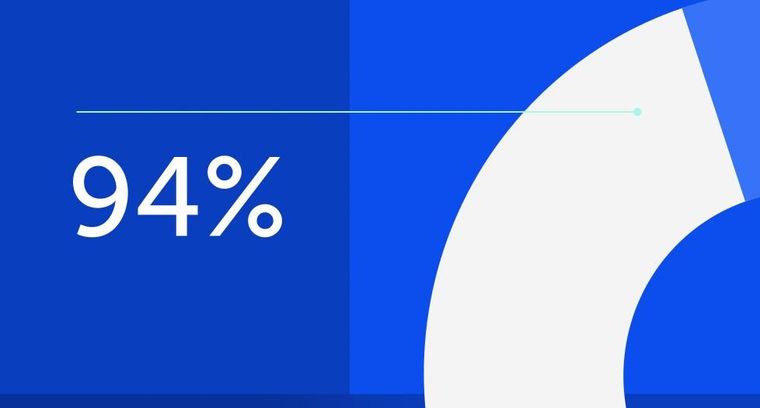
94% of researchers rate our articles as excellent or good
Learn more about the work of our research integrity team to safeguard the quality of each article we publish.
Find out more
ORIGINAL RESEARCH article
Front. Water, 01 July 2024
Sec. Water and Built Environment
Volume 6 - 2024 | https://doi.org/10.3389/frwa.2024.1383382
Rain gardens are an alternative to traditional drainage, able to lower flood risk and reduce environmental contamination from stormwater. Removal of contaminants by rain gardens is driven by both physical processes (such as filtration and sedimentation) and biological metabolic processes by soil microorganisms. To better understand rain garden performance, this study explored the impact of rain gardens on pollution removal and microbial composition and function using rain gardens fed real stormwater from a busy road. Each rain garden had different grain size and hydraulic conductivities as these parameters have been argued to impact pollution removal. All four rain gardens were able to reduce the contaminant load in the stormwaters, reducing the concentration of dissolved metals, suspended solids and chemical oxygen demand. Significantly, road salting in the winter did not cause dissolved metals to be released from the rain gardens, suggesting that rain gardens can continue to reduce contaminant loads during winter salting regimes. Some variation in pollutant removal was seen between the soils tested, but overall no clear trend could be identified based on grain size and hydraulic conductivity with all rain gardens performing broadly similarly. The rain garden soil altered the microbial community in the stormwater, resulting in greater taxonomic evenness and functional richness in the effluent water compared to the influent. Functional richness of the soils was also higher than that of the input waters, indicating that the microbes in the rain gardens were able to perform a wider range of functions than those of the influent. Effluent and soil microbiology was more impacted by sampling date than soil grain size, which may be a result of the soil communities maturing and changing over time. As greater numbers of rain gardens are installed to tackle flooding from climate change, it is important to ensure the environment is protected from urban contaminants in the stormwater. The results in this study further highlight the ability of rain gardens to undertake this important task.
Impermeable surfaces such as roads, pavements, and roofs prevent direct infiltration of rainwater into the ground. Urbanisation increases the proportion of rainfall that becomes runoff from 10 to 55%, when compared to permeable areas such as woodland (Shafique, 2016). Problematically, the high volume and flow rate of surface runoff during storm events can lead to flooding, a problem which will be exacerbated by increased rainfall and storminess driven by climate change (Charlesworth, 2010). Traditional drainage systems such as combined sewers are not resilient against the impacts of climate change and are at risk of sewer overflow (Steis Thorsby et al., 2020), resulting in the discharge of wastewater to the environment.
Sustainable urban drainage systems (SUDS) offer an alternative to conventional drainage to manage stormwater. SUDS and the principles behind them may also be referred to as low impact development (LID), best management practices (BMPs), stormwater control measures (SCMs), and water sensitive urban design (WSUD) (Fletcher et al., 2015). They are able to lower flood risk by slowing stormwater flow or allowing infiltration to soil, as well as providing in situ water treatment to reduce the impact of stormwater pollutants on the environment (Woods Ballard et al., 2015).
Guidance for SUDS design is often based on the system’s ability to control water quantity rather than water quality (Jefferies et al., 2008; Hong et al., 2018), despite evidence that the ability of SUDS to remove pollutants varies depending on the materials used. Pollutant removal is important as stormwater from urban runoff can contain a wide range of contaminants. Solids and sediment pollution may arise from sources including vehicle corrosion, soil erosion, road salting, washing and weathering of roofs, roads and buildings, atmospheric deposition, and vehicle exhaust emissions (D’Arcy et al., 1998; Fewkes, 2012; Woods Ballard et al., 2015; Müller et al., 2020; Nyström et al., 2020). Hydrocarbon pollution is often a result of exhaust emissions, accidental oil spills, and incorrect disposal of chemicals (D’Arcy et al., 1998; Woods Ballard et al., 2015), leaves and decomposing organisms (Kennedy et al., 2016). Potentially toxic elements (PTEs) typically include metals and arsenic and have wide-ranging sources. For example, zinc is released from wheels, tyre filler, brakes, and engine oil (Davis et al., 2001; Müller et al., 2020). Cadmium, copper, lead, and iron are also released by wheels and tyres, while brake wear releases copper, nickel, antimony, lead, and cadmium (Müller et al., 2020). Nitrogen and phosphorus pollution may arise from fertiliser use in green spaces, traffic fumes, leaching from soil, fallen leaves, atmospheric deposition, or animal urine and faeces (D’Arcy et al., 1998; Hunt et al., 2012; Woods Ballard et al., 2015; Müller et al., 2020).
Rain gardens are a form of SUDS that typically consist of landscaped areas containing soil and plants. The composition of the soil mix used in rain gardens can impact the system’s hydrology by controlling the speed of infiltration, which in turn may impact pollutant removal, with slower infiltration argued to allow more time for sequestration and degradation of contaminants (Carpenter and Hallam, 2010; Hunt et al., 2012; Zhang L. et al., 2021). Previous studies have shown that changes in soil composition (such as variation in proportions of sand, soil and clay as well as the addition of modifiers including compost and wastewater treatment by-products) impact metal removal efficiency (Gülbaz et al., 2015; Jay et al., 2019). Finer soil components such as clay have higher surface areas and generally higher concentrations of soil organic matter compared to coarse soil fractions such as sand. This results in greater sorption capacity for metals and hydrocarbons on both the organic matter and soil minerals themselves (Quenea et al., 2009; Zhang et al., 2022). Finer grain sizes will also reduce pore throat size, which increases filtering of particulate pollutants.
