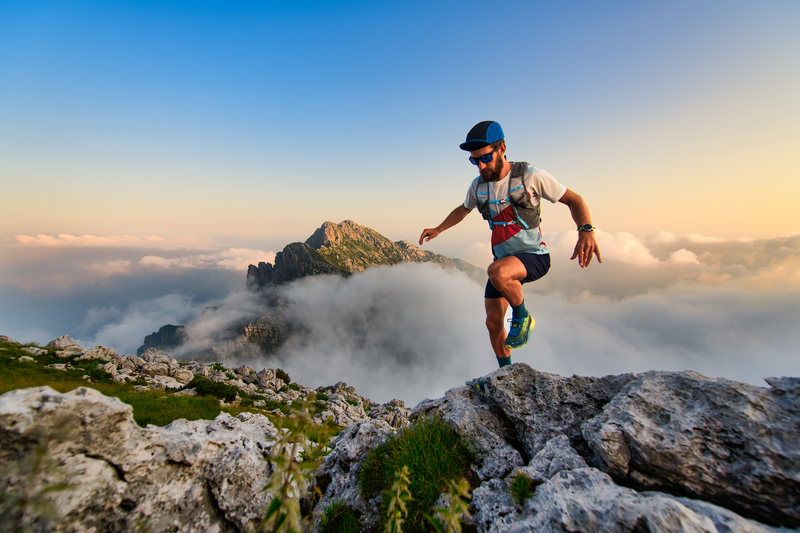
94% of researchers rate our articles as excellent or good
Learn more about the work of our research integrity team to safeguard the quality of each article we publish.
Find out more
ORIGINAL RESEARCH article
Front. Water , 09 August 2024
Sec. Environmental Water Quality
Volume 6 - 2024 | https://doi.org/10.3389/frwa.2024.1397168
Land cover changes alter hydrologic (e.g., infiltration-runoff), biochemical (e.g., nutrient loads), and ecological processes (e.g., stream metabolism). We quantified differences in aquatic ecosystem respiration in two contrasting stream reaches from a forested watershed in Colorado (1st-order reach) and an agricultural watershed in Iowa (3rd-order reach). We conducted two rounds of experiments in each of these reaches, featuring four sets of continuous injections of Cl− as a conservative tracer, resazurin as a proxy for aerobic respiration, and one of the following nutrient treatments: (a) N, (b) N + C, (c) N + P, and (d) C + N + P. With those methods providing consistent information about solute transport, stream respiration, and nutrient processing at the same spatiotemporal scales, we sought to address: (1) Are respiration rates correlated with conservative transport metrics in forested or agricultural streams? and (2) Can short-term modifications of stoichiometric conditions (C:N:P ratios) override respiration patterns, or do long-term physicochemical conditions control those patterns? We found greater respiration in the reach located in the forested watershed but no correlations between respiration, discharge, and advective or transient storage timescales. All the experiments conducted in the agricultural stream featured a reaction-limited transformation of resazurin, suggesting the existence of nutrient or carbon limitations on respiration that our short-term nutrient treatments did not remove. In contrast, the forested stream was characterized by nearly balanced transformation and transient storage timescales. We also found that our short-lived nutrient treatments had minimal influence on the significantly different respiration patterns observed between reaches, which are most likely driven by the longer-term and highly contrasting ambient nutrient concentrations at each site. Our experimental results agree with large-scale analyses suggesting greater microbial respiration in headwater streams in the U.S. Western Mountains region than in second-to-third-order streams in the U.S. Temperate Plains region.
Watersheds are fundamental units of analysis in the study of hydrological processes and are the result of the co-evolution of global-to-local meteorological, geological, chemical, and biological processes over geological timescales (Wang et al., 2016; Li et al., 2018). Human intervention has resulted in watershed modifications with comparable impacts at the local to planetary scales but has occurred over significantly shorter timescales, marking a newly proposed geological epoch (Crutzen, 2006; Zalasiewicz et al., 2011; Wilson et al., 2018). The Anthropocene has been used to describe the period where human activity has become the dominant influence on climatic and environmental processes (Wilson et al., 2018). While only a few places could arguably be considered undisturbed by anthropogenic activities, relatively undisturbed forested watersheds feature low anthropogenic disturbances and pollution, 80% or more natural vegetative cover, and population densities below 5 individuals/km2 (Lewis et al., 1999). Within the myriad of interests in understanding the impacts of human modifications on biotic and abiotic processes, hydrologists and ecologists are particularly interested in quantifying the differences in the functioning of stream ecosystems draining watersheds along the disturbance continuum (i.e., forested-agricultural-urban) to create baselines in assessments, mitigate and prevent pollution, and restore ecosystems (Wohl et al., 2015; Regier et al., 2020; Maaß et al., 2021; Paul et al., 2021).
