- Department of Bioresource Engineering, McGill University, Sainte-Anne-de-Bellevue, QC, Canada
Sugarcane (Saccharum officinarum) is a traditional major crop and export of Guyana. This study aims to assess the current irrigation scenario and propose scenarios to maximize the yield and water use efficiency of sugarcane (S. officinarum) in Guyana, using the AquaCrop model. Field-measured climate and soil data, and local crop parameters were used in the simulations. The crop simulations were calibrated with actual yields from 2005 to 2008. The calibrated parameters were then validated using the 2009 to 2012 yield dataset. The good agreement (RMSE of 7.15%) with the recorded yield during validation and the low sensitivity of calibrated parameters indicate the acceptability of AquaCrop and the parameters used for simulations. During calibration, the yield was weakly sensitive (0.6–2% ΔRMSEn) to changes in crop parameter values with the highest sensitivity observed for the maximum canopy cover (CCx) and the crop coefficient (kcmax). Several irrigation scenarios were then simulated, of which no significant reduction or increase in yield was observed between the scenarios 50% to 100% of the total available water (TAW). A threshold of 50%TAW is advised during dry periods to avoid significant yield loss. It is recommended that this scenario be validated with field experiments. The results of this study will assist in maintaining high sugarcane yields even during dry conditions.
1 Introduction
Sugarcane (S. officinarum) is a traditional major export crop of Guyana. In Guyana, the first crop, called plant cane, is usually planted between November and January and is harvested after 40 weeks (Eastwood, 2009). A ratoon crop, which is grown from shoots left by the harvested crop, takes 36 weeks to be ready for harvest (Eastwood, 2009). Sugarcane is planted on beds in either a ridge-and-furrow or broad-bed design. Most of the fields have traditionally followed the ridge-and-furrow layout, but recently, more plots have been converted to broad beds for mechanized planting and harvesting (GuySuCo, 2018). Water is pumped from conservancies and rivers into the canals leading to the sugarcane estates. It is then routed into the furrows within the fields. The irrigation layout is a continuous open-ended furrow system wherein water is allowed to freely enter and exit the furrows. When water reaches the end of the furrows, it is collected by an in-field collector drain which routes and merges with the main canal network. With the current irrigation scheme, the soil-water content in the field is estimated to be maintained at 70% of total available water (TAW). Most of the agriculture sits along the coasts of Guyana, especially on the rich Hydraquents or “frontland” soils such as Tain clay, Whittaker clay, Corentyne clay, and Skeldon clay (FAO, 1966). Going further inwards are Medihemists or bog, peat and muck soils (GLSC, 2013) which are swamps and marshlands used as reservoirs or conservancies (Steele and Ramdin, 1980; GLSC, 2013). Except for the tidal flats, the riverain and frontland soils have a silty-clayey, or clayey to heavy clay texture. Heavy clays, which are the most common among Guyana's frontland soils (GLSC, 2004), are soils with a clay percentage above 60% (FAO, 2006). They are characterized by relatively high fertility, poor drainage, and waterlogging after heavy rain (GLSC, 2013). Drainage is a major challenge, and as such, various adaptation techniques are employed: an extensive surface drainage network, sugarcane varieties adapted to waterlogged conditions, façade drainage canals, drainage pumps, and dikes. Heavy clay is also characterized by pronounced soil swelling and the formation of deep cracks or gullies during wetting and drying cycles (Kodikara et al., 2002). These soils have a high field capacity (FC), but also a high permanent wilting point (PWP) compared to other soil textures, which results in low available water ranging between 110 and 160 mm per meter of soil depth (Syers et al., 2001; Ibrahim et al., 2002; Dinssa and Elias, 2021). This narrow range makes crops not only susceptible to surface ponding, but also to drought in the dry season.
Sugarcane can be grown in a variety of soil textures including heavy clays such as around the Burdekin River (Holmes, 2014) and Mackay Region (Biggs et al., 2013) in Australia, Grand-Terre in Guadeloupe (Chopin et al., 2015; Sierra et al., 2017), and Nansei Islands in Japan (Arakawa et al., 2021). When planted on heavy clays, sugarcane has a slower root elongation rate, at 28 mm day−1, and shallower rooting depth compared to those planted in other soil textures (Glover, 1967 as cited in van Antwerpen et al., 2022). Breeding for tolerance to flooding (Dlamini, 2021; Kennedy, 2022) and subsurface drainage design (Ritzema et al., 2008) have been done to adapt and improve sugarcane growth on heavy clay soils. Regardless of soil texture, double-row furrow planting (Singh et al., 2022), subsurface drip irrigation (Sheini-Dashtgol et al., 2020), and mulching using dead crop residue (Adetoro et al., 2020) have proven to increase the water productivity of sugarcane. Water-saving scenarios are also one of the common irrigation management strategies to improve water use. Dingre and Gorantiwar (2021) studied the effect of water deficit on sugarcane planted on clay soil in a semi-arid climate under drip irrigation while Santos et al. (2019) studied surface drip irrigation over sandy loam soil in a humid subtropical climate. There are no studies yet on the impact of irrigation thresholds for sugarcane planted under furrow irrigation, specifically on heavy clay soils in a tropical climate. The specific characteristics of heavy clays, and the different wetting pattern of furrow irrigation makes it difficult to apply the results of existing studies to sugarcane estates in the coastlands of Guyana.
