surface model in the Pantanal, obtaining the maximum water storage in April. The total simulated annual average water storage in the Paraguay River Basin is 108.81 mm. This represents ~30% of the total discharge at Asuncion station. In addition, 61% of the surface runoff from the upland areas passes through the floodplain before reaching the main channel.
Figure 8. Average monthly water storage in the floodplains of the Paraguay River Basin. Shaded area indicates the range of monthly volumes of stored water in the floodplain landscape units defined in the SWAT+ setup.
The R2 obtained when comparing the TWS anomaly simulated and observed was 0.72 (Figure 9). The relatively strong correlation indicates that the simulations exhibit a monthly trend comparable to the observed data obtained by GRACE. This suggests that the model captures the evolution of water volume in the Paraguay River Basin. GRACE measures the total mass of water stored on and beneath the Earth's surface, therefore variations in groundwater storage are part of the TWS anomaly captured by GRACE. The TWS anomaly calculation in SWAT+ does not encompass changes in aquifer levels which could explain the remaining 28% of variance.
Figure 9. Comparison between the Total Water Storage Anomaly from GRACE and SWAT+, during the period 2002 to 2020.
Figures 10, 11 show the spatial distribution of the monthly average variation of the flood wave in the Paraguay River Basin. In the Pantanal, the presence of two bottlenecks produces an important damming effect on the magnitude, duration, and extent of floods (Assine et al., 2016). The northern bottleneck, known as Paraguay-Canzi (Assine et al., 2016; Stevaux et al., 2020), causes inundation of the upper Pantanal area from January to April (Figure 10). The flood wave spreads toward the south, reaching important lakes and plains (da Paz et al., 2014). The presence of the southern bottleneck (the Urucum; Assine et al., 2016; Stevaux et al., 2020) delays the flood wave and the flood inundation in the Pantanal reaches its maximum in June, at the same time the northern floodplains begin to drain (Figure 11). In the last months of the year, the Pantanal is dry and the summer rains will begin the inundation pattern.
Figure 10. Flood wave behavior in the Paraguay River Basin from January to June. Inundation begins in the north and moves southward, spreading across the Pantanal. The intensity of the blue color indicates the volume of surface and subsurface flow, with darker blue representing higher values.
Figure 11. Flood wave behavior in the Paraguay River Basin from July to December. The Pantanal is fully flooded in July, and the flood wave gradually moves southward, leaving the Pantanal dry by the end of the year. The intensity of the blue color indicates the volume of surface and subsurface flow, with darker blue representing higher values.
The connectivity between the upland, floodplain and channel systems, and their relationship with the flood pulse, is a key process to understand, as it significantly influences tropical wetlands (Stevaux et al., 2020). The flooding behavior in the Paraguay River Basin is a fundamental phenomenon that provides different ecological functions (Alho, 2008; Ivory et al., 2019), such as the temporal retention of surface water (Ivory et al., 2019), the maintenance of the characteristics of subsurface hydrology (Girard et al., 2003), nutrient cycling (Vourlitis et al., 2017), sequestration and retention of pollutants (de Oliveira Roque et al., 2021), the maintain of plant communities (Alho, 2008), and provides habitat for wildlife (Alho and Silva, 2012). Hence, it is imperative to represent the hydrological processes since there is a close link to the biota in alluvial wetlands.
The presence of the Pantanal wetland in the Paraguay River Basin can difficult the representation of hydrology in models due to its complex hydromorphological characteristics (Stevaux et al., 2020). As a consequence, several hydrologic models are coupled with one, two or three dimensional hydrodynamic models to provide finer representation of water routing (Paz et al., 2011; Yamazaki et al., 2011). Difficulties can arise from combining hydrologic with hydrodynamic models for large-scale applications, these can be related to the data requirements (which typically demands an excessive amount of time and effort), computational power and numerical instabilities (Bravo et al., 2012).
By applying the concept of Landscape Units to depict the upland-floodplain-channel processes, it is possible to represent the temporal water retention in the floodplains. Although the chosen approach remains simplified, as the model does not use a more physical approach to describe the flood wave and water exchanges between the floodplain and the channel, such as Saint-Venant equations or other approaches (Santini, 2020; Bravo et al., 2012), it provides more spatial discretization, keeping the data requirements, numerical stability and computational demand for large-scale complex watersheds, therefore, continuing the ongoing development and applicability of SWAT+ (Bieger et al., 2017; Gassman et al., 2007).