Land cover changes are one of the most common and impactful results of anthropogenic activities and typically involve the transformation of forests, shrubs, and grasslands into agricultural, semi-urban, or urban areas. Along with these changes, terrestrial and aquatic ecosystems are altered (Downing et al., 1999; Newbold et al., 2015; Sleeter et al., 2018; Gomes et al., 2021; Paul et al., 2021) and in some cases even local atmospheric processes such as urban heat islands become concerning (He et al., 2007; Rahaman et al., 2022). Land use and land cover changes alter hydrologic processes beyond the distribution of rainfall, infiltration, and runoff fluxes, impacting water quality and ecological processes (Tasser et al., 2005; Wu et al., 2017; Watson et al., 2018). In recent decades, stream metabolism has become a reference framework to compare results from within (e.g., over time) and across watershed studies linking land use changes to impacts on ecological processes. Stream ecosystem metabolism combines the cumulative photosynthetic and heterotrophic activity and is typically quantified in field settings through diel-oxygen curves and gas-exchange coefficients that allow estimates of gross primary production (GPP) and ecosystem respiration (ER) (Odum 1956; Grace et al., 2015). Stream metabolism is strongly linked to physicochemical attributes of lotic ecosystems, including photosynthetically active radiation, turbidity levels, water depth, and carbon inputs with varying lability (Vannote et al., 1980; Webster, 2007). Together, ER and GPP are measures of the cumulative processes primarily responsible for biological nutrient uptake in streams and thus provide vital information for understanding nutrient dynamics in lotic ecosystems.
The comparison between stream metabolism in forested and agricultural streams can help us understand how less-disturbed stream ecosystems may respond to anthropogenic changes to land use and land cover. While it is generally expected that the increased water temperatures, lability of organic matter, and nutrient concentrations in agricultural streams should result in increased ecosystem respiration compared to forested systems (Griffiths et al., 2013; Tank et al., 2021), it is also expected that the geomorphologic modifications implemented in those streams to increase conveyance capacities should consistently reduce water-sediment interactions, retention times, and carbon and nutrient processing in metabolically active zones (Niyogi et al., 2004; Ocampo et al., 2020; Emanuelson et al., 2022; Dorley et al., 2023). Therefore, it is unclear if stream ER is consistently higher (or lower) in agricultural streams than in forested streams, and why. In this study, we quantified differences in ER in representative reaches of two contrasting watersheds: a 1st-order reach within a forested, sub-alpine to alpine catchment in Colorado and a 3rd-order reach in a predominantly agricultural watershed in Iowa. In each site, we conducted two rounds of experiments, each consisting of four sets of continuous injections of Cl− as a conservative tracer, resazurin as a proxy for aerobic respiration, and one of the following nutrient treatments: (a) N, (b) N + C, (c) N + P, or (d) C + N + P. The co-injection of conservative and reactive tracers and nutrients allowed us to quantify how changes in stoichiometric conditions and discharge affect respiration at the same observational spatiotemporal scales. These methods allowed us to address the following questions about the role of land use and land cover on stream ecosystem metabolism: (1) Are respiration rates correlated with conservative transport metrics in forested or agricultural streams? and (2) Can short-term modifications of stoichiometric conditions (C:N:P ratios) override respiration patterns, or do long-term physicochemical conditions control those patterns?
We conducted tracer injections in two stream reaches located in watersheds with contrasting differences in hydrology, land use, land cover, and biogeochemical regimes. In 2018, we conducted tracer studies in a 450 m stream reach of Como Creek, located in the Front Range of the Rocky Mountains in Colorado (USA). This snowmelt-driven stream, characterized by sequential pools and riffles, drains a forested and largely undisturbed watershed with about 20% alpine meadow-tundra and 80% conifer forest (Ries et al., 2017; Emanuelson et al., 2022). The streambed contains gravel and boulders, and the bedrock is shallow (Natural Resources Conservation Service, n.d.). In 2019, we conducted tracer studies in our second site, an 850-m stream reach of Clear Creek, located in an agricultural region in eastern Iowa (USA). This low-gradient system is exposed to high nutrient loading as it drains a watershed with ~93% of cultivated crops (corn and soybean) and ~6% of urban land use (Ries et al., 2017). The streambed of this reach contains a mix of silty sand and clay particles. Land cover and key site characteristics for the stream reaches in Colorado and Iowa, referred to as forested and agricultural reaches hereafter, are summarized in Figure 1 and Table 1 using information from Model My Watershed (Stroud Water Research Center, 2017).
Figure 1. Land use and land cover obtained from the U.S. Geological Survey’s (USGS) The National Map tool and on-site photos of our (A) forested stream and (B) agricultural streams.
In each reach, we completed two rounds of experiments, each consisting of four sets of injections of Cl− as a conservative tracer, resazurin (Raz) as a proxy for aerobic respiration, and one of the following nutrient treatments: (a) N, (b) N + C, (c) N + P, or (d) C + N + P. After each injection, we allowed the stream to return to ambient conditions for at least 24 h. Discharge values, nutrient injectate ratios, and resazurin masses used are summarized in Table 2. In our study, the nutrient treatments are treated as known system modifications (control variables) to alter metabolism, and we use the transformation of Raz (González-Pinzón et al., 2012, 2014, 2016; Knapp et al., 2018; Dallan et al., 2020), which occurred at the same spatiotemporal scales of the nutrient additions, to calculate how changes in stoichiometric conditions and discharge affect respiration activity.
Table 2. Site discharge, nutrient injectate ratios, and resazurin (Raz) masses added in each injection completed in the forested and agricultural reaches.