Soil composition may also affect the microbial community that resides within the rain gardens (Ulrich et al., 2017). This is significant as microbial communities are known to play an important role in pollutant biodegradation and immobilization processes, including reduction/oxidation of metals (which can drive their immobilization through precipitation), degradation and oxidation of organic compounds, and the adsorption of metals onto microbial surfaces (Jefferies et al., 2008; Ayilara and Babalola, 2023). Thus, changes in rain garden microbiology could cause changes in the ability of microbes to drive pollutant biodegradation and immobilization. Previous studies of the microbial communities of rain gardens found that community composition was impacted by factors including drainage type, organic matter content, and influent quality (Hong et al., 2018; Zhang et al., 2024).
Understanding the microbial community composition of effluent waters from rain gardens is useful as it reveals the microorganisms that that are being released into the natural environment and, moreover, can provide an indication of the microbes that are in the rain garden itself. Microbial composition of output waters has been examined in stormwater quality improvement devices (Liguori et al., 2021), but the microbial ecology of output waters from rain gardens has yet to be examined. Examination of output waters is also useful to indicate how the rain garden may drive changes in the functional capability of microbes in the output stormwater compared to the input stormwater. In comparison to laboratory-based studies, there has been far less research into the microbiology and water quality of rain gardens in the field, and thus more studies on real systems are needed to confirm laboratory observations. Moreover, while soil grain size and hydraulic conductivity have been stated to play an important impact on pollutant removal, no study has yet explored the impact of these on both the microbial ecology and water quality of a real rain garden system. To explore this, we examined water quality and microbial ecology of four pilot rain gardens with different grain size distributions and hydraulic conductivities. These rain gardens each received the same input stormwater and contained the same plant species. Samples were collected for water quality and genomic analysis over several months to examine how water quality and microbial ecology changed over time. In addition to taxonomic diversity analysis, this study undertakes detailed functional alpha and beta diversity analysis on real rain garden systems for the first time. Moreover, we have applied this to input and output waters as well as soil, enabling us to understand how the taxonomic and functional diversity changes through the system.
A system consisting of four rain gardens with a shared stormwater input and individual outputs was installed in Glasgow, UK during March 2019. The rain garden system was designed to take in stormwater from a short section of the adjacent street, which is a busy road that runs across Glasgow and connects to the M8 motorway close to the rain garden site. Stormwater drained into a trough before baffles within the trough distributed the water evenly between the four rain gardens. An open outflow tap near the base of each rain garden allowed water to flow out of each system (Figure 1).
Figure 1. Test rain garden system in Glasgow. (A) Prior to the addition of soil and plants, (B) shortly after installation in March 2019 and (C) in March 2021. (D) Cross section schematic of the rain garden system.
The four rain gardens (referred to as A, B, C & D) differed in the particle size distribution (Figure 2) and hydraulic conductivity of their soil mix (hydraulic conductivity A = 258.2; B = 105.4, C = 152.1; D = 47.1 mm/h; full particle size distributions shown in Supplementary Table S1). All four rain gardens contained the same plant species in the same layout (Supplementary Table S2), to avoid variation in phytoremediation potential, e.g., due to varying root length. Rain garden maintenance included litter removal and weeding for 12 months post-installation.
Water samples were collected from the rain gardens in May, August, and December of 2019. While more frequent analysis intervals can give greater time-dependent detail on pollutant behaviour, here we sampled less frequently, but analysed more parameters across water chemistry and microbiology to give an in-depth view of the system at those times. This study focused on a newly installed rain garden system. This is pertinent as there is a considerable push to install new green infrastructure, such as rain gardens, in urban environments and thus we can expect an increasing number of newly installed systems each year. Understanding their behaviour shortly after installation is therefore highly relevant.
Due to an issue with flow distribution, May samples were taken only from the input and outputs A and C. Flow distribution was corrected by mending the input baffle. We visited the site regularly to confirm that the flow distribution problem had been rectified. Samples were taken during run-off events and input and output samples were taken at the same time. The volume of rainfall for the week preceding sampling was lowest in May (37.4 mm), with greater volumes in August (61 mm) and December (76 mm).
The pH of samples destined for organic and elemental analyses was lowered to 2 with HCl and HNO3, respectively. Samples were stored at 4° C until analysis.
Water samples were analysed for pH and electrical conductivity (EC) using a pH/EC meter (Mettler-Toledo), immediately upon return to the laboratory after sample collection. Concentrations of total suspended solids (TSS) (May, August, December) and total dissolved solids (TDS) (May, August) were measured gravimetrically (filtration through 1.2 μm glass fibre filter paper, drying at 105o C and 180o C respectively). TDS was measured using the pH/EC meter in December. Further detailed analysis of suspended solid composition was not carried out in this study. However elemental and anion analyses were performed on filtered samples, to identify components of the TDS.
Dissolved element analysis was undertaken on filtered (0.45 μm mixed cellulose ester [MCE] filters) water samples. Element concentrations were determined by ICP-OES on an iCAP 6,000 Series ICP Emission Spectrometer with ultrasonic nebuliser (Thermo Fisher Scientific). The following elements were analysed: Al, As, Cd, Cr, Cu, Fe, Mn, Ni, Pb, and Zn.
Chemical oxygen demand (COD) was measured using COD cuvette tests (Hach, 0–150 mg/L test kits) on both filtered (0.45 μm MCE filters) and unfiltered samples to determine the COD associated with dissolved and particulate fractions. COD is a measure of the amount of material in the water that is oxidizable by a strong chemical oxidant. While generally considered to be dominated by oxidisable organic matter, it can also include nitrites and sulphides etc. (Li and Liu, 2019). The test also does not distinguish between organic types, e.g., plant debris vs. animal waste vs. petroleum and oil, instead providing an overall view of COD concentration.
Dissolved anion concentrations (fluoride, bromide, chloride, nitrite, nitrate, sulphate, phosphate) were determined by ion chromatography on a Metrohm 850 Professional IC. Ammonium concentrations were determined colorimetrically using a Konelab analyser. For both anion and ammonium measurement, samples were filtered through 0.45 μm MCE syringe filters prior to analysis.
Water samples were stored at 4° C prior to vacuum filtration through Nalgene Analytical Filter Test Funnels (0.2 μm, cellulose nitrate). In May, a single replicate of each sample was filtered. For August and December, three replicates of each sample were filtered. The funnels were stored at 4° C until DNA extraction. DNA extraction was performed using the QIAGEN DNeasy PowerWater kit, according to the manufacturer’s instructions. Filters were cut into smaller pieces prior to DNA extraction using ethanol-sterilised scissors. DNA was eluted into 50 μL of elution buffer and stored at −80° C. DNA concentrations were measured using a Biotek Epoch Microplate Spectrophotometer.
