- Agricultural Economics, Agricultural College, Shiraz University, Shiraz, Iran
To effectively tackle the issue of increasing irrigation water scarcity, farmers need to convert to modern irrigation systems with lower water use while achieving higher yields and profitability. Unlike the government support to cover a proportion of irrigation modernizing costs by public subsidies in Iran, the adoption rate is low. This is due to farmers' uncertainty about the trade-off between benefits from yield improvement and the added production costs. The historical gross margin of barley, wheat, forage corn, and tomato under surface, drip, permanent sprinkler, and semi-permanent sprinkler irrigation systems was generated using simulation and survey-based data for yield and published data for costs and prices over a 5-year period (2009–2015). The stochastic dominance (SD) and stochastic efficiency with respect to a function (SERF) approaches were used to evaluate the risk efficiency of various irrigation systems for main crops in the Bakhtegan Basin. Estimating certainty equivalent (CE), we ranked irrigation alternatives at different absolute risk-aversion coefficient (ra) levels. The findings show that drip irrigation systems for forage corn and tomato have higher CE values at all levels of absolute risk-aversion coefficient; however, the preferred system for barley and wheat varies with ra. Moreover, estimated risk premiums revealed that risk-neutral farmers would pay to move from surface systems to more efficient systems, whereas risk-averse farmers need to be paid to have the tendency to change their irrigation system. The important policy implication of these results is that risk premiums can consider justifying subsidy allocation in a manner that induces farmers to more risk-efficient irrigation systems.
Introduction
Irrigated agriculture is crucial in fulfilling future food demands, particularly in arid and semi-arid regions (Daccache et al., 2014, 2015; Okyere and Usman, 2021). Water supply uncertainty rises since agriculture's vulnerability increases due to the negative effects of climate change on agriculture (Zabel et al., 2014; Khor and Feike, 2017; Imran et al., 2019; Siyal et al., 2021). To effectively tackle the issue of increasing irrigation water scarcity, farmers need greater flexibility and reliability in their irrigation systems (Mukherji et al., 2009; Yang et al., 2020). Thus, investing in water-saving technologies and replacing less efficient irrigation systems with pressurized ones, namely, sprinkler and drip systems, have received a great attention as a common response to the limited water supply for reducing water use and achieving higher yields and profitability (Espinosa-Tasón et al., 2020; Jing et al., 2020; Krishnan et al., 2020).
Farmers' decisions about the adoption of modern irrigation systems are generally based on benefits expected from water conservation and costs associated with technology change, because capital investment, maintenance costs, system efficiency, and investment return are the most important criteria in the selection of an appropriate irrigation system (Srdjevic and Obradovic, 1997; Deveci et al., 2015; Balana et al., 2020). Therefore, adopting alternative irrigation methods by the farmers is associated with risks that may discourage adoption, especially among risk-averse individuals. Due to this challenge, governments in many regions, namely, the United States, Spain, Mediterranean, and Asian countries, cover a proportion of the irrigation modernizing costs by public subsidies to make incentives for farmers and promote the adoption of water-conserving on-farm irrigation technologies (Scheierling et al., 2006).
