- 1Water Resources Research and Documentation Center, University for Foreigners of Perugia, Perugia, Italy
- 2Department of Economics, Engineering, Society and Business Organization, University of Tuscia, Viterbo, Italy
- 3Department for Innovation in Biological, Agro-Food and Forest Systems, University of Tuscia, Viterbo, Italy
- 4Institute of Environment and College of Arts, Sciences and Education (CASE), Florida International University (FIU), Miami, FL, United States
- 5Department of Civil, Environmental and Architectural Engineering, University of Padua, Padua, Italy
Introduction: Monitoring water levels of ephemeral streams is a difficult yet important task in hydrology, especially when studying minor river flows in remote areas. The installation of flow gauging stations on upstream tributaries is impacted by the lack of economic resources, by accessibility problems and unstable morphological conditions of riverbeds avoiding the implementation of distributed observation networks at large scales. This major challenge in hydrology may be addressed by eventually adopting image-analysis approaches that constitute an effective parsimonious river flow monitoring method, but the demonstration of such techniques is still an open research topic.
Methodology: This study focuses on the testing of a novel technique that employs a white pole “staff gauge” to be photographed using a phototrap (i.e., named stage-cam which is a high-speed camera trigger system). This technology shows to be particularly efficient for observing flood events that represent the most difficult scenario for streamflow monitoring. Furthermore, the testing of this innovative hydrological data-gathering method is performed by adopting citizen science and participatory image analysis to assess the value and effectiveness of non-expert volunteers to operationalize this novel method. Citizen engagement may be essential for supporting distributed flow monitoring supporting large scale image analysis algorithm calibration associated to a continuous series of phototrap images. The Montecalvello watershed, located near Rome, is selected for this pilot case study.
Results: Results of the conducted tests, involving the University of Tuscia student community, are presented toward the demonstration of the effectiveness of citizen science to collect valid quantitative hydrological observations, which may correlate consistently with expert estimates. To better interpret results, the authors consider mean absolute error (MAE) and mean absolute relative error (MARE) as synthetic indices to determine the uncertainties associated to voluntary observations. Low margins of error return positive feedback on the adopted methodology.
Discussion: This research promotes the use of participatory approaches for addressing an actual hydrological monitoring challenge. In addition, it fosters increased citizen knowledge and awareness of the importance and value of hydrological monitoring of small ungauged river basins.
1. Introduction
Monitoring intermittent and ephemeral streams is a significant challenge in hydrology in terms of collecting accurate and continuous data at different spatial and time scales (Davids et al., 2017; Strobl et al., 2020; Noto et al., 2022). Difficult-to-access-environments and unstable riverbed conditions represent a significant portion of upstream areas hindering continuous and accurate hydrologic data acquisition using traditional instruments, such as remote sensing and river gauging stations (Ilja Van Meerveld et al., 2017; Seibert et al., 2019; Noto et al., 2022).
The use of low-cost tools, supported by citizen science and crowdsourcing methods, represents an innovative and effective hydrologic monitoring solution for such challenging domains and scales (Weeser et al., 2018; Zheng et al., 2018). The use of participatory methodologies is recognized as an important technique for next-generation solutions, also considering the value of increased engagement and awareness of indigenous communities. These methods are recognized to be fundamental to potentially address the 23 Unresolved Problems in Hydrology (UPH). UPH—an effort by 230 research scientists sharing the view and plan to project hydrology research efforts into the future—recognized transdisciplinary engagement as an important method to tackle technological, social, cultural, and organizational challenges affecting hydrologic research (Blöschl et al., 2019; Nardi et al., 2021).
Among the UPH, Blöschl et al. (2019) poses two major concerns regarding hydrological measurements and data collection: (1) the adoption of innovative technologies to measure and estimate surface water flows at different spatial and time scales (UPH #16); and (2) the collection of soft data from volunteered observations and their integration with traditional hydrological observations (UPH #17). Other issues focused on synergies and trade-offs between various societal goals in water management (UPH #22).
Crowdsourcing requires the use of suitable technologies to engage non-expert volunteers to carry out empirical research activities—such as data collection and interpretation—and higher-level work such as the co-production of knowledge and dissemination of results (Spasiano et al., 2021). Considering the multidisciplinary perspective of this type of work, the use of citizen science requires standardized and controlled protocols to support the validity and reliability of the results (Cappa et al., 2016; Kosmala et al., 2016).
UPH, with specific focus on the complexity of human-water interactions, prompts the need for adoption of transdisciplinary methodological approaches for solving hydrological challenges. In particular, the introduction of soft data (collected through informal methods) for use in estimates and measurement in hydrologic models, can be important for the estimation of water levels or water flows, and for monitoring of water quality in a stream (See et al., 2013; Zheng et al., 2018; Schrögel and Kolleck, 2019; See, 2019; Etter et al., 2020).
Citizen science constitutes a methodological paradigm, within a participatory approach to scientific research, for its characteristics of multidisciplinary and transdisciplinary collaboration (Eitzel et al., 2017; Gray et al., 2017; Knapp et al., 2019). As stated in Spasiano et al. (2021), multidisciplinary collaboration means the application of citizen science which covers several subject areas; while transdisciplinary indicates the integration of different scientific backgrounds and research designs—with operational, professional, and societal perspectives linked in a unique theoretical framework (Spasiano et al., 2021).
In this experimental hydrology research, citizen science offers benefits which address data scarcity through the involvement and active participation of volunteers. By acting as sensors or interpreters, volunteers are an important data source that extend spatially and temporally (Goodchild, 2007; Assumpção et al., 2018). Citizen science also offers social, cultural, and organizational benefits in terms of: (1) raising awareness, to encourage sustainable behaviors and a better use of water and environmental resources, with the potential for cultural change of citizens in their relationship and common use and management of water and related risks; (2) democratization of science, to promote bottom-up approaches to contextualize scientific research in a local, socio-cultural context; and (3) knowledge gained and social learning, toward adaptive forms of environmental management and decision making based on dialogue, consultation, and sharing of knowledge and practices that could be useful to address public issues (Walker et al., 2021).
In recent times, scientific research has investigated the value of citizen and online communities' involvement in environmental monitoring, and methods and tools to obtain large quantities of data to ensure homogeneous spatial and temporal coverage (Bonney et al., 2009; Dickinson et al., 2012; Zheng et al., 2018; Schrögel and Kolleck, 2019; Spasiano et al., 2022). The development of digital technologies makes widely available the use of tools such as smartphones and other applications for the immediate and standardized collection of data (Njue et al., 2019). Njue et al. (2019) classifies data acquisition procedures, in the context of citizen science, as: (1) automated, (2) semi-automated, and (3) manual, which require the use of smartphone applications, digital solutions, or text media.
Methods and tools also differ according to the specific aspects to be investigated, including water levels, flow, and quality. The application of models and tools related to citizen science do not reflect a single standardized procedure but rely on specific empirical applications commonly aimed at widening and increasing the availability of data through the involvement of citizens, especially in contexts where data acquisition is difficult (Assumpção et al., 2018). Also, the engagement methods differ according to the specific contexts and objectives set in the research phase.
Regarding water level estimations, several studies adopt data collection and public engagement approaches through the combination of automated and manual methods (Njue et al., 2019). These approaches aim to obtain water level estimates by volunteer observations which are then compared to benchmark values, official data, or with qualitative methods (Ilja Van Meerveld et al., 2017). Some studies have implemented crowdsourcing tools for the acquisition of environmental data through mobile-phone and SMS-text messaging (Fienen and Lowry, 2012; Lowry and Fienen, 2013; Weeser et al., 2018).