Model simulations show a general tendency to underestimate wetter periods for all three observation stations. This could be due to a misrepresentation of rainfall, parametization and set up of the model, and simplification of aquifer flow in SWAT+ (Barresi Armoa et al., 2023; Wagner et al., 2022). The Pantanal system is a very complex area where the flood wave can propagate outside the channel and travel long distances outside the main reach (do Nascimento et al., 2023). Moreover, when overbank flow occurs, the water flows across upstream and downstream floodplains (Guilhen, 2023). However, in the current SWAT+ configuration, there is no connection between upstream and downstream floodplain LSUs, which is a considerable simplification. In the Pantanal, water that moves along the floodplain might re-enter the main channel far downstream or even contribute to another river (do Nascimento et al., 2023). In this SWAT+ set up, each floodplain is connected to its closest channel and therefore misrepresenting the real connectivity. In addition, seasonal and permanent lakes can contribute to water storage coming from rainfall (da Paz et al., 2014), which is not accounted for in the current SWAT+ setup. Model performance could be improved by representing a more realistic connectivity, for example, by interconnecting the floodplains and aquifers across the Pantanal to allow water exchanges. In addition, providing more spatial complexity to the model, by adding the permanent lakes and other important water bodies, could ameliorate the estimation of some hydrological components such as the evapotranspiration, therefore improving model accuracy. Nevertheless, despite the complex drainage network limitations, the model was able to capture the hydrological regime in the Paraguay river.
The hydrological cycle seem to be well-simulated. For example, similar water balance results where found in Su and Lettenmaier 2009 (Table 3). Su and Lettenmaier 2009 obtained a long-term surface water storage budget for the Paraguay River Basin after analyzing 20 years of observed data (from 1979 to 1999) and using the Variable Infiltration Capacity (VIC) land surface hydrologic model. These results were assessed with observed data and the ERA-40 reanalysis product (Uppala et al., 2005), providing good insights of the hydrological processes occurring in the Paraguay Basin. Figure 12 shows the spatial distribution of evapotranspiration in the Paraguay River for the austral winter. The highest ET values are occurring in the Pantanal region and other delineated floodplain areas, suggesting a good representation of the hydrological components in the Paraguay River Basin. The same spatial pattern was found in Su and Lettenmaier 2009 and Ruhoff et al. 2022 in which high ET values occur across the Pantanal wetland.
Table 3. Annual average values for water balance components in mm for the Studied Area in the Paraguay River.
Figure 12. Monthly mean evapotranspiration in mm for austral winter (June, July, and August), for the period 1990–2020.
As mentioned, Figure 7 compares the simulated discharges of the SWAT+ model with floodplains and a SWAT+ model without floodplains. These results were obtained with the same parameter values. In the SWAT+ model without floodplains, the flood period begins 2–5 months earlier in almost all simulated years, resulting in very low NSE values. This is because (1) water is not being stored in floodplains when overbank flow occurs and (2) surface and subsurface flow coming from uplands is not retained by the floodplain. The SWAT+ model with floodplains performs much better regarding the correct timing and magnitude of flooding by shifting the high flows and reducing the peaks, although for our calibration values, it tends to start flooding 1–2 months before time in some simulated years, for example, between 2003 and 2004 flooding starts 1 month earlier. Furthermore, some wet periods are being underestimated, for example, during the years 1991 to 1994 and 1995 to 1996. The dry periods are well-simulated, as shown during 2019 and 2020, when an important drought event took place in the Paraguay River Basin (Marengo et al., 2021; Libonati et al., 2022). Calibration values could be improved by further discretizing the watershed to provide a better spatial parametization of the floodplain, such as both depths to the principal and emergency parameters, the floodplain roughness coefficient, and the percolation coefficient.
Schrapffer et al. 2023 estimated that the total water storage in the Pantanal reaches its maximum around April, with an annual mean value of ~66.7 mm for their simulation period (2003–2013). For the same simulation period, SWAT+ estimates 87.81 mm. Differences in the Total Water Storage estimates between Schrapffer et al. 2023 and this study may be attributed to the surface area considered, as this study encompasses not only the Pantanal but the Paraguay River Basin, and the climate forcing. Figure 13 shows the temporal evolution of the average water storage in the floodplains and the streamflow at the outlet. Between January and April, the volume of water stored in the floodplains is greater than the streamflow at the outlet highlighting the importance of the floodplains on flow regulation in the Paraguay River.
Figure 13. Average monthly Water Storage in the floodplains and Discharge (1990–2020) at Asuncion in mm/month.
Other studies in the Paraguay Basin and Pantanal analyzed the surface area inundated or the height of the water (Paz et al., 2011; da Paz et al., 2014; Hamilton et al., 1996). For example, Hamilton et al. 1996 estimated the flooded surface area in the Pantanal, obtaining the maximum around April. As the topography in the Paraguay River is mostly flat, we can assume a homogeneous height for the region, which results in a pattern similar to Hamilton et al. 1996 and da Paz et al. 2014 (Figure 8). Guilhen et al. 2022, performed a similar study in the Madeira Basin, located north of the Paraguay Basin, and also obtained a maximum of water storage around March and April. The total water volume stored in the Pantanal needs further validation by means of remote sensing techniques, such as altimetry analysis. However, the seasonal flooding and the water storage regime was well-reproduced by SWAT+ as the same trends were reported in other studies (Hamilton et al., 1996; Paz et al., 2011; Assine et al., 2016; Schrapffer et al., 2023).