Iran, which is located in an arid and semi-arid region of the world, has experienced less rainfall, rising temperatures, more frequent extreme weather events, and straining water supplies even further (Modarres et al., 2016; Karimi et al., 2018). According to the third national communication to UNFCCC in 2014, in the next decades, Iran will encounter a 2.6°C increase in average temperatures and a 35% decrease in precipitation (NCCOI, 2014). The potential effects of these two parameters have reduced the availability of total water in Iran from upward of 125 to 89 BCM on average (Mesgaran and Azadi, 2018). In this sense, the Iranian government has considered modernizing the irrigation systems as a priority in the 5-year development plans. For almost two decades, the parliament and the government have been supporting the promotion of these systems by allocating subsidies and gratuitous facilities. Accordingly, the government is going to pay up to 85% of the pressurized irrigation systems' expenses in the form of subsidies. Based on the budget allocated to the modernization project of the irrigation systems, the government paid 227.5 million Rials free subsidy per hectares to drip systems, 187.5 million Rials to center/linear sprinkler systems, and 115.5 million Rials to classical permanent and semi-permanent sprinkler systems in 2020 (Ministry of Agriculture Jihad, 2020). Despite the high supporting programs across the country, the implementation of these systems is still relatively low. During the past two decades, only a quarter of the total irrigated land in Iran, which is about 8.7 million hectares, has been equipped with modern irrigation at a rate of roughly 100,000 ha each year. This low adoption rate could be due to the difficulties which farmers may face because of the risks associated with modern irrigation techniques. Despite the increase in water-use efficiency, pressurized irrigation systems, particularly sprinkler, make a significant rise in energy consumption (García-Prats and Guillem-Picó, 2016; Zhao et al., 2020; Islam et al., 2021). The results of Liaghat (2012), a research project that analyzed energy consumption in pressurized irrigation systems in different regions of Iran, showed that the amount of energy consumption to extract one cubic meter of water in the Bakhtegan Basin, on average, is equivalent to 0.7 kWh of electricity for drip irrigation, 1.33 kWh for the classic permanent sprinkler, and 1.09 kWh for the semi-permanent sprinkler system. However, the amount of energy required to extract one cubic meter of water in surface irrigation systems is estimated as 0.4 kWh less than the drip system (Zhao et al., 2020), which is equivalent to 0.3 kWh in the Bakhtegan Basin.
According to the Law of Targeting Subsidies in Iran, rising energy prices, especially fossil fuels, have increased production costs. Therefore, increasing energy costs is a significant risk associated with modernizing irrigation systems. In contrast, many farmers are not sure about the definite impact of modern irrigation systems on the yield of some crops since, achieving the potential efficiencies of these systems as well as increasing product yields is also a management issue (Smith and Baillie, 2009). Hence, the trade-off between benefits from yield improvement and the added production costs caused by changing irrigation systems has a significant impact on the adoption decisions. Due to heterogeneity in farmers' risk preferences, investigating the distributions of net farm income from various options?, which were produced under generic utility function assumptions (Adusumilli et al., 2016, 2020), might aid in the establishment of incentives to encourage farmers to adopt more efficient irrigation systems. Therefore, the aim of this study is to evaluate the risk-efficiency of different kinds of modern irrigation systems for wheat, barley, forage corn, and tomato in the northern region of the Bakhtegan Basin. Estimating certainty equivalent (CE) and risk premiums at different absolute risk-aversion coefficient (ra) levels helps make policy guidelines for justifying subsidy allocation.
The novelty of the study is 3-fold. First, to the best of our knowledge, this is the first attempt to estimate the risk efficiency of alternative irrigation systems. Thus, the results may contribute to the literature in the development of the research design. Second, the analysis is based on a combination of simulation data and survey data which is a reliable way in the absence of experimental field data. The third contribution of this study is the application of stochastic efficiency with respect to a function (SERF). SERF analysis makes it possible to determine utility-efficient alternatives for different risk attitudes without access to accurate risk preferences.
Literature Review
Two categories of research were conducted on different alternatives related to irrigation systems. The first group has examined this issue with respect to the national interests and has used several criteria for comparison. In fact, these studies aimed to determine the influence of various socioeconomic and environmental criteria on irrigation system selection (Rodrigues et al., 2013). Studies in this category have mostly used a multi-criteria decision-making analysis for achieving their purposes. For example, Montazar and Behbahani (2007) developed a model for selecting optimized irrigation systems with three different crops of wheat, sugar beet, and grapes within the Ghazvin irrigation network in Iran, based on various criteria and parameters such as physical, socioeconomic, and environmental factors influencing system efficiency. They applied the analytic hierarchy process (AHP) to examine fifteen irrigation system variables and eight irrigation subsystem variables. In a more recent attempt, Veisi et al. (2022) used AHP to determine different stakeholders' views in the selection of an irrigation system according to its sustainability impact on agriculture in Ardebil Province of Iran. They considered three irrigation systems, namely, traditional irrigation, hydro flume irrigation, and pressurized irrigation. They concluded that all groups of stakeholders believed that pressurized irrigation systems are the most appropriate. Although these studies provide valuable results for the literature, farmers' risk preferences have been overlooked in these studies.