Crop models can simulate the impact of agricultural management decisions on sugarcane yield. Notable models for sugarcane are DSSAT-CANEGRO, AquaCrop, and APSIM-Sugar. DSSAT (Jones et al., 2003) and APSIM (Dias et al., 2019) are process-based models wherein sets of equations describe each process of plant growth, and these processes are linked by internal variables to form a general model to describe the overall growth. AquaCrop, meanwhile, is a crop-water-based model wherein water uptake drives plant growth (Steduto et al., 2009). The conversion of water to yield is governed by three equations calculating the water required for evapotranspiration, the water transpired into biomass, and the partitioning of the above-ground biomass into yield and non-marketable biomass (Steduto et al., 2012). Since AquaCrop is not process-based, it does not model each plant process including those involving crop stress; instead, stress coefficients are factored into the three core equations as a modifier (Raes et al., 2018). These stress coefficients are determined by the indicator parameters and the proximity of their value to stress thresholds. This characteristic allows AquaCrop to simulate the yield and irrigation requirement of sugarcane with fewer, easily measured crop parameters. Its use of conservative and default parameters; and templates for various crops, makes it convenient when information on the numerous model parameters is limited. AquaCrop fits the needs of our study since our crop data are agronomic information and not genotypic data. Moreover, the simulation results are meant to represent the sugarcane estates at the coast and not field-specific yields. For this larger-scale simulation where generalized representative values are needed, AquaCrop is the most suitable.
In a comparison study of crop models by Feleke et al. (2021), AquaCrop has shown satisfactory prediction of canopy cover and yield of maize compared to DSSAT-CERES, and APSIM-Maize for four maize varieties, and three soil textures (sandy clay loam, clay and loam) in Ethiopia, which has a tropical monsoon climate. There is no similar crop model comparison study for sugarcane. Nevertheless, the Feleke et al. (2021) study has strengthened AquaCrop's applicability to simulate crop growth in tropical areas. Furthermore, AquaCrop has been intensively used for research and was used to simulate sugarcane growth to understand the crop's response to projected climate change in Pakistan (Farooq and Gheewala, 2020; Alvar-Beltrán et al., 2021); identify a suitable deficit irrigation design in Khuzestan, Iran (Bahmani and Eghbalian, 2018); and predict the impact of a shifted crop calendar considering climate projections in Phu Yen, Vietnam (Lee and Dang, 2018). Heavy clays have distinct cracking and swelling characteristics that may be difficult to incorporate in model simulations. In a study on several landscapes including Cambisols, Vertisols, Luvisols, and Regosols, run-off was poorly simulated in AquaCrop when cracks were present; however, overall, the soil water content and crop conditions were simulated well in AquaCrop due to the moderate influence of runoff in the soil water balance (Dhouib et al., 2022).
The wet tropical climate (Peel et al., 2007) and clayey frontland soils (Braun and Derting, 1964; GLSC, 2013) of Guyana's coast place more emphasis on drainage for agriculture. The entire irrigation and drainage system of Guyana, the conservancies, and the sea walls were designed to prevent flooding of the low-lying coastal land, which is below sea level (US Army Corps of Engineers, 1998). Yet, the sugarcane estates are prone to agricultural drought because of the intrinsic high PWP of heavy clays, and the shallower rooting depth of sugarcane in these soils. Improvements in irrigation will help achieve better yield, improve water conservation and help the agricultural sector to be prepared for drought conditions. All of the sugarcane estates, about 44,500 ha (GLSC, 2013), are managed by the government under GuySuCo. More efficient water management by the managing organization will cascade to big changes in the coastal agricultural landscape. For the wider scientific community, the results of this study will contribute to the growing body of knowledge on the recommended irrigation thresholds of crops, especially for sugarcane on heavy clay soils. This study aims to: (1) improve the irrigation of sugarcane estates in Guyana by identifying the impact of the current water management system on sugarcane yield and water use efficiency, and (2) Determine alternative irrigation water application scenarios to cope with dry conditions.
2 Methodology
2.1 Data and preparation of simulation files
The study location covers the sugar estates of Albion, Rose Hall, and Port Mourant in Region 6 along the Guyana coast. Sugar production in Albion accounts for 55% of Guyana's total production with 45,000 to 60,000 ha harvested annually from 2009 to 2018 (GuySuCo, 2018). Irrigation is mainly supplied by the Canje Creek which is located southwest of the sugarcane estates. Climate data were obtained from the nearest installed automatic weather station located at 6°4′58” N, 57°15′57” W. Daily data on the rainfall, sunshine hours, wind velocity, and minimum and maximum temperature were measured from 2005 to 2012. The average monthly rainfall at the study site, in Figure 1, shows variability between the months. A long, wet season can be observed from May to August and a short, wet season from December to January. The monthly rainfall varies between 50 and 200 mm. Minimum and maximum temperatures were almost constant throughout the year. The daily reference evapotranspiration (ETo) was computed using the FAO Penman-Monteith within AquaCrop.
The soil at the three estates is Frontland clay, more specifically, the drained phase of Corentyne clay. This soil series is a swampland soil which is prone to waterlogging during heavy rains but has high fertility (GLSC, 2004). It is non-acidic, and soft to moderately firm dark gray clay, with a firmer gray clay subsoil with yellowish mottles (FAO, 1966). Soil sampling at four random sites confirmed heavy clay soils with a sand-silt-clay ratio of 2%−34%−64%. The soil characteristics and soil water retention curve from the four sampling sites were measured. It has a bulk density of 1.11 g/cm3. The percentage of soil moisture by volume at PWP across 3 depths (0–15, 15–30, and 30–45 cm) ranged from 32 to 35%, and the moisture content at field capacity varied from 49 to 51%.
The crop parameters used in the model were taken from the sugarcane file provided in AquaCrop. Default parameters for sugarcane can be found in the AquaCrop reference manual (Raes et al., 2018), with updated crop parameters published by Pereira et al. (2021). Field experiments in Brazil (da Silva et al., 2013; da Costa Faria Martins et al., 2022), Australia, Swaziland (Inman-Bamber and McGlinchey, 2003) and South Africa (Olivier and Singels, 2012) have contributed to improvements to key parameters of the sugarcane crop file, specifically the crop coefficients, maximum root depth, and the threshold of soil water depletion for water stress. When local values were available, such as those listed in Table 1, these values were prioritized and used in the model. Local field information (“F” in Table 1) includes locally measured parameters reported in the literature and field observations obtained by experts in Guyana. Parameters such as crop coefficient, growth duration, and threshold of water stress for stomatal closure were updated with information from Inman-Bamber and McGlinchey (2003), Eastwood (2009), Bastidas-Obando et al. (2017), Gaj and Madramootoo (2017), and Pereira et al. (2021).
