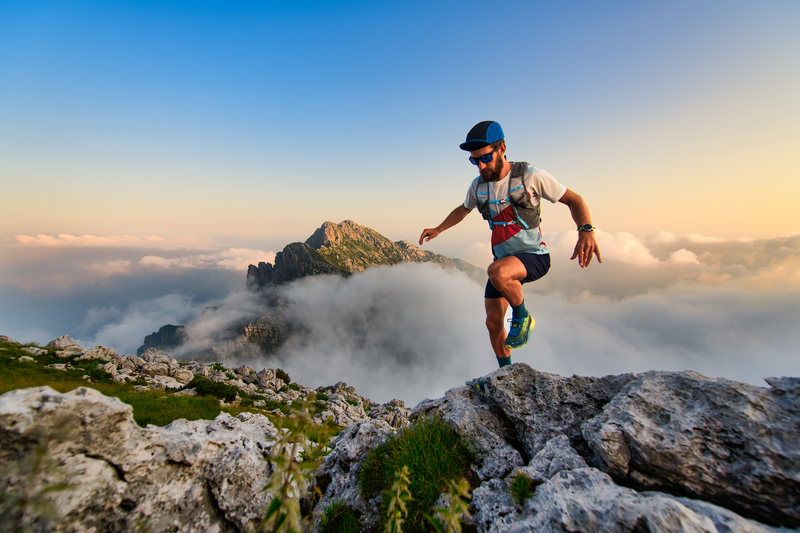
94% of researchers rate our articles as excellent or good
Learn more about the work of our research integrity team to safeguard the quality of each article we publish.
Find out more
EDITORIAL article
Front. Water , 01 June 2023
Sec. Water and Artificial Intelligence
Volume 5 - 2023 | https://doi.org/10.3389/frwa.2023.1215966
This article is part of the Research Topic Data-driven Machine Learning for Advancing Hydrological and Hydraulic Predictability View all 5 articles
Editorial on the Research Topic
Data-driven machine learning for advancing hydrological and hydraulic predictability
The growing influence of machine learning (ML) in every aspect of our lives has led to revolutionary advancements in our understanding, prediction, and decision-making capabilities. One field that stands to benefit greatly from applying these techniques includes hydrology and hydraulics. The ability to predict hydrological and hydraulic phenomena with greater accuracy and reliability is of utmost importance, given the increasing threats posed by climate change and extreme weather/climate events. In this editorial, we explore the significant contributions made by four recent studies that aim to advance hydrological and hydraulic predictability through data-driven ML.
ML models are data hungry. Their prediction performance highly depends on the quality and quantity of applied training data. Additionally, ML models are black-box. The model predictions usually lack physical explanation and are associated with significant errors and uncertainty under changing conditions. Among the four papers in this editorial, two studies focus on addressing data scarcity and the other two focus on explanation and uncertainty quantification of ML models to improve the prediction accuracy and trustworthiness.
In the paper “Simulating hydrological extremes for different warming levels–combining large scale climate ensembles with local observation-based machine learning models” (Hauswirth et al.), the authors have developed an innovative approach that combines large climate ensembles with a local, observation-based ML model to advance hydrological extreme predictability under different warming levels at local scales. This study showed that the combination of the wealth of information improved the prediction performance of hydrological extremes under different climate change scenarios for national, regional, and local scale assessments providing relevant information for water management in terms of long-term planning. This, in turn, provides insights into the potential impacts of different warming levels on hydrological extremes, such as floods and droughts, which are critical for effective water resource management and risk mitigation.
The study “Uncertainty quantification of machine learning models to improve streamflow prediction under changing climate and environmental conditions” (Liu et al.) addresses a key challenge in data-driven hydrological modeling, i.e., the uncertainty inherent in predictions caused by data noise and changing climate conditions. This research developed a novel uncertainty quantification technique and incorporated it into Long Short-Term Memory (LSTM) network to generate reliable and robust streamflow predictions under a changing climate. The calculated uncertainty agrees with confidence level and prediction errors, which helps avoid overconfidence and guides data collection and further model development. Such advancements are crucial, as they enable water managers to make better-informed decisions regarding water allocation, infrastructure planning, and flood control.
In the paper “Super-resolution and uncertainty estimation from sparse sensors of dynamical physical systems,” (Collins et al.) the authors used convolutional neural networks for global reconstruction of system variables from potentially scarce and noisy observations and explored the epistemic uncertainty of the ML model using Monte-Carlo batch normalization and Monte-Carlo dropout methods. They demonstrated the superior performance of the model on a wide range of hydrological applications, including sea-surface temperature, soil moisture, and incompressible near-surface flows. By utilizing super-resolution techniques and uncertainty estimation, this research offers a promising solution for hydrological and hydraulic modeling, where high-resolution data is often scarce but essential for accurate predictions.