Electrical conductivity and temperature signals were recorded with Campbell Scientific CS547A sensors installed at mid-depth in the thalweg. These sensors were connected to Campbell Scientific CR1000 dataloggers to record and store the data. Tracer BTCs of Raz were sampled from the stream thalweg using grab sampling. All samples were filtered in the field through a 0.7 μm GF/F pore-size filter (Sigma-Aldrich) and refrigerated at −4°C to limit degradation. All Raz analyses took place within a week after the end of each study site. At the laboratory, each sample was buffered to a pH of 8.5 (1:10 buffer-to-sample) following Knapp et al. (2018). The fluorescence signals were measured with a Cary Eclipse Fluorescence Spectrophotometer (Agilent Technologies) using excitation/emission wavelengths of 602/632 nm for Raz and 571/584 nm for Rru and converted to concentrations based on an 8-point calibration curve (R2 = 0.99).
We calibrated the conservative transport parameters of the transient storage model presented in Eqs. 1, 2 using Cl− and stream-water electrical conductivity following Dorley et al. (2023). For this, we used a Matlab (The Mathworks Inc., Natick, Massachusetts) script from Knapp et al. (2018), which uses a joint calibration of conservative and reactive solutes through a non-linear, least-squares optimization routine to solve:
where [ML−3] and, [ML−3] are the concentrations in the main channel and aggregate transient storage zone; [L] is the distance of the study reach; [T] is time; [LT−1] and [L2T−1] are parameters representing advective flow velocity and the dispersion coefficient, respectively; [T−1] is a volumetric flux parameter accounting for lateral inputs; [T−1] is the first-order mass transfer rate coefficient parameter between the main channel and the aggregate transient storage zone; [−] is the capacity ratio parameter representing the relative contribution of transient storage-dominated to advection-dominated compartments in the stream, represented as areas along the reach; and and [T−1] are processing-rate coefficients in the main channel and transient storage zones (equaling zero for a conservative tracer).
We completed the parameter estimation using the Differential Evolution Adaptive Metropolis (DREAM [ZS]) algorithm (Vrugt et al., 2009). We jointly fit Cl− and Raz data in a first step of 100,000 model generations. We assessed model convergence using Gelman and Rubin statistics (Gelman and Rubin, 1992). The goodness of fit between measured and simulated BTCs was quantified through the calculation of the residual sum of squares, (nRSS) [−], normalized by the squared theoretical peak tracer concentrations of each tracer BTC of the respective tracer at the given location. The medians of the best 1,000 model simulations were used to assess the agreement between our final model fits and a subset of possible curve fits. The details of the model-calibration procedure that we use in this work were presented in the supporting information of Gootman et al. (2020).
We estimated conservative-transport timescales from the transport parameters to describe the transient-storage timescale, [T]; the advective timescale, [T], as the time to first reach the maximum tracer concentration or near-constant concentrations in longer injections; and the mean travel time between the injection and sampling sites, [T]:
where and are the zeroth and first-centralized temporal moments of the Cl− BTCs from each sampling site and are defined by:
where is a time index, and is the total number of samples available in a BTC.
We used non-dimensional temporal metrics to standardize for differences in reach lengths and compare conservative transport characteristics between the study reaches, i.e., and .
Since we can only get one transformation-rate coefficient from every observed BTC from the direct calibration of the transient storage model, we used the Tracer Addition for Spiraling Curve Characterization (TASCC) framework (Covino et al., 2010) to characterize uptake kinetics over the range of experimental concentrations observed. In TASCC, the ratio of reactive to conservative solute concentrations for every independent sample across the tracer BTCs is compared to the ratio of the concentrations of the injection solution to determine uptake metrics. TASCC-based transformation rate coefficients for Raz were estimated using:
Finally, we calculated reach-scale Damköhler numbers, [−], to differentiate between reaction-limited ( ) or transport-limited ( ) Raz transformation (proxy for respiration) conditions:
We calculated standard deviations (std) based on repeated measures of the distribution of the transport parameters of Eqs. 1, 2 to create upper and lower boundaries of the uncertainties in our measurements (i.e., mean ± std). Because our data were not normally distributed, we used the Mann–Whitney U nonparametric statistical test to determine if there were statistically significant differences in magnitudes within and across sites, following a similar procedure in Ensign and Doyle (2006). For the Mann–Whitney U test, we set our significance level ( , alpha) equal to 0.05.
We explored the Pearson correlation coefficients (r) between the transport parameters of Eqs. 1, 2, and associated metrics, to establish direct (r > 0.1), inverse (r < −0.1), and non-existent correlations (−0.1 < r < 0.1). We classified the strength of the correlations as uncorrelated (0 < r < |0.1|), weakly correlated (|0.1| < r < |0.5|), moderately correlated (|0.5| < r < |0.8|), strongly correlated (|0.8| < r < |1.0|) and included p-values for each correlation.
We compared discharges ( ), calibrated conservative transport parameters (mean velocity, ; mass-transfer rate coefficients, ; and the relative contribution of transient storage-dominated to advection-dominated compartments in the stream ), and non-dimensional temporal metrics ( and ) to identify differences in transport processes between our contrasting study reaches. We found that , , , and were significantly greater in the agricultural reach, whereas values were significantly greater in the forested reach (Figure 2).
