- 1Groupe de recherche interuniversitaire en limnologie (GRIL), Département des sciences biologiques, Université du Québec à Montréal, Montréal, QC, Canada
- 2Center for Reservoir and Aquatic Systems Research and Department of Biology, Baylor University, Waco, TX, United States
- 3Institute of Marine Sciences and Department of Earth, Marine, and Environmental Sciences, University of North Carolina at Chapel Hill, Morehead City, NC, United States
- 4Department of Marine Sciences, University of Georgia, Athens, GA, United States
- 5Climate & Ecosystem Sciences, Earth and Environmental Sciences Area, Lawrence Berkeley National Laboratory, Berkeley, CA, United States
- 6Ecosystems and Global Change Group, Department of Plant Sciences, University of Cambridge, Cambridge, United Kingdom
- 7Earth and Biological Sciences Directorate, Pacific Northwest National Laboratory, Richland, WA, United States
- 8Environmental Radioactivity and Monitoring, Federal Institute of Hydrology (BfG), Koblenz, Germany
- 9Laboratory of Microbial Processes & Biodiversity, Departamento de Hidrobiologia, Universidade Federal de São Carlos (UFSCar), São Carlos, SP, Brazil
- 10Department of Biological and Ecological Engineering, Oregon State University, Corvallis, OR, United States
- 11Ecosystems and Global Change Group, School of the Environment, Trent University, Peterborough, ON, Canada
Introduction: Dissolved organic matter (DOM) composition varies over space and time, with a multitude of factors driving the presence or absence of each compound found in the complex DOM mixture. Compounds ubiquitously present across a wide range of river systems (hereafter termed core compounds) may differ in chemical composition and reactivity from compounds present in only a few settings (hereafter termed satellite compounds). Here, we investigated the spatial patterns in DOM molecular formulae presence (occupancy) in surface water and sediments across 97 river corridors at a continental scale using the “Worldwide Hydrobiogeochemical Observation Network for Dynamic River Systems—WHONDRS” research consortium.
Methods: We used a novel data-driven approach to identify core and satellite compounds and compared their molecular properties identified with Fourier-transform ion cyclotron resonance mass spectrometry (FT-ICR MS).
Results: We found that core compounds clustered around intermediate hydrogen/carbon and oxygen/carbon ratios across both sediment and surface water samples, whereas the satellite compounds varied widely in their elemental composition. Within surface water samples, core compounds were dominated by lignin-like formulae, whereas protein-like formulae dominated the core pool in sediment samples. In contrast, satellite molecular formulae were more evenly distributed between compound classes in both sediment and water molecules. Core compounds found in both sediment and water exhibited lower molecular mass, lower oxidation state, and a higher degree of aromaticity, and were inferred to be more persistent than global satellite compounds. Higher putative biochemical transformations were found in core than satellite compounds, suggesting that the core pool was more processed.
Discussion: The observed differences in chemical properties of core and satellite compounds point to potential differences in their sources and contribution to DOM processing in river corridors. Overall, our work points to the potential of data-driven approaches separating rare and common compounds to reduce some of the complexity inherent in studying riverine DOM.
1. Introduction
Dissolved organic matter (DOM) is a complex mixture consisting of thousands of individual molecular compounds that vary in composition and abundance (Catalán et al., 2021). This complexity is a product of a wide range of DOM sources and transformation processes, sample history and environmental settings (Cooper et al., 2022). DOM is an essential link between the abiotic and biotic world, as it is the primary resource for heterotrophic bacteria (Azam et al., 1983). Hence, DOM composition can affect ecosystem metabolism (Lapierre et al., 2013) and shape the base of the aquatic food-web (Guillemette et al., 2016). With riverine transport being a major connection between land and oceans (Regnier et al., 2022), inland DOM composition can impact not only freshwater but also marine ecosystems.
To enhance our understanding of these complex mixtures, high-resolution mass spectrometry techniques have been employed to investigate DOM composition, metabolism, and transport in diverse ecosystems across scales (Jaffé et al., 2012; Seidel et al., 2015; Wagner et al., 2015; Kellerman et al., 2018; Stegen et al., 2018). Higher resolution introduces significant analytical and conceptual challenges within DOM research that have been reviewed elsewhere (Bahureksa et al., 2021; Qi et al., 2022). While DOM molecules can significantly vary in composition across systems, the notion that a group of its constituents is ubiquitous through space and time has been previously observed in aquatic systems (e.g., Kellerman et al., 2014; Zark and Dittmar, 2018). Yet, the study of the specific characteristics of rare and common DOM molecules in riverine sediments and water at a continental scale have not been evaluated. Using terminology borrowed from ecology (Hanski, 1982), we refer to the common constituents as “core” compounds, differentiating them from those present in only a few settings, which we refer to as “satellite” compounds.
In ecology, the core-satellite (biological) species hypothesis was introduced to explore the bimodal abundance distribution of organisms in the environment. The observed distribution results from the combined effects of colonization, adaptation to local conditions, niche partitioning, and competition dynamics, implying connectivity and stochasticity across geographic gradients (Hanski, 1982; Gaston et al., 2000). Ecological communities often show a positive correlation between species' local abundances and the number of sites where they occur regionally (spatial occupancy) (Hartley, 1998), with a large fraction of species often representing a small fraction of the overall abundance (i.e., left-tailed occupancy-frequency distribution). Core and satellite species can thus reflect functionally different groups (Fonte et al., 2021). Identifying core species can help pinpoint organisms that potentially have key functions, and help infer the processes that determine dominance and endemism (Mehranvar and Jackson, 2001; Lindh et al., 2017). More recently, the core-satellite model has also been applied to test hypotheses relating microbial community structure (Wu et al., 2019) and gene variants (Escalas et al., 2022) to ecosystem function, and to investigate spatial variability in microbial community composition (Ling et al., 2015; Lindh et al., 2017; Zhang et al., 2019; Mateus-Barros et al., 2021). Analogous to microbial communities, DOM mixtures are complex and deconstructing the movement and processing of DOM compounds within and across environments is challenging. For this purpose, it has been suggested to test the applicability of concepts and tools developed to study other complex mixtures to study DOM in the environment (Danczak et al., 2020, 2021; Hu et al., 2022).
The applicability of the core-satellite model to decipher groups of DOM molecules that behave similarly across environments has been indicated in results of prior work, but has not yet been further explored (Zark and Dittmar, 2018; Garayburu-Caruso et al., 2020; Danczak et al., 2021). Studies of aquatic ecosystems have demonstrated that a portion of the DOM pool evades degradation and remains widespread across environments (Lechtenfeld et al., 2014; Zark and Dittmar, 2018). This widely present DOM pool has been characterized as less saturated, more oxygenated and containing fewer nitrogen and sulfur constituents, making it less susceptible or favorable to microbial degradation (Riedel et al., 2013; MacDonald et al., 2021). The ubiquitous occupancy of certain DOM fractions has been interpreted to represent environmental stability, and a recent study has described an “island of stability” associated with old and more persistent compounds (Lechtenfeld et al., 2014). The island of stability partially overlaps with carboxylic-rich alicyclic molecules (CRAMs) that have been suggested to persist over time because of their resistance to microbial degradation (Hertkorn et al., 2006; Roth et al., 2014).
While the processes responsible for forming stable molecules such as CRAMs remain unclear, studies finding persistent compounds across ecosystems collectively suggest that molecules can accumulate in aquatic environments and become common, thus core, components of DOM (Kellerman et al., 2018; Zark and Dittmar, 2018). In contrast, satellite compounds may be readily consumed (short-lived and hence labile), ecosystem-specific, or represent intermediate stages of microbial degradation. It has been suggested that via multiple transformations (i.e., recycling) of complex DOM molecules, thousands of smaller, low molecular weight compounds can be formed (Koch et al., 2005; Hach et al., 2020), which may become part of the satellite molecule pool. Within freshwaters it has been suggested that compounds with a high degree of aromaticity and high nominal oxidation state of carbon (NOSC) tend to be more labile in part due to their light sensitivity and thus have a high potential to be part of the satellite pool. In contrast, aliphatic compounds with a low NOSC tend to be more persistent, and thus have a higher potential to belong to the core molecule pool (Kellerman et al., 2015). In addition to intrinsic molecular characteristics, external conditions may affect persistence (Schmidt et al., 2011; Kellerman et al., 2015). For example, sediments are characterized by longer retention times and stronger biogeochemical gradients than surface waters, which further depend on river bed geology (e.g., porosity), and substantial differences in DOM composition between sediment porewater and surface waters have been observed (Garayburu-Caruso et al., 2020). Furthermore, compounds can drop in concentration below a minimum threshold for them to be available to microorganisms (Jannasch, 1995), and they may no longer be broken down. Such persistence due to dilution was suggested for the deep ocean (Arrieta et al., 2015) and may lead to the presence of molecules across different sites and settings, making them part of the core molecule pool. To our knowledge, this concept is unexplored in river corridors.
DOM fluxes and interactions between river beds and surface waters are bi-directional. Upwelling and perturbation can make hyporheic and sediment DOM available to the surface zone. By contrast, low flow rates may lead to the sinking and accumulation of settling particles that thicken sediments and make surface water DOM available for sediment processing (Angel, 1984; Turner, 2002; Allan et al., 2021). The DOM composition can also be altered by abiotic coagulation processes in sediment porewater. For instance, free ions such as ferrous iron can coagulate with specific fractions of DOM, thereby altering the overall DOM pool (Linkhorst et al., 2017). The zone in the river bed characterized by the mixing of surface- and groundwater (i.e., hyporheic zone) has been shown to have a distinct DOM composition, transforming more labile matter that enters through downwelling, and releasing freshly produced, humified DOM in upwelling zones from gravel bars (Boodoo et al., 2020). It has been suggested that sediment and surface water DOM is largely decoupled at baseflow in summer, with distinct DOM signatures typical of autochthonous (i.e., internally produced) DOM in the surface waters (Fasching et al., 2016). However, rarely are sediment and surface water samples analyzed together using high-resolution approaches, which could provide further insight into the bi-directional relationship of these highly connected environments.
In this study, we investigated the occupancy patterns of molecular DOM in surface water and sediment across river systems of various biomes to assess whether there was a fundamental difference in the composition of frequently and rarely encountered DOM. To that end, we utilized DOM samples collected during the Worldwide Hydrobiogeochemical Observation Network for Dynamic River Systems (WHONDRS) Summer 2019 sampling effort (Borton et al., 2022). We identified core compounds as those commonly observed in surface water and sediment and satellite compounds as those infrequently encountered, with a sparse spatial distribution. Traditionally in population ecology, core and satellite species have been identified by grouping all species above and below a frequency threshold, respectively. This threshold, however, has varied largely from 50 to 90% site-occupancy across studies (Besemer et al., 2013; Barnes et al., 2016; Hassell et al., 2018). Here, we applied novel data-driven approaches to avoid such arbitrary choices. We then estimated molecular properties from the molecular formulae derived from Fourier-transform ion cyclotron resonance mass spectrometry (FT-ICR MS) data and explored similarities and differences between core and satellite compounds. A unique aspect of our work is the comparison between sediment and surface water samples. Capturing shifts in the properties of molecules abundant in both sediment and surface water samples allowed us to draw inferences on the underlying processes common across a wide range of diverse river corridors.
2. Materials and methods
2.1. Sample collection and pre-processing
The WHONDRS consortium based out of the Pacific Northwest National Laboratory (PNNL) was responsible for data collection and preprocessing. Details regarding the design of sampling campaigns and protocols, sample collection, data generation and processing can be found in Garayburu-Caruso et al. (2020) and Borton et al. (2022).