Lastly, the study “Investigation of hydrometeorological influences on reservoir releases using explainable machine learning methods” (Fan et al.) utilizes explainable ML techniques to shed light on the complex relationships between hydrometeorological variables and reservoir releases. The authors applied the explanantion method to the LSTM network, which not only explains the variable importance but also provides variable-wise temporal importance of the hydrometeorological drivers to reservoir release prediction. This explanation will enable better reservoir operation and management practices under a changing climate. The use of explainable ML in this context promotes transparency and interpretability, which are crucial for fostering trust and facilitating the integration of ML models into decision-making processes.
Since the launch of this special issue in Frontiers in Water, numerous papers on this topic have been published (Chen et al., 2023; Karim et al., 2023; Shen et al., 2023; Xu et al., 2023; Yan et al., 2023), including both data-driven ML and hybrid modeling that integrates physics-based numerical and ML models (Slater et al., 2023). This shows the accelerated progress and evolution in utilizing ML in hydrology and hydraulics. The majority of these published works echo the optimistic view of the bright future of this emerging field, a perspective shared by this editorial and the four papers featured in this special issue. Although these papers deal with diverse applications, a recurring theme is the prevalent belief that data-driven and physics-based modeling are complementary. We can take advantages of both modeling techniques to advance hydrological and hydraulic predictability.
In conclusion, these four studies in this special issue and many concurrent publications elsewhere showcase the potential of data-driven ML to revolutionize the field of hydrology and hydraulics. By combining large-scale climate simulations with local observation-based models, incorporating uncertainty quantification techniques, reconstructing high-resolution data from sparse sensors, and employing explainable ML methods, researchers are paving the way for enhanced predictability and decision-making capabilities in water resource management. As we face an increasingly uncertain future due to climate change and other environmental challenges, embracing the power of ML in hydrological and hydraulic research is not only a promising avenue but a necessary one.
All authors listed have made a substantial, direct, and intellectual contribution to the work and approved it for publication.
The authors declare that the research was conducted in the absence of any commercial or financial relationships that could be construed as a potential conflict of interest.
All claims expressed in this article are solely those of the authors and do not necessarily represent those of their affiliated organizations, or those of the publisher, the editors and the reviewers. Any product that may be evaluated in this article, or claim that may be made by its manufacturer, is not guaranteed or endorsed by the publisher.
Chen, C., Zhang, H., Shi, W., Zhang, W., and Xue, Y. (2023). A novel paradigm for integrating physics-based numerical and machine learning models: a case study of eco-hydrological model. Environ. Modell. Softw. 163:105669. doi: 10.1016/j.envsoft.2023.105669
Karim, F., Armin, M. A., Ahmedt-Aristizabal, D., Tychsen-Smith, L., and Petersson, L. (2023). A review of hydrodynamic and machine learning approaches for flood inundation modeling. Water 15:566. doi: 10.3390/w15030566
Shen, C., Appling, A. P., Gentine, P., Bandai, T., Gupta, H., Tartakovsky, A., et al. (2023). Differentiable modeling to unify machine learning and physical models and advance Geosciences. arXiv preprint arXiv. doi: 10.5194/egusphere-egu23-15968
Slater, L. J., Arnal, L., Boucher, M. -A., Chang, A. Y. -Y., Moulds, S., Murphy, C., et al. (2023). Hybrid forecasting: blending climate predictions with AI models, Hydrol. Earth Syst. Sci., 27, 1865–1889. doi: 10.5194/hess-27-1865-2023
Xu, K., Han, Z., Xu, H., and Bin, L. (2023). Rapid prediction model for urban floods based on a light gradient boosting machine approach and hydrological–hydraulic model. Int. J. Disaster Risk Sci. 14, 79–97. doi: 10.1007/s13753-023-00465-2
Keywords: data-driven machine learning, hydrological and hydraulic predictability, uncertainty quantification, explainable machine learning, climate change
Citation: Lu D, Yang T and Liu X (2023) Editorial: Data-driven machine learning for advancing hydrological and hydraulic predictability. Front. Water 5:1215966. doi: 10.3389/frwa.2023.1215966
Received: 02 May 2023; Accepted: 22 May 2023;
Published: 01 June 2023.
Edited and reviewed by: Matteo Giuliani, Polytechnic University of Milan, Italy
Copyright © 2023 Lu, Yang and Liu. This is an open-access article distributed under the terms of the Creative Commons Attribution License (CC BY). The use, distribution or reproduction in other forums is permitted, provided the original author(s) and the copyright owner(s) are credited and that the original publication in this journal is cited, in accordance with accepted academic practice. No use, distribution or reproduction is permitted which does not comply with these terms.
*Correspondence: Dan Lu, bHVkMUBvcm5sLmdvdg==
Disclaimer: All claims expressed in this article are solely those of the authors and do not necessarily represent those of their affiliated organizations, or those of the publisher, the editors and the reviewers. Any product that may be evaluated in this article or claim that may be made by its manufacturer is not guaranteed or endorsed by the publisher.
Research integrity at Frontiers
Learn more about the work of our research integrity team to safeguard the quality of each article we publish.