These applications combine automated and manual methods of data collection with digital tools at social and cultural levels (Weeser et al., 2018). Advances in digital technology enable data collection and public engagement processes to be implemented through smartphones and user interaction and data sharing platforms.
For example, data collection methods can be used on virtual staff gauge locations in which volunteers, in the role of human sensors, qualitatively estimate water levels by means of specific smart device apps (Seibert et al., 2019; Etter et al., 2020; Strobl et al., 2020; Nardi et al., 2021). These solutions, empowered by advanced digital technologies, can support, not only the citizen data collection activity, but also the organizational and communicative aspects that support the active participation of volunteers in the processes of knowledge sharing and co-production through specific platforms (Spagnoletti et al., 2015; Le Coz et al., 2016).
This work illustrates a pilot case study testing methodological approaches of citizen science in water level estimation of intermittent basins, with the specific goal of validating the image recognition algorithms' calibration by means of crowdsourced automation estimations.
On a technological level, Noto et al. (2022) designed and developed a stage-camera system for monitoring water levels in ungauged headwater streams by means of an image-based approach. This method was implemented with a simple, white-painted steel pole to estimate water depth as compared to the out-of-water pole segment displayed (Noto et al., 2022). This stage-camera system allows the acquisition of stream images at regular temporal intervals of 20 minutes. The system proved to be adaptable and reliable to understand and monitor the flow variations in headwater streams.
This research, thus, tests volunteer capabilities to provide reliable data for integration into a calibration strategy of the stage-cam method. The proposed procedure uses mean and median positioning, and variability measures, to assess volunteer performance to assign reliable values and to evaluate the effectiveness of volunteer observations over time.
Starting with positioning measures, the study, then, calculates mean absolute error (MAE) and mean absolute relative error (MARE) as synthetic indices to determine the uncertainties associated to voluntary observations. Qualitative insights from demographics indicators (educational background, university position, and environmental expertise) are also analyzed to evaluate the impact of social and cultural components on volunteers' performance. The proposed test applies principles of citizen science according to a transdisciplinary approach.
The research design is based on citizen science principles in terms of: (1) engagement of a small and controlled group of volunteers; (2) recruitment and training activities; (3) digital communication campaigns to support engagement; and (4) data processing. To conduct this experimental study, we adopted a contributory citizen science approach (Bonney et al., 2009). Our research team organized and coordinated all procedures of the experiment to support the volunteered data collection activity.
The paper is structured as follows: in Section 2, the authors provide an overview of citizen science principles and their application in water science. Section 3 focuses on methods and data collection descriptions at the foundation of the research design. Section 4 illustrates the results derived from data analysis. Sections 5 and 6 provide respectively discussion and concluding remarks.
2. The adopted citizen science framework
2.1. A brief summary of citizen science principles
The term citizen science generally refers to the engagement of non-expert volunteers in research activities. Citizen science knowledge and tools are often employed to face environmental challenges, such as the sustainable management of water resources, flood monitoring, and disaster risk reduction (Buytaert et al., 2014; Annis and Nardi, 2019; Annis et al., 2022). Citizen science approaches vary depending on the level of citizen engagement and the roles assigned to volunteers in data collection, processing, dissemination, and interpretation of results (Jordan et al., 2015; Bonney et al., 2016; Phillips et al., 2019).
In a contributory citizen science perspective, crowdsourcing is a basic technique for engaging volunteers in research activities as data collectors or interpreters (Jordan et al., 2015). Advanced citizen science approaches engage volunteers in the phases of consultation on methods and goals (collaborated citizen science) or co-designed and co-production of scientific knowledge (co-created citizen science; Phillips et al., 2019; Thornhill et al., 2019). These advanced citizen science approaches are applied when research goals match with collective social and environmental needs.
Citizen science is an empirical research approach which has a conceptual definition and theoretical framework subject to academic debate (Eitzel et al., 2017). However, the scientific literature identifies general methodologies and procedures that distinguish citizen science as a valid research approach (Njue et al., 2019; Spasiano et al., 2021) that include:
• Adoption of simple procedures
• Development of suitable (digital) tools
• Implementation of communication strategies
• Analysis and assignment to volunteers of specific roles in the research process and knowledge co-production
2.2. Integration of soft data and crowdsourcing techniques in water science
The use of soft data, as an alternative and complementary source of data, is a common practice in the field of hydrological-hydraulic modeling—such as in the estimation of water levels. However, data types and acquisition techniques can vary depending on context and purposes.
Soft data can be distinguished as intentional or unintentional (Zheng et al., 2018). The first category includes data collected for intentionally scientific purposes. The volunteer collects data—usually via digital mobile devices—for scientific research and environmental monitoring purposes (See et al., 2016). To this regard, citizen science approaches are adopted for the engagement of volunteers. Unintentional data is a category of data generated for purposes other than scientific research and environmental monitoring.
This is incidental data, often unstructured, including textual information and social media contents (Zheng et al., 2018). Data mining techniques are usually used to extract scientifically relevant information from user-generated contents, without the active involvement of volunteers. Unintentional data also includes data acquired by automatic detection tools which do not require direct and intentional action by human agents (Zheng et al., 2018).
In the field of surface water levels and water quality, the acquisition of data from citizen observations is mainly supported by: (1) dedicated tools; and (2) images or videos (Zheng et al., 2018; See, 2019). Dedicated tools include the development or adoption of technological solutions (software packages, apps, and digital platforms) for real-time data collection (See et al., 2016; Zheng et al., 2018; Seibert et al., 2019; Strobl et al., 2020). Images or videos provide data from in-situ instruments and sensors for subsequent analysis by volunteered users (Starkey et al., 2017).
This categorization also reflects a differentiation of activities and roles played by volunteers schematically divided into citizen as sensor (Goodchild, 2007; Elwood et al., 2012; Nardi et al., 2021) or citizen as interpreter (Assumpção et al., 2018). In the first case, volunteers act as sensors for field activities by using mobile digital devices to collect intentional data to be integrated into subsequent analysis and modeling (Le Coz et al., 2016). In the second case, volunteers do not collect data directly but are required to provide interpretations of an event by direct observation, but not necessarily in real time.
The use of soft data in water science is a widespread and well-established practice, notwithstanding some challenges remain. A first order of challenges concerns the ability and reliability of voluntary observations in relation to citizens as data interpreters—how accurate are their observations (Assumpção et al., 2018). Regarding this concern, we designed and tested a procedure of engagement and observation collection, by means of standardized and replicable protocol. The adopted procedure aimed at reducing and quantifying margins of error resulting from subjective evaluations. Standardization, homogenization, and large-scale data collection constitute a second order of problems which must be overcome to confidently use soft data in water science.
3. Methodology and data
This section describes the methods and data used in this research. Firstly, we illustrate the research methodology to understand the data collection process from the engagement activities of volunteers. Secondly, we report the adopted empirical approach to our methodology based upon firsthand experience to support our citizen science project.
3.1. The hydrological application: Stage-cam for water level observation
The stage-cam system represents a low-cost technological solution to acquire images that quantify water level variations in ephemeral streams at regular intervals of time (i.e., 20 minutes). The infrared technology integrated in the stage cam allows the retrieval of images at night and in low visibility conditions, ensuring continuous temporal coverage.
The study area is the Montecalvello Creek Basin in the province of Viterbo (central Italy), about 100 km north of Rome, where 16 stage-cams were installed to monitor the drainage network. With this set up, it was possible to estimate water levels upstream and downstream during the same meteorological event, and also to compare different events at the same site. In front of the camera lens, positioned at the bottom of the watercourse, a white-painted pole was installed to serve as the reference observation tool for estimating stream water levels.