Figure 2. Transport parameters and non-dimensional time metrics for the study reaches. Comparisons are based on the combination of eight nutrient injections conducted at each site (e.g., two rounds of N, N + C, N + P, and C + N + P treatments). Asterisks represent significant differences in magnitudes between rounds with p < 0.01 (**) and p ~ 0 (****) based on the Mann–Whitney U nonparametric statistical test.
Greater discharges in the agricultural reach are expected due to the size of the contributing watershed (Table 1). Despite featuring greater longitudinal slopes, the forested stream had slower velocities due to increased channel roughness and smaller hydraulic radius. Greater mass-transfer rates, , and correspondingly shorter transient-storage timescales, , suggest the existence of transient storage zones that are loaded and unloaded much faster in the agricultural reach. These results agree with our perceptual model and field observations suggesting that the continuous dredging and straightening of an agricultural channel increases its drainage (advective) capacity, as reflected in greater ratios, but also creates recirculation zones along the banks when sediments get deposited and plants grow under low-flow conditions [cf. Figure 6 in Emanuelson et al. (2022)]. Due to the low permeabilities of the streambed sediments (i.e., clay and silt, Table 1), transient storage largely occurred within the main channel and was short-lived in the agricultural reach.
Greater values in the forested reach indicate a greater size-based contribution of transient storage zones in proportion to the main channel. This result, combined with smaller values, suggest that water entering storage zones in the forested reach remains there much longer than in the agricultural reach. Since the forested reach has a shallow bedrock, most transient storage occurred in lateral pools that were loaded during higher flows and became less connected during flow recession periods or even during diel fluctuations forced by evapotranspiration. Interestingly, the ratios were not significantly different between reaches, suggesting that transient storage processes scaled about equally in proportion to mean travel times , which generally decrease with discharge and increase with reach length (Kilpatrick and Wilson, 1989; Jobson, 1997; Camacho and González, 2008). To summarize, we found that both stream reaches had limited interactions with the subsurface (i.e., shallow bedrock in the forested stream and impermeable streambed textures in the agricultural stream), and that surface transient storage zones were loaded and unloaded faster in the agricultural stream (greater values), causing shorter transient storage timescales there ( ). However, when the transient storage timescales were normalized by the mean travel time, we found no significant differences between the two reaches, which suggests that transient storage was proportional to discharge and reach length.
We analyzed changes in the transformation rate coefficients of Raz ( ) with respect to discharge ( ) and the non-dimensional metrics and to identify how comparable physical characteristics influenced stream respiration (Figure 3). We found consistently greater in the forested stream (p ~ 0, Figure 3), but no correlations (p > 0.05) between and each of the three metrics of conservative transport ( and in either reach (Figures 2A–C), or and (not shown). We also analyzed trends within each reach using the dimensional timescales, and found that decreased strongly with discharge in both reaches (R > 0.92 and p < 0.05; Figure 3D), but found no correlations (p > 0.05) between , , and (Figures 3E,F). To summarize, we consistently found greater respiration activity in the forested stream despite the mean water temperature of 9.3°C during the experiments was almost half of that in the agricultural reach (Table 1), but no correlations between respiration, discharge, and advective or transient storage timescales within a given reach.
Figure 3. Correlation plots between the mean values of the transformation rate coefficients of Raz ( ) from each tracer experiment and discharge (A); the non-dimensional conservative transport metrics (B), and (C); the advective timescale (E); and the transient storage timescale (F). Panel (D) shows the correlation between the advective timescale and discharge.
Combining the results from the two rounds of nutrient treatments completed in each reach (e.g., N vs. N), we found significantly greater (p ~ 0) discharges, , and significantly smaller (p ~ 0) transformation rate coefficients of Raz, , in the agricultural reach for the same nutrient treatment (Figure 4). Comparing combined data from the two rounds from each study reach (e.g., N vs. N + C in the forested stream), we found that neither nor were significantly different across nutrient treatments.
Figure 4. Combined discharge (A) and transformation rate coefficients of Raz (B) for the two rounds of experiments conducted in the agricultural and forested reaches organized by nutrient treatment. Asterisks represent significant differences in magnitudes for treatments N, N + C, N + P, and C + N + P with p < 0.05 (*), p < 0.01 (**), and p ~ 0 (****) based on the Mann–Whitney U nonparametric statistical test.
We compared the timescale of the transformation of Raz, which indicates oxygen consumption through aerobic respiration, with respect to the transient storage timescale at both reaches. For this, we used the framework of the non-dimensional Damköhler number (Harvey et al., 2013; Pinay et al., 2015; Krause et al., 2017; Ocampo et al., 2020). Figure 5 shows that the agricultural reach was characterized by reaction-limited conditions, i.e., Da << 1, indicating the suboptimal utilization of resources that become available to transient storage zones. Since transient storage zones are ecotones with some of the most metabolically active compartments found in stream networks (Gooseff et al., 2004; Covino et al., 2010, 2011; Knapp et al., 2017; Gootman et al., 2020), reaction-limited transport typically indicates the existence of nutrient or carbon limitations to metabolism or the absence of enough biomass due to recent unfavorable conditions. In contrast, the forested reach was characterized by Da ~ 1, suggesting the existence of conditions that favored a more optimal utilization of resources supplied to transient storage zones, i.e., the transformation and transient storage timescales were nearly balanced. Even though our experiments featured the addition of multiple nutrient treatments (i.e., N, N + C, N + P, C + N + P) to favor different stoichiometric conditions that could influence stream respiration (inferred through Raz transformation), our results show clear clustering of site-specific Da values (Figure 5). This result suggests that the effects of our nutrient additions were not more influential than the other highly contrasting physicochemical characteristics of the two study reaches and watersheds.