Briefly, for this study, surface water and sediment samples as well as metadata, climate, vegetation, and geospatial data were collected from 97 sites between July and August 2019. Surface water samples were collected in triplicate and filtered through 0.22 μm SterivexTM filters (EMD Millipore) into pre-acidified 40 mL glass vials (I-Chem amber VOA glass vials; ThermoFisher, pre-acidified with 10 μL of 85% phosphoric acid). Sediment samples were collected from a depositional zone upstream, midstream, or downstream within the sampling site. Samples were collected only from sediment saturated with water. Samples were shipped to PNNL on blue ice within 24 h of collection. Once in the lab, surface water samples were frozen at −20 °C until analysis. Sediment samples were individually sieved to <2 mm, subsampled, and stored at −20 °C. Water soluble (i.e., <0.22 μm filter size) organic matter (i.e., DOM) from sediments was extracted prior to analysis by shaking the sediments with MilliQ water for 2 h (Tfaily et al., 2017). Non-purgeable organic carbon (NPOC) was determined in the surface water samples and sediment extracts using a combustion carbon analyzer (TOC-L CSH/CSN E100V) with an ASI-L autosampler. NPOC concentrations were normalized via dilution to 1.5 mg C L−1 across all samples before solid phase extraction (SPE) with PPL (Priority PolLutant, Bond Elut) cartridges using methanol for final elution (Dittmar et al., 2008). The normalization allows for FT-ICR MS data comparison across sites within this study and other WHONDRS sampling campaigns.
2.2. FT-ICR MS data collection
To avoid the impact different instrument parameters can have on FT-ICR MS analysis (Hawkes et al., 2020), the 12 Tesla Bruker SolariX Fourier transform ion cyclotron mass spectrometer (12 T FT-ICR MS; Bruker, SolariX, Billerica, MA, USA) located at the Environmental Molecular Sciences Laboratory in Richland, WA, USA, was used to collect ultrahigh-resolution mass spectra of surface water and sediment extracts for all samples. Data were collected in negative mode with an ion accumulation of 0.05 s for surface water and 0.1 or 0.2 s (depending on sample quality) for sediment from 100–900 m/z at 4 M. BrukerDaltonik Data Analysis software (version 4.2) was used to convert raw spectra to a list of m/z values by applying a signal-to-noise ratio (S/N) of 7 and an absolute intensity threshold to the default value of 100. Peaks were aligned, and molecular formulae were assigned using the Formularity software (Tolić et al., 2017). Formularity outputs were post-processed using the R package ftmsRanalysis (Bramer et al., 2020). This package removes peaks outside of a high confidence m/z range (200 m/z−900 m/z) and/or with a 13C isotopic signature and calculates molecular formula properties and chemical classes (Kim et al., 2003; Koch and Dittmar, 2006; LaRowe and Van Cappellen, 2011). Subsequently, molecular formulae were classified into amino sugar-like, carbohydrate-like, condensed aromatic-like, lignin-likes, lipid-like, protein-like, tannin-like, and unsaturated hydrocarbon-like compounds using the assign_class() function (Kim et al., 2003).
2.3. FT-ICR MS data processing
Peak intensities were transformed into presence-absence data. The up-, mid-, and downstream sediment samples were treated as triplicates. The peaks and molecular formulae were kept for subsequent analyses if they were found in at least one of the replicates. Peaks that were assigned the same molecular formula due to minor mass differences were merged together. Only peaks with an assigned molecular formula and with an elemental combination of C1 − 130, H1 − 200, O1 − 50, N0 − 4, S0 − 2, and P0 − 1 were retained (Riedel and Dittmar, 2014). Only molecular formulae in the range of 0.3 ≥ H/C ≤ 2.2 and O/C ≤ 1.2 (Hawkes et al., 2020) and double bond equivalents minus oxygen ≤10 were considered reliable based on chemical feasibility (Herzsprung et al., 2014).
Molecular properties were computed for peaks with a molecular formula assigned. NOSC (unitless), calculated according to Koch and Dittmar (2006), indicates the nominal oxidation state of carbon. GFE (in kJ/mol C) is an estimate of the Gibbs Free Energy of the half-reaction oxidizing the organic compound on a per carbon basis obtained using the inverse linear relationship with NOSC presented by LaRowe and Van Cappellen (2011); their equation 14, with lower values indicating more favorable thermodynamic conditions for the oxidation of the organic molecule. The double bond equivalent (DBE; unitless) is a measure of unsaturation, indicating the number of double bonds and aromatic rings that a compound has, computed as 1 + C – O – S – 0.5 × (N + P + H) (Koch and Dittmar, 2006). AImod (unitless) quantifies the degree of aromaticity of a molecule by considering the C-C double-bond density and the contribution of oxygen bonds by heteroatoms (based on the assumption that only half of the oxygen is bound; Koch and Dittmar, 2006). The “island of stability” is identified as molecular formulae falling into the following range: H/C: 1.17 ± 0.13, O/C: 0.52 ± 0.10 and molecular mass: 360 ± 28 and 497 ± 51 Da (Lechtenfeld et al., 2014). The island of stability was shown in the Van Krevelen space using solely the H/C and O/C ranges.
Putative biochemical transformations were calculated in the merged dataset following methods previously employed by Graham et al. (2017, 2018), Danczak et al. (2020), and Garayburu-Caruso et al. (2020). Briefly, we determined the pairwise differences between peak masses present in each sample. The differences were matched (1 ppm error) to a database of 1,298 frequently observed biochemical transformations (available on Github), which enabled us to infer the putative gain or loss of a specific molecule via biochemical transformations. For example, a mass difference of m/z = 71.0371 corresponds to the gain or loss of alanine. The number of times a specific peak was involved in a putative transformation was used to calculate the typical number of transformations per peak per sample. For this, we calculated the mean and standard error of the number of transformations for each individual peak in surface waters and sediments from each site. Based on the classification of each peak and its corresponding molecular formulae as core or satellite, the number of transformations were then visualized for various pools.
2.4. Identifying the core-satellite threshold
The core-satellite species hypothesis is frequently used in biodiversity studies; however, the occupancy threshold to determine core-satellite distinction is highly variable with environment (i.e., riverine, marine, soil, or mammal and fish gut microbiomes) and species of interest (i.e., amphibians, bacteria, parasites, macrophytes), which leads to widely varying thresholds used in the literature that range from 50% (Besemer et al., 2013) to 75% (Barnes et al., 2016), 90% (Lindh et al., 2017; Hassell et al., 2018), or 100% (Hugoni et al., 2021). Thus, using the data itself to guide the classification of molecules is desirable to minimize subjectivity. A data-driven framework was applied to find the core-satellite threshold within our dataset (hereafter, “emergent threshold”). The emergent threshold was compared with two additional data-driven approaches, namely principal component analysis and random forest analysis, as outlined in the Supplementary Table S1, yielding similar results. The emergent threshold has the advantage of differentiating the extremes (present at many or few sites) and parsing out a fraction of molecules that are intermittently present across samples. Hence, only the emergent core-satellite definition has been kept for downstream analyses. In this approach, first, occupancy of each molecular formula within each environment (sediment and surface water) was calculated, where occupancy of 100% implies the presence of a molecular formula at every site (sediment = 93 sites, surface water = 95 sites). Next, frequency-occupancy curves were generated for both sediment and surface water samples. Curves were smoothed using the smooth.spline function in base R, setting the smoothing parameter spar to 0.5. The percent occupancy threshold that corresponds to moments of acceleration along the curve was identified by taking the second derivative of the log-transformed curve. All molecular formulae falling below the first moment of minimum acceleration were identified as “satellite”, and all above the second last point of maximum and minimum acceleration were classified as “core”, for sediment and surface water, respectively. This peak detection method is highly sensitive and largely depends on the shape of the curve. As the curve shapes for the two environments were slightly different, two similar but different points of acceleration were used to identify the core threshold for the two environments (Supplementary Figure S1, Stadler and del Giorgio, 2022). Different smoothing methods were compared (Supplementary Figure S2).
2.5. Statistical analyses
All figures and statistical analyses present in this study were conducted using the platforms R (R Core Team, 2022; RStudio Team, 2022) and Python 3.7 (Van Rossum and Drake, 2009). All figures were created using the R ggplot2 package (Wickham, 2016) if not otherwise stated.
To grasp the magnitude of how many molecular formulae were identified as core and satellite, and understand the differences between sediment and surface water samples, species richness was calculated using the estimateR and diversity function in the vegan package (Oksanen et al., 2016). Pairwise Kruskal-Wallis H tests were performed, where the null hypothesis was that the population medians of all of the pairs were equal. The test works on two or more independent samples, which may have different sizes. This statistical test was performed using the stats function in the Python scipy package (Virtanen et al., 2020) in the Google Colab environment. Relative abundance plots were produced using the Python matplotlib package (Hunter, 2007).
Seven cross-environmental groups were defined consisting of molecular formulae based on their core/in-between/satellite classification across environments, where core denotes high occupancy (frequently present molecular formulae), satellite denotes low occupancy and in-between encompass molecular formulae that are present at intermediate frequencies (see section 3.1). All molecular formulae that were consistently in one category across environments were classified as (i) global core, (ii) global in-between, and (iii) global satellite. Any molecular formulae that switched between categories were identified as (iv) Sediment Core—Water Satellite/In-between, (v) Sediment Satellite—Water In-between, (vi) Water Core—Sediment Satellite/In-between and (vii) Water Satellite—Sediment In-between. Sediment core—water satellite and sediment core—water in-between as well as water core—sediment satellite and water core—sediment in-between classes were initially evaluated separately, but were merged due to their overlapping distribution across Van-Krevelen space (not shown).
We tested differences in molecular properties and putative transformations between core, in-between, and satellite groups and between cross-environment groups using analysis of variance. First, we checked data normality and equality of variances. Since test assumptions were not fulfilled, non-parametric versions were applied. We used the kruskal.test function to evaluate general statistical differences among groups. The non-parametric post-hoc Dunn's test was further used to compute two-sided p-values for each pairwise comparison among two groups that were found to be significantly different from each other. Holm's method to adjust p-values for multiple comparisons was used within the dunnTest function (Holm, 1979). Both kruskal.test and dunnTest functions are from the R FSA package (Ogle et al., 2022). Principal Coordinate Analysis (PCoA) based on beta-dispersion statistics [betadisper function in R vegan package (Oksanen et al., 2016)] was used to measure the degree of dissimilarity within each group of interest. We then used Tukey's Honest Significant Difference [Tukey's HSD; TukeyHSD function in R stats package (R Core Team, 2022)] to compare these dissimilarities pair-to-pair and determine if one group presented greater or smaller variation than others. For these analyses, we grouped the molecules by their presence in both sediment and surface water samples. A p-value ≤0.05 was used for determining whether an observed difference was statistically significant.
3. Results
We present the assessment of rare and commonly encountered molecular DOM formulae in surface water and sediments across a wide range of riverine environments. We describe and compare the prevalence of chemical classes between environments, and the estimated extent of transformations they have undergone.
3.1. The FT-ICR MS dataset: General overview
From the FT-ICR MS dataset, a total of 91,338 unique peaks were originally identified in all samples combined. In total 41% (37,528) of all peaks had molecular formulae assigned. After initial data processing (e.g., replicate merging) and quality filtering, 76% (28,660) of all peaks assigned with a molecular formula remained for downstream analyses. From the final complete set of molecular formulae, 38% (10,976) occurred only in sediment samples, 35% (9,907) only in surface water samples, and 27% (7,777) in both environments.
From all molecular formulae identified in sediment samples, 7% (1,294) were classified as core, and 72% (12,791) as satellite molecular formulae. From the total set of molecular formulae identified in surface water samples, 11% (2,073) were classified as core, and 74% (13,880) as satellite molecular formulae. In sediment and surface water samples, 3,599 and 2,800 (20 and 15%) molecular formulae were classified as neither core nor satellite molecules, respectively, and we refer to these as “in-between” molecules. The occupancy threshold for water samples was identified as ≤18% (satellite) and ≥91% (core) occupancy, with molecular formulae occurring at 19–90% of sites classified as in-between (Supplementary Figures S1a–c). The threshold for sediment samples was found to be ≤18% (satellite) and ≥93% (core) occupancy, and molecular formulae of 19–92% occupancy were identified as in-between (Supplementary Figures S1d–f). No molecular formulae were found to occur at all sites across and within surface water and sediment environments. The classifications were compared between the molecular formula and peak datasets, and their fractions were similar, with satellite molecules occurring most frequently in both datasets, followed by in-between and core (Supplementary Table S2).
We confirmed that different approaches to delineate rare vs. common occurrences did not substantially affect the classification of formulae into core, satellite, and in-between groupings of FT-ICR MS data. The designations were largely aligned between diverse methods including an emergent threshold, a multivariate approach based on literature thresholds, and a machine learning algorithm as explained in the methods and Supplementary Table S1.