Sequencing was carried out using the standard Illumina 16S protocol (Illumina, 2013). Libraries of the V3 and V4 regions of the 16S rRNA gene were created via two-step amplification using the 16SAmpliconPCRForwardPrimer and the 16SAmpliconPCRReversePrimer. Sequencing was performed on the Illumina MiSeq using v3 chemistry to produce 300 base pair paired end reads.
Paired-end 16S rRNA gene sequences (109,175 amplicon sequence variants [ASVs], 73 samples [combined dataset including 32 samples used for this study]) were demultiplexed, trimmed to remove adapter sequences (Trim F 17, Trim R 21), and truncated at the point median Phred quality score dropped below 30 (Trunc F 276, Trunc R 223). The open-source bioinformatics pipeline QIIME2 and the DADA2 denoising algorithm (Bolyen et al., 2019) were then used to construct Amplicon Sequencing Variants (ASVs) and generate abundance tables. Overall summary statistics for reads per sample were: Minimum: 12,650; 1st Quantile: 22,028; Median: 28,477; Mean: 69,613; 3rd Quantile: 104,365; Maximum: 269,669.
ASVs were classified by aligning against the SILVA SSU Ref NR database (release v.138) (SILVA, 2020). QIIME2 was also used to generate a rooted phylogenetic tree and a BIOM file containing combined abundance and taxonomy data. The PICRUSt2 QIIME2 plugin was used to predict functional abundance and diversity in the form of KEGG enzymes (KEGG Orthologs [KOs]) and MetaCyc pathways. At this step, 1,712 of 109,011 ASVs were above the maximum Nearest Sequenced Taxon Index (NSTI) cut-off of 2.0 (a measure of closeness to the reference genome with values <2.0 sufficient to obtain a reference hit) and were removed, leading to a very high alignment (98.42%). This high alignment combined with PICRUSt2’s comprehensive database (approx. 20,000 genomes) and the strong correlation between the actual functions obtained through shotgun metagenomics and those predicted by PICRUSt2 on a test soil dataset (rs > 0.8) in the original manuscript describing the software (Douglas et al., 2020) allow high confidence in the predictions in this study. The full QIIME2 workflow is available online (Ijaz, 2019).
Prior to statistical analysis, the output of the QIIME2 pipeline was further processed to remove ASVs that were unassigned or aligned with typical contaminants such as mitochondria and chloroplasts [as is suggested as a typical filtering step for QIIME2 analysis (QIIME2, 2022)]. Additionally, as blanks were sequenced alongside samples and initial DNA concentrations were measured prior to sequencing, R’s decontam package (Davis et al., 2018) was able to be used to remove any contaminants that may have arisen from reagents or library preparation (Salter et al., 2014). For this purpose, after the initial filtering, frequency-based contaminant identification in the decontam package was used to remove 358 contaminant ASVs.
The final reads per sample summary statistics for the 32 samples (60,515 ASVs) used in this study were [Minimum: 9,761; 1st Quantile: 20,384; Median: 76,752; Mean: 88,535; 3rd Quantile: 142,943; Maximum: 253,526].
Statistical analyses were performed in R (version 4.1.2). All figures in this study were generated using R’s ggplot2 package (Wickham et al., 2016).
Taxa bar plots of the overall top 20 most abundant taxa at appropriate taxonomic levels were generated to give an indication of how the abundance of the most common species changes between rain gardens.
R’s vegan package was used for estimating alpha and beta diversity indices (Oksanen et al., 2022). Alpha diversity measurements used were Pielou’s evenness, Rarefied richness, Shannon entropy and Simpson’s index.
For taxonomic beta diversity analysis, Principal Coordinates Analysis (PCoA) plots of ASVs were produced using three different distance measures: Bray-Curtis, Unweighted UniFrac and Weighted UniFrac.
For analysis of functional beta diversity, Hierarchical Meta-Storms (Zhang Y. et al., 2021) was used to generate a PCoA plot. While the calculation of taxonomic beta diversity assumes independence between features (treating each ASV as a distinct entity), this assumption does not hold when applied to functional abundance tables of KEGG Orthologs (KOs) such as those obtained from PICRUSt2. This is because of redundancy in KOs, with most functional pathways able to utilise one of several alternative KOs in enzymatic reactions. Therefore, taking into account only KOs rather than full pathways may result in erroneous detection of functional diversity, as different KOs may be performing the same function in different samples. Hierarchical Meta-Storms takes this redundancy into account by considering not only the relative abundance of individual KOs but also the relative abundances of functions at each level of the KEGG BRITE pathway hierarchy.
The phyloseq package (McMurdie and Holmes, 2013) was used for calculating UniFrac distances. Vegan’s ordiellipse() function was used to draw ellipses representing the standard errors of the weighted sample averages for each category.
Vegan’s adonis() function was used to perform PERMANOVA. PERMANOVA is used to identify significant differences between the centroids/means of groups of samples (e.g., samples from rain garden A vs. B). PERMANOVA also calculates the proportion of variance between groups that can be explained by individual covariates (e.g., water chemistry parameters) and gives the result as an R2 value if significant. For example, an R2 of 0.25 indicates that 25% of variance between groups is a result of changes to that parameter. PERMANOVA in this study was carried out on one environmental covariate at a time, due to the high number of covariates being tested in this study.
During May, when the input TSS concentration was at its highest, the rain gardens were able to reduce suspended solids significantly - from the input TSS of 134 mg/L to output TSS of 2 mg/L and 16 mg/L in outputs A and C, respectively, (Table 1). In August and December, TSS concentrations were much lower and either similar between the input and outputs or reduced to below detection limits. Suspended solids are often carriers for other pollutants including nutrients, metals, and hydrocarbons (Ferrier et al., 2005; Kennedy et al., 2016; Müller et al., 2020), so their removal makes a significant impact on water quality. When the influent contained high concentrations of TSS, large reductions were seen in the effluent. Rain gardens are typically highly effective at removing TSS (Davis et al., 2009; Chapman and Horner, 2010) and the results here are consistent with this.
Table 1. Total suspended solids (TSS), total dissolved solids (TDS), pH, and electrical conductivity (EC) values of rain garden water samples.
TDS concentration was also measured (Table 1). The high TDS in December is likely due to winter road salting. Rain garden D was also subjected to additional salting at a concentration of 10 g/m2 every other working day between December 2019 and March 2020. This is the likely reason why TDS was highest in Output D, as four salting events had taken place prior to sampling. Overall, the impact of rain gardens on TDS was variable.