To set up the soil moisture profile in the model, the soil moisture was initialized at saturation at the start of the growing cycle. This was because when planting cane points, the fields are irrigated first until saturated. To model water movement and availability in the soil, Aquacrop subdivides the soil depth into 12 compartments each of 0.1 m thickness (Steduto et al., 2012). Water and salt fluxes were computed at their boundaries by tracking irrigation, rainfall, evaporation, transpiration, run-off, capillary rise, and deep percolation. The daily rainfall, evaporation and transpiration were computed from the weather data. The sum of the water movements was the total soil water within the root zone. However, only the soil water between the field capacity and the permanent wilting point is available for plant use. This amount is denoted as the total available water (TAW) (mm/m) (Raes et al., 2018).
2.2 Calibration and validation
Without proper calibration, a model will produce yields which are different from actual values. A model must be calibrated so that its outputs are closer to reality. To calibrate a model, parameters must be adjusted. Calibration was done on 14 parameters by simulating several values for each parameter. During calibration, the irrigation scenario of 70%TAW was used. Key parameters which used local information were calibrated minimally to account for variation among the sugarcane estates, while parameters which used AquaCrop values were also calibrated to make the parameters more representative of the Guyana coast. To consider uncertainty in the model values, a sensitivity analysis was done alongside calibration. We tested the effect of varying the values within a range provided in the literature, or within 10% of the default or average value. For each parameter, a value was chosen based on the best agreement between the simulated and the reported actual yields from 2005 to 2008. The actual yield data which was reported by GuySuCo (2013), was converted to dry yield using a 30% dry matter factor (Steduto et al., 2012). The agreement between the actual and simulated yield was determined through the root mean square error (RMSE), percent RMSE (RMSEn) and mean bias error (MBE). For example, for the parameter “maximum canopy cover”, a value of “almost entirely covered (95%)” was the default based on the AquaCrop sugarcane file. The value “well covered (90%)” was also tested to see if it actually reflected the conditions in the field. The simulated yield for 95% had an RMSE of 1.90 ton/ha, RMSEn of 10.03% and MBE of +0.98 ton/ha when compared with the reported yield. Meanwhile, the set which used 90% had an RMSE of 1.64 ton/ha, RMSEn of 8.65% and MBE of +0.71 ton/ha. These lower values signified less error between the simulated and reported yields for 2005 to 2008, which led to a final calibrated value of 90% for the maximum canopy cover. Additional calibration of other parameters was done to further improve the agreement between the simulated and reported yields. An RMSEn value below 10% signifies that the parameters used were acceptable for simulating yield. Once the calibrated parameters were finalized, they were validated by simulating the yields for 2009 to 2012, and computing the RMSE, RMSEn, and MBE values for comparison between the simulated and the reported yields for the Region (GuySuCo, 2013). Same as with calibration, an RMSEn value below 10% confirms that the parameters have been successfully validated for simulating the yield.
2.3 Irrigation management scenario simulations
The irrigation scenarios were all continuous open-furrow irrigation with varying thresholds in terms of the total available soil water in the root zone (TAW). There were seven scenarios simulated, namely, 40, 50, 60, 70, 80, 90, and 100%TAW. Irrigation commenced when the threshold was reached, and irrigation was provided to maintain the %TAW above the threshold. The 100%TAW scenario corresponds to soil water content maintained at field capacity. Simulations were run for each scenario from 2009 to 2012 using the validated crop parameters.
3 Results and analysis
3.1 Yield modeling
Before calibration, the simulated yields for 2005 to 2008 were overestimated by 6.7 metric tons ha−1 or 10.6% compared to reported yields. During calibration, the error of the simulated yield was reduced by as much as 2% upon calibration of the crop coefficient (kcmax; Table 2). After calibration, the simulation error decreased to an MBE of 0.03-ton cane ha−1, and RMSE of 4.32-ton cane ha−1 (RMSE) which is equivalent to 6.83% of the average actual yield (Table 3). The calibrated parameters were then run from 2009 to 2012 for validation. Statistical analysis showed good agreement between the simulated and the actual yield with an MBE of +1.44 metric tons/ha and an RMSE of 4.39 metric tons/ha, which is equivalent to 7% of the reported yield.
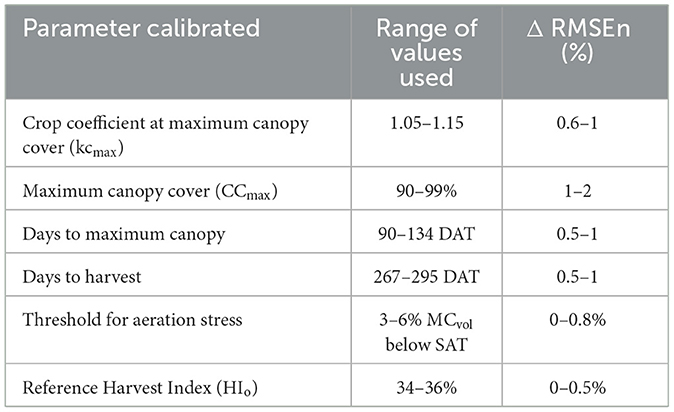
Table 2. Most sensitive calibrated parameters and range of values used with the corresponding ΔRMSEn.