The total number of molecular formulae in surface water and sediment samples was similar (17,684 vs. 18,753). However, when looking at individual samples, we found that the relative abundance of molecular formulae classified as satellite and core significantly differed between samples taken from surface waters vs. sediments (Figure 1A). On average, surface water samples had a significantly higher number of commonly present molecular formulae (i.e., core) than sediment samples. In contrast, there was a significantly larger number of rare compounds (i.e., satellite) in sediment than in surface water samples. There was no statistically significant difference between the number of molecular formulae (i.e., richness) in water and sediment in the in-between pool (Figure 1).
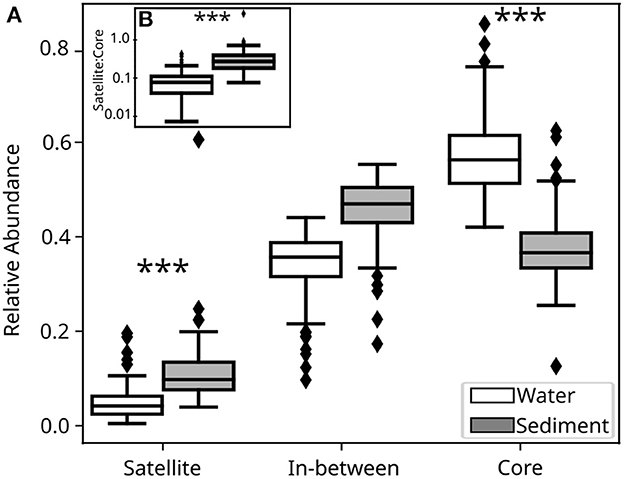
Figure 1. (A) Boxplots comparing the relative abundance of groups within individual samples from surface water (white) and sediment (gray). The inset (B) shows the satellite:core ratios for individual surface water and sediment samples. The relative abundance is the number of molecular formulae assigned to a group (satellite/in-between/core) to the total number of molecular formulae within a sample, whereas the overall ratio is the number of satellite compounds divided by the number of core compounds. Differences in the medians between sediment and surface water samples for each group were tested using the Kruskal-Wallis H-test. Statistical differences (p < 0.05) between the medians of sediments and surface waters are denoted with a triple asterisk. Boxplots show the median and first and third quartiles, whiskers extend to the farthest data point (within 1.5 times the interquartile range), and diamonds are outliers.
The ratio of satellite to core molecular formulae in a sample was below 1 in both sediment and water, pointing to the prevalence of molecular formulae that are commonly present in almost any given sample (Figure 1B). This is particularly the case in surface water samples, which are characterized by a low fraction of satellite (median <0.05) and a large proportion of core molecular formulae (median > 0.5; Figure 1B).
3.2. Molecular characteristics of core and satellite molecular formulae
To evaluate whether molecular formulae classified as core and satellite were chemically different, we first examined their distribution across Van Krevelen space (Supplementary Figure S3). We found that the core molecules clustered around intermediate H/C and O/C ratios across both sediment and surface water samples, whereas the satellite molecules were largely spread out across Van Krevelen space. Core molecules were generally within the region of the island of stability, i.e. molecules that have been found to be highly persistent (Lechtenfeld et al., 2014). In the sediment samples, core molecules tended to be more oxidized than satellite molecules (Supplementary Figure S3a). Core molecular formulae in the sediment samples showed a higher frequency at high O/C (mode ~0.4) compared to satellite molecular formulae, which showed a high frequency at low O/C (mode ~0.23). Satellite molecular formulae in the sediment samples showed a wide range of different H/C (0–2.5), whereas core molecular formulae showed high frequency in intermediate H/C centered at 1.7. For our dataset of surface water samples (Supplementary Figure S3b), we found a similar trend as described above for sediment samples. Core molecules in surface water samples tended to be more oxidized than satellite molecules, indicated by higher O/C ratios in core than in satellite molecular formulae (peaks around 0.5 and 0.3, respectively; Supplementary Figure S3b). Similar to the sediment, surface water samples showed a wide range of different H/C (0–2.5). In contrast to sediment samples, the H/C ratio of core molecules was higher than the H/C ratio of satellite molecules in surface water (Supplementary Figure S3).
We classified all 28,660 molecular formulae into putative chemical compound classes, and calculated their relative contribution to the respective core-satellite classification across environments (e.g., number of mass peaks classified as amino sugars in relation to all mass peaks detected in core molecules of sediment samples) (Figure 2). Within sediment samples, protein-like compounds were the most common molecular class in the core pool (41.3%), followed by lignin-like (28.5%), and lipid-like compounds (12.4%). Similarly, the most common compound classes within the in-between pool were lignin-like (29.8%), protein-like (24.8%), and lipid-like (14.1%) compounds. Among the satellite molecules, lignin-like (29.2%) and lipid-like compounds (23.0%) were more prevalent. Other classes such as condensed aromatic-like, tannin-like, and carbohydrate-like compounds were more evenly distributed compared to the core pool. Compounds classified as protein-like and amino sugar-like were less represented in the satellite (15.1 and 4.5%) than in the core pool (41.3 and 8.4%).
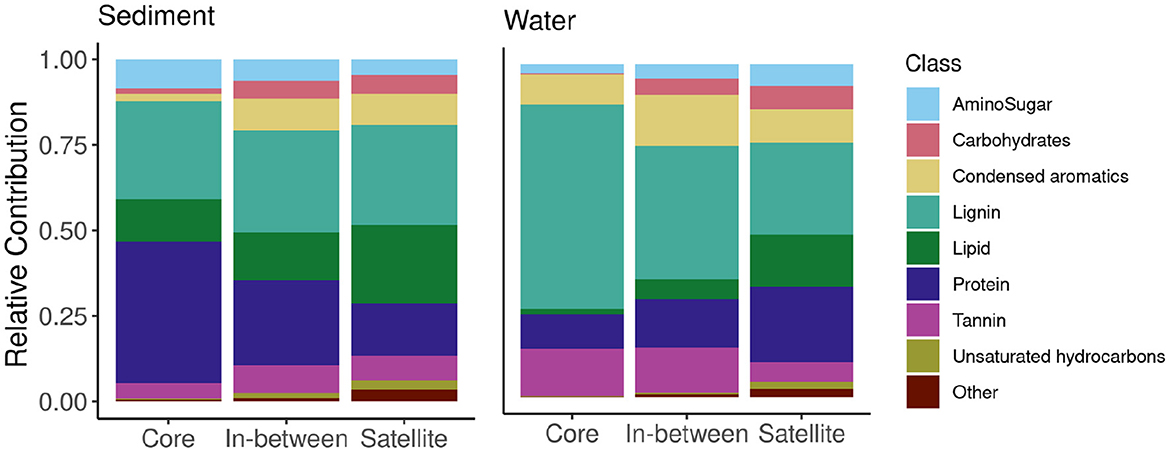
Figure 2. Relative contribution (%) of potential compound classes to core, in-between and satellite molecular formulae in sediment and water samples.
Within surface water samples, the largest number of molecular formulae found in the core pool were lignin-like compounds (61.6%), followed by tannin-like (14.4%), and protein-like (10.2%) compounds, which were less prevalent but still comprised a substantial fraction within the satellite pool (38.8, 10.4, and 13.9%, respectively). Within the in-between pool, lignin-like compounds were most common (49.4%), and tannin-like (13.2%), condensed aromatic-like (11.9%), and protein-like (11.3%) compounds comprised similarly large fractions. Assigned compound classes were more evenly distributed among satellite than core pool in both sediment and surface water samples (Figure 2).
We observed distinct differences in the relative frequency of compounds found in sediment and water samples. In sediments, compounds with the properties of lipids, tannins, carbohydrates, and condensed aromatics were less frequently observed in the core than in the in-between and satellite pools. For protein- and amino sugar-like compounds, we observed a decreasing trend in their frequency from core to satellite pool in sediment, while lignin remained steady. In water samples, we observed mostly the opposite pattern (Figure 2). The exceptions to the general observations were lignin-like and carbohydrate-like compounds that also increased in frequency from core to satellite, and condensed aromatic-like compounds that remained steady.
To further understand how core, in-between and satellite compounds differed between sediment and water, we examined trends in molecular mass, NOSC, DBE, and GFE. The overall trend in all of the examined molecular characteristics from core through in-between to satellite molecules were similar between sediment and water samples (Supplementary Figure S4). Molecular mass, NOSC, and DBE were increasing, while GFE was decreasing, from core to satellite pools. All observed changes from core to satellite were statistically significant for sediment. For water samples, the difference was statistically significant for the increasing trend in molecular mass, but not for DBE. For GFE and NOSC, a statistically significant difference was only found between core and satellite molecules (Supplementary Figure S4).
3.3. Differences and similarities among environments
Some molecular formulae were uniquely core, satellite or in-between in both sediment and water, whereas others occurred in both environments but with different occupancy (e.g., core in sediment and satellite in water). To identify differences and similarities with regard to core-satellite classification among the two environments, we identified seven cross-environment groups as described in the Method section. Most molecular formulae (n = 2,645) were classified as global satellite, while the lowest number of occurrences were observed in the global core (n = 666), global in-between (n = 543), and Sediment Core - Water Satellite/In-between (n = 524) (Supplementary Table S3).
Van Krevelen diagrams for the cross-environment groups (Figures 3A, B) indicated that global core molecules were grouped near the island of stability in the center indicating peptide-like and highly unsaturated compounds (Figure 3A). Global satellite molecules were more spread out across the whole range of H/C-to-O/C ratios representing the full range of compound classes. For the global in-between molecules, there seemed to be two clusters along the H/C range, namely peptide-like/unsaturated aliphatics and compounds with the properties of vascular plant-derived polyphenols and phenols, with the region between them representing highly unsaturated compounds and the island of stability being relatively sparsely occupied.
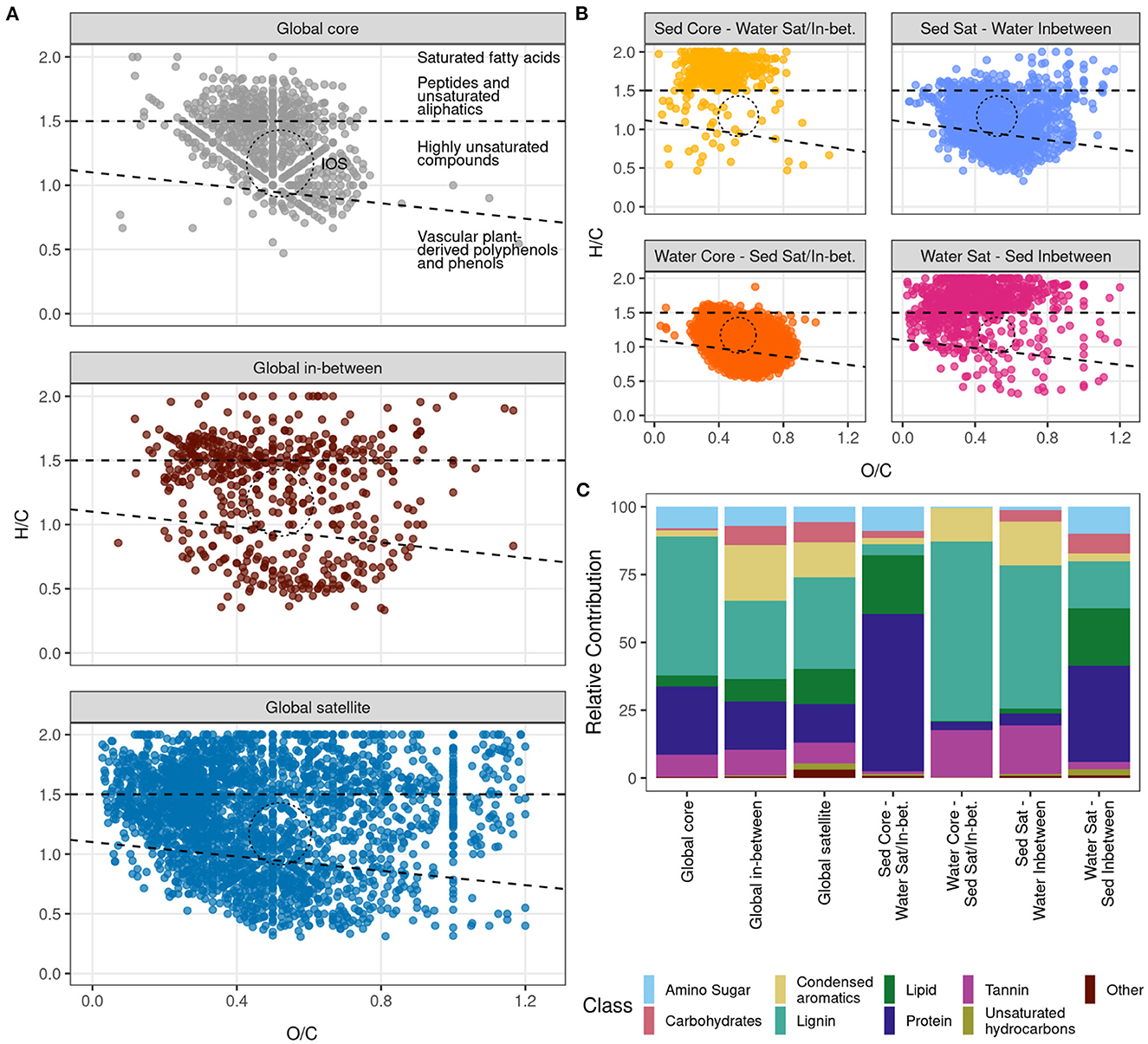
Figure 3. Cross-environmental groups identify common and different molecular formulae between sediment and surface water. (A) Van Krevelen diagram of molecular formulae with common occupancy across environments. (B) Van Krevelen diagram of molecular formulae that change occupancy dependent on environment. (C) Relative contribution of each cross-environmental group in terms of chemical classes. IOS = island of stability.
