- Hydrology and Quantitative Water Management, Wageningen University, Wageningen, Netherlands
Hydrological models play a key role in contemporary hydrological scientific research, but the social practices surrounding the use of these models receive little attention. This study focuses on the recruitment process for scientific positions in which models are used, to understand the implications for model development. Over 400 scientific hydrological vacancies were analyzed, to evaluate whether the job description already prescribed which model must be used, and whether experience with a specific model was an asset. Of the analyzed job positions, 76% involved at least some modeling. Of the PhD positions that involved any modeling, the model is already prescribed in the vacancy text in 17% of the cases, for postdoc positions this was 30%. A small questionnaire revealed that also beyond the vacancies where the model is already prescribed, in many Early-Career Scientist (ECSs) projects the model to be used is pre-determined and, actually, also often used without further discussion. There are valid reasons to pre-determine the model in these projects, but at the same time, this can have long-term consequences for the ECS. An ECS develops a “Modeling Toolkit”, a toolkit that contains all the models where the ECS has experience with. This toolkit influences the research identity the ECS develops, and influences future opportunities of the ECS—it might be strategic to gain experience with popular, broadly used models, or to become part of an efficient modeling team. This serves an instrumental vision on modeling and maintains the status quo. Seeing models as hypotheses calls for a more critical evaluation. ECSs learn the current rules of the game, but should at the same time actively be stimulated to critically question these rules.
1. Introduction
The use of numerical models is popular in the field of hydrology, as in many other fields in the Earth and environmental sciences. Models can be used as frameworks to formulate and test hypotheses, and to make predictions for practical applications, as for instance demanded by policy makers (Beven, 2002). Demonstrated by an increasing number of scientific publications in which models are employed (Burt and McDonnell, 2015; Addor and Melsen, 2019), numerical models are a generally accepted scientific method within the hydrological sciences.
The process of developing numerical models, as simplified representations of reality, is guided by several distinct steps (Beven, 2012; Knoben et al., 2019; Jansen et al., 2021). First, a perceptual, mental, model is developed by the expert. Subsequently, the perceptual model is translated into a conceptual model, leading to a schematization of the relevant storages and fluxes. Equations are assigned to the different components, leading to the mathematical model. The procedural model involves the translation of the mathematical model to computer code, generally with the use of numerical techniques to solve the differential equations. Finally, once the model code is running on a computer, sensitivity analysis, calibration, and model evaluation can be performed.
Modeling is often described as an art (Savenije, 2009), because it requires imagination, inspiration, and creativity. Formal procedures have been developed to support and evaluate the artistic process of model building for each of the model steps, for example in Clark et al. (2008, 2015), Gupta et al. (2012), and Gharari et al. (2021). The steps of defining the perceptual, conceptual, mathematical and procedural model are in practice, however, often simply replaced by selecting an already existing hydrological model.
This moves the decision from defining the model from scratch to selecting the right model. A plethora of hydrological models are available to choose from, and several criteria have been defined to select the most appropriate model for a set target (Jakeman et al., 2006; Boorman et al., 2007; Höge et al., 2018; Sjastad Hagen et al., 2020). But again in practice, it was found that institutes or research teams generally have a preference for a particular model, and that this model is used for the majority of the research in that team (Addor and Melsen, 2019).
This also became apparent from interviews held with modelers (Melsen, 2022). One PhD candidate stated:
“When I applied for the PhD it was a call from this team. And this team was working on [model] so it was more like, for me it was an opportunity to explore an already established model.”
Another interviewee in this study, a postdoctoral researcher, specifically approached a research team, because this modeler appreciated the model configuration that was used there:
“I had ideas to implement specific features in coarse scale global models. I contacted [person], I wrote a proposal and finally it worked out that the model is now much more realistic over these landscapes. [...] I was motivated by the fact that this model is operationally used for the soil moisture product from the [satellite] mission.”
These responses demonstrate how much model selection in practice deviates from the theoretical “ideal”. Rather than starting with defining a perceptual model, a researcher is often confronted with a prescribed model or moves to a place where a specific model is used. Most personnel changes in scientific teams occur for Early Career Scientist (ECS) positions: PhD candidates and postdoctoral researchers. The (model)experience that ECSs gain in these positions play an essential part in their scientific development (Babel et al., 2019). This triggered the following question: What is the role of recruitment for ECS in hydrological modeling? How often is the model prescribed in job advertisements? And what does this mean for model development and use?
To answer these questions, vacancy texts for scientific positions in the field of hydrological modeling were analyzed to investigate how often vacancy texts already prescribe which model will be used. A small questionnaire was developed to put the results of this analysis into context. Reflection on these results and the implications for model development and use lead to the introduction of the term “Modeling Toolkit”: researchers fill up their toolkit with models with which they have experience. This toolkit influences future opportunities and the development of a research identity.
2. Methods
The goal of this study was to reflect on recruitment practices for hydrological modeling, by determining how often vacancy texts already prescribe which model must be used. Therefore, databases were sought that provide an overview of vacancies in hydrology. A drawback is that most online vacancy texts can no longer be accessed once the position is filled. Therefore, several websites that advertise academic positions were contacted (amongst others academicpositions.com, globalacademyjobs.com, academictransfer.com). Only AcademicTransfer, a website hosting academic positions for Dutch universities, was willing to share their database. Since this database is heavily biased toward Dutch positions only, the methods and results of the analysis of this database are presented in Supplementary material 2. A more informal circuit where vacancies are shared is the About Hydrology mailing list. This is an informal Google group, established in 2013 by professor Rigon from the university of Trento. Everyone can sign up for the mailing list, and share announcements relevant for the hydrological community. Announcements are moderated by the initiator and colleagues before they are forwarded to the whole group, which currently contains about 5,000 subscribers. All emails sent through this mailing list between 2013 and 2020 have been screened for vacancies and a database was built based on that. Most announcements apply to positions in Europe and the US, which means that also this database is geographically biased. Since 2% of the About Hydrology vacancies were based in the Netherlands, there might be some overlap with the vacancies in the AcademicTransfer database. The final About Hydrology database consists of copied emails from the mailing list, and separate pdf and Word files that were sent as attachment. To further guide the interpretation of the analysis of the About Hydrology database, a short questionnaire was sent out to a stratified random sample of persons that announced a vacancy through the mailing list.