Figure 5. Transformation timescale vs. transient storage time scale compared across the agricultural and forested sites. Da is the Damköhler number, which can be calculated as a ratio of the transient storage timescale to the transformation timescale and serves as an indicator for reaction- vs. transport-limited conditions.
From our field sampling, ambient concentrations of nitrate averaged 0.035 (±0.002) mg/L in the forested reach and 5.727 (±0.688) mg/L in the agricultural reach, i.e., a two-order of magnitude difference. We corroborated the trends found in our values with Smith et al. (2003), who generated estimates of background total nitrogen (TN) and total phosphorous (TP) yield and concentrations throughout the stream-river network in 14 ecoregions of the conterminous U.S. That study found 75th % quartile TN = 0.21 (±0.05) mg/L and TP = 0.02 (±0.005) for the Western Forested Mountains region, where our forested reach is located. Similarly, Smith et al. (2003) found 75th % quartile TN = 0.62 (±0.26) mg/L and TP = 0.06 (±0.020) for the Corn Belt and Northern Great Plains region, where our agricultural reach is located. Our results and those from Smith et al. (2003) suggest clear differences in ambient stoichiometric conditions, which are associated with the drastic differences in land use and land cover in our two study watersheds, and most likely influence the significant differences in respiration activities found.
Hill et al. (2017) analyzed 1879 streams and rivers across the continental U.S. and found increased microbial respiration from east to west. When the data were organized by U.S. ecoregions, the weighted mean (±standard error) of stream microbial respiration in headwaters located in the Western Mountains region was 6.99 (±2.72) mol C m−2 d−1, and 0.59 (±0.22) mol C m−2 d−1 in second-to-third order streams for the Temperate Plains region. Our findings of greater respiration activities in Como Creek, the forested stream, agree with those from the metanalysis by Hill et al. One of the most consequential effects of these findings is that headwater streams in the Western Mountains region are expected to generate an order of magnitude higher respiratory C losses than second and third-order streams in the Temperate Plains region.
To summarize, we found that our nutrient treatment injections had a minimal influence on the significantly different respiration rates. This result is most likely due to the more significant influence of ambient nutrient concentrations on metabolic processes at the two contrasting reaches and watersheds we studied. Interestingly, all the experiments conducted in the agricultural stream featured a reaction-limited transformation of Raz, a proxy for aerobic respiration, suggesting the existence of nutrient or carbon limitations that our short-term nutrient treatments did not remove. Beyond limitations associated with nutrients or carbon, biomass limitation is also plausible. Contrary to the agricultural site, the forested stream featured a greater and more balanced transformation of Raz with respect to the transient storage timescales.
This study quantified stream respiration differences between two contrasting stream ecosystems (forested vs. agricultural) to answer: (1) Are respiration rates correlated with conservative transport metrics in forested or agricultural streams? and (2) Can short-term modifications of stoichiometric conditions (C:N:P ratios) override respiration patterns, or do long-term physicochemical conditions control those patterns? Regarding research question (1), our results showed that both stream reaches had limited interactions with the subsurface (i.e., shallow bedrock in the forested stream and impermeable streambed textures in the agricultural stream), and that surface transient storage zones were loaded and unloaded faster in the agricultural stream (greater values), causing shorter transient storage timescales there ( ). However, when the transient storage timescales were normalized by the mean travel time, we found no significant differences between the two reaches, which suggests that transient storage was similarly proportional to discharge and reach length. Interestingly, we consistently found greater respiration activity in the forested stream, but no correlations between respiration, discharge, and advective or transient-storage time-scale metrics associated with conservative transport.
With regards to research question (2), our results showed that our nutrient treatments had a negligible influence in the significantly different respiration rates between the two sites. Due to the lack of correlations between respiration, discharge, and timescales and the minimal influence of our nutrient treatments in the results, it is likely that respiration rates are mainly influenced by differences in ambient nutrient concentrations at each site, and associated differences in microbial composition and function. Our results agree with large-scale analyses suggesting greater microbial respiration in headwater streams in the Western Mountains region, compared to second-to-third order streams in the temperate plains region.
The raw data supporting the conclusions of this article will be made available by the authors, without undue reservation.
JD: Conceptualization, Data curation, Formal analysis, Funding acquisition, Investigation, Methodology, Project administration, Resources, Software, Supervision, Validation, Visualization, Writing – original draft, Writing – review & editing. JS: Investigation, Methodology, Writing – review & editing. TC: Investigation, Methodology, Writing – review & editing. KS: Investigation, Methodology, Writing – review & editing. MG: Investigation, Methodology, Writing – review & editing. RG-P: Investigation, Methodology, Writing – review & editing, Conceptualization, Data curation, Formal analysis, Funding acquisition, Project administration, Resources, Software, Supervision, Validation, Visualization, Writing – original draft.
The author(s) declare that financial support was received for the research, authorship, and/or publication of this article. The National Science Foundation provided funding support through grants NSF EAR-1642399, NSF EAR-1642368, NSF EAR-1642402, NSF EAR-1642403, and NSF 1914490.