O/C and H/C ratios of molecular formulae that were designated to be core in surface water and less common (i.e., satellite or in-between) in sediments grouped tightly in the center around the island of stability similar to the global core molecules, indicating highly unsaturated compounds. Similarly, molecular formulae that were in-between in water but satellite in sediment clustered in the center but with a generally larger spread. In contrast, molecular formulae that were core in sediment and either satellite or in-between in surface water were largely found in the region of a high H/C of 1.5–2.0, and an O/C of 0.2–0.7, characteristic of saturated fatty acid-like, peptide-like and unsaturated aliphatic-like compounds. Molecular formulae that were in-between in sediment but satellite in water clustered in the same region but were generally more spread out, with more molecular formulae in the range of highly unsaturated and low-oxygen compounds. Sediment core—water satellite/in-between, and water satellite—sediment in-between groups mostly did not overlap with the island of stability (Figure 3B).
To further explore the characteristics of each cross-environment group, we examined the relative abundance of chemical compound classes within each of the identified seven cross-environmental groups (Figure 3C; Supplementary Figure S5: pie charts). The global core pool was characterized by lignin- and protein-like molecular formulae (51.2 and 25.2%, respectively). While the importance of these two chemical compound classes decreased from in-between to satellite molecules, condensed aromatics became more prevalent in the global in-between (20.4%) and satellite (13.0%) pools as compared to the global core (2.3%). Similarly, the contribution of lipids and carbohydrates was larger in the global in-between (8.3 and 13.1%) and satellite pools (6.9 and 7.4%) than in the global core (4.1 and 0.8%). A large proportion of the molecular formulae that were considered core in sediment but satellite or in-between in surface water were classified as protein-like (58.0%) and lipid-like (21.6%) compounds. In contrast, molecular formulae classified as core in water but satellite or in-between in sediment samples were identified to be mostly lignin-like (66.2%), and, to a smaller extent, tannin-like (17.3%) and condensed aromatic-like (12.4%) compounds. Molecular formulae that were satellite in sediment and in-between in water were characteristic of lignin (52.8%), followed by tannin-like (18.1%), and condensed aromatic-like (15.9%) compounds. Water satellite and sediment in-between molecular formulae were characterized by a higher proportion of protein-like (35.5%), followed by lipid-like (21.2%), and lignin-like (17.4%) compounds.
To statistically test the differences in the observed cross-environmental groups, a multivariate approach was used (PCoA; Supplementary Figure S5). The upper panel (Supplementary Figure S5a) depicts compounds that are core in at least one environment (sediment and/or water), whereas the bottom panel (Supplementary Figure S5b) considers molecular formulae that are classified as satellite in at least one environment. We refer to the upper and bottom panel as core and satellite perspectives, respectively. Both PCoA of core and satellite perspectives indicate a similar pattern, where the first two PCoA axes center around the global core and satellite, respectively. The axes then differentiate the two extremes of molecular formulae that switch pools from core to satellite and vice versa between the two studied environments. Collectively, the first two axes of the PCoAs explain 83.5 and 21.6% for core and satellite perspectives, respectively. Almost all pairwise comparisons showed statistically significant differences (Tukey's HSD), except when the molecular formulae that were satellite in sediment but core in water were compared with the molecular formulae that were core in sediment but satellite in water (Supplementary Table S4).
We further described each of our cross-environment groups by the molecular mass, NOSC, DBE, GFE, and AImod of their molecular formulae (Figure 4). Within the global groups, the global core was characterized by the lowest molecular mass (median ± standard deviation: 370.1 ± 78.4 m/z), NOSC (−0.43 ± 0.53) and DBE (6 ± 2.7), and highest AImod (0.17 ± 0.17) and GFE (72.5 ± 15.17 kJ/mol C). The global satellite had the highest molecular mass (493.09 ± 128.9) and DBE (8 ± 5.2), but lowest AImod (0.12 ± 0.2). The global satellite's NOSC was slightly higher (−0.34 ± 0.8) than that of the global core, and correspondingly, their GFE (70.2 ± 21.7 kJ/mol C) was slightly lower. The global in-between pool was, in general, between global core and satellite for all indices, except for their high NOSC (−0.18 ± 0.7) and corresponding low GFE (65.5 ± 18.9 kJ/mol C) in relation to the other two global groups.
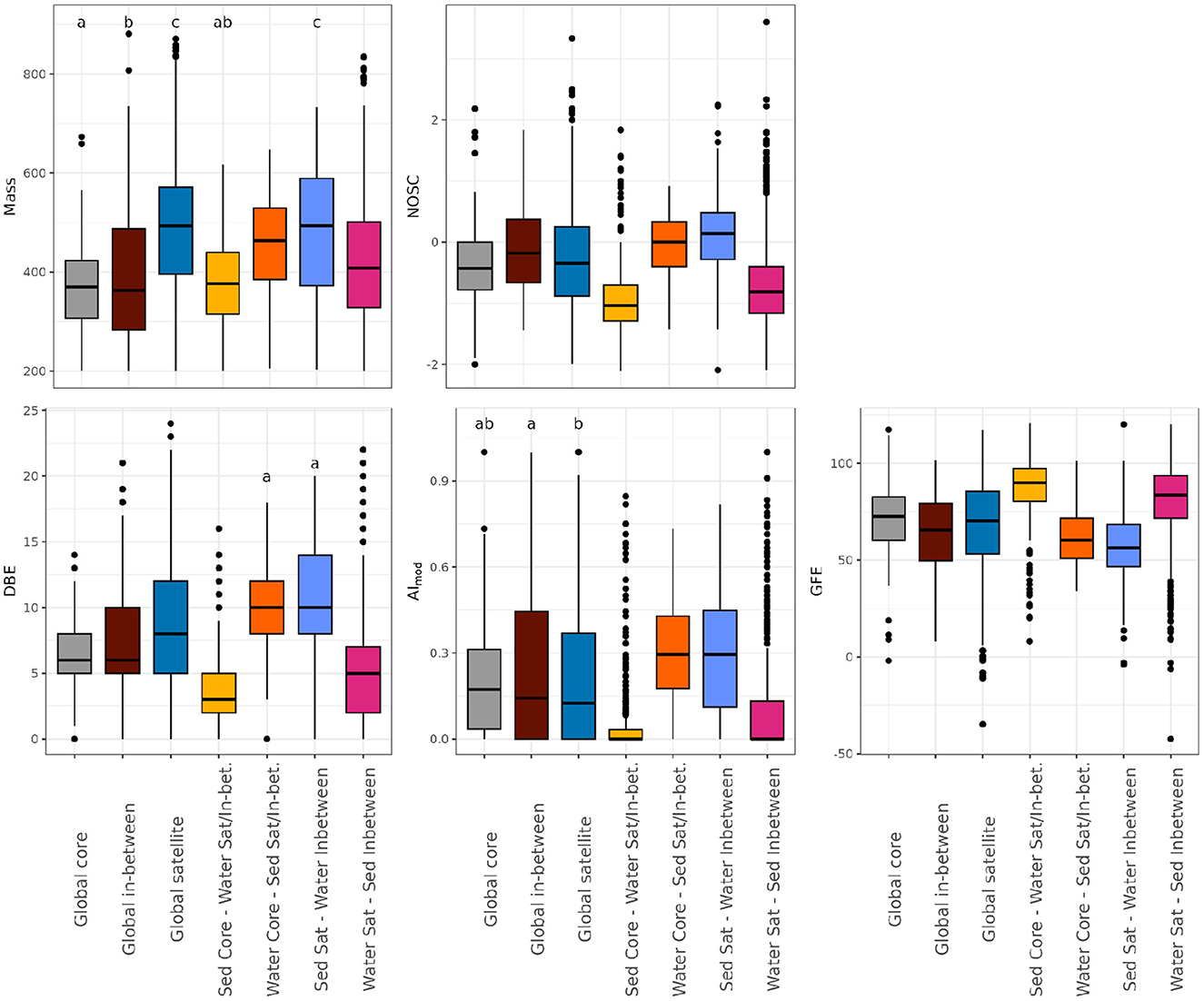
Figure 4. Chemical properties of dissolved organic matter (DOM)—molecular mass (m/z), nominal oxidation state of carbon (NOSC), double-bond equivalents (DBE), modified aromaticity index (AImod), and Gibbs Free energy (GFE; kJ/mol C) —in relation to cross-environment core/satellite-classification. The number of molecular formulae per category is as follows (see also Table S3): global core = 666, global in-between = 543, global satellite = 2,645, sediment core—water satellite/in-between = 524, water core—sediment satellite/in-between = 1,367, sediment satellite—water in-between = 1,151, and water satellite—sediment in-between = 881. Boxplots without identical letters were statistically different from each other (Kruskal-Wallis one-way analysis of variance followed by Dunn's test). Each boxplot's upper and lower hinges correspond to the first and third quartiles, respectively. The whiskers extend from the hinge to the largest and smallest value within 1.5 times the interquartile range. Data beyond whiskers are displayed as outlier points.
When comparing compounds that switch classes depending on the environment, a few coherent patterns emerged. For instance, molecular formulae that were core in sediment and less common in water (Figure 4, yellow) were consistently lowest in molecular mass (376.7 ± 86.7 m/z), NOSC (−1.1 ± 0.6), DBE (3 ± 2.5) and AImod (0 ± 0.1), and highest in GFE (89.9 ± 16.2 kJ/mol C). Molecular formulae that were in-between in sediment and satellite in water (Figure 4, pink) followed a similar pattern as those that were core in sediment and satellite/in-between in water (Figure 4, yellow), with some of the lowest values across indices (mass: 408.2 ± 124.5 m/z, NOSC: −0.8 ± 0.7, DBE: 5 ± 3.9, AImod: 0 ± 0.17, GFE: 83.6 ± 19.4 kJ/mol C). The two classes [sediment core—water satellite/in-between (yellow) vs. sediment in-between—water satellite (pink)] were statistically different from each other (Figure 4). Molecular formulae that were core in water and less common in sediment (Figure 4, orange) were similar to those that were satellite in sediment and in-between in water (Figure 4, pastel blue) across all indices (mass: 463.2 ± 95.7 vs. 493.2 ± 128.5, NOSC: 0.0 ± 0.5 vs. 0.1 ± 0.5, AImod: 0.3 ± 0.2 vs. 0.3 ± 0.2, GFE: 60.3 ± 13.6 vs. 56.4 ± 15.7). However, they remained statistically different from each other with the exception of DBE (10 ± 2.7 and 10 ± 4.3, Figure 4).