2.1. Vacancies in the About Hydrology mailing list
All the announcements in the About Hydrology Google group, since it was established on 15 June 2013 until 31 December 2020 (6.5 years), were scanned and all job announcement were stored. In a second screening, the job announcements were further filtered: Only job announcement related to academic positions were considered (PhD, post-doc, lecturer, assistant / associate / full professor), and only the announcements that contained a substantial part of the job description in the email, or which had the full job description in the attachment, were considered. This excluded a substantial part of the job announcements: many were very short and contained a meanwhile outdated link to an external website. Also very short job descriptions with reference to a contact person, projects that could both be an MSc or PhD project, and scholarship opportunities without predefined specified goals were excluded. Furthermore, duplicates were removed. Finally, 409 vacancies were maintained that were manually analyzed. Each of the 409 vacancies was read to determine:
1. Country where the position was based.
2. For which position the vacancy was (PhD, postdoc, lecturer, assistant / associate professor, full professor). It was often encountered that tenure track positions were offered which, dependent on the final selected applicant, would be at the assistant or associate professor level. Therefore, these two positions were merged into one category called “Tenure (track)”.
3. Whether the job involved any modeling or simulations. Three categories were defined: no modeling or simulations involved, partly modeling or simulations involved, or purely modeling and/or simulating. This step required interpretation, which was guided by the background of the author, who has experience in hydrological modeling. For example, a vacancy about downscaling techniques of climate data and subsequent hydrological modeling was defined as purely modeling because downscaling can be seen as data preparation for the hydrological model. Furthermore, there is interpretation in what can be called “modeling”. This analysis only focused around numerical models, that explicitly aim to describe the processes in the system. Therefore, other important modeling approaches such as machine learning and statistical models were not considered as modeling in the context of this study. A vacancy that only involved machine learning would therefore be classified as “no modeling or simulations involved”, which is only true for the definition of modeling as adopted here.
4. If any numerical modeling was involved in the job, whether a model name was mentioned in the vacancy main text, and if yes, which model.
5. If any numerical modeling was involved in the job, whether a model name was mentioned in the requirements or relevant experience section, and if yes, which model.
This analysis provided an overview of the percentage research vacancies that involve modeling, how often the model is already prescribed in the vacancy text, and how often specific experience with a model is considered an asset.
2.2. Questionnaire
To further guide the interpretation of these results, a short questionnaire was sent out to a stratified random sample of people that announced vacancies through the About Hydrology mailing list. The sample was stratified into three groups: (1) a model was mentioned in the vacancy text, (2) certain experience with a model was mentioned as an asset, and (3) the job included modeling but no model or specific model experience was mentioned. Although the stratified samples were initially drawn randomly from the database, they have been adapted to not approach the same person twice, in order to minimize the efforts for the respondents to optimize response rates. The questionnaire was sent through email to the contact person of the vacancy. After 2 weeks, if applicable, a reminder was sent.
Supplementary Table 1 gives an overview of the questions that were asked. In the case that a model was prescribed in the vacancy, the questions were designed to investigate if this model was indeed used during the project. When certain experience was mentioned as an asset, questions were asked to determine if this was also the model that was planned to be used during the project, and if this model was indeed used. In case no model or specific model experience was mentioned, it was asked whether the hiring person already had a model in mind for the project, and if this model was indeed used. All questions were tailored and personalized based on the considered vacancy. The final question was if the respondents were willing to share the contact details of the hired candidate, or could forward the questionnaire to the hired candidate. As such, it was possible to ask the same questions to the hired candidate, plus some additional questions about how their perception of models and their careers took shape during or after the project.
Table 1 shows the number of questionnaires that were sent out and the obtained response rates. From all the vacancies for PhD and postdoc positions that involved at least some modeling, 16% was approached to fill out the questionnaire (with strong variations among the three classes that were sampled in a stratified manner). The final usable response rate compared to this total was 8%, again with strong variations among the stratified sample. The response rate relative to the number of sent out questionnaires was 47%. Given that it was actively avoided to send the same questionnaire twice to the same person for two different vacancies, a relatively large share of different institutes was covered. Response rates are, however, on the lower end, and combined with the relatively small sample of questionnaires sent out, the responses cannot be considered representative. Nevertheless, the responses can provide context to the results that were found based on analyzing the About Hydrology vacancy database.
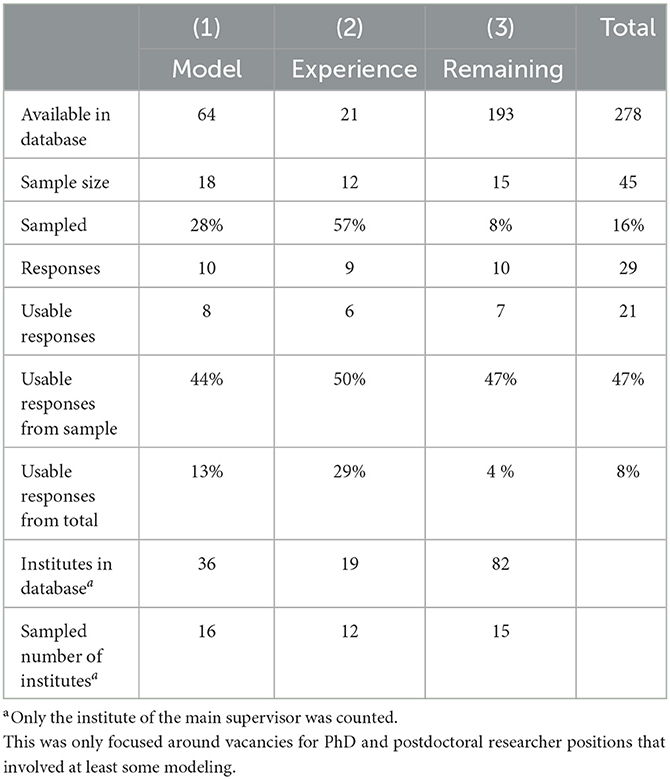
Table 1. Overview of the (relative) sample size to which the questionnaire was sent, and the obtained response rates for the stratified samples: (1) is the group where models were prescribed in the vacancy, (2) where experience with a certain model was mentioned as an asset, and (3) where no specific model or experience was mentioned.
Classified as “usable responses” are those that answered at least some of the questions from the questionnaire. In one case, the approached person preferred to have a meeting and the questionnaire was used in the context of a semi-structured interview. The responses that were discarded for instance included that this person did not manage to find a suitable candidate for this project, that the project was not funded in the end, and in one case that the project did not involve a numerical model (only statistical modeling). This latter example indicates that this project was miss-classified as a modeling project during the construction and interpretation of the About Hydrology database.
The number of respondents that provided contact details from the hired candidate or that forwarded the questionnaire to the hired candidate was substantially lower than the number of usable responses. Contact details for four hired candidates for group 1 (model prescribed), four hired candidates for group 2 (experience prescribed) and one hired candidates for group 3 (no model or experience prescribed) were provided—nine contacts in total. The response among this group, however, was maximal: 100% of the approached hired candidates responded and answered the questions. This group is, however, highly biased toward successful researchers, since many respondents indicated that they were reluctant to provide contact details of the hired candidate in case the project did not go well.
3. Results and discussion
In this section, first the results from the analysis on vacancy texts in the About Hydrology database are presented. Subsequently, the results from the questionnaire are used to provide further context. The results obtained from the AcademicTransfer database, which is highly biased toward Dutch vacancies, are presented in Supplementary material 2.