3.2 Irrigation management scenarios
Each irrigation scenario was run from 2005 to 2012 to get simulated yields. An ANOVA of the yields shows that there is a significant difference among the scenarios (f-test, p < 0.05). The yield increased with increasing %TAW and plateaued at 80%TAW with 63-ton cane/ha, as shown in Figure 2. A lower mean yield was predicted at 50%TAW, however, a one-way t-test shows that the yield distribution at 50, 60, 70, 80, and 90%TAW is not significantly different from 100%TAW. The t-test compares not only the mean values but also the spread of the yield distribution considering variability in the weather. The 100%TAW was used as a reference since in this scenario, the soil is at field capacity, water stress is not present, and the highest yield was obtained. There was a statistically significant reduction in yield at 40%TAW. The irrigation requirements were also simulated and shown in Figure 3. Irrigation requirements decrease with a lower maintained %TAW.
Water productivity (WPet) is the yield produced per unit of water used for evaporation and transpiration (Steduto et al., 2012). The 60%TAW obtained the highest mean Wpet of 1.64 kg of dry biomass produced for each cubic meter of water used by evaporation and transpiration. However, both a one-way ANOVA and pairwise t-tests have confirmed that the differences in water productivity between all scenarios were minimal and not significant p > 0.05.
4 Discussion
4.1 Calibration and validation
The crop yield modeling provides important insights for forecasting yield and identifying impacts of stressors and management decisions. While there is statistical confidence in the changes in yield due to varying irrigation conditions, there is uncertainty in the exact value of projected yield because of inherent variability in the weather, spatial variability in the region, deviation from assumed values, and inherent errors in the model. By calibrating the model parameters to sugarcane cultivation in Guyana, we increased confidence in the results of AquaCrop. Without calibration, the simulated yield at 70%TAW, the current irrigation scheme, did not correctly represent the actual yield. The calibration of parameters led to a predicted yield error of 6.83% of the average actual yield. With an RMSEn of 7%, the validation conducted over a different set of years established the reliability of the model and the parameters used. The RMSEn falls within the range of error obtained in similar sugarcane validation studies using AquaCrop, such as by Alvar-Beltrán et al. (2021) who got an RMSEn of 11.6% for the yield of sugarcane in Pakistan; and Wellens et al. (2021) who obtained an RMSEn of 6.4% for biomass of sugarcane in Senegal. Another calibration-validation study by Jones and Singels (2018) using DSSAT-CANEGRO obtained an RMSEn of 23.46% for the aerial dry mass of sugarcane growth in South Africa. In our study, the AquaCrop model was observed to be most sensitive to changes in the values of the crop coefficient (kcmax) and maximum canopy cover (CCx) with the resulting RMSEn fluctuating between 0.6 and 2% (Table 2). These values were quite minimal and suggest that the crop parameters used before calibration were already representative of the conditions in the field. Notably, in Table 1, kcmax was a field-measured value provided in the Agriculture Operation Guidelines of GuySuCo (Eastwood, 2009). Meanwhile, the CCx was taken from the AquaCrop sugarcane base file.
4.2 Irrigation management scenarios
An increase in water productivity or a decrease in the irrigation water can improve the irrigation water use efficiency. We inspected first the WPet wherein there was no significant difference observed between the seven irrigation scenarios. The low irrigation scenarios do not increase nor decrease the water productivity of sugarcane in the Guyana coastal plains, but these scenarios could be as productive as full irrigation. The WPet can be improved through management practices which reduce evaporation, such as the application of soil amendments or mulches (Zahra et al., 2021; Kalanaki et al., 2022), shift to subsurface drip irrigation (Aydinsakir et al., 2021), crop rotations (Araya et al., 2017), and shift to alternate furrow irrigation (Mintesinot et al., 2004). These methods could be explored to improve the water productivity of sugarcane in Guyana. Table 4 shows a summary of irrigation studies on sugarcane with the recommended soil water thresholds, to achieve optimal water use and yield for different agro-climatic areas. Similar to our results, the water productivity, whether based on evapotranspiration (WPet) or transpiration (WPtr), was not significantly increased by reduced irrigation (Santos et al., 2019; Jamnani et al., 2022). Tayade et al. (2020) have shown a yield reduction in WPet between 100% and 50%. In cultivar studies (Coelho et al., 2018; Santos et al., 2019; Contiliani et al., 2023), an increase in water productivity has been observed between the interaction of deficit irrigation and sugarcane cultivar. The development of cultivars adapted to dry conditions is a more definitive method for increasing the water productivity of sugarcane compared to water-saving irrigation scenarios.
The second means of improving water use efficiency is to decrease the irrigation water provided while maintaining the yield. Lower irrigation thresholds such as 90, 80, 70, 60, 50, and 40% TAW, reduced the water use of each scenario. The scenarios between 80 and 100%TAW obtained the highest mean yield. The yield decreased below 80%TAW, but these changes were not statistically significant until 50%TAW. At 40%TAW, a significant (p < 0.05) yield penalty could be obtained. In Table 4, other sugarcane modeling studies on limited irrigation show that cane yield was not significantly reduced by some irrigation treatments (Bhingardeve et al., 2017; Bahmani and Eghbalian, 2018; Coelho et al., 2018; Dingre and Gorantiwar, 2021; Júnior et al., 2021; Jamnani et al., 2022). However, the threshold where the yield starts to significantly decrease is not consistent between these studies since the differences between their sites' soil texture and climate affect soil-water storage and the frequency of soil-water replenishment by rainfall. However, the different soil, climate and irrigation set-ups of the studies make it difficult to form a general conclusion regarding the appropriate irrigation threshold for sugarcane. In some of the reported studies (Dingre and Gorantiwar, 2021; Jamnani et al., 2022), when the soil-water was below a threshold (e.g. 60%TAW), an irrigation depth equal to the difference between 100%TAW and the threshold was applied. In other studies (Bhingardeve et al., 2017; Bahmani and Eghbalian, 2018; Santos et al., 2019; Tayade et al., 2020), the threshold of soil water content was not used; instead, a deficit was applied to the irrigation required for full irrigation, i.e., a 60%ETc treatment means that the irrigation provided was 60% of the 100%ETc treatment. The work of Dingre and Gorantiwar (2021) is most similar to our study in terms of the TAW and irrigation set-up. They evaluated 100, 70, and 40%TAW thresholds, wherein a significant reduction in yield was observed at the 40%TAW, like our study results. Regardless of the differences in methods and agro-climatic environment, the studies were conclusive that water-saving irrigation treatments can reduce irrigation water consumption at no significant reduction in the yield of sugarcane.