At peak flows, a black ribbon was tied to the pole as a reference point to estimate water levels by an out-of-water-length method (Figure 1). Image analysis algorithms, used on the collected white pole images allowed the estimation of the distance in pixels between the black ribbon and the water surface elevation, providing the surface water level at a specific range of time.
Common hydrologic monitoring instruments are not effective in low-flow surface water environments, typically because a benchmark is not available for the calibration or the validation of data. The use of citizen science volunteers is a promising solution to obtain large data samples from numerous observations of streamflow levels. However, the use of citizen science implies, firstly, the need to identify a potential group of volunteers as field testers to verify the validity of the adopted procedures; secondly, the implementation of simple and replicable procedures for non-expert volunteer groups; thirdly, the use of digital technologies that enable volunteers to join the project, to access information, and to carry out the activities remotely and in a dependable and controlled environment.
Unlike most citizen science projects, in this study our research team asked volunteers to act as interpreters. Volunteers were not required to acquire data from their own but were asked to examine images provided by teams of experts according to established procedures. This solution tended to mitigate the potential margin of error but also limited the role of volunteers as contributors.
3.2. Training and engagement methodology of volunteers
The engagement methodology adopted in this project was based on simple procedures illustrated in Figure 2. The methodological procedure was articulated as follows: (1) recruitment and training; (2) engagement and digital communication; (3) data collection; and (4) results dissemination.
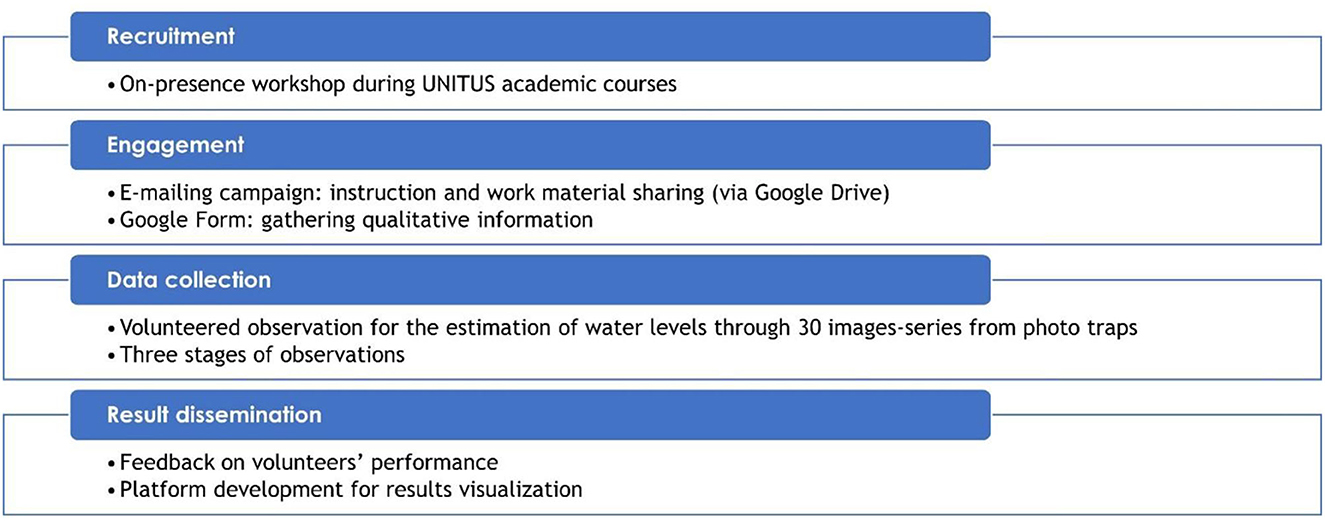
Figure 2. Methodological procedure adopted to support active participation of volunteers (UNITUS: University of Tuscia, Viterbo, Italy).
Recruitment activities, designed by the research team, included a dedicated brief workshop to prepare prospective citizen science students. The workshop (1) presented the research project; (2) framed the environmental and social issues related to the monitoring and management of water courses; (3) explained citizen science as a method of research toward shared and participatory solutions for water challenges; and (4) provided training on volunteered activities to estimate stream water levels through the observation of photo-traps frames.
The engagement activity took place during in-person meetings with students of different academic levels and disciplinary background. The research team organized brief workshops of 30 min each during classroom sessions to involve as many students as possible. During in-person workshops, researchers provided a theoretical introduction to citizen science to explain the use of non-expert volunteers in environmental and hydrogeological monitoring.
Researchers also trained students on the required activities for the project, to be carried out remotely. The training phase involved: (1) observation of images through open-source software GIMP; (2) recording observations on a spreadsheet shared in the cloud. The meetings were held over 4 months—from January to April 2022. At the end of each workshop, the researchers asked participants to provide email addresses in order to: (1) identify and involve in the project only people interested to participate voluntarily; (2) share the material (images to observe and tutorials) to carry out the required activities remotely; (3) share research progress and results; (4) support the project through communication campaigns. The engagement methodology was designed to test and verify the voluntary character of the project. No monetary awards, university credits, nor other types of benefits were provided to students.
The data collection activity consisted of a stage of direct observations aimed at analyzing a sequence of continuous images related to a given upstream basin site. Volunteers were asked to examine sequences of 30 images during a rainfall event. The purpose was to estimate variations in streamflow levels. Subsequent replication observation stages were planned to cross-test the validity of the methodological procedure, volunteers' capabilities, and their interests to the project in the short term.
At the end of observation stage, the results are shared by a dedicated web interface and dashboard which allowed participants to visualize their observed data and project outcomes.
3.3. Data
The firsthand experimental test was based exclusively on direct observations of volunteers to assess their reliability by means of positioning, variability measures, and error estimation. Our research team developed a specific and controlled protocol of procedures to guarantee the effectiveness and validity of data collection, and to reduce uncertainty resulting from subjective perceptions. During the in-person workshops, we instructed volunteers on the procedure to acquire controlled data and on how to share it with researchers. In this regard, each volunteer received an online folder with the materials needed to perform the required tasks: (1) subfolders with images to analyze; (2) a table to report observations; and (3) tutorials with guided steps to carry out the tasks.