The impact of road salting was further supported by EC values which were highest in December and, moreover, highest in rain garden D in December which had received additional salting (Table 1). The observation that the EC values are also higher in rain gardens A to C compared to input is likely due to timing compared to salting, where sufficient time has passed since a salting event to wash the road of salt (hence the lower input values), but that higher salinity water is still resident in the rain garden. The pH of the samples remained broadly similar across sample source and time, with the rain gardens causing a slight increase in pH compared to input water (Table 1). Tests on the raw soil material showed that the pH values of soil mixes A, B, C and D were 8.25, 7.89, 8.14 and 7.98, respectively. Evidently, the soils with higher pH generally caused a greater increase in pH in the effluent suggesting that the pH of the chosen soil mix could directly impact the pH of any water released to the environment. Potentially, buffering of the pH by the soils to help maintain neutral or slightly alkali pH might help to ensure dissolved metal mobility is low by supporting metal precipitation and activation of anionic binding sites on surfaces.
Full COD results are shown in Table 2 alongside the percentage change in COD after filtering. The highest COD was seen in the May input (133 mg/L), which was reduced to 87 mg/L and 49 mg/L in Outputs A and C, respectively. COD measurements in August and December samples were much lower and quite consistent between the influent and effluent, ranging from 11 to 25 mg/L. Similarly to the TSS results, the rain gardens are having a bigger impact on pollution reduction when the input waters are more polluted. Filtering of the water samples led to reductions in COD concentration of up to 97%, suggesting that much of the COD reactive material in the samples was bound to suspended solids rather than dissolved. From this we would expect that filtration and sedimentation processes inside the rain garden soils would play a key role in reducing COD.
Table 2. Chemical oxygen demand (COD) measurements for each sample, and the percentage difference resulting from filtering through 0.45 μm filters.
The concentrations of ammonium and nitrite decreased or remained below detection limits between the input and outputs in most samples (Table 3). In aerobic conditions, ammonium and nitrite can be converted to nitrates by nitrifying bacteria in the soil before these nitrates are taken up and used by plants (Brown and Hunt, 2011; Winston et al., 2020), and this may be contributing to the diminished ammonium and nitrite concentrations in the outputs. Nitrate concentrations generally remained low but increased in the outputs in May. Given that the concentration of phosphate also increased between the May influent and effluent while remaining low in other samples, nutrient loss from the rain garden soil soon after installation could have contributed to this increase. While further investigation is needed, it would appear that newly installed rain gardens have potential to contribute nutrients into the environment during early phases of operation.
Concentrations of fluoride, bromide, chloride, and sulphate did not decrease between the input and outputs indicating that the rain gardens are unable to remove these contaminants from the runoff (Table 3).
Chloride concentrations were highest in December, particularly in Output D. As with TDS and EC, this is likely due to the impact of winter road salting as well as the additional salting of rain garden D.
As many SUDS are not designed for salt removal, changes in road salting strategies such as reduced quantities or plant-based additives (Borst and Brown, 2014) may be required to minimise the impact of chloride on surface water. Chloride contamination is a particularly important consideration in infiltration-based SUDS, like many rain gardens, as this has potential to result in contamination of groundwater (Stone and Marsalek, 2011).
Table 4 shows the dissolved concentrations of key potentially toxic elements (PTEs) that are commonly found in stormwater or are regulated in surface water.
In May and December, the dissolved concentrations of most PTEs decreased between the input and outputs or remained below detection limits. In August, most dissolved PTE concentrations either increased or remained below detection limits in all outputs. However, they remained lower than or comparable to the effluent concentrations seen in May and December. Maximum reductions of Cu, Zn, and Cd ranged from 80–90%, comparable to those seen in previous rain garden studies (Chapman and Horner, 2010; David et al., 2015). While maximum Pb removal was lower than Cu, Zn, and Cd, it remained comparable to other rain gardens (David et al., 2015).
Winter road salting has potential to cause the desorption of PTEs from soil (Woods Ballard et al., 2015), resulting in the release of contaminants into the environment. Mechanisms include the salt weakening the electrostatic attraction between the soil grain and the PTE, competition between salt cation and PTE for adsorption sites, and complexation of PTEs with salt anions (Acosta et al., 2011). However, in this case it appears that the addition of salt has not caused significant desorption, as high concentrations of PTEs were not observed in the December effluent. While this may indicate that salt concentrations are insufficient to cause desorption, it may also indicate that PTE removal is occurring via precipitation into a solid phase rather than adsorption, and PTEs are therefore not re-mobilised by salt.
There is no clear difference in PTE removal between rain gardens and thus it cannot be determined if any of these soil particle size distributions is most suitable for treatment of PTE-contaminated stormwater.
When all the contaminants above are considered, there is no clear difference in contaminant removal between different rain gardens, suggesting that grain size and hydraulic conductivity have not had a significant impact on treatment, at least not within the range of grain sizes and hydraulic conductivities used here. Broadly, all rain gardens appeared to work equally well at removing contaminants.
It should be noted here that undertaking more frequent analysis along with flow rate measurement enables calculation of total pollutant loads and mass-based retention. This was not done here, although the sampling points were collected during different seasons and rainfall volumes and therefore provide insights into those events. The ability to determine total pollutant loads is relevant for understanding impacts on the natural environment, such as a receiving water body. For rain gardens that have not been designed for research purposes, frequent monitoring could become a challenge if required by regulatory authorities. For example, the Scottish Environment Protection Agency’s Sustainable Urban Drainage Systems guidance [WAT-RM-08; (SEPA, 2019)], notes there may be situations when routine chemical sampling may not be suitable and downstream ecological monitoring may be more appropriate to determine impact. Therefore, research to understand and improve rain garden design and performance, including quantification of total pollutant loads, are key to ensuring future installed rain gardens work to protect the environment optimally. Furthermore, regular time interval water quality analysis would also be pertinent to understand the breakthrough or pulse of pollution during storm events, and how this changes over the duration of a storm under different rain garden designs. Note that in this study it had been raining for at least 4 days straight prior to sampling and moreover, was still raining during the sampling, in which case the input and output water quality was likely much closer to steady state.
Analysis of input and output water microbiology is useful to (1) understand how the rain gardens change stormwater community composition and (2) provide an indication of what is happening in the rain garden itself as the changes between input and output must be due to processes within the rain garden.
Figure 3 shows relative abundances of the top 20 phyla and genera in each of the water samples. There was a noticeable difference between the taxonomy of the influent and the effluent, indicating that microbial community composition was significantly altered by passing through the rain garden. However, effluent communities from the four rain gardens appear generally similar to one another, suggesting that the differences in soil particle size distribution between gardens are not having a notable impact on community composition in the output. The composition of all communities varied based on sampling date, which may be due to maturation of rain garden soil microbial communities as they become more established and seasonal differences such as temperature and pollutant concentration (e.g., road salting in winter).
Figure 3. Bar plots representing the top 20 most abundant taxa at phylum and genus level in water samples (input waters and output waters from rain gardens A, B, C and D), for May, August and December. Taxa outside the top 20 are labelled “Others.”