We are proposing a lower irrigation threshold wherein 50%TAW is the maintained soil-water content. There are other measures to manage irrigation when water is limited such as full irrigation of a reduced planted area (Ostad-Ali-Askari et al., 2017), and alternate furrow irrigation (Mintesinot et al., 2004; Tayade et al., 2020). The choice of furrow irrigation management to implement would benefit from an economic analysis which will consider the price of goods offered, the predicted yield and brix of sugarcane, and costs of water, labor, and materials.
4.3 The case of El Nino years
Among the years studied, the 2007 and 2010 growing periods received the lowest rainfall. These years coincide with the weak El Nino from Aug 2006 to Feb 2007, and moderate El Nino from June 2009 to April 2010 (NOAA, 2023). For both El Nino periods, using the current irrigation scheme at 70%TAW, at least 2,980 cubic meters of irrigation water per hectare are required for one whole growing season. The bulk of this irrigation volume was required between January to March when the sugarcane was at the tillering stage. Even in normal years, irrigation is necessary during these months for high yield. During the El Nino, these months became drier, and consequently, the irrigation requirement increased. Tillering is not the most water-intensive stage of sugarcane, however, water stress received during this stage has a significant impact on the yield compared to grand growth or maturity stages (Dingre and Gorantiwar, 2021). The next stage, the grand growth stage, is the most water-intensive stage due to its long duration and the development of the crop's full canopy (Eastwood, 2009). It starts in March and ends around July. The rainfall during these months is normally high and well-distributed, as shown in Figure 1. With the El Nino, even with reduced rainfall during these months, irrigation was not as crucial as that required during the tillering stage. Alternative irrigation methods, such as block scheduling or deficit irrigation, while maintaining the soil water content to at least 50%TAW could be applied from January to March if irrigation water supplies are limited. Moreover, the 50%TAW irrigation can already be started around November or December to increase stored irrigation water in the conservancies in anticipation of the dry conditions from January to March. Another method is to reduce the planting area of sugarcane based on the projected hectarage which can be fully irrigated during the tillering stage (January to March).
5 Conclusion
Irrigation management scenarios were simulated for sugarcane grown along the Guyana coastal plains on heavy clay soil. The sugarcane crop file of AquaCrop was successfully calibrated with reported yields from 2005 to 2008 and validated with yield data from 2009 to 2012. During calibration, the simulated yield did not show high sensitivity to changes in the values of crop parameters. The good agreement between the simulated and the reported yields during both calibration and validation showed that AquaCrop and its corresponding sugarcane crop file can be used to reliably simulate yields when used with field-measured soil and climate data, and key crop parameters: maximum canopy cover, crop coefficient, days to maximum canopy, and days to harvest.
Sugarcane yield at the current irrigation of 70% of the total available water (TAW), was compared with other irrigation management scenarios of 40, 50, 60, 80, 90, and 100%TAW. The yield was highest at 80%TAW to 100%TAW. An irrigation scenario of 70%TAW provides high yields and optimal water use. Keeping the soil-water content above 70%TAW uses more irrigation water, with minimal or no increase in the yield. Meanwhile, by using the 50%TAW threshold, yield is still not significantly affected and water use can be reduced by 63% compared to field capacity. This makes this irrigation scenario a viable management threshold during dry conditions when irrigation water is scarce. It is recommended to conduct field tests with the 50%TAW threshold to validate the yield.
A relationship between the hydraulic head maintained at the main irrigation channel regulator and the moisture level at 70%TAW in the field also ought to be determined to control the moisture in the fields, as it will further help understand the wetting pattern at the driest downstream sections of the fields.
Data availability statement
The datasets presented in this study can be found in online repositories. The names of the repository/repositories and accession number(s) can be found at: https://doi.org/10.6084/m9.figshare.23802297.
Author contributions
GM: Data curation, Formal analysis, Investigation, Methodology, Validation, Visualization, Writing—original draft, Writing—review & editing. CM: Conceptualization, Formal analysis, Funding acquisition, Methodology, Resources, Supervision, Writing—review & editing.
Funding
The authors declare that financial support was received from the International Development Research Centre (232165), Global Affairs Canada (106525-002), Natural Sciences and Engineering Research Council of Canada (223348), and the Macdonald Stewart Foundation for the research, authorship and publication of this article.
Acknowledgments
We would like to thank the following for providing data and information: GuySuCo, Raffaella Maria Pilati de Carvalho and Dr. Felexce Ngwa for collecting and analyzing the soil and climate data. We acknowledge a grant from the International Development Research Centre (IDRC), Ottawa, Canada, and with the financial support of the Government of Canada provided through Global Affairs Canada, the support of the NSERC and a fellowship to the senior author by the Macdonald Stewart Foundation under the Liliane and David M. Stewart Fellowship in Water Resources.
Conflict of interest
The authors declare that the research was conducted in the absence of any commercial or financial relationships that could be construed as a potential conflict of interest.
Publisher's note
All claims expressed in this article are solely those of the authors and do not necessarily represent those of their affiliated organizations, or those of the publisher, the editors and the reviewers. Any product that may be evaluated in this article, or claim that may be made by its manufacturer, is not guaranteed or endorsed by the publisher.