3.1. Models mentioned in the About Hydrology vacancies
In total, 409 vacancies that were shared through the About Hydrology mailing list between 2013 and 2020 were manually analyzed. The vacancies applied to positions in 23 different countries. Most positions were based in the United States (34%), followed by Germany (8%), Canada (8%), France (7%) and the UK (7%), Italy (6%), and Switzerland (5%). Of the evaluated vacancies, 50% were PhD positions, 37% postdoc positions, 2% lecturer positions, 9% assistant professor and tenure track positions, and 2% professorships.
The vast majority of the vacancies (76%) involves at least some modeling or simulation work (as defined for this study, see Section 2), 43% of the vacancies were classified as purely modeling vacancies. This is very well in line with the numbers found in the bibliometric analysis of Burt and McDonnell (2015), where about 75% of the hydrological scientific publications in 2010 related to runoff were model-based. For the AcademicTransfer database, 69% of the vacancies was related to modeling (Supplementary material 2), which is also in the same order. However, to what extent modeling or simulating is actually the activity that has to be carried out, depends on the position. For tenure track and professor positions, modeling is often mentioned as one of the research themes that the applicant could cover, but that does not imply that the finally hired candidate will actually carry out modeling or simulation work themselves, while for PhD and postdoc positions, the modeling and simulation activities were sometimes quite explicitly described (examples will be provided below). The vacancies that do not include modeling or simulation work often involve laboratory work, field work and/or data analysis of historical data sets. The vacancies that are purely based on modeling and/or simulations for instance relate to model development or setting up a data assimilation framework. An overview of the percentage of vacancies that involve modeling and simulating, divided across different scientific positions, is depicted in the top left panel of Figure 1.
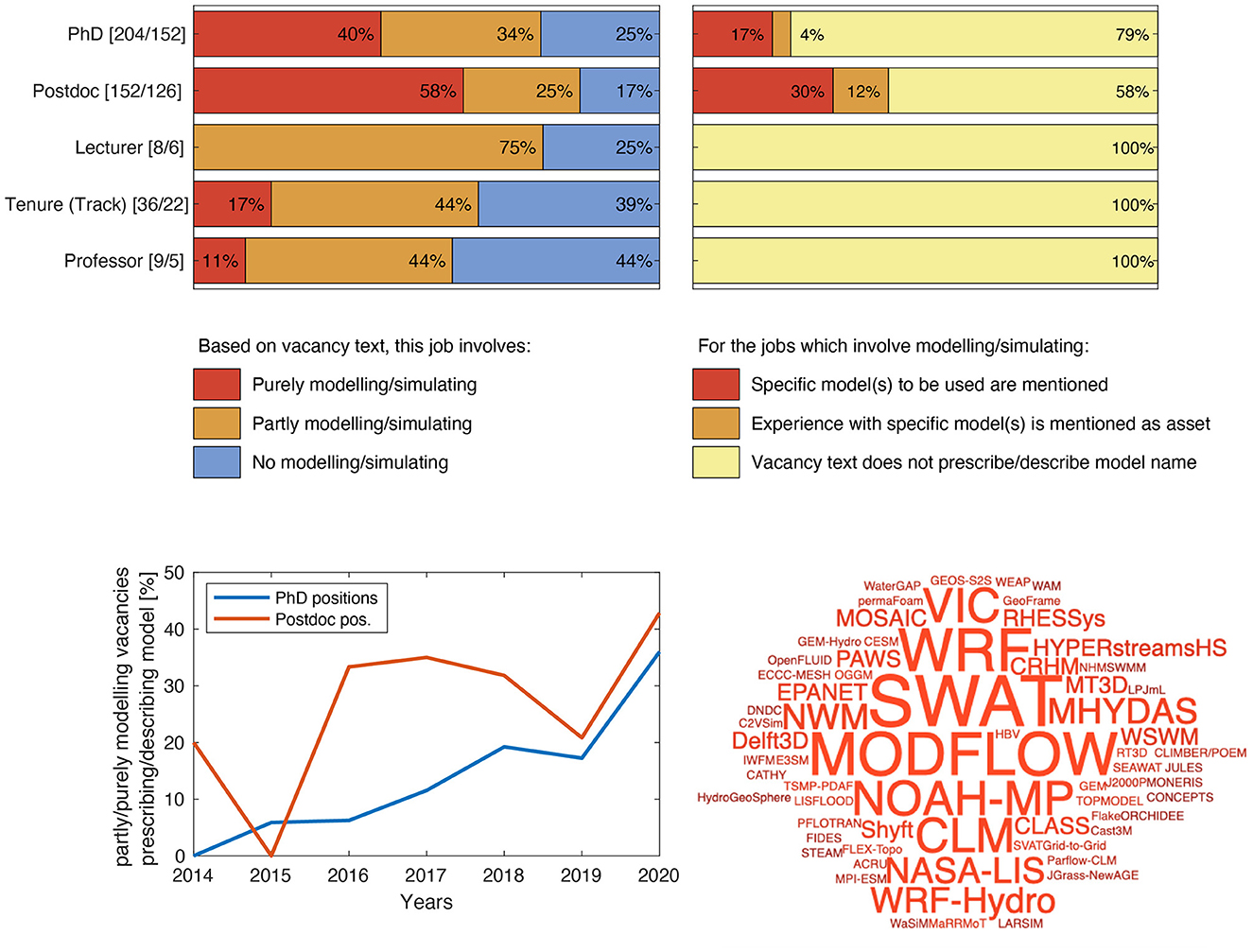
Figure 1. Results based on the vacancies announced through the About Hydrology mailing list. Top left: The percentage of vacancies that involved modeling, broken down by position. Top right: The percentage of vacancies in which the model is already mentioned in the vacancy text. The numbers between square brackets behind the positions indicate the total number of considered vacancies, and the number of vacancies that involved modeling, respectively. Bottom left: Percentage of vacancies mentioning a model, over time. Bottom right: Word cloud of the model names that were found in the vacancies. The size is proportional to the frequency that the model was encountered.
Only the vacancies that involve modeling or simulations as part of the job description were considered to determine the percentage of vacancy texts that prescribe a model. The top right panel of Figure 1 shows the percentage of modeling vacancies in which the model is prescribed. It stands out that for the higher academic positions, no prescribed model was found. Assistant, associate, and full professors are expected to develop their own research line, and given these vacancies this is not bounded by prescribed model use. Although it does occur that models are prescribed for more senior scientific positions: In the Dutch AcademicTransfer database, one instance was found where the model was prescribed for a tenure track position, while for two tenure track vacancies, relevant model experience was prescribed (see Supplementary material 2).