At phylum level, the influent was dominated by Proteobacteria at all sampling times. Patescibacteria was more abundant in May and this might be associated with the high COD input at that time as the Patescibacteria phylum has been found associated with hydrocarbon-contaminated soils and petroleum-associated communities (Bodor et al., 2021; González-Martín et al., 2022). The phylum Nitrospira, which is capable of nitrification (D’Angelo et al., 2023), was also present and therefore may be influencing concentrations of ammonium, nitrite, and nitrate in the rain garden system.
The abundances of Actinobacteriota, Acidobacteriota, and Bacteroidota in the effluent increased consistently over the sampling period. Acidobacteriota can tolerate metals and hydrocarbons (Liguori et al., 2021), so increasing concentrations of captured pollutants in the rain gardens may be leading to a greater abundance of Acidobacteriota in the soil and subsequently in the output of the gardens. Liguori et al. (2021) also identified Actinobacteriota and Bacteroidota as prevalent phyla in the influent and effluent of stormwater treatment devices. Rain garden soil analysed by Zhang et al. (2024) contained Proteobacteria, Bacteroidota, Acidobacteria, and Actinobacteriota as dominant phyla - phyla that were common in the input and output waters here, as well as the soil (Section 3.8). Hong et al. (2018) also reported Actinobacteriota in rain garden soils, where it was believed to be supporting nitrogen fixation in exchange for plant polysaccharides.
At genus level, input waters were again shown to be different to the output waters. Abundances of Sphingomonas, Noviherbaspiriullum, and Methylobacterium Methylorubrum were greater in the influent but continued to be present in the effluent. Sphingomonas is associated with hydrocarbon degradation (Bodor et al., 2021) and may be capable of nitrification (Baskaran et al., 2020), Noviherbaspirillum has been found in oil-contaminated soils (Lin et al., 2013), and Methylobacterium-Methylorubrum may be involved in stress-resistance in extreme conditions (Moura et al., 2021).
Examination of taxonomic diversity showed no statistically significant difference in rarefied richness between any of the water samples (Figure 4A). However, the Shannon entropy of the input water was significantly lower than that of each of the four outputs, and Pielou’s evenness and Simpson’s index were also significantly lower in the input compared to most of the output waters. This suggests that although the overall number of species in the water remained consistent between the input and outputs, the community becomes more even after passing through the rain gardens – i.e. the species in the effluent are present in more similar abundances rather than a few species dominating. PCoA plots based on Bray-Curtis, Unweighted UniFrac, and Weighted UniFrac showed output samples clustering by sampling date rather than by garden (Figure 5). This is significant as it illustrates that the differences in soil particle size and hydraulic conductivity are having a smaller impact than changes occurring in the rain garden over time, such as maturation of the microbial community in the rain garden or seasonal changes.
Figure 4. Alpha diversity estimates for (A) taxonomy at OTU level and (B) function based on the PICRUSt2 prediction of the KEGG metagenome. Overall significance taking into account all five water sources is shown in the strip titles (input waters and output waters for rain gardens A, B, C and D), and significant pairwise differences are represented by annotated lines connecting two categories. The following annotations are used to denote significance: ‘***’ (p ≤ 0.001), ‘**’ (p ≤ 0.01), ‘*’ (p ≤ 0.05), and ‘.’ (p ≤ 0.1).
Figure 5. Principal co-ordinate analysis (PCoA) of water samples using Bray-Curtis, Unweighted UniFrac, and Weighted UniFrac distances to represent taxonomic beta diversity and Hierarchical Meta-Storms to represent functional beta diversity. Ellipses for were drawn using 95% confidence intervals based on standard error of the ordination points for each category.
PERMANOVA identified date (May vs. Aug vs. Dec) followed by water source (In vs. A vs. B vs. C vs. D) as the factors having the greatest impact on beta diversity using BrayCurtis and Weighted UniFrac, and vice versa for Unweighted UniFrac. This again illustrates again that rain garden maturation and/or seasonal differences are having a significant impact on community composition.
Previous studies have reported that differences in pollutant type and load can influence microbial community composition in the soil (Hong et al., 2018; Zhang et al., 2024). Zhang et al. (2024) reported accumulation of metals in the soil was a key factor driving changes in microbial community composition over the lifetime of a rain garden system, ultimately driving a decrease in abundance of some dominant microbes (Zhang et al., 2024). Potentially then, the accumulation of pollutants in the rain gardens here is driving the microbial community’s changes over time and is having a greater influence over microbial community composition than hydraulic conductivity or grain size variation.
Examination of functional diversity revealed that functional richness was significantly higher in the effluent than in the influent (Figure 4B). This suggests that organisms within the rain garden soil are capable of performing a wider range of functions than those in the original input stormwater, and that this is then impacting the output communities. This highlights the importance of undertaking diversity analysis based on function, in addition to taxonomy. For example, Figure 4 illustrates that there is no significant difference in taxonomic richness between input and any output waters, while there is a significant difference in functional richness. Recent studies are gaining valuable insights into rain garden microbial composition (e.g., Hong et al., 2018; Zhang et al., 2024) based on taxonomic assessment. However, as illustrated here, taxonomic diversity may not always reflect functional diversity, and therefore addition of statistical functional diversity analysis will be valuable going forward in gaining a greater insight into rain garden microbial performance.
PCoA using Hierarchical Meta-Storms to determine variation in functional diversity between samples yielded distinct clusters for the August and December output samples (Figure 5). May output samples neither clustered closely with one another nor with other outputs. Broadly speaking, the clustering appeared to become tighter over time, which suggests that the rain gardens became more functionally similar to each other over the sampling period. This again supports the idea of microbial communities in each rain garden developing over time into a similar compositional and functional make-up.
PERMANOVA identified sampling date as having the greatest impact on functional beta diversity, again highlighting how the community in each rain garden is developing over time.
Based on output water microbial analysis we would therefore argue that the grain size and hydraulic conductivity are having a minimal impact on microbial community composition and function within this rain garden system.
Microbial analysis of the output waters provides bulk insights into microbiology of the rain garden soils. The rain garden soil is likely to contain heterogeneities which may include an aerobic surface layer, plant root zone, and anaerobic layer at depth, which all may have different microbial signatures. Output water will have passed through these zones and thus be influenced by each one. The output water is an indicator of the water composition in the rain garden soil’s pores. The microbial composition of this pore fluid will be influenced by microorganisms attached to soil particles which will detach into the pore fluid to different extents. Therefore, while the output water is a useful indicator of microbiology in the rain garden, it is not an exact match and we therefore also analysed soil microbiology.