After visualizing image sequences, volunteers filled a shared spreadsheet to report the value of the coordinate Y—at the point of intersection between the water level and the reference pole—to estimate changes in water levels (Figure 3). To derive these water level estimates, absolute reference values were established:
• Fixed pole reference value [pixel]
• Pole length [mm]
• Pole length [pixel]
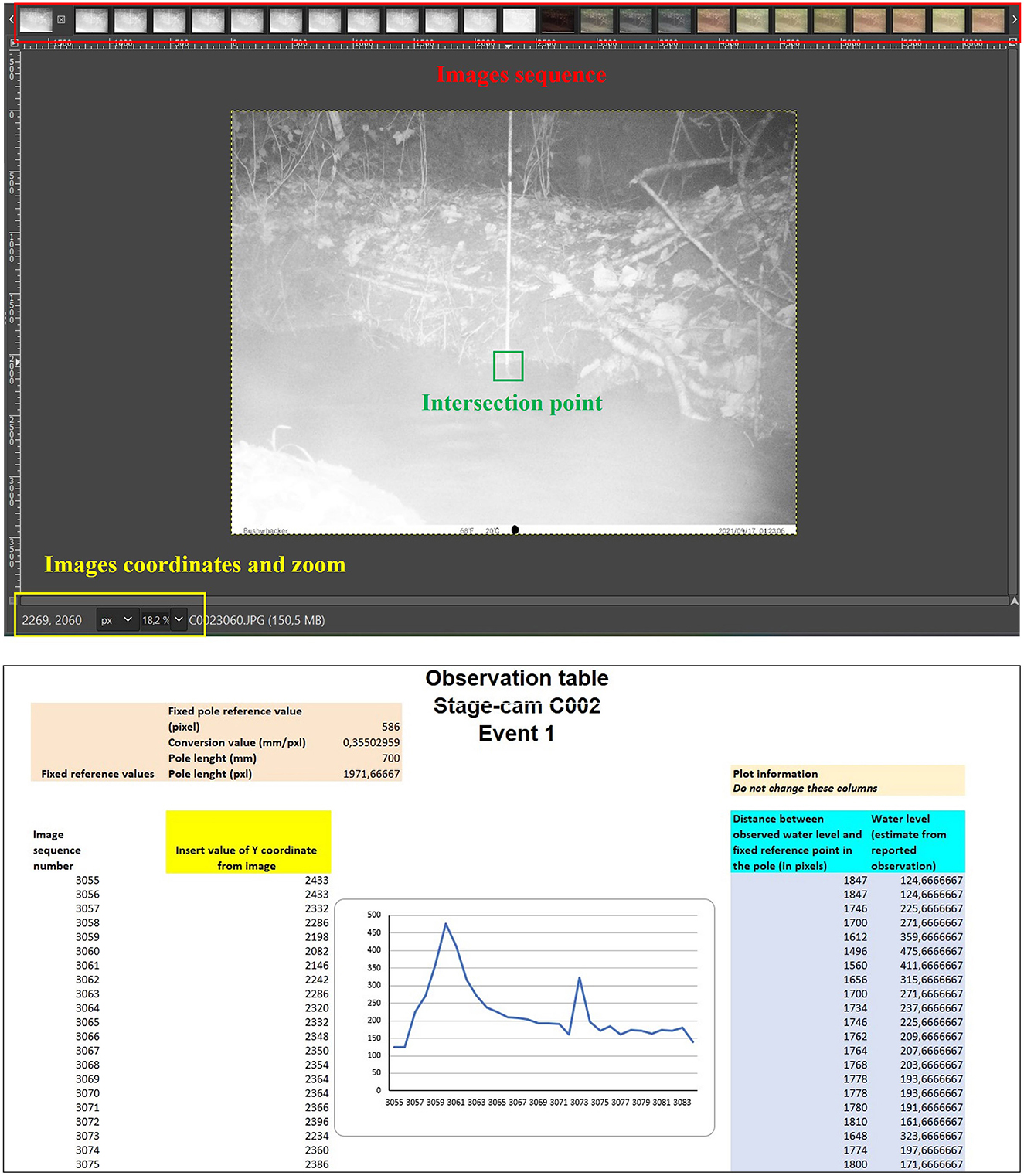
Figure 3. Working environment for data collection and observations recording: (upper figure) A screenshot from GIMP displays the time series of 30 images related to stream water levels; (lower figure) The observation table shows values provided from image visualization by volunteers.
Then, we derived the difference between the observed water level and the fixed reference point on the pre-installed white pole. This value, subtracted from the length of the observation pole, provided the estimate of water levels for each of the 30 images (Figure 3).
4. Results
In a recent study, Capdevila et al. (2020) points out how citizens' attributes can constitute potential success factors of improved data quantity and quality utilizing citizen science. These citizen attributes include: (1) knowledge and experience; (2) awareness of environmental issues; and (3) socio-economic backgrounds of citizens (Capdevila et al., 2020). Starting from this theoretical framework scheme, we created a preliminary survey for participants after the initial engagement step. Since this sample was limited to students from the university community, qualitative information collection was focused on: (1) educational level; (2) university career background; and (3) non-academic environmental expertise to relate the data collection activity to specific socio-cultural patterns (Figure 4).
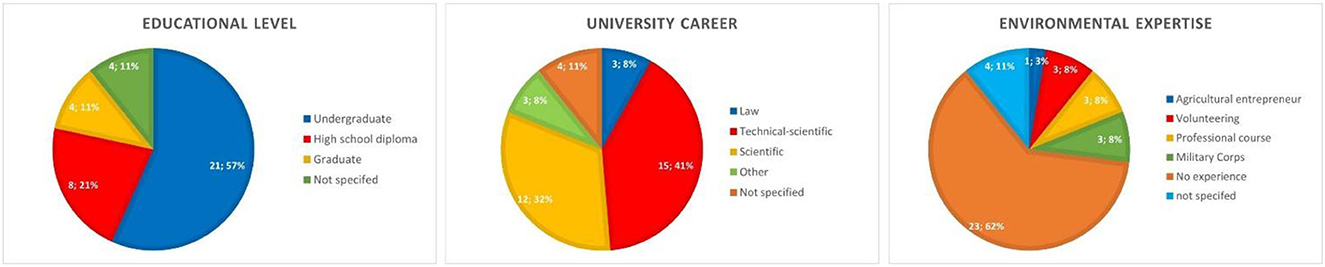
Figure 4. Qualitative attributes of volunteers engaged in active participation: (left panel) educational level; (center panel) university career path; (right panel) environmental expertise.
Figure 4 suggests that the collected sample is quite heterogeneous. Indeed, volunteer academic backgrounds span from humanities to engineering, and from high school to graduate-level programs. Most students did not have any expertise or prior background in similar environmental topics.
4.1. Insights from observation stage
We analyzed the observations of 37 student volunteers (our maximum number of active volunteers). Figure 5 summarizes the variation of water levels from the collected volunteer observations. The boxplot depicts the confidence intervals and positioning trends for each photo-trap image.
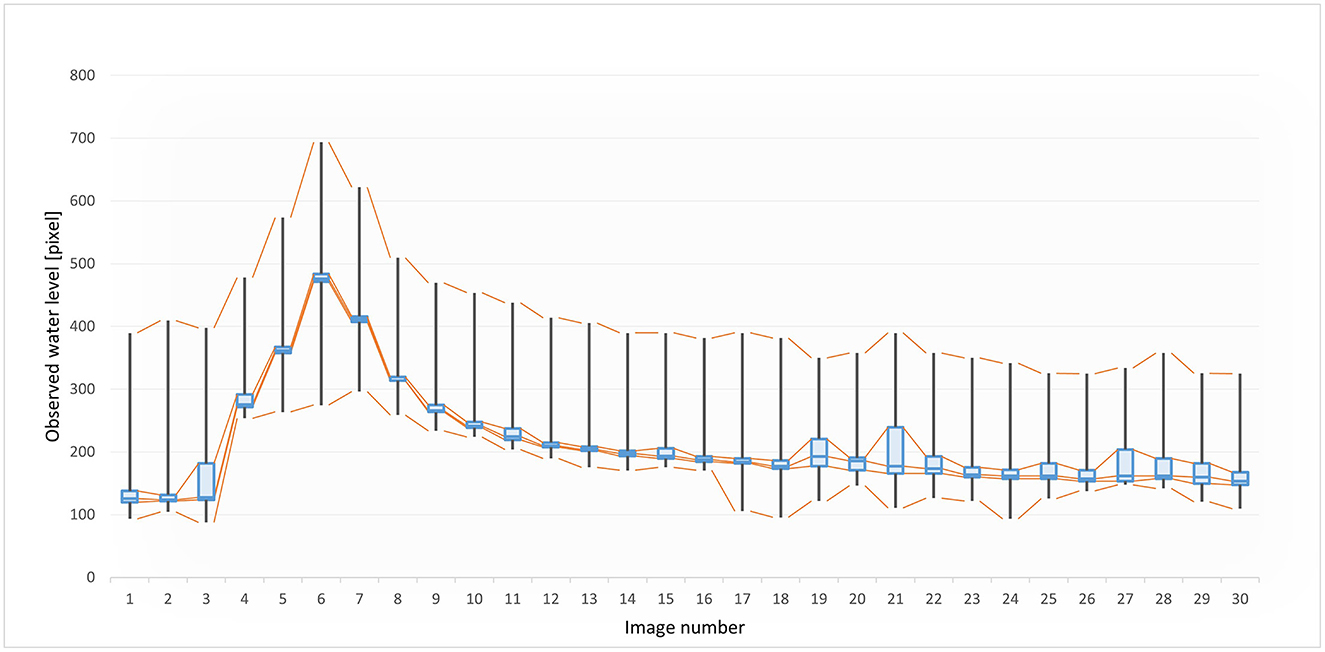
Figure 5. Variation of observed water levels at the initial observation stage. A total of 37 student volunteers took part in this stage.