In hydrological terms, the Pantanal wetland functions as a massive sponge, absorbing floodwaters from the headwaters of the Paraguay River Basin, temporarily storing them and then slowly releasing the water back into the main river (Stevaux et al., 2020). This process causes a delay in the flood peak of the Paraguay River compared to the Paraná River where the two rivers meet. For these reasons, the Pantanal (and wetlands in general) plays an important role in climate, hydrology, biocultural diversity (Wantzen et al., 2024) and minimal disturbances in the hydrological cycle can alter its functioning. According to projections of climate models used for the last AR6 report from the Intergovernmental Panel on Climate Change (IPCC), the surface area of wetlands in South America could be reduced by 28% at the end of the 21st century (2081–2100) under the SSP370 scenario (Hardouin et al., 2024). Furthermore, current human activities are disturbing the hydrologic cycle in the Paraguay River (Alho and Silva, 2012; Alho, 2008; Hamilton, 2002) threatening this UNESCO World Heritage Site. Moreover, other human-induced projects that menaces to alter the channel-floodplain connection could cause a multitude of negative impacts that can affect the Pantanal region economically, socially and ecologically (Wantzen et al., 2024). It is therefore imperative to continue to provide more scientific evidence on the effect of the channel-floodplain interactions in maintaining the hydrology of the Paraguay River Basin.
Other model limitations are related to its configuration and data availability:
1. Reservoirs: The Manso Dam, located on one of the main tributaries of the Paraguay River Basin, was reported to cause hydrological changes in the Paraguay River's hydrology by releasing water during the dry season and retaining water during the wet season (Jardim et al., 2020). In this study, reservoir analysis is not considered due to the lack of reservoir management data.
2. Agriculture: Information about agriculture practices was not available. Therefore, a general decision table for crops provided by the Land Use data input was used for this SWAT+ model.
3. Groundwater in SWAT+: The default value of the soil infiltration rate (k) for floodplains HRUs is set to 0.01 mm/hr, which prevents the soils from exceeding field capacity and limits the soil percolation value. While a good calibration can overcome this issue, as explained in Sánchez-Gómez et al. 2024, the representation of aquifer processes remains simplified in SWAT+ and it is recommended to use the groundwater flow module for SWAT+ proposed by Bailey et al. 2020 to realistically simulate the soil saturation and percolation.
In this study, we developed code routines in SWAT+ to represent the interactions between uplands, floodplains and channels. The model was applied in the Paraguay River Basin, in which the hydrology is affected by the Pantanal's complex floodplain network. SWAT+ offers two routing methodologies (Variable Storage Capacity and Muskingum) that were reported as insufficient in floodplain-dominated zones. However, by using the concept of Landscape Units, assuming a reservoir-like floodplain behavior, and by including a simple overbank flow equation, SWAT+ is able to satisfactorily capture the hydrological regime in the Paraguay River Basin. This represents an improvement for the ongoing development of SWAT+, as it maintains the ease of the model in terms of data requirements, computational power, numerical stability, while adding spatial complexity in terms of the model outputs. The model was calibrated for discharge and the results varied from satisfactory to good. Spatial representation of evapotranspiration and the flood wave behavior were well-captured by the model. Flooding in the Paraguay River is seasonal, and regulated by the presence of bottlenecks. The simulated trend and value of the volume of water stored in the Pantanal was in accordance with what has been reported in the literature. Further remote sensing analysis is necessary to calibrate and validate the volume of water stored in the Paraguay River. Simulated results indicate that ~61% of the surface water travel through the floodplain before reaching the outlet, highlighting the role of the Pantanal in retaining inorganic and organic particles, and thus maintaining water quality. Future assessments of the hydrology, sediments, and human activities such as agricultural practice, land use change and pollutants transport in large-scale floodplain-dominated zones watersheds with SWAT+, could bring more scientific evidence to the importance of protecting wetland systems.
The raw data supporting the conclusions of this article will be made available by the authors, without undue reservation.
OB: Data curation, Formal analysis, Funding acquisition, Methodology, Resources, Visualization, Writing – original draft, Writing – review & editing. JA: Conceptualization, Investigation, Methodology, Software, Validation, Writing – review & editing. KB: Formal analysis, Investigation, Methodology, Validation, Writing – review & editing. SS: Conceptualization, Formal analysis, Funding acquisition, Investigation, Resources, Supervision, Validation, Writing – review & editing. JS-P: Conceptualization, Formal analysis, Funding acquisition, Investigation, Resources, Supervision, Validation, Writing – review & editing.
The author(s) declare financial support was received for the research, authorship, and/or publication of this article. This study was partially supported through the grant EUR TESS N°ANR-18-EURE-0018 in the framework of the Programme des Investissements d'Avenir. Osvaldo Luis Barresi Armoa, was supported by the scholarship program Becas Don Carlos Antonio López BECAL N°88/2021.
The authors gratefully acknowledge the valuable comments and suggestions provided by the reviewers, which have contributed to the improvement of this article.