To briefly explore microbial community structure of the soil itself, at the end of the monitoring period soil samples were collected from the top 10 cm of each rain garden. Surface soils all displayed visually similar taxonomy (Figure 6). Figure 7 shows alpha diversity measures for the soil samples. There was no significant difference between taxonomic rarified richness, again broadly supporting the idea that the grain size and hydraulic conductivity differences were not having a notable impact on microbial ecology within the rain garden. However, there were statistically significant differences in Pielou’s evenness and Simpson’s index between some of the soils, and thus grain size was not without some impact on microbiology, although these differences were smaller compared to the differences observed between input and output waters. Functional richness of the surface soils was higher than the input waters, supporting the idea that the microbes in the rain gardens are able to perform a wider range of functions than those in the input water. Interestingly, functional richness was lowest in soil D, potentially indicating the addition of salt had reduced the number of functions in the community. Across the range of alpha diversity parameters tested, soil D generally displayed greater difference to the other soils functionally, with less difference taxonomically. However, this did not impact the ability to remove pollutants, with soil D performing similarly to the unsalted soils. PERMANOVA identified that hydraulic conductivity had no significant impact on functional beta diversity.
Figure 6. Bar plots representing the top 20 most abundant taxa at phylum and genus level in surface soils. Taxa outside the top 20 are labelled “Others.”
Figure 7. Soil alpha diversity estimates for (A) taxonomy at OTU level and (B) function based on the PICRUSt2 prediction of the KEGG metagenome. Overall significance taking into account all four soil types is shown in the strip titles, and significant pairwise differences are represented by annotated lines connecting two categories. The following annotations are used to denote significance: ‘***’ (p ≤ 0.001), ‘**’ (p ≤ 0.01), ‘*’ (p ≤ 0.05), and ‘.’ (p ≤ 0.1).
Hong et al. (2018) reported that soil pH was a key driving factor in controlling microbial community composition in rain garden soils. Likewise, adjusting soil composition has the potential to drive changes in soil microbiology and thus influence microbial performance (Ulrich et al., 2017). Comparison of soil and water pH in this study illustrated that the soil was able to provide a buffering impact to control the pH of the system. As a result, adjusting soil composition to control factors like pH may enable microbial community compositions to be tuned towards more favorable operation. In contrast, findings in this study indicate that variations in hydraulic conductivity/grain size had a relatively minimal influence on microbial composition and pollutant removal (at least not across the range studied here). Potentially then, adjusting soil composition (i.e., soil chemistry), rather than adjusting grain size, may be more successful in tuning microbial ecology to better performance. However, this must be set against the potential impact of metal accumulation in the rain garden over time (Zhang et al., 2024), which eventually may become the dominant factor controlling microbial ecology.
Each rain garden was able to ensure output stormwater contained low levels of contaminants. Winter road salting increased TDS, EC, and chloride in the stormwater, but did not lead to associated leaching of metals from the rain garden soil into the output waters. Some variation in contaminant removal was seen between rain gardens, but no clear trend could be identified based on grain size and hydraulic conductivity and all rain gardens performed broadly similarly. Bacteria resistant to metals and capable of hydrocarbon degradation were found in both the influent and effluent waters and in the rain garden soils. Passing through the rain gardens increased the taxonomic evenness and overall diversity of the stormwater microbial community. Functional richness of the output waters and soils was higher than the input waters, indicating that the microbes in the rain gardens are able to perform a wider range of functions than those in the input water. Microbial community composition and function differed between the influent and effluent but showed little difference between output waters from different rain gardens. Moreover, PERMANOVA analysis identified that hydraulic conductivity had no significant impact on functional beta diversity in the soil. Therefore, soil particle size and hydraulic conductivity distribution in this study appeared to have relatively small impacts on community composition. Instead, the communities showed variation by date, suggesting that age of the rain garden (and associated community maturation), or varying pollutant levels may have more impact on effluent microbiology than soil grain size within the rain garden.
The original contributions presented in the study are included in the article/Supplementary material, further inquiries can be directed to the corresponding author. The sequencing datasets generated in this study can be found in the European Nucleotide Archive (Accession number PRJEB75431).
EC: Conceptualization, Methodology, Writing – original draft, Writing – review & editing, Data curation, Formal analysis, Investigation. UI: Formal analysis, Methodology, Writing – review & editing. IJ: Methodology, Writing – review & editing, Conceptualization. VP: Conceptualization, Methodology, Writing – review & editing, Supervision, Writing – original draft.
The author(s) declare that financial support was received for the research, authorship, and/or publication of this article. This work was supported by an EPSRC PhD studentship to EC and a Royal Society Short Industry Fellowship to VP. Funding for the construction of the rain gardens was provided by Glasgow City Council through the Glasgow City Region City Deal.
Next, generation sequencing was carried out at Glasgow Polyomics. We thank Karina Garces-Mirza and the Glasgow City Council (Avenues Programme) for supporting access to the rain gardens and scientific discussion. We thank Urban Movement for supporting the design and construction of the rain gardens. Thanks to Carla Lopez for ammonia analysis.
IJ was employed by Civic Engineers Ltd., which was involved in the design and installation of the rain garden system.
The remaining authors declare that the research was conducted in the absence of any commercial or financial relationships that could be construed as a potential conflict of interest.
The author(s) declared that they were an editorial board member of Frontiers, at the time of submission. This had no impact on the peer review process and the final decision.
All claims expressed in this article are solely those of the authors and do not necessarily represent those of their affiliated organizations, or those of the publisher, the editors and the reviewers. Any product that may be evaluated in this article, or claim that may be made by its manufacturer, is not guaranteed or endorsed by the publisher.