We thank Karin Emanuelson, Jackie Randell, Ruendy Castillo, Antonio Jesus Perez, and Lillian Jones for their field and laboratory assistance.
The authors declare that the research was conducted in the absence of any commercial or financial relationships that could be construed as a potential conflict of interest.
All claims expressed in this article are solely those of the authors and do not necessarily represent those of their affiliated organizations, or those of the publisher, the editors and the reviewers. Any product that may be evaluated in this article, or claim that may be made by its manufacturer, is not guaranteed or endorsed by the publisher.
Bahr, J. M., and Rubin, J. (1987). Direct comparison of kinetic and local equilibrium formulations for solute transport affected by surface reactions. Water Resour. Res. 23, 438–452. doi: 10.1029/WR023i003p00438
Camacho, L. A., and González, R. A. (2008). Calibration and predictive ability analysis of longitudinal solute transport models in mountain streams. Environ. Fluid Mech. 8, 597–604. doi: 10.1007/s10652-008-9109-0
Covino, T. P., McGlynn, B., and Mallard, J. (2011). Stream-groundwater exchange and hydrologic turnover at the network scale. Water Resour. Res. 47, 1–11. doi: 10.1029/2011WR010942
Covino, T. P., Mcglynn, B. L., and Mcnamara, R. A. (2010). Tracer additions for spiraling curve characterization (TASCC): quantifying stream nutrient uptake kinetics from ambient to saturation. Limnol. Oceanogr. Methods 8, 484–498. doi: 10.4319/lom.2010.8.484
Crutzen, P. J. (2006). The anthropocene, Earth system science in the Anthropocene, Eds. E. Ehlers and T. Krafft (Berlin, Heidelberg: Springer), 13–18
Dallan, E., Regier, P., Marion, A., and González-Pinzón, R. (2020). Does the mass balance of the reactive tracers Resazurin and Resorufin close at the microbial scale? J. Geophys. Res. Biogeosciences 125, 1–10. doi: 10.1029/2019JG005435
Dorley, J., Singley, J., Covino, T., Singha, K., Gooseff, M., Van Horn, D., et al. (2023). Physical and stoichiometric controls on stream respiration in a headwater stream. Biogeosciences 20, 3353–3366. doi: 10.5194/bg-20-3353-2023
Downing, J. A., Mcclain, M., Twilley, R., Melack, J. M., Elser, J., Rabalais, N. N., et al. (1999). Influence of land use on water quality. Biogeochemistry 46, 109–148. doi: 10.1007/BF01007576
Emanuelson, K., Covino, T. P., Ward, A. S., Dorley, J., and Gooseff, M. N. (2022). Conservative solute transport processes and associated transient storage mechanisms: comparing streams with contrasting channel morphologies, land use and land cover. Hydrol. Process. 36:36. doi: 10.1002/hyp.14564
Ensign, S. H., and Doyle, M. W. (2006). Nutrient spiraling in streams and river networks. J. Geophys. Res. Biogeosciences 111:e114. doi: 10.1029/2005jg000114
Gelman, A., and Rubin, D. B. (1992). Inference from iterative simulation using multiple sequences. Stat. Sci. 7:11136. doi: 10.1214/ss/1177011136
Gomes, E., Inácio, M., Bogdzevič, K., Kalinauskas, M., Karnauskaitė, D., and Pereira, P. (2021). Future land-use changes and its impacts on terrestrial ecosystem services: a review. Sci. Total Environ. 781:146716. doi: 10.1016/j.scitotenv.2021.146716
González-Pinzón, R., Haggerty, R., and Argerich, A. (2014). Quantifying spatial differences in metabolism in headwater streams. Freshw. Sci. 33, 798–811. doi: 10.1086/677555
González-Pinzón, R., Haggerty, R., and Myrold, D. D. (2012). Measuring aerobic respiration in stream ecosystems using the resazurin-resorufin system. J. Geophys. Res. Biogeosciences 117, 1–10. doi: 10.1029/2012JG001965
González-Pinzón, R., Peipoch, M., Haggerty, R., Martí, E., and Fleckenstein, J. H. (2016). Nighttime and daytime respiration in a headwater stream. Ecohydrology 9, 93–100. doi: 10.1002/eco.1615
Gooseff, M. N., McKnight, D. M., Runkel, R. L., and Duff, J. H. (2004). Denitrification and hydrologic transient storage in a glacial meltwater stream, McMurdo dry valleys, Antarctica. Limnol. Oceanogr. 49, 1884–1895. doi: 10.4319/lo.2004.49.5.1884
Gootman, K. S., González-Pinzón, R., Knapp, J. L. A., Garayburu-Caruso, V., and Cable, J. (2020). Spatiotemporal variability in transport and reactive processes across a first‐ to fifth‐order fluvial network. Water Resour. Res. 56, 1–18. doi: 10.1029/2019WR026303
Grace, M. R., Giling, D. P., Hladyz, S., Caron, V., Thompson, R. M., and Mac Nally, R. (2015). Fast processing of diel oxygen curves: Estimating stream metabolism with BASE (BAyesian Single-station Estimation). Limnology and Oceanography: Methods 13, e10011. doi: 10.1002/lom3.10011
Griffiths, N. A., Tank, J. L., Royer, T. V., Roley, S. S., Rosi-Marshall, E. J., Whiles, M. R., et al. (2013). Agricultural land use alters the seasonality and magnitude of stream metabolism. Limnol. Oceanogr. 58, 1513–1529. doi: 10.4319/lo.2013.58.4.1513
Harvey, J. W., Böhlke, J. K., Voytek, M. A., Scott, D., and Tobias, C. R. (2013). Hyporheic zone denitrification: controls on effective reaction depth and contribution to whole-stream mass balance. Water Resour. Res. 49, 6298–6316. doi: 10.1002/wrcr.20492
He, J. F., Liu, J. Y., Zhuang, D. F., Zhang, W., and Liu, M. L. (2007). Assessing the effect of land use/land cover change on the change of urban heat island intensity. Theor. Appl. Climatol. 90, 217–226. doi: 10.1007/s00704-006-0273-1
Hill, B. H., Elonen, C. M., Herlihy, A. T., Jicha, T. M., and Mitchell, R. M. (2017). A synoptic survey of microbial respiration, organic matter decomposition, and carbon efflux in U.S. streams and rivers. Limnol. Oceanogr. 62, S147–S159. doi: 10.1002/lno.10583
Jobson, H. E. (1997). Predicting travel time and dispersion in rivers and streams. Hydraul. Eng. 123, 971–978. doi: 10.1061/(ASCE)0733-9429(1997)123:11(971)
Kilpatrick, F. A., and Wilson, F. J. (1989). Measurement of time of travel in streams by dye tracing, A9.