The authors declare that the research was conducted in the absence of any commercial or financial relationships that could be construed as a potential conflict of interest.
All claims expressed in this article are solely those of the authors and do not necessarily represent those of their affiliated organizations, or those of the publisher, the editors and the reviewers. Any product that may be evaluated in this article, or claim that may be made by its manufacturer, is not guaranteed or endorsed by the publisher.
Abbaspour, K. C., Rouholahnejad, E., Vaghefi, S., Srinivasan, R., Yang, H., and Kløve, B. (2015). A continental-scale hydrology and water quality model for europe: Calibration and uncertainty of a high-resolution large-scale swat model. J. Hydrol. 524, 733–752. doi: 10.1016/j.jhydrol.2015.03.027
Alho, C. J., and Silva, J. S. (2012). Effects of severe floods and droughts on wildlife of the pantanal wetland (Brazil)—a review. Animals 2, 591–610. doi: 10.3390/ani2040591
Alho, C. J. R. (2008). Biodiversity of the pantanal: response to seasonal flooding regime and to environmental degradation. Brazil. J. Biol. 68, 957–966. doi: 10.1590/S1519-69842008000500005
Alsdorf, D. E., Rodríguez, E., and Lettenmaier, D. P. (2007). Measuring surface water from space. Rev. Geophys. 45:197. doi: 10.1029/2006RG000197
Arnold, J. G., Bieger, K., White, M. J., Srinivasan, R., Dunbar, J. A., and Allen, P. M. (2018). Use of decision tables to simulate management in swat+. Water 10:713. doi: 10.3390/w10060713
Arnold, J. G., Moriasi, D. N., Gassman, P. W., Abbaspour, K. C., White, M. J., Srinivasan, R., et al. (2012). Swat: model use, calibration, and validation. Trans. ASABE 55, 1491–1508. doi: 10.13031/2013.42256
Arnold, J. G., Srinivasan, R., Muttiah, R. S., and Williams, J. R. (1998). Large area hydrologic modeling and assessment part I: model development 1. J. Am. Water Resour. Assoc. 34, 73–89. doi: 10.1111/j.1752-1688.1998.tb05961.x
Assine, M. L., Macedo, H. A., Stevaux, J. C., Bergier, I., Padovani, C. R., and Silva, A. (2016). Avulsive rivers in the hydrology of the pantanal wetland. Dyn. Pant. Wetl. South Am. 351, 83–110. doi: 10.1007/698_2015_351
Bailey, R. T., Bieger, K., Arnold, J. G., and Bosch, D. D. (2020). A new physically-based spatially-distributed groundwater flow module for swat+. Hydrology 7:75. doi: 10.3390/hydrology7040075
Barresi Armoa, O. L., Sauvage, S., Houska, T., Bieger, K., Schürz, C., and Sánchez Pérez, J. M. (2023). Representation of hydrological components under a changing climate–a case study of the uruguay river basin using the new version of the soil and water assessment tool model (swat+). Water 15:2604. doi: 10.3390/w15142604
Bates, P., Stewart, M., Desitter, A., Anderson, M., Renaud, J.-P., and Smith, J. A. (2000). Numerical simulation of floodplain hydrology. Water Resour. Res. 36, 2517–2529. doi: 10.1029/2000WR900102
Beck, H. E., Vergopolan, N., Pan, M., Levizzani, V., Van Dijk, A. I., Weedon, G. P., et al. (2017). Global-scale evaluation of 22 precipitation datasets using gauge observations and hydrological modeling. Hydrol. Earth Syst. Sci. 21, 6201–6217. doi: 10.5194/hess-21-6201-2017
Berbery, E. H., and Barros, V. R. (2002). The hydrologic cycle of the la plata basin in South America. J. Hydrometeorol. 3, 630–645. doi: 10.1175/1525-7541(2002)003<0630:THCOTL>2.0.CO;2
Bieger, K., Arnold, J. G., Rathjens, H., White, M. J., Bosch, D. D., Allen, P. M., et al. (2017). Introduction to swat+, a completely restructured version of the soil and water assessment tool. J. Am. Water Resour. Assoc. 53, 115–130. doi: 10.1111/1752-1688.12482
Bieger, K., Arnold, J. G., Rathjens, H., White, M. J., Bosch, D. D., and Allen, P. M. (2019). Representing the connectivity of upland areas to floodplains and streams in swat+. J. Am. Water Resour. Assoc. 55, 578–590. doi: 10.1111/1752-1688.12728
Bravo, J. M., Allasia, D., Paz, A. R. d., Collischonn, W., and Tucci, C. E. M. (2012). Coupled hydrologic-hydraulic modeling of the upper paraguay river basin. J. Hydrol. Eng. 17, 635–646. doi: 10.1061/(ASCE)HE.1943-5584.0000494
Camargo, A. L. G., Girard, P., Sanz-Lazaro, C., Silva, A. C. M., De Faria, É., Figueiredo, B. R. S., et al. (2022). Microplastics in sediments of the Pantanal wetlands, Brazil. Front. Environ. Sci. 10:1017480. doi: 10.3389/fenvs.2022.1017480