Results indicate a high variability of the student observations, despite a strong concentration around the median values for each analyzed image. To better interpret these trends, we estimated the MAE and the MARE of each student volunteer and compared each observation with a benchmark value. The benchmark value was derived from the observations provided by an expert from our research team. Furthermore, researchers estimated the mean absolute error for each volunteer to see how far each observation deviated from the benchmark value.
Finally, we estimated the average mean absolute error of all volunteers which was equal to 24.5 pixels. This average value was, however, within a wide confidence interval of errors between 3.6 and 201.9 pixels, where the median value was 16.7 pixels.
The average MARE recorded in this observation stage was equal to 0.14 (14%). Average MARE is a measurement to evaluate the performance of all participating volunteers on the single event.
The sample of volunteers active in the observation stage presented heterogeneous qualitative attributes in terms of education, university career background, and environmental expertise. Sample heterogeneity prompted to verify possible impacts of the qualitative attributes on the volunteers' performances. Figure 6 shows the distribution of the absolute mean error for each category within groups (1) educational level, (2) university career path, and (3) environmental expertise. Interestingly, there is counterintuitive evidence, indeed, that the lowest mean absolute error (16.2 pixels) was provided by undergraduate volunteers while the highest values are recorded among those who have a master's degree (MAE = 64.6 pixels). Similarly, it was not expected that, considering university career paths, that the lowest error (10.7 pixels) is provided by those who follow a career path in law disciplines. No considerable differences were reported related to environmental expertise. In fact, the difference in MAE between the environmental expertise group and the no-expertise group was 5.3 pixels. These results, however, do not reflect the specific trend of each individual group, but rather the distribution of outlier values among individual volunteers linked to personal biases and uncertainties.
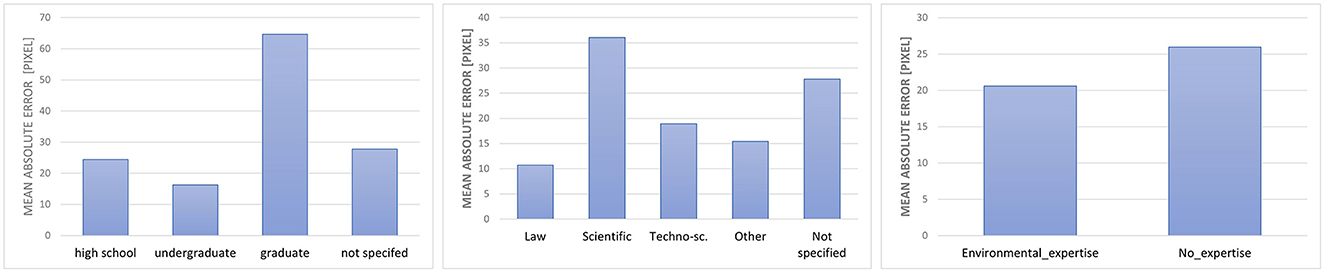
Figure 6. Mean absolute error (MAE) in initial observation stage sorted by qualitative attributes of volunteers: (left panel) educational level; (center panel) university career path; (right panel) environmental expertise.
Personal biases were also confirmed in Figure 7. The continuous distribution (Y-axis) of the stream-water level is uneven due to the uncertainties recorded for each image (X-axis) by each student volunteer (Z-axis) in the observation stage of the study.
4.2. Preliminary insights from replication stages
We replicated the methodological procedure for estimating stream water levels and extended the observation activity of the student volunteers to two additional replication stages. The replication of observations had four main purposes: (1) increase the amount of data collected to obtain more significant estimates; (2) test the validity of the methodological procedure; (3) test the motivations of volunteers; and (4) evaluate any variation in volunteer performance over time. Furthermore, we asked the 37 participants to observe a second continuous series of 30 images of a downstream site during the same rainfall event. Finally, researchers asked student volunteers to examine a third continuous series of 30 images relative to the initial upstream site during a different rainfall event.
During the replication stages a drastic drop in participants was recorded, undermining the initial goals of this study. Only five volunteers replicated the observation activities of the water flow image series.
The secondary results provided an indicative insight into volunteers' performance and demonstrated the replicability of the methodological procedure designed for estimating stream water levels during a flood event. The limitation of the secondary sample size to only five observations did not permit analysis of these observations. Regardless, the results reported below showed the potential effectiveness of the proposed methodological procedure. The secondary results, which emerged from the small but motivated group of active student volunteers, showed promising variations—from the reduction of both the average MAE and the average MARE indices from the first stage of observations.
In the replication stage, the recorded average MAE among five volunteers also active in replication stages was equal to 120.2 pixels within a range between a minimum value of 12.4 pixels and maximum of 221.4 pixels. Compared to the observation stage, the average MARE increased to 25%. Regardless, focusing our attention on only the five consistently active volunteers, we noted a substantial improvement in performance across the first two phases of the study. Among the five consistently active volunteers, an average MARE equal to 28% at the first observation stage was recorded.
The same five volunteers completed the second replications stage and demonstrated strong interest to contribute to the citizen science experiment. In the final replication stage of the study, the average MAE was equal to 25.29 pixels, and the average MARE significantly decreased to 6%.
The MAE and MARE indices were useful in interpreting the performance of volunteers to better understand, if during the different stages of the study, volunteers had acquired a greater ability to provide reliable observations. Outcomes from MAE and MARE pointed out a decreasing margin of error in the performances of five consistently volunteers across the three observation stages. The decreasing trend is also confirmed by the error distribution within volunteers (Figure 8), where recorded error was attributable primarily to one participant.
The limited sample size does not permit generalization of the effective changes recorded in the observation skills of volunteers. Furthermore, the small number of volunteers does not allow us to fully understand the possible impacts of the training activities across the three different stages of the study. However, the convergence of observed values around the median values, especially in the final replication stage, suggests that the repetition of simple tasks, by small but motivated groups, can lead to an improvement in volunteer performance.
5. Discussion
In this study concepts and methods of citizen science were empirically tested to improve innovative procedures for hydrological monitoring. The active participation of 131 volunteers, among the large number of students who participated in the in-person initial workshop (around 400 people), addressed the researcher's decision to work remotely with the support of web-based cloud technologies to permit the collection of a large set of observations.
Student engagement in the role of observers was purely based on volunteer assistance. No incentives or remuneration was provided to support or motivate the participation of volunteers. They did, however, gain a sense of engagement and learned about selected study methods during the activity. Our aim was to analyze the student participation and to understand—also at an indicative level—the possible motivations that encouraged student volunteers to take an active part in a scientific research process.