References
Adetoro, A. A., Abraham, S., Paraskevopoulos, A. L., Owusu-Sekyere, E., Jordaan, H., and Orimoloye, I. R. (2020). Alleviating water shortages by decreasing water footprint in sugarcane production: the impacts of different soil mulching and irrigation systems in South Africa. Groundw. Sustain. Dev. 11, 100464. doi: 10.1016/j.gsd.2020.100464
Alvar-Beltrán, J., Heureux, A., Soldan, R., Manzanas, R., Khan, B., and Dalla Marta, A. (2021). Assessing the impact of climate change on wheat and sugarcane with the AquaCrop model along the Indus River Basin, Pakistan. Agric. Water Manag. 253, 106909. doi: 10.1016/j.agwat.2021.106909
Arakawa, Y., Kusaba, T., Kubotera, H., Uezono, I., Miyamaru, N., Saeki, Y., et al. (2021). “Kyushu and Okinawa regions,” in The Soils of Japan, eds R. Hatano, H. Shinjo, and Y. Takata (Singapore: Springer), 327–365. doi: 10.1007/978-981-15-8229-5_10
Araya, A., Kisekka, I., Gowda, P. H., and Prasad, P. V. V. (2017). Evaluation of water-limited cropping systems in a semi-arid climate using DSSAT-CSM. Agric. Syst. 150, 86–98. doi: 10.1016/j.agsy.2016.10.007
Aydinsakir, K., Dinc, N., Buyuktas, D., Kocaturk, M., Ozkan, C. F., and Karaca, C. (2021). Water productivity of soybeans under regulated surface and subsurface drip irrigation conditions. Irrig. Sci. 39, 773–787. doi: 10.1007/s00271-021-00744-0
Bahmani, O., and Eghbalian, S. (2018). Simulating the response of sugarcane production to water deficit irrigation using the AquaCrop model. Agric. Res. 7, 158–166. doi: 10.1007/s40003-018-0311-0
Bastidas-Obando, E., Bastiaanssen, W. G. M., and Jarmain, C. (2017). Estimation of transpiration fluxes from rainfed and irrigated sugarcane in South Africa using a canopy resistance and crop coefficient model. Agric. Water Manag. 181, 94–107. doi: 10.1016/j.agwat.2016.11.024
Bhingardeve, S. D., Pawar, D. D., Hasure, R. R., and Dingre, S. K. (2017). Yield and yield attributes of sugarcane under deficit irrigated subsurface drip irrigation. Int. J. Agri. Innov. Res. 5, 974–982. Available at: https://api.semanticscholar.org/CorpusID:197465709
Biggs, J. S., Thorburn, P. J., Crimp, S., Masters, B., and Attard, S. J. (2013). Interactions between climate change and sugarcane management systems for improving water quality leaving farms in the Mackay Whitsunday region, Australia. Agric. Ecosyst. Environ. 180, 79–89. doi: 10.1016/j.agee.2011.11.005
Braun, E. G., and Derting, J. F. (1964). Map for the Reconnaissance Soil Survey of Northeast British Guiana. Available online at: https://esdac.jrc.ec.europa.eu/content/map-reconnaissance-soil-survey-northeast-british-guiana (accessed October 18, 2022).
Chopin, P., Doré, T., Guindé, L., and Blazy, J.-M. (2015). MOSAICA: a multi-scale bioeconomic model for the design and ex ante assessment of cropping system mosaics. Agric. Syst. 140, 26–39. doi: 10.1016/j.agsy.2015.08.006
Coelho, A. P., Dalri, A. B., Landell, E. P. A., Fischer Filho, J. A., Libardi, L. G. P., Bettiol, J. V. T., et al. (2018). Productivity, technological attributes and water use efficiency of sugarcane cultivars under regulated deficit irrigation. J. Agric. Sci. 10, 174. doi: 10.5539/jas.v10n7p174
Contiliani, D. F., Nebó, J. F. C. O., Ribeiro, R. V., Landell, M. G. de A., Pereira, T. C., Ming, R., et al. (2023). Drought-triggered leaf transcriptional responses disclose key molecular pathways underlying leaf water use efficiency in sugarcane (Saccharum spp.). Front. Plant Sci. 14, 1182461. doi: 10.3389/fpls.2023.1182461
da Costa Faria Martins, S., dos Santos, M. A., Lyra, G. B., de Souza, J. L., Lyra, G. B., Teodoro, I., et al. (2022). Actual evapotranspiration for sugarcane based on bowen ratio-energy balance and soil water balance models with optimized crop coefficients. Water Resour. Manag. 36, 4557–4574. doi: 10.1007/s11269-022-03263-5
da Silva, V. P. R., da Silva, B. B., Albuquerque, W. G., Borges, C. J. R., de Sousa, I. F., and Neto, J. D. (2013). Crop coefficient, water requirements, yield and water use efficiency of sugarcane growth in Brazil. Agric. Water Manag. 128, 102–109. doi: 10.1016/j.agwat.2013.06.007
Dhouib, M., Zitouna-Chebbi, R., Prévot, L., Molénat, J., Mekki, I., and Jacob, F. (2022). Multicriteria evaluation of the AquaCrop crop model in a hilly rainfed Mediterranean agrosystem. Agric. Water Manag. 273, 107912. doi: 10.1016/j.agwat.2022.107912
Dias, H. B., Inman-Bamber, G., Bermejo, R., Sentelhas, P. C., and Christodoulou, D. (2019). New APSIM-Sugar features and parameters required to account for high sugarcane yields in tropical environments. Field Crops Res. 235, 38–53. doi: 10.1016/j.fcr.2019.02.002
Dingre, S. K., and Gorantiwar, S. D. (2021). Sugarcane response to different soil water replenishment-based deficit irrigation treatments during different growth stages in an Indian semi-arid region. Irrig. Drain. 70, 1155–1171. doi: 10.1002/ird.2609
Dinssa, B., and Elias, E. (2021). Characterization and classification of soils of Bako Tibe District, West Shewa, Ethiopia. Heliyon 7, e08279. doi: 10.1016/j.heliyon.2021.e08279
Dlamini, P. J. (2021). Drought stress tolerance mechanisms and breeding effort in sugarcane: a review of progress and constraints in South Africa. Plant Stress 2, 100027. doi: 10.1016/j.stress.2021.100027
Eastwood, D. (2009). Agriculture Operations Guidelines. Georgetown: Guyana Sugar Corporation Limited.