The Supplementary material for this article can be found online at: https://www.frontiersin.org/articles/10.3389/frwa.2024.1383382/full#supplementary-material
Acosta, J. A., Jansen, B., Kalbitz, K., Faz, A., and Martínez-Martínez, S. (2011). Salinity increases mobility of heavy metals in soils. Chemosphere 85, 1318–1324. doi: 10.1016/j.chemosphere.2011.07.046
Ayilara, M. S., and Babalola, O. O. (2023). Bioremediation of environmental wastes: the role of microorganisms. Front. Agron. 5:1183691. doi: 10.3389/fagro.2023.1183691
Baskaran, V., Patil, P. K., Antony, M. L., Avunje, S., Nagaraju, V. T., Ghate, S. D., et al. (2020). Microbial community profiling of ammonia and nitrite oxidizing bacterial enrichments from brackishwater ecosystems for mitigating nitrogen species. Sci. Rep. 10:5201. doi: 10.1038/s41598-020-62183-9
Bodor, A., Bounedjoum, N., Feigl, G., Duzs, Á., Laczi, K., Szilágyi, Á., et al. (2021). Exploitation of extracellular organic matter from Micrococcus luteus to enhance ex situ bioremediation of soils polluted with used lubricants. J. Hazard. Mater. 417:125996. doi: 10.1016/j.jhazmat.2021.125996
Bolyen, E., Rideout, J. R., Dillon, M. R., Bokulich, N. A., Abnet, C. C., Al-Ghalith, G. A., et al. (2019). Reproducible, interactive, scalable and extensible microbiome data science using QIIME 2. Nat. Biotechnol. 37, 852–857. doi: 10.1038/s41587-019-0209-9
Borst, M., and Brown, R. A. (2014). Chloride released from three permeable pavement surfaces after winter salt application. J. Am. Water Resour. Assoc. 50, 29–41. doi: 10.1111/jawr.12132
Brown, R. A., and Hunt, W. F. (2011). Underdrain configuration to enhance bioretention exfiltration to reduce pollutant loads. J. Environ. Eng. 137, 1082–1091. doi: 10.1061/(ASCE)EE.1943-7870.0000437
Carpenter, D. D., and Hallam, L. (2010). Influence of planting soil mix characteristics on bioretention cell design and performance. J. Hydrol. Eng. 15, 404–416. doi: 10.1061/(ASCE)HE.1943-5584.0000131
Chapman, C., and Horner, R. R. (2010). Performance assessment of a street-drainage bioretention system. Water Environ. Res. 82, 109–119. doi: 10.2175/106143009X426112
Charlesworth, S. M. (2010). A review of the adaptation and mitigation of global climate change using sustainable drainage in cities. J. Water Clim. Change 1, 165–180. doi: 10.2166/wcc.2010.035
D’Angelo, T., Goordial, J., Lindsay, M. R., McGonigle, J., Booker, A., Moser, D., et al. (2023). Replicated life-history patterns and subsurface origins of the bacterial sister phyla Nitrospirota and Nitrospinota. ISME J. 17, 891–902. doi: 10.1038/s41396-023-01397-x
D’Arcy, B. J., Usman, F., Griffiths, D., and Chatfield, P. (1998). Initiatives to tackle diffuse pollution in the UK. Water Sci. Technol. 38, 131–138. doi: 10.2166/wst.1998.0393
David, N., Leatherbarrow, J. E., Yee, D., and McKee, L. J. (2015). Removal efficiencies of a bioretention system for trace metals, PCBs, PAHs, and dioxins in a semiarid environment. J. Environ. Eng. 141:04014092. doi: 10.1061/(ASCE)EE.1943-7870.0000921
Davis, A. P., Hunt, W. F., Traver, R. G., and Clar, M. (2009). Bioretention technology: overview of current practice and future needs. J. Environ. Eng. 135, 109–117. doi: 10.1061/(ASCE)0733-9372(2009)135:3(109)
Davis, N. M., Proctor, D. M., Holmes, S. P., Relman, D. A., and Callahan, B. J. (2018). Simple statistical identification and removal of contaminant sequences in marker-gene and metagenomics data. Microbiome 6:226. doi: 10.1186/s40168-018-0605-2
Davis, A. P., Shokouhian, M., and Ni, S. (2001). Loading estimates of lead, copper, cadmium, and zinc in urban runoff from specific sources. Chemosphere 44, 997–1009. doi: 10.1016/S0045-6535(00)00561-0
Douglas, G. M., Maffei, V. J., Zaneveld, J. R., Yurgel, S. N., Brown, J. R., Taylor, C. M., et al. (2020). PICRUSt2 for prediction of metagenome functions. Nat. Biotechnol. 38, 685–688. doi: 10.1038/s41587-020-0548-6
Ferrier, R. C., D’arcy, B. J., MacDonald, J., and Aitken, M. (2005). Diffuse pollution - what is the nature of the problem? Water Environ. J. 19, 361–366. doi: 10.1111/j.1747-6593.2005.tb00574.x
Fewkes, A. (2012). A review of rainwater harvesting in the UK. Struct. Surv. 30, 174–194. doi: 10.1108/02630801211228761
Fletcher, T. D., Shuster, W., Hunt, W. F., Ashley, R., Butler, D., Arthur, S., et al. (2015). SUDS, LID, BMPs, WSUD and more – the evolution and application of terminology surrounding urban drainage. Urban Water J. 12, 525–542. doi: 10.1080/1573062X.2014.916314
González-Martín, J., Cantera, S., Lebrero, R., and Muñoz, R. (2022). Optimization of acrylic-styrene latex-based biofilms as a platform for biological indoor air treatment. Chemosphere 287:132182. doi: 10.1016/j.chemosphere.2021.132182
Gülbaz, S., Kazezyilmaz-Alhan, C. M., and Copty, N. K. (2015). Evaluation of heavy metal removal capacity of bioretention systems. Water Air Soil Pollut. 226:376. doi: 10.1007/s11270-015-2640-y
Hong, J., Geronimo, F. K., Choi, H., and Kim, L.-H. (2018). Impacts of nonpoint source pollutants on microbial community in rain gardens. Chemosphere 209, 20–27. doi: 10.1016/j.chemosphere.2018.06.062
Hunt, W. F., Davis, A. P., and Traver, R. G. (2012). Meeting hydrologic and water quality goals through targeted bioretention design. J. Environ. Eng. 138, 698–707. doi: 10.1061/(ASCE)EE.1943-7870.0000504
Ijaz, U. Z. (2019). QIIME2 Tutorial. Available at: https://github.com/umerijaz/tutorials/blob/master/qiime2_tutorial.md
Jay, J. G., Tyler-Plog, M., Brown, S. L., and Grothkopp, F. (2019). Nutrient, metal, and organics removal from Stormwater using a range of bioretention soil mixtures. J. Environ. Qual. 48, 493–501. doi: 10.2134/jeq2018.07.0283
Jefferies, C., Napier, F., Fogg, P., and Nicholson, F. (2008). Source control pollution in sustainable drainage. Edinburgh: SNIFFER.
Kennedy, P., Allen, G., and Wilson, N. (2016). The management of hydrocarbons in stormwater runoff: a literature review. Auckland: Auckland Council.
Li, D., and Liu, J. (2019). “Water quality detection for lakes” in Water quality monitoring and management. Eds. Li, D., and Liu, S. (Cambridge: Academic Press), 221–231.