For PhD and postdoc positions, the picture is different: 17% of the PhD and 30% of the postdoc job descriptions explicitly mention which model has to be employed (18 and 22% in the AcademicTransfer database, respectively). Altogether, this means that there are 64 vacancies in the About Hydrology database which explicitly prescribe which model will be used in the project. These 64 vacancies are associated to 36 different institutes (Table 1). For the vacancies that are purely modeling based, the percentage of vacancies that prescribe model use goes up to 24% for PhD candidates and 40% for postdocs. Example quotes from vacancy texts are “The PhD-candidate will set-up an ensemble of runs with the model TSMP-PDAF for the African continent”, and “The postdoctoral researcher will work with existing Soil Water and Assessment Tool (SWAT) watershed models”.
There can be several reasons why the percentage of prescribed models is higher for postdoc positions than for PhD positions. Firstly, it is possible that models are not mentioned in PhD vacancies because most PhD applicants might not yet be familiar with these model names. Secondly, postdoc positions are generally for a shorter period of time, leaving less time to thoroughly investigate which model would suit the research question. To make efficient use of the limited time in a postdoctoral position, the model is already prescribed. Thirdly, there are several institutes that are responsible for large modeling infrastructures of (inter)national importance. These institutes make more use of postdoc than PhD positions and need their effort to maintain and further develop these infrastructures. An example is the WRF(-hydro) model, which is developed and housed at the National Center for Atmospheric Research and which is the core of the National Water Model (NWM) of the US.
For a smaller percentage of vacancies, models are mentioned in the context that it is an asset when the candidate has experience with it, which can be an indication that this model is foreseen to be used. For PhD positions this is only 5%, of which 1 percent point also explicitly mentions the model in the vacancy text—hence, 4% of the PhD vacancies mentions a model only in the context of experience. An example quote is: “Experience with hydrological catchment modeling approaches such as SWAT is an advantage.” As this quote shows, experience with a model is usually less strictly formulated, but refers to experience with models “such as” a specific model. It can be explained why only a small fraction of the vacancy texts refers to experience with specific models for PhD candidates. Many applicants for a PhD position only obtained model experience during their studies or internship. These few weeks or months of experience would also fit inside a PhD project. This is also demonstrated by a vacancy that explicitly describes that the selected candidate will be trained with the foreseen model: “In particular, the modeling student will conduct a year long exchange to LBNL to learn PFLOTRAN”.
For postdoc positions, 21% of the job descriptions describe experience with a specific model as asset (of which 9 percent point also describe the model in the vacancy—12 percent point only mention a model in an experience context). Like for PhD positions, also for postdoc positions the required experience is, in general, less strictly related to a specific model, as in this example: “The ideal experience would include basin-scale groundwater modeling using MODFLOW and MT3D or RT3D, or similar programs”. That the percentage of vacancies that consider experience with specific models as asset is higher for postdoc positions, can be explained because the postdoc can have obtained substantial experience with these models during earlier PhD work and previous postdoc positions. Rather than considering specific model experience as asset, there was one example where a model was explicitly not mentioned: “According to the skills of the applicant, the chosen model will be mastered by the applicant or proposed by the supervision team”.
The lower left panel of Figure 1 depicts the fraction of modeling involved vacancies that mention the model name in the advertisement over time. For the postdoc positions, no clear temporal trend is visible in prescribed models, whereas for PhD positions, the number of vacancies prescribing models seems to steadily increase over time. However, the evaluated sample is small which hampers drawing strict conclusions on temporal trends in model prescription. The word cloud (bottom right panel of Figure 1) indicates which specific models were mentioned in the vacancies, either to be used, or advantageous to have experience with. In total, 70 different models were mentioned, sometimes with minor differences, such as SWAT and SWAT+, or the notion that WRF-Hydro is an essential part of the National Water Model NWM. Most frequently mentioned were SWAT, Modflow, WRF, NOAH-MP, and CLM. This shows that for hydrological positions, not only hydrological models, but also climate and weather models (CLM, WRF) are prescribed.
Modeling studies without model name mentioned in the vacancy text can broadly fall into three categories:
(1) The candidate indeed has the freedom to select their own model.
(2) The project is about developing or building a new model.
(3) A model is prescribed but not mentioned in the advertisement.
Given this last category, the percentage of vacancies for which the model is prescribed might in reality be higher than what is estimated here. The results from the questionnaire in the next section will provide more insights on that.
3.2. Questionnaire results for further context
Whereas the analysis of the About Hydrology vacancy database can provide insights into to what extent ECSs are confronted with a prescribed model in their projects, this is of course only the vacancy and the project can have taken a different turn. Therefore, a stratified random sample was taken from the vacancies in the database, and the contact persons were approached to fill out a short questionnaire. In this way, model use during the project could be reconstructed. Figure 2 provides an overview of the responses for part of the questions in the questionnaire. Given the relatively small sample of respondents, no patterns could be identified in the responses for PhD and postdoctoral vacancies separately, so they are treated together. The results are now discussed per group: (1) a specific model is mentioned in the vacancy text, (2) experience with a specific model is considered an asset and (3) the remaining vacancy texts that involve at least some modeling.
From the vacancies for which a model was mentioned in the vacancy text, for 7 out of 8 responses this model was indeed used during the project. In fact, using this model or switching to another model has not even been discussed for these 7 projects. A possible reason is that, given that the model is mentioned in the vacancy text, the expectations from both the supervisor and the hired candidate in terms of model use are clear directly from the start. Reasons to select the prescribed model for these seven projects were:
• It was a requirement from the funding agency (1).
• The model was developed by the hiring person or the hiring lab (3).
• It covers a very specific process that is needed for the project (2).
• It fitted the research question and the expertise of the group (1).
One respondent did discuss model use for this project and did decide to switch models. The reason for switching from A to B was that the end users of this project, consultants in the US, were more familiar with modeling framework B. As such, the project might better accommodate the wishes of the funder (state government agencies). However, the experience with framework B was no undivided success, since certain relevant processes were not described well in this framework.
One of the potential reasons not to switch model in the project could be that this model was “promised” in the grant proposal. Therefore, one of the questions in the questionnaire was if the model was already specified in the proposal. Unfortunately, the question was not formulated unambiguously (a methodological flaw) which lead to some answers not being directly usable. Three out of seven respondents indicated that they did specify the model in the research proposal. These three also did use the model that was specified. Only one respondent indicated that the model was a critical component for the funding agency.
For the vacancies which mention experience with a specific model as asset, it was asked if this was also the model that was used during the project. Four out of six respondents indicate that this was indeed the case, while for two projects, another model was used. In both these cases, the reason for using another model was that the processes in these models better suited the research question after a comparison, for one of these projects also the postdoc's familiarity with the model was mentioned as reason. In at least four out of six cases, model selection has actively been discussed, which is a notable difference compared to the projects where specific models are already mentioned in the vacancy text. However, three out of four discussions did not lead to a change in used model compared to the initial plan. Reasons for not switching included for one case a combination of familiarity with the model and the representation of certain processes, while for another respondent the experience of the hired postdoc was decisive to not switch. Concerning grant proposals, there was only one respondent who confirmed that the model was mentioned in the proposal, while still a different model was used for this project in the end. This project was funded internally, and therefore there was no commitment or accountability to stick with the initially mentioned model. In two other cases, several options were mentioned in the proposal, and the final used model was one of them.