The second category of studies includes those which consider selecting irrigation strategies from the farmers' perspective. Bernardo (1988) used crop simulation and stochastic dominance (SD) method to evaluate the impact of irrigation system conformity on risk-efficient irrigation strategy selection. The analysis revealed that while risk aversion may explain some of the study-area irrigators' extensive irrigation practices, other considerations were found to influence irrigation scheduling decisions. Harris and Mapp (1986) and Epperson et al. (1992) applied SD and SDRF to compare water-saving irrigation strategies. Their considered strategies were full irrigation and different deficit irrigation strategies. Duenhas and Saad (2009) evaluated the economic viability of selecting three kinds of irrigation systems (drip, micro-irrigation, and traveling sprinkler) using Monte Carlo simulation and stochastic dominance for citrus orchards in Brazil. Paydar and Qureshi (2012) highlighted the growing uncertainty associated with implementing various irrigation management systems, as well as the absence of appropriate tools to evaluate various possibilities under uncertain conditions. The purpose of this study was to provide a risk-based strategy to water demand management and planning, as well as to apply “Modern Portfolio Theory” to handle climate-induced variability and uncertainty in the irrigation water supply.
The general conclusion that emerges from the literature review is that the adoption decision of irrigation systems is a risky choice. In contrast, studies on risk assessment of different irrigation systems are very few and scattered. This gap should be filled with more studies on different climatic conditions as well as more agricultural crops. Despite the obvious importance of financial information in deciding whether to use alternative irrigation options (Adusumilli et al., 2016), there is less information about their profitability prospects under risk conditions. Moreover, in examining alternative farming practices, risk analysis utilizing SERF has been frequently used in recent years (Özkan et al., 2015; Liu et al., 2017; Adusumilli et al., 2020; Fan et al., 2020). The SERF technique ranks the preference of decision-makers (DMs) according to the value of the CE of each alternative. The superiority of the SERF approach over the conventional SD methods, namely, SD and SDRF, is due to the use of the CE concept. Hardaker et al. (2015) used the estimated CE values as a baseline for the risk-aversion coefficient to rank efficient utility solutions. Accordingly, the SERF method can be effective in ranking different irrigation systems when considerations of risk are involved.
According to the literature review, there is a critical need to assist farmers' decision-making on appropriate irrigation systems, but there has been less effort to do so. This study attempts to fill in this gap by assessing the risk efficiency of different types of modern irrigation systems for four main crops in a semi-arid region. Another advantage of this study is that in the absence of experimental data on the net return of crops irrigated by various types of systems, we use available field data from a variety of sources, including published data as well as surveys with farmers and experts to construct historical data on gross margins for various types of irrigation systems. The data collection procedure is explained in Section Materials and Methods.
Materials and Methods
Data
Figure 1 shows the flowchart of analysis stages. To apply SD and SERF analysis, we need to construct historical data on gross margin for different kinds of irrigation systems. In the condition of experimental data absence, we combined simulation and survey procedure. Since the yield for each crop depends on several parameters of climate, soil, water availability, and plant coefficients, we simulated the yield of selected crops in the study area using the WEAP-MABIA software for the case of a surface irrigation system. Then, we estimated the yield of each crop under different modern irrigation systems by conducting interviews with study area farmers and the benefit from field data from two research stations of Shiraz university as well as two monitored farms of the Jihad Agriculture Organization. These four selected farms record the annual accounting information. We observed surface, drip, and sprinkler irrigation systems for selected crops at these farms and estimated the approximate difference between yields under surface irrigation and other modern irrigation systems. In the second stage, we presented the generated yield data to farmers and experts to get sure about the reliability of our estimation. The conduct of this procedure of simulation makes our assessment of crop yields more powerful and reliable in the lack of historical experimental information.