Cerón, W. L., Molina-Carpio, J., Ayes Rivera, I., Andreoli, R. V., Kayano, M. T., and Canchala, T. (2020). A principal component analysis approach to assess chirps precipitation dataset for the study of climate variability of the La Plata basin, Southern South America. Natur. Hazard. 103, 767–783. doi: 10.1007/s11069-020-04011-x
Chawanda, C. J., Arnold, J., Thiery, W., and van Griensven, A. (2020). Mass balance calibration and reservoir representations for large-scale hydrological impact studies using SWAT+. Clim. Change 163, 1307–1327. doi: 10.1007/s10584-020-02924-x
Collischonn, W., Allasia, D., Da Silva, B. C., and Tucci, C. E. (2007). The MGB-IPH model for large-scale rainfall—runoff modelling. Hydrol. Sci. J. 52, 878–895. doi: 10.1623/hysj.52.5.878
Collischonn, W., Tucci, C. E. M., and Clarke, R. (2001). Further evidence of changes in the hydrological regime of the river paraguay: part of a wider phenomenon of climate change? J. Hydrol. 245, 218–238. doi: 10.1016/S0022-1694(01)00348-1
da Paz, A. R., Collischonn, W., Bravo, J. M., Bates, P. D., and Baugh, C. (2014). The influence of vertical water balance on modelling pantanal (Brazil) spatio-temporal inundation dynamics. Hydrol. Process. 28, 3539–3553. doi: 10.1002/hyp.9897
de Oliveira Roque, F., Guerra, A., Johnson, M., Padovani, C., Corbi, J., Covich, A. P., et al. (2021). Simulating land use changes, sediment yields, and pesticide use in the upper paraguay river basin: Implications for conservation of the pantanal wetland. Agricult. Ecosyst. Environ. 314:107405. doi: 10.1016/j.agee.2021.107405
do Nascimento, S. C., Melo, M. M. M., and da Paz, A. R. (2023). River-floodplain interaction and flood wave routing along rivers flowing through Pantanal wetlands. J. South Am. Earth Sci. 125:104296. doi: 10.1016/j.jsames.2023.104296
dos Santos Pinto, R. M., Weigelhofer, G., Diaz-Pines, E., Brito, A. G., Zechmeister-Boltenstern, S., and Hein, T. (2020). River-floodplain restoration and hydrological effects on GHG emissions: biogeochemical dynamics in the parafluvial zone. Sci. Tot. Environ. 715:136980. doi: 10.1016/j.scitotenv.2020.136980
Farr, T. G., Rosen, P. A., Caro, E., Crippen, R., Duren, R., Hensley, S., et al. (2007). The shuttle radar topography mission. Rev. Geophys. 45:183. doi: 10.1029/2005RG000183
Fenton, J. D. (2019). Flood routing methods. J. Hydrol. 570, 251–264. doi: 10.1016/j.jhydrol.2019.01.006
Ferreira Barbosa, M. L., Haddad, I., da Silva Nascimento, A. L., Máximo da Silva, G., Moura da Veiga, R., Hoffmann, T. B., et al. (2022). Compound impact of land use and extreme climate on the 2020 fire record of the Brazilian Pantanal. Glob. Ecol. Biogeogr. 31, 1960–1975. doi: 10.1111/geb.13563
Fleischmann, A. S., Paiva, R. C., Collischonn, W., Sorribas, M. V., and Pontes, P. R. (2016). On river-floodplain interaction and hydrograph skewness. Water Resour. Res. 52, 7615–7630. doi: 10.1002/2016WR019233
Funk, C., Peterson, P., Landsfeld, M., Pedreros, D., Verdin, J., Shukla, S., et al. (2015). The climate hazards infrared precipitation with stations—a new environmental record for monitoring extremes. Scient. Data 2, 1-21. doi: 10.1038/sdata.2015.66
Garcia, L. C., Szabo, J. K., de Oliveira Roque, F., Pereira, A. M. M., da Cunha, C. N., Damasceno-J'unior, G. A., et al. (2021). Record-breaking wildfires in the world's largest continuous tropical wetland: integrative fire management is urgently needed for both biodiversity and humans. J. Environ. Manage. 293:112870. doi: 10.1016/j.jenvman.2021.112870
Gassman, P. W., Reyes, M. R., Green, C. H., and Arnold, J. G. (2007). The soil and water assessment tool: historical development, applications, and future research directions. Trans. ASABE 50, 1211–1250. doi: 10.13031/2013.23637
Girard, P., da Silva, C. J., and Abdo, M. (2003). River–groundwater interactions in the Brazilian Pantanal. The case of the Cuiabá river. J. Hydrol. 283, 57–66. doi: 10.1016/S0022-1694(03)00235-X
Guilhen, J. (2023). Caractérisation des dynamiques hydro-sédimentaires dans les zones alluviales ripariennes du bassin versant de l'Amazone par une approche couplant modélisation et télédétection (Ph. D. thesis). Institut National Polytechnique de Toulouse-INPT, Toulouse, France.