For the vacancies that do not specify any model in the text, and do not mention experience with any specific model as asset, five out of seven respondents did already have a model in mind before the project started. For the two that did not have a model in mind, one project was focused on machine learning and just wanted any “standard” numerical model as a reference. In the end, VIC was used in this project because it was already set-up for the test basin. For the other project there were some requirements that the model needed to fulfill, but the model choice would depend on the experience of the hired person.
For the five other respondents, it is not surprising that the hiring persons already had a model in mind for the project. After all, this is often part of evaluating the feasibility of the proposed research. What is surprising, however, is that for four out of five responses, there has not even been any discussion about using this model or switching to another, which seems to indicate that also for vacancies that do not mention any model, model choice is still often taken for granted. Four out of five respondents that did have a model in mind, already mentioned this model in the research proposal.
In summary, the questionnaire responses indicate that irrespective of whether a model is mentioned in the vacancy text or not, or whether experience with a specific model is mentioned as asset or not, for the majority of projects there is already a candidate model in mind that is not actively discussed once the project starts: model choice for the ECS project is taken for granted. In the cases that there is discussion, the majority of these discussions does not lead to switching to a different model. One of the reasons not to discuss model use or switch models could be because there is commitment to the model in the grant proposal. One respondent for instance indicated that this was a hard requirement from the funder. There are, however, also examples in the responses where no model was specified in the proposal, where the model was still switched despite being mentioned in the proposal, or where multiple models were mentioned in the proposal. This indicates that grant commitment alone cannot explain why model choice is often not discussed in research projects.
4. The hired candidates and their “Modeling Toolkit”
The results in the previous sections show that for many ECS projects, the hiring person already has a model in mind, and often this model is used without further discussion. This can also be understood: the hiring person generally has more experience than the ECS on this topic, and as such might better be able to estimate which model might be feasible for the project. This was also indicated by one of the respondents, who made a distinction there between PhD and postdoc-positions:
“..for PhD students, as they rarely have really experienced in an advanced code, I think it's easier if we tell them a little bit what code they should use. But for postdocs, as long as it does the job, it's fine.”
So, indeed hiring persons can be open to switch models if the ECS comes up with another proposal. Model selection driven by the experience of the applicant was even explicitly mentioned in some of the vacancy texts and questionnaire responses. But this is of course only possible when the early-career scientist has the experience to come up with a model proposal, and this is where the introduction of the term “Modeling Toolkit” becomes relevant.
Simplified, the development of such a toolkit can be described in the following way. In the first project of the ECS, usually a PhD-project, the supervisor suggests a model because the ECS does not have the experience yet to make this consideration. Throughout this project, the ECS gains experience with this model, and it becomes part of their “Modeling Toolkit”. In the next position of this researcher, either a model is proposed again by the supervisor, or the researcher can propose the model they have in their “Modeling Toolkit”. So, either the researcher extents their toolkit with a new model, or re-uses tools from their toolkit. If this researcher in the end obtains a (semi)-permanent position, they have a certain amount of models in their toolkit, which they might decide to further explore in their career. Babel et al. (2019) for instance interviewed senior scientists from different Earth and Universe disciplines, and the majority of the interviewees continued developing the modeling concepts they worked on during their PhD or as young postdoctoral researcher. The “Modeling Toolkit” that early career scientists obtain can thus be decisive for the course of their scientific career.
Responses on the questionnaire seem to confirm this perspective. First of all, four out of six respondents from vacancies which mention experience with a specific model as asset indicated that the hired candidate indeed had experience with this model. Although one respondent mentioned that this experience was not decisive in the selection of the candidate, it does show that experience with a certain model influences the chances for the ECS on a next position. It might therefore be beneficial to gain experience with widely used and widely known models, such as VIC, WRF(-Hydro), SWAT or MODFLOW. The use of a popular model can also further increase the exposure of the research. One of the hired candidates that responded to the questionnaire even indicated that their experience with VIC lead to job offers:
Question: “Did your experience with VIC influence for which jobs you applied after?
”Answer: “Yes. I got some job offers for positions which I did not even apply for.”
Although these offers probably also relate to the qualifications of this researcher, and not only to the experience of this researcher with this model. From the hired respondents of which the project was already finished, four out of five indicated that the model with which they gained experience in the project still played a role in their current position, demonstrating how the “Modeling Toolkit” is carried along to next positions.
The role of the “Modeling Toolkit” not only influences job opportunities, but can also influence the developed research line, as becomes evident from this response from a hired ECS:
Question: “Did your experience with [model] influence for which jobs you applied after?
”Answer: “Yes. Indirectly, because [model] clearly became a key tool for some of the research directions I proposed for later project proposals, including the one leading to my current position. And while I sometimes think I was lucky to find a tool that matched the research lines I wanted to develop, the other way around is likely: I was actually influenced by the possibilities with [model] to feel like further exploring water pathways with metrics such as water ages (calculated in the model).”
The “Modeling Toolkit” thus creates a reinforcing loop of model use, especially for ECSs that are developing their research identity. All in all, there are good reasons for a supervisor to advice a model for an ECS research project, but it should not be underestimated how long the effect of this advice can last. Besides, this system can work as a wheel to make popular models more popular: if more vacancies with a particular model are open, then also more young researchers obtain this model in their “Modeling Toolkit”, which influences their research identity and research line and which might make them, at a later stage in their career, also hire ECSs to work with this model. As such, the “Modeling Toolkit” can confirm and maintain the status quo in current hydrological modeling.
5. Implications for hydrological modeling
The results show that Early-Career Scientists (ECSs) are confronted with model choices that are, also for good reasons, often imposed. This happens especially at a stage in their career where they are developing and establishing themselves as researchers, and therefore these choices might have a long lasting effect on their developing research epistemology. Of course there will be further development of the research vision throughout the course of their career, and they might decide to break with the traditions they have worked with so far, but either way each experience they gained contributes to the development of their research vision and they carry their “Modeling Toolkit” along, whether in active use or catching dust. The question is what this implies for progress in scientific hydrological modeling and the use of hydrological models to support decision making.
5.1. Efficient research and successful science
As already mentioned, there might be good reasons to propose or impose a model for a research project. One of the clearest reasons is that the supervisors have more experience to make this evaluation. However, as demonstrated by Addor and Melsen (2019), model choice is often the result of legacy, where legacy includes practicality, convenience, and experience. Therefore, in many cases a model is not selected based on the experienced evaluation of the supervisor, but based on experience of the supervisor. This is also what appeared from the questionnaires, where a model was often selected because someone from the supervision-team, or the research team in general, had experience with this model.