Overall, global and sediment satellites had the highest molecular mass among all cross-environment groups (dark and pastel blue, and orange in Figure 4). Highest NOSC, DBE, AImod, and molecular mass and lowest GFE were recorded for molecules that were either core in water, or satellite in sediment (orange and pastel blue in Figure 4). Molecular formulae that were core in sediment, or satellite in surface water, were generally low in NOSC, DBE, AImod, and molecular mass (yellow and pink in Figure 4).
To evaluate frequency at which a specific molecule could have been gained or lost during metabolism within each cross-environmental group, the mean number of putative transformations was identified (Figure 5). The number of mean transformations computed per molecular formula was highest for those in the global core (42.4 ± 28.2), and lowest for those in the global satellite pool (7.1 ± 9.1). Molecular formulae that were either core in either environment and satellite or in-between in the other (yellow and orange in Figure 5), or global in-between (brown in Figure 5), were characterized by having undergone a larger number of putative transformations than molecules in other cross-environment classes. Core formulae in sediments—satellite or in-between in water had a comparable number of mean transformations (21.2 ± 18.3 as core formulae in surface water—and satellite or in-between in sediment; 24.5 ± 20.8). Overall, core molecules had more putative transformations than satellite or in-between molecules in both environments.
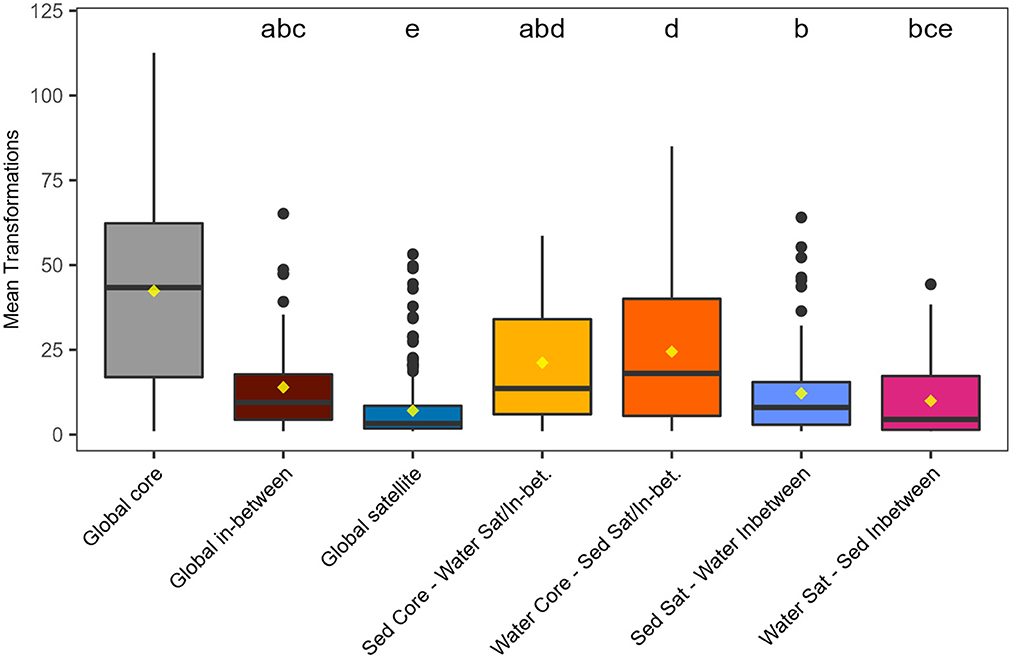
Figure 5. Mean number of putative transformations per molecular formula (see Methods). Horizontal lines indicate medians, the yellow dots indicate the mean. Boxplots without matching letters were statistically different from each other (Kruskal-Wallis one-way analyses of variance followed by Dunn's test). Upper and lower hinges correspond to the first and third quartiles, respectively. The whiskers extend to the largest and smallest value within 1.5 times the interquartile range. Data beyond whiskers are displayed as outlier points.
4. Discussion
Inspired by the ecological concept of core and satellite species, we analyzed the molecular characteristics of DOM from 97 river systems worldwide. Rather than separating rarely vs. commonly occurring molecular formulae using arbitrary thresholds, we used a novel data-driven approach based on occupancy patterns to classify molecular formulae. Our approach was largely consistent with other methods tested in this study, based on principal components and random forests. These data-driven approaches have the advantage that they avoid potential biases associated with arbitrary thresholds that can differ substantially between studies.
4.1. Chemical characteristics of core and satellite pools
Our analyses showed that the satellite compounds are represented by a wider chemical range of compounds than the core DOM. This can be seen in the broader H/C and O/C ranges of rare (satellite) molecular formulae compared to the more tightly grouped core compounds. This difference may reflect that core compounds are mainly produced through a few shared processes (e.g., biomass leaching, bio-/photo-degradation). In contrast, satellite compounds may originate or be produced from a broader range of unique allochthonous and autochthonous sources across the various biomes sampled, and may also derive from other events (e.g., precipitation, anthropogenic contamination). At the same time, as explored below, the core compounds may also represent the persistent components of DOM, resulting from specific sources or accumulation of highly processed DOM.
To gain insight into the controls on DOM composition and infer the reasons for the emergence of core and satellite species in sediments and surface waters, we assessed the chemical characteristics of molecular formulae. Core compounds generally appeared more stable, with intermediate H/C and O/C ratios that aligned with the island of stability, which had been found to be persistent across aquatic ecosystems (Lechtenfeld et al., 2014; Kellerman et al., 2018). Similarly, Sleighter et al. (2014) experimentally showed that recalcitrant DOM was commonly found at H/C and O/C ratios of 1–1.5 and 0.25–0.6, respectively. Satellite compounds tended, on average, to have a higher NOSC, DBE, aromaticity and molecular weight, potentially indicating greater lability (Roth et al., 2019). The higher NOSC also corresponds to lower Gibbs energies of oxidation (LaRowe and Van Cappellen, 2011), which energetically favors higher microbial activity, and thus may point to satellite DOM being used preferentially as an electron donor. According to the size-reactivity continuum (Amon and Benner, 1996), the lower molecular masses of core compounds may point to substrates that have effectively supported microbial metabolism in the past, while larger satellite compounds may be fresher and more labile. However, Roth et al. (2019) have demonstrated that the evolution of molecular masses during the reworking of plant-derived material is complex. Masses can decrease, increase, and then decrease during soil passage and comprise roughly constant pools of low, mid and high molecular masses (Roth et al., 2019). Additionally, aquatic primary production has also been found to produce polymeric organic molecules contributing to the high molecular mass pool (Fogg, 1977). Hence, molecular mass alone is not a direct indicator of lability, which highlights the importance of jointly assessing multiple indicators of DOM reactivity.
4.2. Processes shaping core and satellite compounds
Differences in compound class composition further hint to different source contributions and processing between core and satellite DOM.
4.2.1. Core
Core DOM generally had fewer lipid-like and more lignin-like compounds than satellite and in-between groupings, suggesting a greater contribution from terrestrial vegetation sources (Bianchi et al., 2004). Such prevalence of terrestrial compounds (lignin-like, tannin-like and condensed aromatic-like) was especially evident in the core and in-between pools of surface waters, which may arise through the dynamic hydrological connection that surface waters have to surrounding soils and vegetation (Aitkenhead-Peterson et al., 2003). Biogeochemical reworking (photo- and biodegradation) of terrestrial humic DOM could further explain the strong terrestrial signature in the core pool, especially in surface waters (Yamashita et al., 2008). For instance, ultraviolet radiation can transform larger macromolecules into low molecular weight compounds, with the remaining high molecular weight compounds being more resistant to further photodegradation (Opsahl and Benner, 1998), potentially contributing to the increased core/satellite ratio in surface water compared to sediment. Furthermore, biodegradation has been shown to produce humic-like compounds through microbial transformation of DOM (Ogawa et al., 2001; Fasching et al., 2014), and cell death may contribute to humification as well (Stadler et al., 2020). In agreement with the interpretation that the core pool of surface water is a result of extensive processing, DOM in surface water showed evidence of being more biologically degraded, e.g., more oxidized, as reflected in the higher O/C ratios in water than in sediment samples, and higher values of NOSC, which energetically favors higher microbial activity (Zhang et al., 2021).
The sediment core pool also had a prevalence of lignin-like compounds, which may be impacted by oxygen availability. Differences in redox conditions can affect microbial processing and hence the persistence of substances (Lau et al., 2018). For example, lignin degradation is stimulated by molecular oxygen (Kirk and Farrell, 1987), though lignin breakdown can also take place under anoxic conditions (e.g., Benner et al., 1984). While no in-situ oxygen data were collected in this effort, the observation that lignin-like compounds are prevalent in the sediment core pool may indicate reduced degradation due to lower oxygen concentration in river beds. However, the largest fraction within the sediment core was attributed to protein-like compounds. Sediments and water-sediment interfaces are considered to be environments of high microbial reworking (Boodoo et al., 2017). It is commonly assumed that protein-like compounds are readily consumed (Fellman et al., 2009). Hence, it is surprising to see that these protein-like compounds seem to be ubiquitous in sediments. Recently, high microbial activity in surficial sediments could result in an enrichment of protein-like components in porewaters (Zhou et al., 2022). It has also been shown that protein-like compounds are being adsorbed to sediments more readily than humic-like compounds (Shi et al., 2019), which may allow these protein-like compounds to evade degradation, and consequently accumulate and form a large proportion of the sediment core pool. Moreover, longer residence times in sediments may allow for the microbial degradation of more complex molecules (Catalán et al., 2016), lowering their occurrence in the sediment. The scarcity of protein-like compounds in the surface water core pool may be a result of shorter residence times, where more refractory compounds are consumed less, leading to a lower relative frequency of these more labile protein-like compounds.
4.2.2. Satellite
In contrast to the clear dominance of a few compound classes in the core pools, satellite compounds in both sediment and surface water environments exhibited a mixture of the various classes examined. Lignin-like compounds were moderately prevalent in both sediments and surface waters, in both core and satellite pools. Their presence in the satellite pool may reflect differences in vegetation across watersheds, as our dataset covers a range of biomes. Other common compound classes in both surface water and sediment satellite pools were lipid-like and carbohydrate-like molecules, which likely indicate the most fresh form of DOM that is unique to their producers (e.g., algae, microorganisms, and macrophytes). These compounds may be readily consumed and hence be rare in both core and in-between pools. The main difference between surface water and sediment satellite pools was the dominance of protein-like compounds in surface waters, which may be explained by the difference in the matrix of the two environments. Protein-like compounds are commonly assumed to be labile (Fellman et al., 2009), and hence, their dominance in the satellite pool is expected. As discussed earlier, adsorption of protein-like molecules may facilitate the accumulation of these compounds only in sediments as adsorption to suspended particles is negligible (Murray, 1973).
4.3. Cross-environment groupings
4.3.1. Global core and satellite compounds
Through the identification of compounds that were consistent in their groupings across sediments and surface waters (named “global”), a few patterns emerged that may link these compounds to common sources and/or processes across surface waters and sediments. Lignin-like and protein-like compounds were the most frequent in the global core pool. We interpret this to be at least partially due to those compounds being persistent depending on environmental conditions. The high frequency of lignin-like compounds across the dataset may not be surprising given the major influence of terrestrial organic matter on freshwaters. These compounds showed a combination of the highest number of putative transformations and relatively lower molecular mass, which may indicate that these compounds have been highly processed. Since lignin-like compounds are common in surface waters as well as sediments, a proportion of these compounds may have been already degraded, and recycled within the soil realm (Schmidt et al., 2011) before being introduced into freshwaters. Another process that can explain lignin-like compounds may be a sequence of photo- and biodegradation processes in the surface waters (Cory and Kling, 2018) and subsequent sedimentation. Additionally, the global core contains a large fraction of protein-like compounds, which make up only a small fraction of the compounds in the surface water core. Protein adsorption to sediments (Shi et al., 2019) was one of the processes that may explain the high frequency of protein-like compounds in sediments. However, evidence in soil research also indicates that nitrogen-containing molecules such as proteins are preserved in soils as nitrogen availability decreases with decomposition stage (Roth et al., 2019). Hence, protein-like compounds may be highly degraded, persistent products of microbial soil organic matter recycling (Roth et al., 2019) that are then transported into freshwaters (Hutchins et al., 2017).