The choice of voluntary participation obviously entailed risks that limited the dynamics of participation. Considering the initial group of 131 participants, in fact, only 37 volunteers contributed to the further development of the project by successfully performing the first observation stage test. In the replication stages, we found a drastic drop in participation, where only five volunteers contributed.
The difference between active and passive volunteers is a common issue within online communities. The active participation of 37 out of 131 volunteers can be considered a satisfactory result. On the other hand, the drastic drop in participation during the replication is an aspect that need to be investigated. Authors hypothesize that this drop in participation is due to the following three factors: (1) repetitive tasks were requested; (2) there was a gap between communication and organizational phases of the project; and (3) the participants lacked substantial interest and motivation over time. At the present, these are only hypotheses and further investigations are required for future work on how to increase and maintain volunteers' engagement over time.
From available data indicative insights on the background of participating student volunteers can be derived. Among the 37 active participants, 25 volunteers declared to have a university degree (21 bachelor's degrees and four master's degrees). However, this is not particularly significant in a sample limited to university students. On the other hand, more relevant indications can be derived from the declared university career background. Most participants (41%) stated they were on a technical-scientific career path, and 32% were on a scientific pathway.
A higher educational level, combined with a disciplinary affinity with the hydrological monitoring study, seems to be a logical reason for active and lasting participation of a volunteer. This contrasts with other types of university careers that do not have an affinity with hydrological monitoring and could explain why only five students participated in the two replication stages of the research. One unexpected behavior has to do with volunteers with environmental expertise. The lack of environmental expertise does not seem to have a major impact on participation in the project. Of the 37 volunteers, a smaller percentage (38%) stated that their environmental experiences were obtained primarily from service in military corps activities related to environmental issues. Other students stated their environmental experiences were gained through job-related purposes. This is reasonable since students are still undergoing academic and professional training. In the light of the experiment carried out, there is, therefore, no common matrix that justifies long-term participation. However, there are indications that require further investigation. Further input derived from a follow-up questionnaire, which focuses on the analysis of motivations of student volunteers.
At the end of our experimental citizen science test, research team asked student participants which of the following motivations led them to participate in the project: (1) interest in environmental issues; (2) scientific curiosity; (3) personal satisfaction; (4) desire to be part of a scientific community; (4) sense of duty; (5) contributing to scientific research; (6) opportunity to improve knowledge and skills; or (7) environmental sensitivity. The replies received focused on three primary aspects: (a) interest in environmental issues; (b) contributing to scientific research; and (c) opportunity to improve knowledge and skills. The volunteers unanimously stated that they were very satisfied with the experience gained and moderately satisfied of their improved knowledge and skills. They also agreed that their contribution has been useful for scientific research. However, student volunteers stated that their level of awareness regarding environmental issues remained unchanged.
What is evident from the initial observations is the constant participation of a small but motivated group of volunteers (total = 5) in all stages of the project. Another aspect is the reliability of the observations and the trends in performance, as attested by the average MARE index equal to 0.14 in the peak of total participants (37 volunteers). The general convergence of water level estimations from volunteer observations, and the relative low average MARE index (0.14), are probably affected by training. This allowed each volunteer to replicate autonomously and remotely the methodological designed for data acquisition through direct observations. Therefore, it appears that the training allowed, albeit in a limited and restricted sample, the replicability of the methodological procedure of data acquisition. This was achieved through direct observation of a continuous series of images, relative to different sites and rainfall events, during the replication stages. The average MARE index, equal to 0.06 obtained in the last replication stage, is an encouraging sign—albeit it was obtained from a very small sample.
In general, we have not found incidences of qualitative variables related to the backgrounds of student volunteers, but rather the results are conditioned by outlier values resulting from the subjectivity of the single observer. However, we do not have a significant sample size to provide more detailed assessments of the incidence of qualitative variables. This aspect of the research identified a general improvement in volunteer performance due to the use of simple, repetitive tasks.
The data used in this study are not included into the category of User-Generated Contents (UGC), as they were not released through social media or digital platforms (Zheng et al., 2018; See, 2019; Sy et al., 2019; Modaresnezhad et al., 2020). Rather, data was acquired using crowdsourcing methods according to precise protocols set in the research design phase of the study (Brabham, 2008; Estellés-Arolas and González-Ladrón-De-Guevara, 2012; Palacios et al., 2016; Lowry et al., 2019; Martinez-Corral et al., 2019).
Our data collection activity conforms to the contributory typology of citizen science (Bonney et al., 2016; Aristeidou et al., 2017; Gray et al., 2017). The contributory approach indicates that research questions and research design adapt to a top-down research process coordinated by expert scientists. The role of the volunteer is limited to interpretation of guided data collection. Subsequently, data processing and analysis therefore remain with the competence of the experts.
Volunteers' engagement, however, is applied to the dissemination of results, with the support of digital dashboards, to increase knowledge and awareness on issues related to environmental management and monitoring, and awareness of sustainability and conflict reduction related to the improved use of water resources. Public engagement during information dissemination is also important because it will highlight the role of the volunteers in the formulation of scientific knowledge. This is useful not only for research but also for setting decision-making scenarios for the public to be included, informed, and active participants in the process.
6. Conclusions
Our research evaluated some principles of citizen science, to obtain estimates of water levels in an ephemeral stream, through visual image analysis. In this regard, we designed a procedural protocol for the validation of volunteer observations. The procedure was assessed on the Montecalvello river basin, but it is replicable in other geographic settings. To evaluate the validity of the research methodology, we relied on a sample of university students from the Tuscia University community to establish a controlled group of volunteers.
The MAE and MARE of volunteer observations yields satisfactory and promising results in terms of the reliability of data from volunteered observations. The average MAE of 24.5 pixels implies that the overall error margin recorded by the volunteers is 70 mm (1 mm = 0.35 pixel). The smallest recorded MAE of 3.57 pixels implies that the margin of error obtained is 10.2 mm. Furthermore, the data shows a decrease in the margin of error from the first to the last phase, indicating how training and replicability can affect the improved validity of the data obtained.
The application of the pilot case has been useful to highlight issues and limits related to the effective participation of volunteers and the reliability of their observations. The adoption of communication techniques, aimed at encouraging participation and the application of the test on a controlled sample, however, affected the activity of the volunteers. The main issue encountered during this work was the recruitment and engagement of student volunteers. We found that it was difficult to maintain high and constant motivation of volunteers since they did not appear to be motivated—in terms of material gain—in the scientific research activity. Continuous communication campaigns to update and encourage participation in the various phases also had very limited effects.
As a result, this research assesses the difficulty of establishing stable and lasting volunteer communities for citizen science projects, regardless of whether they are in-person or virtual online communities. In our study, we experimented a mixed mode of approaches in which, at the initial phases of in-person recruitment through classroom workshops, we followed up with activities through online methods. This leads us to conclude that a greater effectiveness of citizen science projects can be ensured by temporally limited activities which are addressed to limited groups of participants who are strongly motivated by common interests, and personal or collective appreciation for the proposed research topics. In our study we adopted a contributory approach, where research questions and methodologies followed a top-down model. Approaches related to other types of citizen science—such as collaborated or co-created—could provide different indications of the motivations that support volunteer participation. Regardless, a scientific research activity established on a bottom-up approach would imply a greater level of participation and involvement but could not be established in our specific study.
Data availability statement
The raw data supporting the conclusions of this article will be made available by the authors, without undue reservation.
Ethics statement
Written informed consent from the participants was not required to participate in this study in accordance with the national legislation and the institutional requirements.