Liguori, R., Rommel, S. H., Bengtsson-Palme, J., Helmreich, B., and Wurzbacher, C. (2021). Microbial retention and resistances in stormwater quality improvement devices treating road runoff. FEMS Microbes 2:xtab008. doi: 10.1093/femsmc/xtab008
Lin, S.-Y., Hameed, A., Arun, A. B., Liu, Y.-C., Hsu, Y.-H., Lai, W.-A., et al. (2013). Description of Noviherbaspirillum malthae gen. Nov., sp. nov., isolated from an oil-contaminated soil, and proposal to reclassify Herbaspirillum soli, Herbaspirillum aurantiacum, Herbaspirillum canariense and Herbaspirillum psychrotolerans as Noviherbaspirillum soli comb. nov., Noviherbaspirillum aurantiacum comb. nov., Noviherbaspirillum canariense comb. nov. and Noviherbaspirillum psychrotolerans comb. nov. based on polyphasic analysis. Int. J. Syst. Evol. Microbiol. 63, 4100–4107. doi: 10.1099/ijs.0.048231-0
McMurdie, P. J., and Holmes, S. (2013). Phyloseq: an R package for reproducible interactive analysis and graphics of microbiome census data. PLoS One 8:e61217. doi: 10.1371/journal.pone.0061217
Moura, J. B., Delforno, T. P., Do Prado, P. F., and Duarte, I. C. (2021). Extremophilic taxa predominate in a microbial community of photovoltaic panels in a tropical region. FEMS Microbiol. Lett. 368:fnab105. doi: 10.1093/femsle/fnab105
Müller, A., Österlund, H., Marsalek, J., and Viklander, M. (2020). The pollution conveyed by urban runoff: a review of sources. Sci. Total Environ. 709:136125. doi: 10.1016/j.scitotenv.2019.136125
Nyström, F., Nordqvist, K., Herrmann, I., Hedström, A., and Viklander, M. (2020). Removal of metals and hydrocarbons from stormwater using coagulation and flocculation. Water Res. 182:115919. doi: 10.1016/j.watres.2020.115919
Oksanen, J., Blanchet, F. G., Kindt, R., Legendre, P., Minchin, P., O’Hara, B., et al. (2022). Vegan: Community ecology package. R package version 2.6-2. Available at: https://CRAN.R-project.org/package=vegan
QIIME2 (2022). Filtering data. Available at: https://docs.qiime2.org/2022.8/tutorials/filtering/
Quenea, K., Lamy, I., Winterton, P., Bermond, A., and Dumat, C. (2009). Interactions between metals and soil organic matter in various particle size fractions of soil contaminated with waste water. Geoderma 149, 217–223. doi: 10.1016/j.geoderma.2008.11.037
Salter, S. J., Cox, M. J., Turek, E. M., Calus, S. T., Cookson, W. O., Moffatt, M. F., et al. (2014). Reagent and laboratory contamination can critically impact sequence-based microbiome analyses. BMC Biol. 12:87. doi: 10.1186/s12915-014-0087-z
SEPA (2019). Regulatory method (WAT-RM-08) sustainable urban drainage systems (SUDS or SUD systems). Scotland: Scottish Environment Protection Agency.
Shafique, M. (2016). A review of the bioretention system for sustainable storm water management in urban areas. Mater. Geoenviron. 63, 227–236. doi: 10.1515/rmzmag-2016-0020
SILVA (2020). SILVA rRNA Database. Available at: https://www.arb-silva.de/
Steis Thorsby, J., Miller, C. J., and Treemore-Spears, L. (2020). The role of green stormwater infrastructure in flood mitigation (Detroit, MI USA) – case study. Urban Water J. 17, 838–846. doi: 10.1080/1573062X.2020.1823429
Stone, M., and Marsalek, J. (2011). Adoption of best practices for the environmental management of road salt in Ontario. Water Qual. Res. J. Canada 46, 174–182. doi: 10.2166/wqrjc.2011.105
Ulrich, B. A., Vignola, M., Edgehouse, K., Werner, D., and Higgins, C. P. (2017). Organic carbon amendments for enhanced biological attenuation of trace organic contaminants in biochar-amended Stormwater biofilters. Environ. Sci. Technol. 51, 9184–9193. doi: 10.1021/acs.est.7b01164
Wickham, H., Navarro, D., and Pedersen, T. L. (2016). ggplot2: Elegant graphics for data analysis. 2nd Edn. New York, USA: Springer.
Winston, R. J., Arend, K., Dorsey, J. D., and Hunt, W. F. (2020). Water quality performance of a permeable pavement and stormwater harvesting treatment train stormwater control measure. Blue-Green Syst. 2, 91–111. doi: 10.2166/bgs.2020.914
Woods Ballard, B., Wilson, S., Udale-Clarke, H., Illman, S., Scott, T., Ashley, R., et al. (2015). The SuDs manual. London: CIRIA.
Zhang, T., Cheng, J., Tan, H., Luo, S., and Liu, Y. (2022). Particle-size-based elution of petroleum hydrocarbon contaminated soil by surfactant mixture. J. Environ. Manag. 302:113983. doi: 10.1016/j.jenvman.2021.113983
Zhang, Y., Jing, G., Chen, Y., Li, J., and Su, X. (2021). Hierarchical Meta-storms enables comprehensive and rapid comparison of microbiome functional profiles on a large scale using hierarchical dissimilarity metrics and parallel computing. Bioinform. Adv. 1:vbab003. doi: 10.1093/bioadv/vbab003
Zhang, L., Lu, Q., Ding, Y., and Wu, J. (2021). A procedure to design road bioretention soil media based on runoff reduction and pollutant removal performance. J. Clean. Prod. 287:125524. doi: 10.1016/j.jclepro.2020.125524
Keywords: stormwater, water quality, rain garden, microbial ecology, water treatment
Citation: Corbett E, Ijaz UZ, Jackson I and Phoenix VR (2024) Stormwater quality and microbial ecology in an urban rain garden system. Front. Water. 6:1383382. doi: 10.3389/frwa.2024.1383382
Received: 07 February 2024; Accepted: 10 June 2024;
Published: 01 July 2024.
Edited by:
Abbas Roozbahani, Norwegian University of Life Sciences, NorwayReviewed by:
Caitlyn A. Hall, The University of Arizona, United StatesCopyright © 2024 Corbett, Ijaz, Jackson and Phoenix. This is an open-access article distributed under the terms of the Creative Commons Attribution License (CC BY). The use, distribution or reproduction in other forums is permitted, provided the original author(s) and the copyright owner(s) are credited and that the original publication in this journal is cited, in accordance with accepted academic practice. No use, distribution or reproduction is permitted which does not comply with these terms.
*Correspondence: Vernon R. Phoenix, dmVybm9uLnBob2VuaXhAc3RyYXRoLmFjLnVr
Disclaimer: All claims expressed in this article are solely those of the authors and do not necessarily represent those of their affiliated organizations, or those of the publisher, the editors and the reviewers. Any product that may be evaluated in this article or claim that may be made by its manufacturer is not guaranteed or endorsed by the publisher.
Research integrity at Frontiers
Learn more about the work of our research integrity team to safeguard the quality of each article we publish.