The global satellite pool consists of an even distribution of compound classes, is characterized by relatively high molecular masses, and shows the least putative transformations among all pools. These results are in agreement with our earlier interpretation that satellite compounds may be freshly produced and many compounds observed in this category have not (yet) been extensively processed. Given that these satellite compounds were rare but present in both surface water and sediments, they may be produced by similar processes in their respective environments, or their rare presence in both environments indicates the highly connected nature of sediments and the surface water, such that the two environments rapidly exchange highly labile compounds before them being consumed within their respective environment of origin.
When focusing on the global in-between groups, two distinct clusters were evident in the Van Krevelen diagram. One cluster contained compounds that were mainly condensed aromatic-like, and another cluster consisted of lipid- and protein-like compounds. Their collective chemical properties (mass, NOSC, GFE, AImod, and DBE) and the number of putative transformations consistently showed values between those of the global core and global satellite pools. This potentially indicates that these in-between compounds were decomposition products that may have derived from the highly diverse pool of satellite compounds, and in the process of being transformed to core compounds.
4.3.2. Sediment-surface water interactions
Unlike compounds that are consistently common or rare across the two examined environments, molecular formulae that are more frequent in one and less frequent in the other may help us further understand processes that are different among surface waters and sediments.
Compounds that were more frequent in surface waters than in sediments were characterized by a high frequency of lignin-, tannin- and condensed aromatic-like signatures, with a relatively high mass, NOSC, DBE and aromaticity. These compounds were found to be rare in the sediment; hence, it is likely that they are a product of photodegradation, where part of them are respired (Hutchins et al., 2017) or continuously transformed (Cory and Kling, 2018). Those more frequent in sediments than in surface waters showed generally lower DBE, NOSC, aromaticity and mass, and had a strong signature of protein- and lipid-like compounds. River beds can host higher microbial biomass than the water column, likely leading to elevated levels of exudates and necromass (Nadell et al., 2009), which may contribute to the high lipid- and protein-like signature of the compounds common in riverine sediments but rare in surface waters. Such transformations at the interface between sediment and surface water can contribute to the differences in core and satellite compounds in these two environments. The degree of divergence in DOM composition between surface waters and sediments also depends on their exchange rates, which depend on the hydrological and geological setting. Thus, any changes in physical, biogeochemical or ecological characteristics at this interface can affect DOM composition (e.g., Wong and Williams, 2010).
4.4. Summary and implications
Our data-driven approach for identifying core and satellite species in riverine surface waters and sediments offers promise to help understand the DOM biogeochemistry in river corridors. Through our explorative work, we found that comparing the characteristics of different DOM pools offers insight into their potential environmental roles. These analyses may also point to the processes generating variability in DOM composition across large environmental gradients and highlight shared and unique biogeochemical processes that regulate the production, transformation, and consumption of DOM in aquatic ecosystems. Ultimately, our analysis showed that most of the DOM molecular formulae identified in any sample belong to a core group of compounds found in all samples, but that the entire DOM pool is hugely diversified by the unique contribution of satellite compounds. It is noteworthy, however, that our study only represents summer conditions and riverine samples, and hence does not account for network hydrology which can greatly impact freshwater DOM composition (Casas-Ruiz et al., 2020). Furthermore, our data does not resolve distinct isomers within each molecular formula. We encourage further studies to consider isomeric diversity that shed light on underlying biogeochemical processes (Osterholz et al., 2015). As core compounds are associated with a higher number of putative transformations than the less common satellite compounds, we expect that they may also exhibit a higher isomeric diversity, characteristic of more processed DOM (Zark and Dittmar, 2018).
With a wide range of riverine systems sampled at the continental scale, large variations in biome, climate, hydrology, and geology are expected to alter the relative composition of DOM in surface water (satellite in water). As compounds are transformed, the end products may become more similar and accumulate. Our analyses support that ubiquitous DOM may be more persistent than rare DOM molecules, which has important implications for carbon budgets and modeling. Specifically, our results suggest distinct commonly-present vs. actively-turned-over DOM pools. For this reason, observational studies of DOM—with individual samples capturing mainly core compounds—may overestimate the persistence of DOM and underestimate its reactivity. Furthermore, differences between core compounds found in sediment and surface water samples point to differences in biogeochemical processing in the two settings, with compounds of terrestrial origin dominant in both environments but protein-like compounds contributing disproportionately to the persistent pool in sediments. These findings highlight and challenge the traditional conception of nutrient-rich compounds to be highly reactive in any setting, and support recent findings of other studies (Kellerman et al., 2015; Roth et al., 2019). Literature is scarce on riverine DOM dynamics in both sediment and surface water, yet needed to deepen our understanding of the tight relationship between these environments. More generally, our findings suggest that core-satellite groupings identified through the data-driven approach we developed herein can aid in detecting and interpreting patterns of complex mixtures of DOM.
Data availability statement
Publicly available datasets were analyzed in this study. These data can be found here: The FT-ICR MS data and corresponding metadata analyzed for this study can be found in the ESS-DIVE under doi: 10.15485/1603775 and doi: 10.15485/1729719 [as described in Borton et al. (2022)]. The scripts used in this study can be found in https://github.com/WHONDRS-Crowdsourced-Manuscript-Effort/Topic1.
Author contributions
MS and CM organized the team effort. MS, MM, VG-C, KB, EM-B, EF, CM, AL, and AT analyzed the data. MS, CM, AL, AT, MB, VG-C, EM-B, CS, KB, MM, EF, and DD drafted parts of the manuscript. All authors discussed the outcomes of the workshop initiating this crowdsourced effort and conceptualized a first sketch of this manuscript. All authors reviewed and approved the final version of the manuscript.
Funding
CM and KB were supported by the US National Science Foundation under grant OCE-1832178 to CM. MS and MM were supported by the Natural Sciences and Engineering Research Council of Canada/Hydro-Québec Industrial Research Chair under grant 387312-14 to Paul del Giorgio. AT was supported by H2020 ERC Starting Grant 804673 sEEIngDOM and the Canada Research Chairs Program. EM-B was supported by the Coordenação de Aperfeiçoamento de Pessoal de Nível Superior, Brasil (CAPES), Finance Code 001. DD was supported in part by the Watershed Function Science Focus Area and ExaSheds projects at Lawrence Berkeley National Laboratory funded by the U.S. Department of Energy, Office of Science, and Biological and Environmental Research under Contract No. DE-AC02-05CH11231. VG-C was supported by the U.S. Department of Energy, Office of Science, Office of Biological and Environmental Research, Environmental System Science (ESS) Program. This contribution originates from the River Corridor Scientific Focus Area (SFA) project at Pacific Northwest National Laboratory (PNNL). Pacific Northwest National Laboratory is operated by Battelle Memorial Institute for the U.S. Department of Energy under Contract DE-AC05-76RL01830. EF was supported by the Gates Cambridge Scholarship (OPP1144).
Acknowledgments
We thank the WHONDRS consortium for facilitating generation of data used in this manuscript, including study design, crowdsourced sample collection, sample analysis, and public data publishing. We also thank the organizers and participants of the virtual crowdsourced workshop (Borton et al., 2022) where the initial scientific questions and hypotheses were developed.
Conflict of interest
The authors declare that the research was conducted in the absence of any commercial or financial relationships that could be construed as a potential conflict of interest.
Publisher's note
All claims expressed in this article are solely those of the authors and do not necessarily represent those of their affiliated organizations, or those of the publisher, the editors and the reviewers. Any product that may be evaluated in this article, or claim that may be made by its manufacturer, is not guaranteed or endorsed by the publisher.
Supplementary material
The Supplementary Material for this article can be found online at: https://www.frontiersin.org/articles/10.3389/frwa.2023.1156042/full#supplementary-material
References
Aitkenhead-Peterson, J. A., Mcdowell, W. H., and Neff, J. C. (2003). “Sources, production, and regulation of allochthonous dissolved organic matter inputs to surface waters,” in Aquatic Ecosystems, eds. S. E. G. Findlay, R. L. Sinsabaugh (New York, NY: Elsevier) 25–70. doi: 10.1016/B978-012256371-3/50003-2
Allan, J. D., Castillo, M. M., and Capps, K. A. (2021). “Stream Microbial Ecology,” in Stream Ecology, eds. J. D., Allan, M. M., Castillo, K. A. Capps (Cham, Springer) 225–245. doi: 10.1007/978-3-030-61286-3_8
Amon, R. M. W., and Benner, R. (1996). Bacterial utilization of different size classes of dissolved organic matter. Limnol. Oceanogr. 41, 41–51. doi: 10.4319/lo.1996.41.1.0041
Angel, M. V. (1984). “Detrital organic fluxes through pelagic ecosystems,” in Flows of energy and materials in marine ecosystems, ed. M. J. R. Fasham (Boston, MA: Springer) 475–516. doi: 10.1007/978-1-4757-0387-0_19
Arrieta, J. M., Mayol, E., Hansman, R. L., Herndl, G. J., Dittmar, T., and Duarte, C. M. (2015). Dilution limits dissolved organic carbon utilization in the deep ocean. Science 348, 331–333. doi: 10.1126/science.1258955
Azam, F. T., Fenchel, J. G., Field, J. S., Gray, L.-A., and Meyer-Reil Thingstad, F. (1983). The ecological role of water-column microbes in the sea. Marine Ecol. Progr. Ser. 10, 257–263. doi: 10.3354/meps010257