Author contributions
AS gave substantial contributions to the conception or design of the work, data interpretation, and drafted the work. FN and SG revised critically the manuscript and agree to be accountable for all aspects of the work. SN gave substantial contribution to the work design and data acquisition. AB provided approval for publication of contents. All authors contributed to the article and approved the submitted version.
Funding
The financial contribution of PROLOGIS applied research project (Grant agreement no. 7209) to the WARREDOC University for Foreigners of Perugia is gratefully acknowledged in support of this publication.
Acknowledgments
Authors would like to extend their sincere thanks to Tom Cech, formerly Director of One World One Water Center at Metropolitan State University of Denver, for his efforts in mother tongue proof editing work. Special acknowledgments to students from University of Tuscia engaged in this firsthand citizen science experience. Their efforts in volunteered data collection activities were essential for this research contribution.
Conflict of interest
The authors declare that the research was conducted in the absence of any commercial or financial relationships that could be construed as a potential conflict of interest.
Publisher's note
All claims expressed in this article are solely those of the authors and do not necessarily represent those of their affiliated organizations, or those of the publisher, the editors and the reviewers. Any product that may be evaluated in this article, or claim that may be made by its manufacturer, is not guaranteed or endorsed by the publisher.
References
Annis, A., and Nardi, F. (2019). Integrating VGI and 2D hydraulic models into a data assimilation framework for real time flood forecasting and mapping. Geo Spat. Inform. Sci. 22, 223–236. doi: 10.1080/10095020.2019.1626135
Annis, A., Nardi, F., and Castelli, F. (2022). Simultaneous assimilation of water levels from river gauges and satellite flood maps for near-real-time flood mapping. Hydrol. Earth Syst. Sci. 26, 1019–1041. doi: 10.5194/hess-26-1019-2022
Aristeidou, M., Scanlon, E., and Sharples, M. (2017). Profiles of engagement in online communities of citizen science participation. Comput. Human. Behav. 74, 246–256. doi: 10.1016/j.chb.2017.04.044
Assumpção, T. H., Popescu, I., Jonoski, A., and Solomatine, D. P. (2018). Citizen observations contributing to flood modelling: Opportunities and challenges. Hydrol. Earth Syst. Sci. 22, 1473–1489. doi: 10.5194/hess-22-1473-2018
Blöschl, G., Bierkens, M. F. P., Chamble, A., Cudennec, C., Destouni, G., and Fiori, A. (2019). Twenty-three unsolved problems in hydrology (UPH)—A community perspective. Hydrol. Sci. J. 64, 1141–1158. doi: 10.1080/02626667.2019.1620507
Bonney, R., Cooper, C. B., Dickinson, J., Kelling, S., Phillips, T., Rosenberg, K. V., et al. (2009). Citizen science: A developing tool for expanding science knowledge and scientific literacy. BioScience 59, 977–984. doi: 10.1525/bio.2009.59.11.9
Bonney, R., Phillips, T., Ballard, H. L., and Enck, J. W. (2016). Can citizen science enhance public understanding of science? Publ. Understand. Sci. 25, 2–16. doi: 10.1177/0963662515607406
Brabham, D. C. (2008). Crowdsourcing as a model for problem solving: An introduction and cases. Convergence 14, 75–90. doi: 10.1177/1354856507084420
Buytaert, W., Zulkafli, Z., Grainger, S., Acosta, L., Alemie, C., Bastiaensen, J., et al. (2014). Citizen science in hydrology and water resources: Opportunities for knowledge generation, ecosystem service management, and sustainable development. Front. Earth Sci. 2, 26. doi: 10.3389/feart.2014.00026
Capdevila, S. L., Kokimova, A., Sinha Ray, S., Avellán, T., Kim, J., and Kirschke, S. (2020). Success factors for citizen science projects in water quality monitoring. Sci. Tot. Environ. 728, 137843. doi: 10.1016/j.scitotenv.2020.137843
Cappa, F., Laut, J., Nov, O., Giustiniano, L., and Porfiri, M. (2016). Activating social strategies: Face-to-face interaction in technology-mediated citizen science. J. Environ. Manage. 182, 374–384. doi: 10.1016/j.jenvman.2016.07.092
Davids, J. C., Van de Giesen, N., and Rutten, M. (2017). Continuity vs. the crowd—tradeoffs between continuous and intermittent citizen hydrology streamflow observations. Environ. Manag. 60, 12–29. doi: 10.1007/s00267-017-0872-x
Dickinson, J. L., Shirk, J., Bonter, D., Bonney, R., Crain, R. L., Martin, J., et al. (2012). The current state of citizen science as a tool for ecological research and public engagement. Front. Ecol. Environ. 10, 291–297. doi: 10.1890/110236
Eitzel, M., Cappadonna, J., Santos-Lang, C., Duerr, R. E., Virapongse, A., West, S. E., et al. (2017). Citizen science terminology matters: Exploring key terms. Citizen Sci. 2, 1. doi: 10.5334/cstp.96
Elwood, S., Goodchild, M. F., and Sui, D. Z. (2012). Researching volunteered geographic information: Spatial data, geographic research, and new social practice. Ann. Assoc. Am. Geogr. 102, 571–590. doi: 10.1080/00045608.2011.595657
Estellés-Arolas, E., and González-Ladrón-De-Guevara, F. (2012). Towards an integrated crowdsourcing definition. J. Inform. Sci. 38, 189–200. doi: 10.1177/0165551512437638
Etter, S., Strobl, B., Seibert, J., and Ilja Van Meerveld, H. J. (2020). Value of crowd-based water level class observations for hydrological model calibration. Water Resour. Res. 56, 1–17. doi: 10.1029/2019WR026108
Fienen, M. N., and Lowry, C. S. (2012). Social Water-A crowdsourcing tool for environmental data acquisition. Comput. Geosci. 15, 164–169. doi: 10.1016/j.cageo.2012.06.015
Goodchild, M. F. (2007). Citizens as sensors: The world of volunteered geography. GeoJournal, 2007, 211–221. doi: 10.1007/s10708-007-9111-y
Gray, S., Jordan, R., Crall, A., Newman, G., Hmelo-Silver, C., Huang, J., et al. (2017). Combining participatory modelling and citizen science to support volunteer conservation action. Biol. Conserv. 37, 76–86. doi: 10.1016/j.biocon.2016.07.037
Ilja Van Meerveld, H. J., Vis, M. J. P., and Seibert, J. (2017). Information content of stream level class data for hydrological model calibration. Hydrol. Earth Syst. Sci. 21, 4895–4905. doi: 10.5194/hess-21-4895-2017
Jordan, R., Crall, A., Gray, S., Phillips, T., and Mellor, D. (2015). Citizen science as a distinct field of inquiry. BioScience 65, 208–211. doi: 10.1093/biosci/biu217
Knapp, C. N., Reid, R. S., Fernandes-Gimenez, M. E., Klein, J. A., and Galvin, K. A. (2019). Placing transdisciplinarity in context: A review of approaches to connect scholars, society and action. Sustainability 11, 18. doi: 10.3390/su11184899
Kosmala, M., Wiggings, A., Swanson, A., and Simmons, B. (2016). Assessing data quality in citizen science. Front. Ecol. Environ. 14, 551–560. doi: 10.1002/fee.1436