Guilhen, J., Parrens, M., Sauvage, S., Santini, W., Mercier, F., Al Bitar, A., et al. (2022). Estimation of the Madeira floodplain dynamics from 2008 to 2018. Front. Water 4:952810. doi: 10.3389/frwa.2022.952810
Hamilton, S. (2002). Human impacts on hydrology in the Pantanal wetland of South America. Water Sci. Technol. 45, 35–44. doi: 10.2166/wst.2002.0377
Hamilton, S. K. (1999). Potential effects of a major navigation project (paraguay–paraná hidrovía) on inundation in the pantanal floodplains. Regul. River. Res. Manag. 15, 289–299.
Hamilton, S. K., and McClain, M. (2002). Hydrological controls of ecological structure and function in the Pantanal wetland (Brazil). Ecohydrol. South Am. River. Wetland. 6, 133–158.
Hamilton, S. K., Sippel, S. J., and Melack, J. M. (1996). Inundation patterns in the Pantanal wetland of South America determined from passive microwave remote sensing. Archiv für Hydrobiologie 137, 1–23.
Hardouin, L., Decharme, B., Colin, J., and Delire, C. (2024). Climate-driven projections of future global wetlands extent. Earth's Fut. 12:e2024EF004553. doi: 10.1029/2024EF004553
Hersbach, H., Bell, B., Berrisford, P., Hirahara, S., Horányi, A., Muñoz-Sabater, J., et al. (2020). The ERA5 global reanalysis. Quart. J. Royal Meteorol. Soc. 146, 1999–2049. doi: 10.1002/qj.3803
Houska, T., Kraft, P., Chamorro-Chavez, A., and Breuer, L. (2019). SPOTPY: a python library for the calibration, sensitivity-and uncertainty analysis of earth system models. Geophys. Res. Abstr. 21.
Ivory, S. J., McGlue, M. M., Spera, S., Silva, A., and Bergier, I. (2019). Vegetation, rainfall, and pulsing hydrology in the pantanal, the world's largest tropical wetland. Environ. Res. Lett. 14:124017. doi: 10.1088/1748-9326/ab4ffe
Jardim, P. F., Melo, M. M. M., Ribeiro, L. d. C., Collischonn, W., and Paz, A. R. d. (2020). A modeling assessment of large-scale hydrologic alteration in South American pantanal due to upstream dam operation. Front. Environ. Sci. 8:567450. doi: 10.3389/fenvs.2020.567450
Krepper, C. M., García, N. O., and Jones, P. D. (2006). Paraguay river basin response to seasonal rainfall. Int. J. Climatol. 26, 1267–1278. doi: 10.1002/joc.1313
Leone, M., Gentile, F., Porto, A. L., Ricci, G., Schürz, C., Strauch, M., et al. (2024). Setting an environmental flow regime under climate change in a data-limited mediterranean basin with temporary river. J. Hydrol. 52:101698. doi: 10.1016/j.ejrh.2024.101698
Libonati, R., Geirinhas, J. L., Silva, P. S., Russo, A., Rodrigues, J. A., Belém, L. B., et al. (2022). Assessing the role of compound drought and heatwave events on unprecedented 2020 wildfires in the pantanal. Environ. Res. Lett. 17:e015005. doi: 10.1088/1748-9326/ac462e
Liu, Y., Yang, W., and Wang, X. (2008). Development of a swat extension module to simulate riparian wetland hydrologic processes at a watershed scale. Hydrol. Process. 22, 2901–2915. doi: 10.1002/hyp.6874
Marengo, J. A., Cunha, A. P., Cuartas, L. A., Deusdará Leal, K. R., Broedel, E., Seluchi, M. E., et al. (2021). Extreme drought in the Brazilian pantanal in 2019–2020: characterization, causes, and impacts. Front. Water 3:639204. doi: 10.3389/frwa.2021.639204
Marques, J. F., Alves, M. B., Silveira, C. F., E Silva, A. A., Silva, T. A., Dos Santos, V. J., et al. (2021). Fires dynamics in the pantanal: impacts of anthropogenic activities and climate change. J. Environ. Manag. 299:113586. doi: 10.1016/j.jenvman.2021.113586
Metcalfe, C. D., and Menone, M. L. (2020). The paraná river basin and its ecosystem services. Paraná River Basin 2020, 1–16. doi: 10.4324/9780429317729-1
Moriasi, D. N., Arnold, J. G., Van Liew, M. W., Bingner, R. L., Harmel, R. D., and Veith, T. L. (2007). Model evaluation guidelines for systematic quantification of accuracy in watershed simulations. Trans. ASABE 50, 885–900. doi: 10.13031/2013.23153
Neitsch, S. L., and Arnold, J. G. (2009). Soil and Water Assessment Tool Theoretical Documentation. College Station, TX: Texas A&M University: College of Agriculture and Life Sciences.