As also discussed in Addor and Melsen (2019) and in Melsen (2022), legacy-reasons to select a model can create an efficient research environment: a modeling ecosystem is set-up which makes working with the model extremely efficient, for instance with automated preparation of input files. One of the hired candidates that was approached for this study wrote the following response:
“I am open to work with different models but I am also more aware that it can take significant amounts of time to get used to models. It also makes it more ridiculous if post-doc positions are advertised for two years and less that require learning a new model. I think that this takes at least half a year for a complex model.”
Working in a team with experienced colleagues in a well-organized modeling ecosystem is an effective environment for ECSs, because they have to spend less time on technical and organizational matters around using a model, and can focus more time on the actual analyses and on writing scientific publications. Furthermore, if the whole team is working on the same model there are more opportunities for collaboration with direct colleagues. Internal ties foster mutual trust, which leads to more knowledge sharing among team members (Garcia-Sánchez et al., 2019). It has also been shown that more cohesion inside the team can lead to more productivity in terms of publications (Stvilia et al., 2011; Love et al., 2021), and more in general it has been shown that science and high impact research is becoming more team-dominated (Wuchty et al., 2007). As such, becoming member of a well-organized modeling team can give an ECS better qualifications, e.g., in terms of publication record, for a follow-up position within the sciences than a peer that has to invest time in selecting an appropriate model or setting up a model without an experienced mentor.
Interviews with postdoctoral researchers in the UK revealed that several of them perceive their publication record as the main obstacle for them to secure a lectureship (Menard and Shinton, 2022), which could therefore be a motivation to apply for positions in such an efficient modeling environment [at the same time, Milojevic et al. (2018) found that productivity is not a reliable predictor for “survivability” in academia]. Furthermore, it is important to remark that ECSs have different perspectives on what they consider a successful career, and this does not necessarily has to be obtaining a tenure track position. From a questionnaire held by Nature among 7,670 postdoctoral researchers worldwide (go.nature.com/3tmckuq), 63% indicated to hope to pursue a career in academia, while 11% did not want to continue in academia and 26% was unsure. But also career paths inside academia can take different directions. Menard and Shinton (2022) identified different career paths for ECSs, and not all ECSs aim for a lectureship or tenure-track position. Aiming for a research-only or support-staff career (both usually consisting of a chain of short-term contracts) can lead to different strategic choices in the positions one applies for.
5.2. The essential tension
The “Modeling Toolkit”, which is usually mainly build up during the early part of ones scientific career, in combination with the reward of working with established models and in well-established teams around a model for future career perspectives, implies that the models that are currently popular and invested in, will remain popular and invested in. This is further reinforced by several mechanisms in current academia. One is the conventional-approach bias from reviewers (Martin, 2000), which makes it easier to publish work with established models than to propose a new approach. Another mechanism is the neo-liberal direction that higher education has taken, which puts more focus on productivity and output targets which are measured through audits (as for instance in the tenure-track system). More in general, it has been demonstrated that scientific papers have become less disruptive over time, and the authors of this study attribute that to the use of a narrower set of existing knowledge (Park et al., 2023), also described in the context of the “publish or perish”-culture. Re-appearing in several studies based on interviews with ECSs is that within this system “you have to read the game” (Archer, 2008) or “play the game” (Billot, 2010) to secure ones position. This implies identifying hot topics and partnering with big names to increase the chances of obtaining a grant (Billot, 2010).
These mechanisms lead to a model lock-in, creating dependency on certain models because of all the investments done, also in terms of human capital. This is a very instrumental perspective on modeling. The model concept itself does not seem to be questioned, the model is merely used as an instrument to answer questions, comparable to lab equipment. One might argue that in practical applications, such as real time forecasting and national models for decision-support, an instrumental vision can be justified by earlier investments, increased efficiency, and correct use of the tool. But especially practical applications have “real-world” impacts (Lane, 2014). Social practices around modeling, such as recruitment strategy as discussed here, influence which models are used and which results are obtained (Melsen et al., 2018). These results, which inform real-world decision making, might be biased toward certain interests, and might represent certain groups better than others (Saltelli et al., 2020). It is difficult to correct or even observe these biases in such a model lock-in system.
Models can also be perceived as hypotheses (Clark et al., 2011). In that capacity, the underlying assumptions should continuously be critically questioned and tested, and new hypotheses should be formulated. Inquisitiveness is a frequently mentioned scientific characteristics, in the same way that modeling is described as an “art” that requires imagination, inspiration, and creativity (Savenije, 2009). The current, instrumental, system seems to provide limited space for this creativity, because it rewards working within the boundaries of the current discipline. Even though challenging existing conceptions has proven to contribute to scientific progress (Balietti et al., 2015), which would indicate that we need to perceive models more as hypotheses and less as instruments. Also the title of the paper of Burt and McDonnell (2015), “the need for outrageous hydrological hypotheses”, is an active call to stimulate scientists to think out of the box and to challenge current paradigms in hydrology.1 At the same time, as described in the previous section, the current scientific system rewards people that function within this system, and does not necessarily reward people that question this system. Or, in the context of this study, prescribing a model in ECSs projects, despite good underlying reasons, detains early career scientists into currently leading paradigms, which might hamper their ability to question this paradigm—especially since it is rewarding to function within current paradigms and “play the game”.
The reward of functioning within the “disciplinary matrix” on the one hand, and the need for outrageous hypotheses to stimulate scientific progress on the other, seem to resemble two opponents. Kuhn (1977) refers to these opponents as “the essential tension”. Tension, because one seems to contradict the other, and essential, because he argues that this contradiction is necessary: “.. history strongly suggests that, though one can practice science—as one does philosophy or art or political science—without a firm consensus, this more flexible practice will not produce the pattern of rapid consequential scientific advance to which recent centuries have accustomed us” (p. 232)—that is, most progress is made in disciplines that have strong disciplinary rules. The tension between commitment to the discipline, in this case hydrological modeling, and questioning this commitment, is an everlasting challenge that should be reconciled both at the individual and the team level.
The mechanisms described in this study, related to recruitment for modeling positions, is just one example of the many social practices that shape the construction of scientific knowledge. There is a growing interest in analyzing the practices of modeling in hydrology, and how this propagates into knowledge construction. Examples include the interviews with modelers conducted by Babel et al. (2019) and Melsen (2022) which show the role of habits and colleagues in modeling, respectively. Packett et al. (2020) discusses how gender is mainstreamed into model construction and use, while Krueger et al. (2012) discusses the role of expert opinion in modeling. Broader perspectives on modeling and their non-neutrality are for instance discussed in Saltelli et al. (2020) and Krueger and Alba (2022). Even more broadly, Lave (2016) discusses how social dynamics shape river science itself. What these studies have in common is that they challenge the notion of scientific knowledge as empirical and objective. Increased understanding and transparency about the role of social processes in knowledge production can provide guidance in choosing research practices and in placing the gained insights into context (Lave, 2016; Melsen, 2022), and there are still ample dynamics to explore.