Bahureksa, W., Tfaily, M. M., Boiteau, R. M., et al. (2021). Soil organic matter characterization by fourier transform ion cyclotron resonance mass spectrometry (FTICR MS): A critical review of sample preparation, analysis, and data interpretation. Environ. Sci. Technol. 55, 9637–9656. doi: 10.1021/acs.est.1c01135
Barnes, C. J., Burns, C. A., van der Gast, C. J., McNamara, N. P., and Bending, G. D. (2016). Spatio-temporal variation of core and satellite arbuscular mycorrhizal fungus communities in Miscanthus giganteus. Front. Microbiol. 7, 1–12. doi: 10.3389/fmicb.2016.01278
Benner, R., Maccubbin, A. E., and Hodson, R. E. (1984). Anaerobic biodegradation of the lignin and polysaccharide components of lignocellulose and synthetic lignin by sediment microflora. Appl. Environ. Microbiol. 47, 998–1004. doi: 10.1128/aem.47.5.998-1004.1984
Besemer, K., Singer, G., Quince, C., Bertuzzo, E., Sloan, W., and Battin, T. J. (2013). Headwaters are critical reservoirs of microbial diversity for fluvial networks. Proc. R Soc. B. 280, 20131760. doi: 10.1098/rspb.2013.1760
Bianchi, T. S., Filley, T., Dria, K., and Hatcher, P. G. (2004). Temporal variability in sources of dissolved organic carbon in the lower Mississippi river. Geochim. Cosmoch. Acta 68, 959–967. doi: 10.1016/j.gca.2003.07.011
Boodoo, K. S., Fasching, C., and Battin, T. (2020). Sources, transformation and fate of dissolved organic matter in the gravel bar of a prealpine stream. J. Geophys. Res. Biogeosci. 125, e2019JG005604. doi: 10.1029/2019JG005604
Boodoo, K. S., Trauth, N., Schmidt, C., Schelker, J., and Battin, T. J. (2017). Gravel bars are sites of increased CO2 outgassing in stream corridors. Sci. Rep. 7, 14401. doi: 10.1038/s41598-017-14439-0
Borton, M. A., Collins, S. M., Graham, E. B., Garayburu-Caruso, V. A., Goldman, A. E., de Melo, M., et al. (2022). It takes a village: using a crowdsourced approach to investigate organic matter composition in global rivers through the lens of ecological theory. Front. Water 4, 870453. doi: 10.3389/frwa.2022.870453
Bramer, L. M., White, A. M., Stratton, K. G., Thompson, A. M., Claborne, D., Hofmockel, K., et al. (2020). ftmsRanalysis: An R package for exploratory data analysis and interactive visualization of FT-MS data. PLoS Comput. Biol. 16, e1007654. doi: 10.1371/journal.pcbi.1007654
Casas-Ruiz, J. P., Spencer, R. G. M., Guillemette, F., von Schiller, D., Obrador, B., Podgorski, D. C., et al. (2020). Delineating the continuum of dissolved organic matter in temperate river networks. Global Biogeochem. Cycl. 34, 1–15. doi: 10.1029/2019GB006495
Catalán, N., Marcé, R., Kothawala, D., and Tranvik, L. J. (2016). Organic carbon decomposition rates controlled by water retention time across inland waters. Nat. Geosci. 9, 501–504. doi: 10.1038/ngeo2720
Catalán, T. S., Shorte, S., and Dittmar, T. (2021). Marine dissolved organic matter: a vast and unexplored molecular space. Appl. Microbiol. Biotechnol. 105, 7225–7239. doi: 10.1007/s00253-021-11489-3
Cooper, W. T., Chanton, J. C., D'Andrilli, J., et al. (2022). A history of molecular level analysis of natural organic matter by FTICR mass spectrometry and the paradigm shift in organic geochemistry. Mass Spec. Rev. 41, 215–239. doi: 10.1002/mas.21663
Cory, R. M., and Kling, G. W. (2018). Interactions between sunlight and microorganisms influence dissolved organic matter degradation along the aquatic continuum. Limnol. Oceanogr. Lett. 3, 102–116. doi: 10.1002/lol2.10060
Danczak, R. E., Chu, R. K., Fansler, S. J., Goldman, A. E., Graham, E. B., Tfaily, M. M., et al. (2020). Using metacommunity ecology to understand environmental metabolomes. Nat. Commun. 11, 6369. doi: 10.1038/s41467-020-19989-y
Danczak, R. E., Goldman, A. E., Chu, R. K., Toyoda, J. G., Garayburu-Caruso, V. A., Tolić, N., et al. (2021). Ecological theory applied to environmental metabolomes reveals compositional divergence despite conserved molecular properties. Sci. Total Environ. 788, 147409. doi: 10.1016/j.scitotenv.2021.147409
Dittmar, T., Koch, B., Hertkorn, N., and Kattner, G. (2008). A simple and efficient method for the solid-phase extraction of dissolved organic matter (SPE-DOM) from seawater. Limnol. Oceanogr. Methods 6, 230–235. doi: 10.4319/lom.2008.6.230
Escalas, A., Paula, F. S., Guilhaumon, F., Yuan, M., Yang, Y., Wu, L., et al. (2022). Macroecological distributions of gene variants highlight the functional organization of soil microbial systems. ISME J. 16, 726–737. doi: 10.1038/s41396-021-01120-8
Fasching, C., Ulseth, A. J., Schelker, J., Steniczka, G., and Battin, T. J. (2016). Hydrology controls dissolved organic matter export and composition in an Alpine stream and its hyporheic zone. Limnol. Oceanogr. 61, 558–571. doi: 10.1002/lno.10232
Fasching, C. B., Behounek, G. A., and Singer Battin, T. J. (2014). Microbial degradation of terrigenous dissolved organic matter and potential consequences for carbon cycling in brown-water streams. Sci. Rep. 4, 1–7. doi: 10.1038/srep04981
Fellman, J. B., Hood, E., D'Amore, D. V., et al. (2009). Seasonal changes in the chemical quality and biodegradability of dissolved organic matter exported from soils to streams in coastal temperate rainforest watersheds. Biogeochemistry 95, 277–293. doi: 10.1007/s10533-009-9336-6
Fogg, G. E. (1977). Excretion of organic matter by phytoplankton. Limnol. Oceanogr. 22, 576–577. doi: 10.4319/lo.1977.22.3.0576
Fonte, L. F. M. D., Latombe, G., Gordo, M., Menin, M., de Almeida, A. P., Hui, C., et al. (2021). Amphibian diversity in the Amazonian floating meadows: a Hanski core-satellite species system. Ecography 44, 1325–1340. doi: 10.1111/ecog.05610
Garayburu-Caruso, V. A., Danczak, R. E., Stegen, J. C., Renteria, L., Mccall, M., Goldman, A. E., et al. (2020). Using community science to reveal the global chemogeography of river Metabolomes. Metabolites 10, 518. doi: 10.3390/metabo10120518
Gaston, K. J., Blackburn, T. M., Greenwood, J. J., Gregory, R. D., Quinn, R. M., and Lawton, J. H. (2000). Abundance–occupancy relationships. J. Appl. Ecol. 37, 39–59. doi: 10.1046/j.1365-2664.2000.00485.x
Graham, E. B., Crump, A. R., Kennedy, D. W., Arntzen, E., Fansler, S., Purvine, S. O., et al. (2018). Multi 'omics comparison reveals metabolome biochemistry, not microbiome composition or gene expression, corresponds to elevated biogeochemical function in the hyporheic zone. Sci. Total Environ. 642, 742–753. doi: 10.1016/j.scitotenv.2018.05.256
Graham, E. B., Tfaily, M. M., Crump, A. R., Goldman, A. E., Bramer, L. M., Arntzen, E., et al. (2017). Carbon inputs from riparian vegetation limit oxidation of physically bound organic carbon via biochemical and thermodynamic processes. J. Geophys. Res. 122, 3188–3205. doi: 10.1002/2017JG003967
Guillemette, F., McCallister, S. L., and Giorgio, P. A. (2016). Selective consumption and metabolic allocation of terrestrial and algal carbon determine allochthony in lake bacteria. ISME J. 10, 1373–1382. doi: 10.1038/ismej.2015.215
Hach, P. F., Marchant, H. K., Krupke, A., Riedel, T., Meier, D. V., Lavik, G., et al. (2020). Rapid microbial diversification of dissolved organic matter in oceanic surface waters leads to carbon sequestration. Sci. Rep. 10, 1–10. doi: 10.1038/s41598-020-69930-y
Hanski, I. (1982). Dynamics of regional distribution: the core and satellite species hypothesis. Oikos. 38, 210–221. doi: 10.2307/3544021
Hartley, S. (1998). A positive relationship between local abundance and regional occupancy is almost inevitable (but not all positive relationships are the same). J. Animal Ecol. 67, 992–994. doi: 10.1046/j.1365-2656.1998.6760992.x
Hassell, N., Tinker, K. A., Moore, T., and Ottesen, E. A. (2018). Temporal and spatial dynamics in microbial community composition within a temperate stream network. Environ. Microbiol. 20, 3560–3572. doi: 10.1111/1462-2920.14311
Hawkes, J. A., D'Andrilli, J., Agar, J. N., Barrow, M. P., Berg, S. M., Catalán, N., et al. (2020). An international laboratory comparison of dissolved organic matter composition by high resolution mass spectrometry: Are we getting the same answer? Limnol. Oceanogr. Methods 18, 235–258. doi: 10.1002/lom3.10364
Hertkorn, N., Benner, R., Frommberger, M., Schmitt-Kopplin, P., Witt, M., Kaiser, K., et al. (2006). Characterization of a major refractory component of marine dissolved organic matter. Geochim. Cosmoch Acta 70, 2990–3010. doi: 10.1016/j.gca.2006.03.021
Herzsprung, P., Hertkorn, N., Von Tumpling, W., Harir, M., Friese, K., and Schmitt-Kopplin, P. (2014). Understanding molecular formula assignment of Fourier transform ion cyclotron resonance mass spectrometry data of natural organic matter from a chemical point of view. Anal. Bioanal. Chem. 406, 7977–7987. doi: 10.1007/s00216-014-8249-y
Holm, S. (1979). A Simple Sequentially Rejective Multiple Test Procedure. Scandin. J. Stat. 6, 65–70.
Hu, A., Jang, K.-S., Meng, F., Stegen, J., Tanentzap, A. J., Choi, M., et al. (2022). Microbial and environmental processes shape the link between organic matter functional traits and composition. Environ. Sci. Technol. 56, 10504–10516. doi: 10.1021/acs.est.2c01432
Hugoni, M., Nunan, N., Thioulouse, J., Dubost, A., Abrouk, D., Martins, J. M., et al. (2021). Small-scale variability in bacterial community structure in different soil types. Microbial. Ecol. 82, 470–483. doi: 10.1007/s00248-020-01660-0
Hunter, J. (2007). Matplotlib: a 2D graphics environment. Comput. Sci. Eng. 9, 90–95. doi: 10.1109/MCSE.2007.55
Hutchins, R. H. S., Aukes, P., Schiff, S. L., Dittmar, T., Prairie, Y. T., and del Giorgio, P. A. (2017). The optical, chemical, and molecular dissolved organic matter succession along a boreal soil-stream-river continuum. J. Geophy. Res. Biogeosci. 122, 2892–2908. doi: 10.1002/2017JG004094
Jaffé, R., Yamashita, Y., Maie, N., Cooper, W. T., Dittmar, T., Dodds, W. K., et al. (2012). Dissolved organic matter in headwater streams: Compositional variability across climatic regions of North America. Geochim. Cosmoch. Acta 94, 95–108. doi: 10.1016/j.gca.2012.06.031
Jannasch, H. W. (1995). “The Microbial Turnover of Carbon in the Deep-Sea Environment,” in Direct Ocean Disposal of Carbon Dioxide, eds. N., Handa, T., Ohsumi (Tokyo: Terra Scientific Publishing Company) 1–11.
Kellerman, A., Dittmar, T., Kothawala, D., and Tranvik, L. J. (2014). Chemodiversity of dissolved organic matter in lakes driven by climate and hydrology. Nat. Commun. 5, 3804. doi: 10.1038/ncomms4804
Kellerman, A. M., Guillemette, F., Podgorski, D. C., Aiken, G. R., Butler, K. D., and Spencer, R. G. M. (2018). Unifying concepts linking dissolved organic matter composition to persistence in aquatic ecosystems. Environ. Sci. Technol. 52, 2538–2548. doi: 10.1021/acs.est.7b05513
Kellerman, A. M., Kothawala, D. N., Dittmar, T., and Tranvik, L. J. (2015). Persistence of dissolved organic matter in lakes related to its molecular characteristics. Nat. Geosci. 8, 454–459. doi: 10.1038/ngeo2440
Kim, S., Kramer, R. W., and Hatcher, P. G. (2003). Graphical method for analysis of ultrahigh-resolution broadband mass spectra of natural organic matter, the van Krevelen diagram. Anal Chem. 75, 5336–5344.