Knapp, J. L. A., González-Pinzón, R., Drummond, J. D., Larsen, L. G., Cirpka, O. A., and Harvey, J. W. (2017). Tracer-based characterization of hyporheic exchange and benthic biolayers in streams. Water Resour. Res. 53, 1575–1594. doi: 10.1002/2016WR019393
Knapp, J. L. A., González-Pinzón, R., and Haggerty, R. (2018). The Resazurin-Resorufin system: insights from a decade of “smart” tracer development for hydrologic applications. Water Resour. Res. 54, 6877–6889. doi: 10.1029/2018WR023103
Krause, S., Lewandowski, J., Grimm, N. B., Hannah, D. M., Pinay, G., McDonald, K., et al. (2017). Ecohydrological interfaces as hot spots of ecosystem processes: ECOHYDROLOGICAL INTERFACES AS HOT SPOTS. Water Resour. Res. 53, 6359–6376. doi: 10.1002/2016WR019516
Lewis, W. M., Melack, J. M., McDowell, W. H., McClain, M., and Richey, J. E. (1999). Nitrogen yields from undisturbed watersheds in the Americas. Biogeochemistry 46, 149–162. doi: 10.1007/BF01007577
Li, X., Cheng, G., Lin, H., Cai, X., Fang, M., Ge, Y., et al. (2018). Watershed system model: the essentials to model complex human-nature system at the River Basin scale. J. Geophys. Res. Atmospheres 123, 3019–3034. doi: 10.1002/2017JD028154
Maaß, A.-L., Schüttrumpf, H., and Lehmkuhl, F. (2021). Human impact on fluvial systems in Europe with special regard to today’s river restorations. Environ. Sci. Eur. 33:119. doi: 10.1186/s12302-021-00561-4
Newbold, T., Hudson, L. N., Hill, S. L. L., Contu, S., Lysenko, I., Senior, R. A., et al. (2015). Global effects of land use on local terrestrial biodiversity. Nature 520, 45–50. doi: 10.1038/nature14324
Niyogi, D. K., Simon, K. S., and Townsend, C. R. (2004). Land use and stream ecosystem functioning: nutrient uptake in streams that contrast in agricultural development. Arch. Für Hydrobiol. 160, 471–486. doi: 10.1127/0003-9136/2004/0160-0471
Ocampo, C., Oldham, C., and Sivapalan, M. (2020). Nitrate attenuation in agricultural catchments: shifting balances between transport and reaction—Ocampo—2006—water resources research : Wiley Online Library.
Odum, H. T. (1956). Primary Production in Flowing Waters. Limnology and Oceanography 1, 102–117. doi: 10.4319/lo.1956.1.2.0102
Paul, V., Sankar, M. S., Vattikuti, S., Dash, P., and Arslan, Z. (2021). Pollution assessment and land use land cover influence on trace metal distribution in sediments from five aquatic systems in southern USA. Chemosphere 263:128243. doi: 10.1016/j.chemosphere.2020.128243
Pinay, G., Peiffer, S., De Dreuzy, J.-R., Krause, S., Hannah, D. M., Fleckenstein, J. H., et al. (2015). Upscaling nitrogen removal capacity from local hotspots to low stream orders’ drainage basins. Ecosystems 18, 1101–1120. doi: 10.1007/s10021-015-9878-5
Rahaman, Z. A., Kafy, A.-A., Saha, M., Rahim, A. A., Almulhim, A. I., Rahaman, S. N., et al. (2022). Assessing the impacts of vegetation cover loss on surface temperature, urban heat island and carbon emission in Penang city, Malaysia. Build. Environ. 222:109335. doi: 10.1016/j.buildenv.2022.109335
Regier, P. J., González-Pinzón, R., Van Horn, D. J., Reale, J. K., Nichols, J., and Khandewal, A. (2020). Water quality impacts of urban and non-urban arid-land runoff on the Rio Grande. Sci. Total Environ. 729:138443. doi: 10.1016/j.scitotenv.2020.138443
Ries, K. G., Newson, J. K., Smith, M. J., Guthrie, J. D., Steeves, P. A., Haluska, T., et al. (2017). StreamStats, version 4. VA: Reston.