6. Conclusion
Modeling is a key activity in the hydrological sciences, demonstrated by the fact that 76% of the analyzed job descriptions, based on job announcements distributed through the About Hydrology mailinglist, involved at least some numerical modeling. It was found that for 17% of the PhD positions that involve any modeling, the model is already predetermined in the vacancy text. For postdoc positions, this is 30%. Based on a small questionnaire, it can be concluded that also beyond the vacancies where the model is already prescribed, in many Early-Career Scientist (ECS) projects, the model to be used is pre-determined and actually often used without much further discussion.
There are valid reasons for prescribing a model for ECS projects, such as funding requirements, grant commitment, and the experience of the supervisor in making this evaluation. At the same time, this can have long-term consequences for the ECS: first of all because the ECS is still developing a research identity which will be influenced by the experiences they obtain, but secondly because throughout their career, experience with models will become part of their “Modeling Toolkit”. This toolkit influences their future opportunities. Experience with a popular model can for instance be beneficial for obtaining a next position, or working in a team around a certain model can be more productive in terms of publications and therefore give a better position compared to peers. These mechanisms reinforce the use of popular models rather than to question them—it serves an instrumental vision on modeling.
Models can also be seen as hypotheses, which should continuously be tested and questioned. The current academic system does not necessarily seem to reward this vision, even though it stimulates the progress of science. Kuhn (1977) described this as “the essential tension”: There is a tension between functioning within the system (the so-called disciplinary matrix) which is rewarding, and actively questioning this system to stimulate progress. As a hydrological modeling community, we should actively search for this tension. ECSs will learn the current rules of the game, while at the same time should be actively stimulated to critically question these rules.
Of course, ECSs should also not be underestimated. The PhD candidate that was quoted in the introduction for instance, saw this position as an opportunity to work with an established model. This person was not pushed into the disciplinary matrix as a passive bystander. The postdoctoral researcher that was quoted in the introduction had a clearly developed model vision. This person wrote a proposal in order to be able to work with the modeling framework that this person perceived as useful. With a developed model vision, a researcher can be critical in selecting which vacancies to apply to, to make sure that the modeling approach aligns with how this person perceives which model-based knowledge is justified. It seems, therefore, that everything starts with pro-actively stimulating ECSs to develop a vision on research and modeling.
Data availability statement
The datasets presented in this study can be found in online repositories. The names of the repository/repositories and accession number(s) can be found below: https://www.hydroshare.org/resource/f6661d92db1740f498f92ffca090789c/.
Ethics statement
Ethical review and approval was not required for the study on human participants in accordance with the local legislation and institutional requirements. The patients/participants provided their written informed consent to participate in this study.
Author contributions
The research idea, research design, data collection, processing and analysis, and writing of the manuscript was all done by LM.
Funding
This study was conducted with a personal Veni grant (nr. 17297, entitled What about the modeler? The human-factor in constructing Earth and environmental predictions) from the Dutch Research Council.
Acknowledgments
LM would like to thank Frans Visser and Arno de Groof from AcademicTransfer (www.academictransfer.com) for kindly making their database available for this research, and for transferring the database from PostgresSQL to Excel format.
Conflict of interest
The author declares that the research was conducted in the absence of any commercial or financial relationships that could be construed as a potential conflict of interest.
Publisher's note
All claims expressed in this article are solely those of the authors and do not necessarily represent those of their affiliated organizations, or those of the publisher, the editors and the reviewers. Any product that may be evaluated in this article, or claim that may be made by its manufacturer, is not guaranteed or endorsed by the publisher.
Supplementary material
The Supplementary Material for this article can be found online at: https://www.frontiersin.org/articles/10.3389/frwa.2023.1149590/full#supplementary-material
Footnotes
1. ^in fact, Burt and McDonnell (2015) even emphasize this in the context of the majority of hydrological research being focused around models only, which can be counteracted by seeing models as a hypothesis. But indeed, considering modeling as a valid research method can be seen as a paradigm in itself in hydrology.
References
Addor, N., and Melsen, L. (2019). Legacy, rather than adequacy, drives the selection of hydrological models. Water Resour. Res. 55, 378–390. doi: 10.1029/2018WR022958
Archer, L. (2008). The new neoliberal subjects? Younger academics' constructions of professional identity. J. Educ. Policy 23, 265–285. doi: 10.1080/02680930701754047
Babel, L., Vinck, D., and Karssenberg, D. (2019). Decision-making in model construction: unveiling habits. Env. Mod. Softw. 120, 104490. doi: 10.1016/j.envsoft.2019.07.015
Balietti, S., Mäs, M., and Helbing, D. (2015). On disciplinary fragmentation and scientific progress. PLoS ONE. 10, e0118747. doi: 10.1371/journal.pone.0118747
Beven, K. (2002). Towards a coherent philosophy for modelling the environment. Proc. Math. Phys. Eng. Sci. 458, 2465–2484. doi: 10.1098/rspa.2002.0986
Beven, K. J. (2012). “Down to basics: runoff processes and the modelling process,” Rainfall-Runoff Modelling, The Primer, 2nd Edn. (West Sussex: Wiley-Blackwell).