Kirk, T. K., and Farrell, R. (1987). Enzymatic “combustion”: the microbial degradation of lignin. Ann. Rev. Microbiol. 41, 465–501. doi: 10.1146/annurev.mi.41.100187.002341
Koch, B. P., and Dittmar, T. (2006). From mass to structure: an aromaticity index for high-resolution mass data of natural organic matter. Rapid Commun. Mass Spectrom. 20, 926–932. doi: 10.1002/rcm.2386
Koch, B. P., Witt, M., Engbrodt, R., Dittmar, T., and Kattner, G. (2005). Molecular formulae of marine and terrigenous dissolved organic matter detected by electrospray ionization Fourier transform ion cyclotron resonance mass spectrometry. Geochim. Cosmochim. Acta 69, 3299–3308. doi: 10.1016/j.gca.2005.02.027
Lapierre, J.-F., Guillemette, F., Berggren, M., and del Giorgio, P. A. (2013). Increases in terrestrially derived carbon stimulate organic carbon processing and CO2 emissions in boreal aquatic ecosystems. Nat. Commun. 4, 2972. doi: 10.1038/ncomms3972
LaRowe, D. E., and Van Cappellen, P. (2011). Degradation of natural organic matter: A thermodynamic analysis. Geoch. Cosmoch. Acta. 75, 2030–2042. doi: 10.1016/j.gca.2011.01.020
Lau, M. P., Niederdorfer, R., Sepulveda-Jauregui, A., and Hupfer, M. (2018). Synthesizing redox biogeochemistry at aquatic interfaces. Limnologica 68:59–70. doi: 10.1016/j.limno.2017.08.001
Lechtenfeld, O. J., Kattner, G., Flerus, R., McCallister, S. L., Schmitt-Kopplin, P., and Koch, B. P. (2014). Molecular transformation and degradation of refractory dissolved organic matter in the Atlantic and Southern Ocean. Geoch. Cosmoch. Acta 126, 321–337. doi: 10.1016/j.gca.2013.11.009
Lindh, M. V., Sjostedt, J., Ekstam, B., Casini, M., Lundin, D., Hugerth, L. W., et al. (2017). Metapopulation theory identifies biogeographical patterns among core and satellite marine bacteria scaling from tens to thousands of kilometers. Environ. Microbiol. 19, 1222–1236. doi: 10.1111/1462-2920.13650
Ling, F., Hwang, C., LeChevallier, M. W., Andersen, G. L., and Liu, W.-T. (2015). Core-satellite populations and seasonality of water meter biofilms in a metropolitan drinking water distribution system. ISME J. 10, 582–595. doi: 10.1038/ismej.2015.136
Linkhorst, A., Dittmar, T., and Waska, H. (2017). Molecular fractionation of dissolved organic matter in a shallow subterranean estuary: the role of the iron curtain. Environ. Sci. Technol. 51, 1312–1320. doi: 10.1021/acs.est.6b03608
MacDonald, E. N., Tank, S. E., Kokelj, S. V., Froese, D. G., and Hutchins, R. H. (2021). Permafrost-derived dissolved organic matter composition varies across permafrost end-members in the western Canadian Arctic. Environ. Res. Lett. 16, 024036. doi: 10.1088/1748-9326/abd971
Mateus-Barros, E., de Melo, M. L., Bagatini, I. L., Caliman, A., and Sarmento, H. (2021). Local and geographic factors shape the occupancy-frequency distribution of freshwater bacteria. Microb. Ecol. 81, 26–35. doi: 10.1007/s00248-020-01560-3
Mehranvar, L., and Jackson, D. A. (2001). History and taxonomy: their roles in the core-satellite hypothesis. Oecologia 127, 131–142. doi: 10.1007/s004420000574
Murray, A. P. (1973). Protein adsorption by suspended sediments: Effects of pH, temperature, and concentration. Environ. Pollut. 4, 301–312. doi: 10.1016/0013-9327(73)90097-9
Nadell, C. D., Xavier, J. B., and Foster, K. R. (2009). The sociobiology of biofilms. FEMS Microbiol. Rev. 33, 206–224. doi: 10.1111/j.1574-6976.2008.00150.x
Ogawa, H., Amagai, Y., Koike, I., Kaiser, K., and Ronald, B. (2001). Production of refractory dissolved organic matter by bacteria. Science 292, 917–920. doi: 10.1126/science.1057627
Ogle, D. H., Doll, J. C., Wheeler, P., and Dinno, A. (2022). FSA: Fisheries Stock Analysis. R package version 0.9.3.
Oksanen, J., Blanchet, F. G., Kindt, R., Legendre, P., Minchin, P. R., O'hara, R. B., et al. (2016). “Vegan: community ecology package,” in R package version. 321–6.
Opsahl, S., and Benner, R. (1998). Photochemical reactivity of dissolved lignin in river and ocean waters. Limnol. Oceanogr. 43, 1297–1304. doi: 10.4319/lo.1998.43.6.1297
Osterholz, H., Niggemann, J., Giebel, H.-A., Simon, M., and Dittmar, T. (2015). Inefficient microbial production of refractory dissolved organic matter in the ocean. Nat. Commun. 6, 1–8. doi: 10.1038/ncomms8422
Qi, Y., Xie, Q., Wang, J. J., He, D., Bao, H., Fu, Q. L., et al. (2022). Deciphering dissolved organic matter by Fourier transform ion cyclotron resonance mass spectrometry (FT-ICR MS): from bulk to fractions and individuals. Carbon Res. 1, 3. doi: 10.1007/s44246-022-00002-8
R Core Team (2022). R: A language and environment for statistical computing. R Foundation for Statistical Computing, Vienna, Austria. version R 4.2.2.
Regnier, P., Resplandy, L., Najjar, R. G., and Ciais, P. (2022). The land-to-ocean loops of the global carbon cycle. Nature 603, 401–410. doi: 10.1038/s41586-021-04339-9
Riedel, T., and Dittmar, T. (2014). A method detection limit for the analysis of natural organic matter via Fourier transform ion cyclotron resonance mass spectrometry. Anal. Chem. 86, 8376–8382. doi: 10.1021/ac501946m
Riedel, T., Zak, D., Biester, H., and Dittmar, T. (2013). Iron traps terrestrially derived dissolved organic matter at redox interfaces. Proc. Nat. Acad. Sci. 110, 10101–10105. doi: 10.1073/pnas.1221487110
Roth, V. N., Dittmar, T., Gaupp, R., and Gleixner, G. (2014). Ecosystem-specific composition of dissolved organic matter. Vadose Zone J. 13, 1–10. doi: 10.2136/vzj2013.09.0162
Roth, V. N., Lange, M., Simon, C., Hertkorn, N., Bucher, S., Goodall, T., et al. (2019). Persistence of dissolved organic matter explained by molecular changes during its passage through soil. Nat. Geosci. 12, 755–776. doi: 10.1038/s41561-019-0417-4
RStudio Team (2022). RStudio: Integrated Development Environment for R.PBC, Boston, MA, RStudio. version RStudio 2022.7.1.554.
Schmidt, M. W. I., Torn, M. S., Abiven, S., Dittmar, T., Guggenberger, G., Janssens, I. A., et al. (2011). Persistence of soil organic matter as an ecosystem property. Nature 478, 49–56. doi: 10.1038/nature10386
Seidel, M., Yager, P. L., Ward, N. D., Carpenter, E. J., Gomes, H. R., Krusche, A. V., et al. (2015). Molecular-level changes of dissolved organic matter along the Amazon River-to-ocean continuum. Marine Chem. 177, 218–231. doi: 10.1016/j.marchem.2015.06.019
Shi, W., Peng, H., Wu, J., Wu, M., Li, D., Xie, W., et al. (2019). Adsorption of soluble microbial products by sediments. Ecotoxicol. Environ. Safety 169, 874–880 doi: 10.1016/j.ecoenv.2018.11.005
Sleighter, R. L., Cory, R. M., Kaplan, L. A., Abdulla, H. A. N., and Hatcher, P. G. (2014). A coupled geochemical and biogeochemical approach to characterize the bioreactivity of dissolved organic matter from a headwater stream. J. Geophys. Res. Biogeosci. 119, 1520–1537, doi: 10.1002/2013JG002600
Stadler, M., and del Giorgio, P. A. (2022). Terrestrial connectivity, upstream aquatic history and seasonality shape bacterial community assembly within a large boreal aquatic network. ISME J. 16, 937–947. doi: 10.1038/s41396-021-01146-y
Stadler, M., Ejarque, E., and Kainz, M. J. (2020). In-lake transformations of dissolved organic matter composition in a subalpine lake do not change its biodegradability. Limnol. Oceanogr. 65, 1554–1572. doi: 10.1002/lno.11406
Stegen, J. C., Johnson, T., Fredrickson, J. K., Wilkins, M. J., Konopka, A. E., Nelson, W. C., et al. (2018). Influences of organic carbon speciation on hyporheic corridor biogeochemistry and microbial ecology. Nat. Commun. 9, 585. doi: 10.1038/s41467-018-02922-9
Tfaily, M. M., Chu, R. K., Toyoda, J., Tolić, N., Robinson, E. W., Paša-Tolić, L., et al. (2017). Sequential extraction protocol for organic matter from soils and sediments using high resolution mass spectrometry. Anal. Chim. Acta. 972, 54–61. doi: 10.1016/j.aca.2017.03.031
Tolić, N., Liu, Y., Liyu, A., Shen, Y., Tfaily, M. M., Kujawinski, E. B., et al. (2017). Formularity: Software for automated formula assignment of natural and other organic matter from ultrahigh-resolution mass spectra. Analyt. Chem. 89, 12659–12665. doi: 10.1021/acs.analchem.7b03318
Turner, J. T. (2002). Zooplankton fecal pellets, marine snow and sinking phytoplankton blooms. Aquatic Microbial Ecol. 27, 57–102. doi: 10.3354/ame027057
Virtanen, P., Gommers, R., Oliphant, T. E., Haberland, M., Reddy, T., Cournapeau, D., et al. (2020). SciPy 1.0: Fundamental algorithms for scientific computing in python. Nat. Methods 17, 261–272. doi: 10.1038/s41592-019-0686-2
Wagner, S., Riedel, T., Niggemann, J., Vähätalo, A. V., Dittmar, T., and Jaffe, R. (2015). Linking the molecular signature of heteroatomic dissolved organic matter to watershed characteristics in world rivers. Environ. Sci. Technol. 49, 23, 13798–13806. doi: 10.1021/acs.est.5b00525
Wickham, H. (2016). ggplot2: Elegant Graphics for Data Analysis. New York: Springer-Verlag. doi: 10.1007/978-3-319-24277-4
Wong, J. C. Y., and Williams, D. D. (2010). Sources and seasonal patterns of dissolved organic matter (DOM) in the hyporheic zone. Hydrobiologia 647, 99–111. doi: 10.1007/s10750-009-9950-2
Wu, L., Ning, D., Zhang, B., Li, Y., Zhang, P., Shan, X., et al. (2019). Global diversity and biogeography of bacterial communities in wastewater treatment plants. Nat. Microbiol. 4, 1183–1195. doi: 10.1038/s41564-019-0426-5
Yamashita, Y., Jaff,é, R., Maie, N., and Tanoue, E. (2008). Assessing the dynamics of dissolved organic matter (DOM) in coastal environments by excitation emission matrix fluorescence and parallel factor analysis (EEM-PARAFAC). Limnol. Oceanogr. 53, 1900–1908. doi: 10.4319/lo.2008.53.5.1900
Zark, M., and Dittmar, T. (2018). Universal molecular structures in natural dissolved organic matter. Nat. Commun. 9, 1–8. doi: 10.1038/s41467-018-05665-9
Zhang, G., Li, B., Guo, F., Liu, J., Luan, M., Liu, Y., et al. (2019). Taxonomic relatedness and environmental pressure synergistically drive the primary succession of biofilm microbial communities in reclaimed wastewater distribution systems. Environ. Int. 124, 25–37. doi: 10.1016/j.envint.2018.12.040
Zhang, J., Feng, Y., Wu, M., Chen, R., Li, Z., Lin, X., et al. (2021). Evaluation of microbe-driven soil organic matter quantity and quality by thermodynamic theory. mBio 12, e03252–e03220. doi: 10.1128/mBio.03252-20
Keywords: DOM (dissolved organic matter), FT-ICR-MS, rivers, sediment, surface water, high-resolution mass spectrometry (HRMS)
Citation: Stadler M, Barnard MA, Bice K, de Melo ML, Dwivedi D, Freeman EC, Garayburu-Caruso VA, Linkhorst A, Mateus-Barros E, Shi C, Tanentzap AJ and Meile C (2023) Applying the core-satellite species concept: Characteristics of rare and common riverine dissolved organic matter. Front. Water 5:1156042. doi: 10.3389/frwa.2023.1156042
Received: 01 February 2023; Accepted: 13 March 2023;
Published: 30 March 2023.
Edited by:
Zhifeng Yan, Tianjin University, ChinaReviewed by:
Yulin Qi, Tianjin University, ChinaYuanbi Yi, Hong Kong University of Science and Technology, Hong Kong SAR, China
Copyright © 2023 Stadler, Barnard, Bice, de Melo, Dwivedi, Freeman, Garayburu-Caruso, Linkhorst, Mateus-Barros, Shi, Tanentzap and Meile. This is an open-access article distributed under the terms of the Creative Commons Attribution License (CC BY). The use, distribution or reproduction in other forums is permitted, provided the original author(s) and the copyright owner(s) are credited and that the original publication in this journal is cited, in accordance with accepted academic practice. No use, distribution or reproduction is permitted which does not comply with these terms.
*Correspondence: Masumi Stadler, m.stadler.jp.at@gmail.com; Christof Meile, cmeile@uga.edu