FAO (1966). Report to the Government of Guyana on Soil Surveys. Rome: Food and Agriculture Organization of the United Nations. Available online at: https://edepot.wur.nl/483994
FAO (2006). Guidelines for Soil Description, 4th Edn. Rome: FAO. Available online at: http://www.fao.org/3/a0541e/a0541e.pdf
Farooq, N., and Gheewala, S. H. (2020). Assessing the impact of climate change on sugarcane and adaptation actions in Pakistan. Acta Geophys. 68, 1489–1503. doi: 10.1007/s11600-020-00463-8
Feleke, H. G., Savage, M., and Tesfaye, K. (2021). Calibration and validation of APSIM–Maize, DSSAT CERES–Maize and AquaCrop models for Ethiopian tropical environments. S. Afr. J. Plant Soil 38, 36–51. doi: 10.1080/02571862.2020.1837271
Gaj, N., and Madramootoo, C. A. (2017). Long-term simulations of the hydrology for sugarcane fields in the humid tropics: case study on Guyana's Coastland. J. Irrig. Drain. Eng. 143, 05017002. doi: 10.1061/(ASCE)IR.1943-4774.0001204
GLSC (2004). Region VI Sub-Regional Land Use Plan. Georgetown: Guyana Lands and Surveys Commission. Available online at: https://www.forestcarbonpartnership.org/system/files/documents/Guyana_Region_VI_Sub-Regional_Land_Use_Plan_0.pdf (accessed October 18, 2022).
GLSC (2013). Guyana National Land Use Plan. Georgetown: Guyana Lands and Surveys Commission. Available online at: https://glsc.gov.gy/wp-content/uploads/2017/05/Summary-Booklet-of-the-National-Land-Use-Plan.pdf (accessed October 18, 2022).
GuySuCo (2018). Annual Report 2018. Georgetown: Guyana Sugar Corporation Available online at: https://guysuco.gy/index.php?option=com_k2andview=itemandid=786:2018-reportandItemid=262andlang=en (accessed October 18, 2022).
Holmes, G. (2014). Australia's pesticide environmental risk assessment failure: the case of diuron and sugarcane. Mar. Pollut. Bull. 88, 7–13. doi: 10.1016/j.marpolbul.2014.08.007
Ibrahim, A. C. S., Adam, H., and Adeeb, A. (2002). Water-use efficiency of sorghum and groundnut under traditional and current irrigation in the Gezira scheme, Sudan. Irrig. Sci. 21, 115–125. doi: 10.1007/s00271-002-0057-z
Inman-Bamber, N. G., and McGlinchey, M. G. (2003). Crop coefficients and water-use estimates for sugarcane based on long-term Bowen ratio energy balance measurements. Field Crops Res. 83, 125–138. doi: 10.1016/S0378-4290(03)00069-8
Jamnani, M. R., Mirzaei, F., and Liaghat, A. (2022). Evaluation of sugarcane irrigation using AquaCrop model and remote sensing. Irrig. Drain. 71, 1034–1047. doi: 10.1002/ird.2718
Jones, J. W., Hoogenboom, G., Porter, C. H., Boote, K. J., Batchelor, W. D., Hunt, L. A., et al. (2003). The DSSAT cropping system model. Eur. J. Agron. 18, 235–265. doi: 10.1016/S1161-0301(02)00107-7
Jones, M. R., and Singels, A. (2018). Refining the Canegro model for improved simulation of climate change impacts on sugarcane. Eur. J. Agron. 100, 76–86. doi: 10.1016/j.eja.2017.12.009
Júnior, E. J. A., Júnior, J. A., Sena, C. C. R., Casaroli, D., Evangelista, A. W. P., and Battisti, R. (2021). Responses of different varieties of sugarcane to irrigation levels in the Cerrado. Aust. J. Crop Sci. 15, 1110–1118. doi: 10.21475/ajcs.21.15.08.p2978
Kalanaki, M., Karandish, F., Afrasiab, P., Ritzema, H., Khamari, I., and Tabatabai, S. M. (2022). Assessing the influence of integrating soil amendment applications with saline water irrigation on Ajwain's yield and water productivity. Irrig. Sci. 40, 71–85. doi: 10.1007/s00271-021-00759-7
Kennedy, A. J. (2022). Sugarcane variety development in the Caribbean. Sugar Tech. 24, 64–72. doi: 10.1007/s12355-020-00936-6
Kodikara, J., Barbour, S. L., and Fredlund, D. (2002). Structure development in surficial heavy clay soils: a synthesis of mechanisms. Aust. Geomech. J. 37, 25–40.
Lee, S. K., and Dang, T. A. (2018). Application of AquaCrop model to predict sugarcane yield under the climate change impact: a case study of Son Hoa district, Phu Yen province in Vietnam. Crop Res. 19, 310. doi: 10.5958/2348-7542.2018.00047.5
Mintesinot, B., Verplancke, H., Van Ranst, E., and Mitiku, H. (2004). Examining traditional irrigation methods, irrigation scheduling and alternate furrows irrigation on vertisols in northern Ethiopia. Agric. Water Manag. 64, 17–27. doi: 10.1016/S0378-3774(03)00194-X
NOAA (2023). NOAA National Weather Service Climate Prediction Center. Available online at: https://origin.cpc.ncep.noaa.gov/products/analysis_monitoring/ensostuff/ONI_v5.php (accessed February 16, 2023).
Olivier, F. C., and Singels, A. (2012). The effect of crop residue layers on evapotranspiration, growth and yield of irrigated sugarcane. Water SA 38, 77–86. doi: 10.4314/wsa.v38i1.10
Ostad-Ali-Askari, K., Shayannejad, M., Eslamian, S., Zamani, F., Shojaei, N., Navabpour, B., et al. (2017). “Deficit irrigation: optimization models,” in Handbook of Drought and Water Scarcity: Management of Drought and Water Scarcity, eds S. Eslamian and F. A. Eslamian (Boca Raton, FL: CRC Press), 373–389.