Ngo-Duc, T., Laval, K., Ramillien, G., Polcher, J., and Cazenave, A. (2007). Validation of the land water storage simulated by organising carbon and hydrology in dynamic ecosystems (orchidee) with gravity recovery and climate experiment (grace) data. Water Resour. Res. 43:4941. doi: 10.1029/2006WR004941
Parrens, M., Al Bitar, A., Frappart, F., Papa, F., Calmant, S., Crétaux, J.-F., et al. (2017). Mapping dynamic water fraction under the tropical rain forests of the amazonian basin from SMOS brightness temperatures. Water 9:350. doi: 10.3390/w9050350
Paz, A. R. d., Collischonn, W., Tucci, C. E., and Padovani, C. R. (2011). Large-scale modelling of channel flow and floodplain inundation dynamics and its application to the pantanal (Brazil). Hydrol. Process. 25, 1498–1516. doi: 10.1002/hyp.7926
Pereira, G., Ramos, R. d. C., Rocha, L. C., Brunsell, N. A., Merino, E. R., Mataveli, G. A. V., et al. (2021). Rainfall patterns and geomorphological controls driving inundation frequency in tropical wetlands: how does the pantanal flood? Progr. Phys. Geogr. 45, 669–686. doi: 10.1177/0309133320987719
Pontes, P. R. M., Fan, F. M., Fleischmann, A. S., de Paiva, R. C. D., Buarque, D. C., Siqueira, V. A., et al. (2017). Mgb-iph model for hydrological and hydraulic simulation of large floodplain river systems coupled with open source GIS. Environ. Model. Softw. 94, 1–20. doi: 10.1016/j.envsoft.2017.03.029
Rahman, M. M., Thompson, J. R., and Flower, R. J. (2016). An enhanced swat wetland module to quantify hydraulic interactions between riparian depressional wetlands, rivers and aquifers. Environ. Model. Softw. 84, 263–289. doi: 10.1016/j.envsoft.2016.07.003
Rathjens, H., Bieger, K., Chaubey, I., Arnold, J., Allen, P., Srinivasan, R., et al. (2016). Delineating floodplain and upland areas for hydrologic models: a comparison of methods. Hydrol. Process. 30, 4367–4383. doi: 10.1002/hyp.10918
Ruhoff, A., de Andrade, B. C., Laipelt, L., Fleischmann, A. S., Siqueira, V. A., Moreira, A. A., et al. (2022). Global evapotranspiration datasets assessment using water balance in South America. Rem. Sens. 14:2526. doi: 10.3390/rs14112526
Sánchez-Gómez, A., Schürz, C., Molina-Navarro, E., and Bieger, K. (2024). Groundwater modelling in SWAT+: considerations for a realistic baseflow simulation. Groundw. Sustain. Dev. 26:101275. doi: 10.1016/j.gsd.2024.101275
Santini, W. (2020). Caractérisation de la dynamique hydro-sédimentaire du bassin de l'Ucayali (Pérou), par une approche intégrant réseau de mesures, télédétection et modélisation hydrologique (Ph. D. thesis), Université Paul Sabatier-Toulouse III, Toulouse, France.
Schmidt, R., Flechtner, F., Meyer, U., Neumayer, K.-H., Dahle, C., König, R., et al. (2008). Hydrological signals observed by the grace satellites. Surv. Geophys. 29, 319–334. doi: 10.1007/s10712-008-9033-3
Schrapffer, A., Polcher, J., Sörensson, A., and Fita, L. (2023). Introducing a new floodplain scheme in orchidee (version 7885): validation and evaluation over the pantanal wetlands. Geoscient. Mod. Dev. 16, 5755–5782. doi: 10.5194/gmd-16-5755-2023
Schrapffer, A., Sörensson, A., Polcher, J., and Fita, L. (2020). Benefits of representing floodplains in a land surface model: pantanal simulated with orchidee CMIP6 version. Clim. Dyn. 55, 1303–1323. doi: 10.1007/s00382-020-05324-0
Schürz, C., Čerkasova, N., Strauch, M., Plunge, S., Piniewski, M., Arnold, J., et al. (2022). “A framework for constructing swat+ model setups based on contiguous objects and their connectivity,” in 2022 International SWAT Conference; Conference date: 13-07-2022 Through 15-07-2022. Available online at: https://swatconference.tamu.edu/#/book-of-abstracts/2022-prague (accessed June, 2024).