Le Coz, J., Patalano, A., Collins, D., Guillén, N. F., García, C. M., Smart, G. M., et al. (2016). Crowdsourced data for flood hydrology: Feedback from recent citizen science projects in Argentina, France and New Zealand. J. Hydrol. 36, 766–777. doi: 10.1016/j.jhydrol.2016.07.036
Lowry, C. S., Fienen, M., Hall, D. M., and Stepenuck, K. F. (2019). Growing pains of crowdsourced stream stage monitoring using mobile phones: The development of crowd hydrology. Front. Earth Sci. 7, 128. doi: 10.3389/feart.2019.00128
Lowry, C. S., and Fienen, M. N. (2013). CrowdHydrology: Crowdsourcing hydrologic data and engaging citizen scientists. GroundWater 51, 151–156. doi: 10.1111/j.1745-6584.2012.00956.x
Martinez-Corral, A., Grijalvo, M., and Palacios, M. (2019). An organisational framework for analysis of crowdsourcing initiatives. Int. J. Enterpreneur. Behav. Res. 25, 1652–1670. doi: 10.1108/IJEBR-06-2018-0402
Modaresnezhad, M., Iyer, L., Palvia, P., and Taras, V. (2020). Information Technology (IT) enabled crowdsourcing: A conceptual framework. Inform. Process. Manag. 57, 2. doi: 10.1016/j.ipm.2019.102135
Nardi, F., Cudennec, C., Abrate, T., Annis, A., Assumpção, T. H., Aubert, A. H., et al. (2021). Citizens AND HYdrology (CANDHY): Conceptualizing a transdisciplinary framework for citizen science addressing hydrological challenges. Hydrol. Sci. J. 2021, 528. doi: 10.5194/iahs2022-528
Njue, N., Stenfert Kroese, J., Gräf, J., Jacobs, S. R., Weeser, B., Breuer, L., et al. (2019). Citizen science in hydrological monitoring and ecosystem services management: State of the art and future prospects. Sci. Tot. Environ. 693, 337. doi: 10.1016/j.scitotenv.2019.07.337
Noto, S., Tauro, F., Petroselli, A., Apollonio, C., Botter, G., and Grimaldi, S. (2022). Low-cost stage-camera system for continuous water-level monitoring in ephemeral streams. Hydrol. Sci. J. 67, 9. doi: 10.1080/02626667.2022.2079415
Palacios, M., Martinez-Corral, A., Nisar, A., and Grijalvo, M. (2016). Crowdsourcing and organizational forms: Emerging trends and research implications. J. Bus. Res. 69, 1834–1839. doi: 10.1016/j.jbusres.2015.10.065
Phillips, T. B., Ballard, H. L., Lewenstein, B. V., and Bonney, R. (2019). Engagement in science through citizen science: Moving beyond data collection. Sci. Educ. 103, 665–690. doi: 10.1002/sce.21501
Schrögel, P., and Kolleck, A. (2019). The many faces of participation in science: Literature review and proposal for a three-dimensional framework. Sci. Technol. Stud. 32, 77–99. doi: 10.23987/sts.59519
See, L. (2019). A review of citizen science and crowdsourcing in applications of pluvial flooding. Front. Earth Sci. 7, 44. doi: 10.3389/feart.2019.00044
See, L., Comber, A., Salk, C., Fritz, S., van der Velde, M., Perger, C., et al. (2013). Comparing the quality of crowdsourced data contributed by expert and non-experts. PLoS ONE. 8:e69958. doi: 10.1371/journal.pone.0069958
See, L., Mooney, P., Foody, G., Bastin, L., Comber, A., Estima, J., et al. (2016). Crowdsourcing, citizen science or volunteered geographic information? The current state of crowdsourced geographic information. ISPRS Int. J. Geo-Inform. 5, 5. doi: 10.3390/ijgi5050055
Seibert, J., Strobl, B., Etter, S., Hummer, P., and Ilja Van Meerveld, H. J. (2019). Virtual staff gauges for crowd-based stream level observations. Front. Earth Sci. 7, 1–10. doi: 10.3389/feart.2019.00070
Spagnoletti, P., Resca, A., and Lee, G. (2015). A design theory for digital platforms supporting online communities: A multiple case study. J. Inform. Technol. 30, 364–380. doi: 10.1057/jit.2014.37
Spasiano, A., Grimaldi, S., Braccini, A. M., and Nardi, F. (2021). Towards a transdisciplinary theoretical framework of citizen science: Results from a meta-review analysis. Sustainability 13, 7904. doi: 10.3390/su13147904
Spasiano, A., Nardi, F., Grimaldi, S., and Braccini, A. M. (2022). “Engagement of online communities within a citizen science framework for improving innovative participation models: Insights from hydrology and environmental monitoring,” in Exploring Digital Resilience. ItAIS 2021. Lecture Notes in Information Systems and Organisation, Vol. 57, eds R. Cuel, D. Ponte, and F. Virili (Cham: Springer), 41–54. doi: 10.1007/978-3-031-10902-7_4
Starkey, E., Parkin, G., Birkinshaw, S., Large, A., Quinn, P., and Gibson, C. (2017). Demonstrating the value of community-based (“citizen science”) observations for catchment modelling and characterization. J. Hydrol. 19, 801–817. doi: 10.1016/j.jhydrol.2017.03.019
Strobl, B., Etter, S., Ilja Van Meerveld, H. J., and Seibert, J. (2020). Accuracy of crowdsourced streamflow and stream level class estimates. Hydrol. Sci. J. 65, 823–841. doi: 10.1080/02626667.2019.1578966
Sy, B., Frischknecht, C., Dao, H., Consuegra, D., and Giuliani, G. (2019). Flood hazard assessment and the role of citizen science. J. Flood Risk Manag. 12, 519. doi: 10.1111/jfr3.12519
Thornhill, I., Loiselle, S., Clymans, W., and Van Noordwijk, C. G. E. (2019). How citizen scientists can enrich freshwater science as contributors, collaborators, and co-creators. Freshw. Sci. 38, 231–235. doi: 10.1086/703378
Walker, D. W., Smigaj, M., and Tani, M. (2021). The benefits and negative impacts of citizen science applications to water as experienced by participants and communities. Wiley Interdiscipl. Rev. 8, 1–32. doi: 10.1002/wat2.1488
Weeser, B., Stenfert Kroese, J., Jacobs, S. R., Njue, N., Kemboi, Z., Ran, A., et al. (2018). Citizen science pioneers in Kenya – A crowdsourced approach for hydrological monitoring. Sci. Tot. Environ. 631, 1590–1599. doi: 10.1016/j.scitotenv.2018.03.130
Keywords: citizen science, water level estimation, crowdsourced data, image analysis, ephemeral rivers, stage-cam
Citation: Spasiano A, Grimaldi S, Nardi F, Noto S and Braccini AM (2023) Testing the theoretical principles of citizen science in monitoring stream water levels through photo-trap frames. Front. Water 5:1050378. doi: 10.3389/frwa.2023.1050378
Received: 21 September 2022; Accepted: 09 January 2023;
Published: 30 January 2023.
Edited by:
Alessandro Pagano, Istituto di Ricerca Sulle Acque IRSA-CNR, ItalyReviewed by:
David Hill, Thompson Rivers University, CanadaNico Valentini, Bureau de Recherches Géologiques et Minières, France
Copyright © 2023 Spasiano, Grimaldi, Nardi, Noto and Braccini. This is an open-access article distributed under the terms of the Creative Commons Attribution License (CC BY). The use, distribution or reproduction in other forums is permitted, provided the original author(s) and the copyright owner(s) are credited and that the original publication in this journal is cited, in accordance with accepted academic practice. No use, distribution or reproduction is permitted which does not comply with these terms.
*Correspondence: Andrea Spasiano, andrea.spasiano@unistrapg.it