Peel, M. C., Finlayson, B. L., and McMahon, T. A. (2007). Updated world map of the Koppen-Geiger climate classification. Hydrol. Earth Syst. Sci. 11, 1633–1644. doi: 10.5194/hess-11-1633-2007
Pereira, L. S., Paredes, P., Hunsaker, D. J., López-Urrea, R., and Mohammadi Shad, Z. (2021). Standard single and basal crop coefficients for field crops: Updates and advances to the FAO56 crop water requirements method. Agric. Water Manag. 243, 106466. doi: 10.1016/j.agwat.2020.106466
Raes, D., Steduto, P., Hsiao, T. C., and Fereres, E. (2018). AquaCrop Reference Manual (version 6.0 - 6.1). Available online at: https://www.fao.org/aquacrop/resources/referencemanuals/en/ (accessed October 18, 2022).
Ritzema, H. P., Satyanarayana, T. V., Raman, S., and Boonstra, J. (2008). Subsurface drainage to combat waterlogging and salinity in irrigated lands in India: lessons learned in farmers' fields. Agric. Water Manag. 95, 179–189. doi: 10.1016/j.agwat.2007.09.012
Santos, L. C., Coelho, R. D., Barbosa, F. S., Leal, D. P. V., Fraga Júnior, E. F., Barros, T. H. S., et al. (2019). Influence of deficit irrigation on accumulation and partitioning of sugarcane biomass under drip irrigation in commercial varieties. Agric. Water Manag. 221, 322–333. doi: 10.1016/j.agwat.2019.05.013
Sheini-Dashtgol, A., Boroomand-Nasab, S., and Naseri, A.-A. (2020). Investigation effect of installation depths and dripper spacing on water productivity and sugarcane yield in subsurface drip irrigation. Agric. Sci. Pract. 5, 212–223. doi: 10.31248/JASP2020.225
Sierra, J., Causeret, F., and Chopin, P. (2017). A framework coupling farm typology and biophysical modelling to assess the impact of vegetable crop-based systems on soil carbon stocks. Application in the Caribbean. Agric. Syst. 153, 172–180. doi: 10.1016/j.agsy.2017.02.004
Singh, K., Pal, R., Chalotra, N., and Brar, A. S. (2022). Water productivity of sugarcane influenced by planting techniques, mulching and irrigation scheduling in Indo-Gangetic Plains of India. Sugar Tech. 24, 408–418. doi: 10.1007/s12355-021-01041-y
Steduto, P., Hsiao, T. C., Fereres, E., and Raes, D. (2012). Crop Yield Response to Water. Food and Agriculture Organization of the United Nations. Available online at: https://www.fao.org/3/i2800e/i2800e00.htm (accessed October 18, 2022).
Steduto, P., Hsiao, T. C., Raes, D., and Fereres, E. (2009). AquaCrop—The FAO crop model to simulate yield response to water: I. Concepts and underlying principles. Agron. J. 101, 426–437. doi: 10.2134/agronj2008.0139s
Steele, J. G., and Ramdin, H. N. (1980). Soil Resources of Guyana. Georgetown: Caribbean Food Corps Society.
Syers, J. K., Penning de Vries, F. W. T., and Nyamudeza, P., (eds.). (2001). The Sustainable Management of Vertisols. Oxon: CABI.
Tayade, A., Vasantha, S., Kumar, R. A., Anusha, S., Kumar, R., and Hemaprabha, G. (2020). Irrigation water use efficiency and water productivity of commercial sugarcane hybrids under water-limited conditions. Trans. ASABE 63, 123–132. doi: 10.13031/trans.13548
US Army Corps of Engineers (1998). Water Resources Assessment of Guyana. US Army Corps of Engineers. Available online at: https://www.sam.usace.army.mil/Portals/46/docs/military/engineering/docs/WRA/Guyana/Guyana%20WRA.pdf (accessed October 18, 2022).
van Antwerpen, R., van Heerden, P. D. R., Keeping, M. G., Titshall, L. W., Jumman, A., Tweddle, P. B., et al. (2022). “Chapter 2: A review of field management practices impacting root health in sugarcane,” in Advances in Agronomy, ed D. L. Sparks (Academic Press), 79–162. doi: 10.1016/bs.agron.2022.02.002
Wellens, J., Stasolla, M., Sall, M. T., Tychon, B., and Neyt, X. (2021). “Assimilation of sentinel-1 change detection in the aquacrop model: case of sugarcane,” in 2021 IEEE International Geoscience and Remote Sensing Symposium IGARSS (Brussels: IEEE), 1069–1072. doi: 10.1109/IGARSS47720.2021.9555163
Keywords: AquaCrop, sugarcane, Guyana, irrigation management, water use efficiency, furrow irrigation, heavy clay
Citation: Mortel GMM and Madramootoo CA (2023) Improving water use efficiency of surface irrigated sugarcane. Front. Water 5:1278306. doi: 10.3389/frwa.2023.1278306
Received: 16 August 2023; Accepted: 01 November 2023;
Published: 22 November 2023.
Edited by:
Paul Celicourt, Université du Québec, CanadaReviewed by:
Jean-Pascal Matteau, Laval University, CanadaKaveh Ostad-Ali-Askari, American University in Dubai, United Arab Emirates
Copyright © 2023 Mortel and Madramootoo. This is an open-access article distributed under the terms of the Creative Commons Attribution License (CC BY). The use, distribution or reproduction in other forums is permitted, provided the original author(s) and the copyright owner(s) are credited and that the original publication in this journal is cited, in accordance with accepted academic practice. No use, distribution or reproduction is permitted which does not comply with these terms.
*Correspondence: Guia Marie M. Mortel, guia.mortel@mail.mcgill.ca