Shrestha, R. K., Seidl, A. F., and Moraes, A. S. (2002). Value of recreational fishing in the Brazilian pantanal: a travel cost analysis using count data models. Ecol. Econ. 42, 289–299. doi: 10.1016/S0921-8009(02)00106-4
Stevaux, J. C., de Azevedo Macedo, H., Assine, M. L., and Silva, A. (2020). Changing fluvial styles and backwater flooding along the upper paraguay river plains in the Brazilian pantanal wetland. Geomorphology 350:106906. doi: 10.1016/j.geomorph.2019.106906
Su, F., and Lettenmaier, D. P. (2009). Estimation of the surface water budget of the La Plata basin. J. Hydrometeorol. 10, 981–998. doi: 10.1175/2009JHM1100.1
Thompson, A., Baffaut, C., Lohani, S., Duriancik, L., Norfleet, M., and Ingram, K. (2020). Purpose, development, and synthesis of the soil vulnerability index for inherent vulnerability classification of cropland soils. J. Soil Water Conserv. 75, 1–11. doi: 10.2489/jswc.75.1.1
Tortato, F. R., and Izzo, T. J. (2017). Advances and barriers to the development of Jaguar-tourism in the Brazilian pantanal. Perspect. Ecol. Conserv. 15, 61–63. doi: 10.1016/j.pecon.2017.02.003
Uppala, S. M., Kållberg, P., Simmons, A. J., Andrae, U., Bechtold, V. D. C., et al. (2005). The ERA-40 re-analysis. Quart. J. Royal Meteorol. Soc. 131, 2961–3012. doi: 10.1256/qj.04.176
Viana, L. F., do Amaral Crispim, B., Kummrow, F., do Nascimento, V. A., de Pádua Melo, E. S., de Lima, N. A., et al. (2022). Bioaccumulation, genotoxicity, and risks to native fish species from inorganic contaminants in the pantanal Sul-Mato-Grossense, Brazil. Environ. Pollut. 314:120204. doi: 10.1016/j.envpol.2022.120204
Vourlitis, G. L., Hentz, C. S., Pinto Jr, O. B., Carneiro, E., and de Souza Nogueira, J. (2017). Soil N, P, and C dynamics of upland and seasonally flooded forests of the Brazilian pantanal. Glob. Ecol. Conserv. 12, 227–240. doi: 10.1016/j.gecco.2017.11.004
Wagner, P. D., Bieger, K., Arnold, J. G., and Fohrer, N. (2022). Representation of hydrological processes in a rural lowland catchment in northern germany using SWAT and SWAT+. Hydrol. Process. 36:e14589. doi: 10.1002/hyp.14589
Wantzen, K. M., Assine, M. L., Bortolotto, I. M., Calheiros, D. F., Campos, Z., Catella, A. C., et al. (2024). The end of an entire biome? world's largest wetland, the pantanal, is menaced by the Hidrovia project which is uncertain to sustainably support large-scale navigation. Sci. Tot. Environ. 908:167751. doi: 10.1016/j.scitotenv.2023.167751
White, M. J., Arnold, J. G., Bieger, K., Allen, P. M., Gao, J., Čerkasova, N., et al. (2022). Development of a field scale swat+ modeling framework for the contiguous us. J. Am. Water Resour. Assoc. 58, 1545–1560. doi: 10.1111/1752-1688.13056
Wong, T. H., and Laurenson, E. M. (1983). Wave speed–discharge relations in natural channels. Water Resour. Res. 19, 701–706.
Keywords: SWAT+, floodplain, water routing, Paraguay River, Pantanal, flood wave, Landscape Units, connectivity
Citation: Barresi Armoa OL, Arnold JG, Bieger K, Sauvage S and Sánchez-Pérez JM (2024) Large wetlands representation in SWAT+: the case of the Pantanal in the Paraguay River Basin. Front. Water 6:1451648. doi: 10.3389/frwa.2024.1451648
Received: 19 June 2024; Accepted: 04 November 2024;
Published: 29 November 2024.
Edited by:
Andrew Ogilvie, Institut de Recherche Pour le Développement (IRD), FranceReviewed by:
Venkatramanan Senapathi, National College, Tiruchirappalli, IndiaCopyright © 2024 Barresi Armoa, Arnold, Bieger, Sauvage and Sánchez-Pérez. This is an open-access article distributed under the terms of the Creative Commons Attribution License (CC BY). The use, distribution or reproduction in other forums is permitted, provided the original author(s) and the copyright owner(s) are credited and that the original publication in this journal is cited, in accordance with accepted academic practice. No use, distribution or reproduction is permitted which does not comply with these terms.
*Correspondence: Osvaldo Luis Barresi Armoa, b3N2YWxkby1sdWlzLmJhcnJlc2ktYXJtb2FAdW5pdi10bHNlMy5mcg==; José Miguel Sánchez-Pérez, am9zZS1taWd1ZWwuc2FuY2hlei1wZXJlekB1bml2LXRsc2UzLmZy
Disclaimer: All claims expressed in this article are solely those of the authors and do not necessarily represent those of their affiliated organizations, or those of the publisher, the editors and the reviewers. Any product that may be evaluated in this article or claim that may be made by its manufacturer is not guaranteed or endorsed by the publisher.
Research integrity at Frontiers
Learn more about the work of our research integrity team to safeguard the quality of each article we publish.