Billot, J. (2010). The imagined and the real: identifying the tensions for academic identity. Higher Educ. Res. Dev. 29, 709–721. doi: 10.1080/07294360.2010.487201
Boorman, D. B., Williams, R. J., Hutchins, M. G., Penning, E., Groot, S., and Icke, J. (2007). A model selection protocol to support the use of models for water management. Hydrol. Earth Syst. Sci. 11, 634–646. doi: 10.5194/hess-11-634-2007
Burt, T., and McDonnell, J. (2015). Whither field hydrology? the need for discovery science and outrageous hydrological hypotheses. Water Resour. Res. 51, 5919–5928. doi: 10.1002/2014WR016839
Clark, M., Kavetski, D., and Fenicia, F. (2011). Pursuing the method of multiple working hypotheses for hydrological modeling. Water Resour. Res. 47:W09301. doi: 10.1029/2010WR009827
Clark, M., Nijssen, B., Lundquist, J., Kavetski, D., Rupp, D., Woods, R., et al. (2015). A unified approach for process-based hydrologic modeling: 1. modeling concept. Water Resour. Res. 51, 2498–2514. doi: 10.1002/2015WR017198
Clark, M. P., Slater, A. G., Rupp, D. E., Woods, R. A., Vrugt, J. A., Gupta, H. V., et al. (2008). Framework for understanding structural errors (FUSE): a modular framework to diagnose differences between hydrological models. Water Resour. Res. 44:W00B02. doi: 10.1029/2007WR006735
Garcia-Sánchez, P., Diaz-Diaz, N., and De Saá-Pérez, P. (2019). Social capital and knowledge sharing in academic research teams. Int. Rev. Admin. Sci. 85, 191–207. doi: 10.1177/0020852316689140
Gharari, S., Gupta, H. V., Clark, M. P., Hrachowitz, M., Fenicia, F., Matgen, P., et al. (2021). Understanding the information content in the hierarchy of model development decisions: learning from data. Water Resour. Res. 57, e2020WR027948. doi: 10.1029/2020WR027948
Gupta, H. V., Clark, M. P., Abramowitz, J. A. V. G., and Ye, M. (2012). Towards a comprehensive assessment of model structural adequacy. Water Resour. Res. 48. doi: 10.1029/2011WR011044
Höge, M., Wöhling, T., and Nowak, W. (2018). A primer for model selection: the decisive role of model complexity. Water Resour. Res. 54, 1688–1715. doi: 10.1002/2017WR021902
Jakeman, A. J., Letcher, R. A., and Norton, J. P. (2006). Ten iterative steps in development and evaluation of environmental models. Environ. Model. Softw. 21, 602–614. doi: 10.1016/j.envsoft.2006.01.004
Jansen, K. F., Teuling, A. J., Craig, J. R., Dal Molin, M., Knoben, W. J. M., Parajka, J., et al. (2021). Mimicry of a conceptual hydrological model (hbv): What's in a name? Water Resour. Res. 57, e2020WR029143. doi: 10.1029/2020WR029143
Knoben, W. J. M., Freer, J. E., Fowler, K. J. A., Peel, M. C., and Woods, R. A. (2019). Modular assessment of rainfall–runoff models toolbox (MARRMoT) v1.2: an open-source, extendable framework providing implementations of 46 conceptual hydrologic models as continuous state-space formulations. Geosci. Model Dev. 12, 2463–2480. doi: 10.5194/gmd-12-2463-2019
Krueger, T., and Alba, R. (2022). Ontological and epistemological commitments in interdisciplinary water research: uncertainty as an entry point for reflexion. Front. Water 4, 1038322. doi: 10.3389/frwa.2022.1038322
Krueger, T., Page, T., Hubacek, K., Smith, L., and Hiscock, K. (2012). The role of expert opinion in environmental modelling. Environ. Model. Softw. 36, 4–18. doi: 10.1016/j.envsoft.2012.01.011
Kuhn, T. (1977). “The essential tension: tradition and innovation in scientific research?” in The Essential Tension - Selected studies in Scientific Tradition and Change (Chicago, IL: Univeristy of Chicago Press), 225–239.
Lane, S. N. (2014). Acting, predicting and intervening in a socio-hydrological world. Hydrol. Earth Syst. Sci. 18, 927–952. doi: 10.5194/hess-18-927-2014
Lave, R. (2016). Stream restoration and the surprisingly social dynamics of science. WIREs Water 3, 75–81. doi: 10.1002/wat2.1115
Love, H., Cross, J., Fosdick, B., Crooks, K., VandeWoude, S., and Fisher, E. (2021). Interpersonal relationships drive successful team science: an exemplary case-based study. Human. Soc. Sci. Commun. 8, 1–10. doi: 10.1057/s41599-021-00789-8
Melsen, L. (2022). It takes a village to run a model: the social practices of hydrological modelling. Water Resour. Res. 58, e2021WR030600. doi: 10.1029/2021WR030600
Melsen, L., Vos, J., and Boelens, R. (2018). What is the role of the model in socio-hydrology? discussion of ‘prediction in a socio-hydrological world'. Hydrol. Sci. J. 63, 1435–1443. doi: 10.1080/02626667.2018.1499025
Menard, C., and Shinton, S. (2022). The career paths of researchers in long-term employment on short-term contracts: case study from a UK University. PLoS ONE 17, e0274486. doi: 10.1371/journal.pone.0274486
Milojevic, S., Radicchi, F., and Walsh, J. (2018). Changing demographics of scientific careers: the rise of the temporary workforce. Proc. Natl. Acad. Sci. U.S.A. 115, 12616–12623. doi: 10.1073/pnas.1800478115
Packett, E., Grigg, N., Wu, J., Cuddy, S., Wallbrink, P., and Jakeman, A. (2020). Mainstreaming gender into water management modelling processes. Environ. Model. Softw. 127, 104683. doi: 10.1016/j.envsoft.2020.104683
Park, M., Leahey, E., and Funk, R. (2023). Papers and patents are becoming less disruptive over time. Nature 613, 138–144. doi: 10.1038/s41586-022-05543-x
Saltelli, A., Benini, L., Funtowicz, S., Giampietro, M., Kaiser, M., Reinert, E., et al. (2020). The technique is never neutral. how methodological choices condition the generation of narratives for sustainability. Environ. Sci. Policy 106, 87–98. doi: 10.1016/j.envsci.2020.01.008
Savenije, H. (2009). HESS opinions: the art of hydrology. Hydrol. Earth Syst. Sci. 13, 157–161. doi: 10.5194/hess-13-157-2009
Sjastad Hagen, J., Cutler, A., Trambauer, P., Weerts, A., Suarez, P., and Solomatine, D. (2020). Development and evaluation of flood forecasting models for forecast-based financing using a novel model suitability matrix. Prog. Disaster Sci. 6, 100076. doi: 10.1016/j.pdisas.2020.100076
Stvilia, B., Hinnant, C., Schindler, K., Worrall, A., Burnett, G., Burnett, K., et al. (2011). Composition of scientific teams and publication productivity at a national science lab. J. Am. Soc. Inform. Sci. Technol. 62, 270–283. doi: 10.1002/asi.21464
Keywords: hydrological modeling, recruitment, vacancy, model development, model epistemology, job advertisement
Citation: Melsen LA (2023) The Modeling Toolkit: how recruitment strategies for modeling positions influence model progress. Front. Water 5:1149590. doi: 10.3389/frwa.2023.1149590
Received: 02 February 2023; Accepted: 20 April 2023;
Published: 12 May 2023.
Edited by:
Tobias Krueger, Humboldt University of Berlin, GermanyReviewed by:
Rossella Alba, Humboldt University of Berlin, GermanyFrancesca Pianosi, University of Bristol, United Kingdom
Copyright © 2023 Melsen. This is an open-access article distributed under the terms of the Creative Commons Attribution License (CC BY). The use, distribution or reproduction in other forums is permitted, provided the original author(s) and the copyright owner(s) are credited and that the original publication in this journal is cited, in accordance with accepted academic practice. No use, distribution or reproduction is permitted which does not comply with these terms.
*Correspondence: Lieke A. Melsen, lieke.melsen@wur.nl
†ORCID: Lieke A. Melsen orcid.org/0000-0003-0062-1301