Sleeter, B. M., Liu, J., Daniel, C., Rayfield, B., Sherba, J., Hawbaker, T. J., et al. (2018). Effects of contemporary land-use and land-cover change on the carbon balance of terrestrial ecosystems in the United States. Environ. Res. Lett. 13:e540. doi: 10.1088/1748-9326/aab540
Smith, R. A., Alexander, R. B., and Schwarz, G. E. (2003). Natural background concentrations of nutrients in streams and rivers of the conterminous United States. Environ. Sci. Technol. 37, 3039–3047. doi: 10.1021/es020663b
Stroud Water Research Center (2017). Model My Watershed [Software]. Available at: https://wikiwatershed.org/ and http://wikiwatershed.org/model.php
Tank, J. L., Speir, S. L., Sethna, L. R., and Royer, T. V. (2021). The case for studying highly modified agricultural streams: farming for biogeochemical insights. Limnol. Oceanogr. Bull. 30, 41–47. doi: 10.1002/lob.10436
Tasser, E., Tappeiner, U., and Cernusca, A. (2005). Ecological effects of land-use changes in the European Alps. Global Change and Mountain Regions: An Overview of Current Knowledge. 409–420
Vannote, R. L., Minshall, G. W., Cummins, K. W., Sedell, J. R., and Cushing, C. E. (1980). The River Continuum Concept. Canadian Journal of Fisheries and Aquatic Sciences 37, 130–137. doi: 10.1139/f80-017
Vrugt, J. A., Ter Braak, C. J. F., Diks, C. G. H., Robinson, B. A., Hyman, J. M., and Higdon, D. (2009). Accelerating Markov chain Monte Carlo simulation by differential evolution with self-adaptive randomized subspace sampling. Int. J. Nonlinear Sci. Numer. Simul. 10:e273. doi: 10.1515/IJNSNS.2009.10.3.273
Wang, G., Mang, S., Cai, H., Liu, S., Zhang, Z., Wang, L., et al. (2016). Integrated watershed management: evolution, development and emerging trends. J. For. Res. 27, 967–994. doi: 10.1007/s11676-016-0293-3
Watson, S. J., Luck, G. W., Spooner, P. G., and Watson, D. M. (2018). Land-u sequence change, vol. 12, 241–249.
Webster, J. R. (2007). Spiraling down the river continuum: stream ecology and the U-shaped curve. Journal of the North American Benthological Society 26, 375–389. doi: 10.1899/06-095.1
Wilson, C. G., Abban, B., Keefer, L. L., Wacha, K., Dermisis, D., Giannopoulos, C., et al. (2018). The intensively managed landscape critical zone observatory: a scientific testbed for understanding critical zone processes in agroecosystems. Vadose Zone J. 17, 1–21. doi: 10.2136/vzj2018.04.0088
Wohl, E., Lane, S. N., and Wilcox, A. C. (2015). The science and practice of river restoration. Water Resour. Res. 51, 5974–5997. doi: 10.1002/2014wr016874
Wu, W., Dong, C., Wu, J., Liu, X., Wu, Y., Chen, X., et al. (2017). Ecological effects of soil properties and metal concentrations on the composition and diversity of microbial communities associated with land use patterns in an electronic waste recycling region. Sci. Total Environ. 601–602, 57–65. doi: 10.1016/j.scitotenv.2017.05.165
Keywords: aquatic respiration, land use, forested, agricultural, stream, stream metabolism
Citation: Dorley J, Singley J, Covino T, Singha K, Gooseff MN and González-Pinzón R (2024) Differences in aquatic respiration in two contrasting streams: forested vs. agricultural. Front. Water. 6:1397168. doi: 10.3389/frwa.2024.1397168
Received: 07 March 2024; Accepted: 11 July 2024;
Published: 09 August 2024.
Edited by:
Prabhakar Sharma, Nagaland University, IndiaReviewed by:
Jacinto Elías Sedeño-Díaz, Instituto Politécnico Nacional (IPN), MexicoCopyright © 2024 Dorley, Singley, Covino, Singha, Gooseff and González-Pinzón. This is an open-access article distributed under the terms of the Creative Commons Attribution License (CC BY). The use, distribution or reproduction in other forums is permitted, provided the original author(s) and the copyright owner(s) are credited and that the original publication in this journal is cited, in accordance with accepted academic practice. No use, distribution or reproduction is permitted which does not comply with these terms.
*Correspondence: Ricardo González-Pinzón, Z29uemFyaWNAdW5tLmVkdQ==
Disclaimer: All claims expressed in this article are solely those of the authors and do not necessarily represent those of their affiliated organizations, or those of the publisher, the editors and the reviewers. Any product that may be evaluated in this article or claim that may be made by its manufacturer is not guaranteed or endorsed by the publisher.
Research integrity at Frontiers
Learn more about the work of our research integrity team to safeguard the quality of each article we publish.