Based on our interviews with the farmers and experts, the yield of a selected crop has no significant difference across different types of sprinkler systems, and what makes difference is the cost of them. The results showed that for barley, the average yield under drip irrigation is about 1 ton more than the yield under surface irrigation. While the yield of barley under sprinkler irrigation is lower than drip irrigation; according to Chouhan et al. (2014), this difference is 12%. The average yield of wheat in the Doroodzan region under a drip system will have 2 tons more than the surface system. This amount is about 1 ton for the sprinkler irrigation system. On average, in the Doroodzan irrigation area, the yield of tomato under the drip system is about 20 tons higher than the yield under surface irrigation. In the case of forage corn, farmers mentioned that the average yield under drip irrigation is about 8 tons more than surface irrigation, while under sprinkler irrigation, it is approximately equal to the yield under surface irrigation. Table 1 shows the average yield of selected crops under different irrigation systems.
The data for price, cost, and inputs for barley, forage corn, wheat, and tomato production used in the investigation were derived from the Ministry of Agriculture Jihad's agricultural production cost databank. Moreover, the average total fixed, maintenance, and operation costs of different irrigation systems per hectare were collected from the pressurized irrigation systems office of the Ministry of Agriculture Jihad. We utilized linear regression to detrend yield and cost data before simulating agricultural yields, prices, and fuel, fertilizer, and irrigation system costs using the Microsoft Excel Add-In, SIMETAR. The 100 simulated iterations are then used to estimate the net returns per hectare for each crop production.
WEAP-MABIA Model
The Water Evaluation and Planning System (WEAP) is a useful and practical tool for comprehensive water management (Blanco-Gutiérrez et al., 2013; Esteve et al., 2015), which was developed by the Stockholm Environment Institute (SEI). WEAP, in addition to being a tool for forecasting and policy analysis, by considering the supply and demand sides of water resources, can provide a comprehensive delineation of the current state of water supply resources as well as the demand side of the basin (Yates et al., 2005). By employing the MABIA method in WEAP, the processes of evapotranspiration, runoff, infiltration, and irrigation requirements at the basin can be simulated. The MABIA method is a daily simulation of evapotranspiration, irrigation and planned requirements, crop growth, and yield, which includes some modules to estimate reference evapotranspiration and soil water capacity (Jabloun and Sahli, 2012). The period for MABIA is daily, whereas the usual period step for WEAP is monthly. Hence, for each WEAP monthly period, MABIA is implemented daily and then aggregated monthly (Esteve et al., 2015).
To apply the SERF analysis approach, we need to extract the cumulative distribution function (CDF) by using crop yield data under different irrigation systems for several years. In the absence of experimental field data, we used the results of an available calibrated WEAP-MABIA in our project and combined it with survey data. The model was calibrated by comparing the observed and simulated values of river flow, yield, and water requirement. Plant parameter including basal crop coefficient was used for calibration, and the values of calibrated water need and yield are presented in Table 2. Model accuracy is measured using the standardized bias score that showed a good level of accuracy with a bias of <20% (see Esteve et al., 2015).
Stochastic Dominance and SERF
Considering the importance of farmer decision analysis, several attempts conducted to construct DMs' utility functions (Anderson and Hardaker, 2003). The use of SD and efficiency criteria methodologies for ranking is recommended when risk preferences cannot be accurately determined. Hadar and Russell (1969) and Hanoch and Levy (1975) were the first to introduce the general concepts of first-degree stochastic dominance (FSD) and second-degree stochastic dominance (SSD) criteria. In FSD, the alternatives are ranked based on two assumptions, namely, (1) DMs prefer more wealth to less and (2) their absolute risk-aversion coefficient, which is a function of wealth, falls within the range –∞ < ra(w) < +∞. The assumption of risk aversion of DMs is added to these two assumptions in the SSD criterion, and as a result, the range of absolute risk-aversion coefficient is narrowed to 0 < ra(w) < +∞ (Hardaker and Lien, 2004). Then, given two alternatives A and B, each with its own probability distribution of outcomes x described by CDFs FA(x) and FB(x), A dominates B in the FSD if and only if the following conditions are met:
with at least one significant inequality. In terms of graphic depiction, this means that the CDF of A must always be below and to the right of the CDF of B. Neither CDF has the first-degree dominance over the other when they cross. In addition, SSD restricts the utility function:
with at least one significant inequality. As a result, this criterion compares the outcome distributions based on the regions covered by respective CDFs. SSD requires that the cumulative area under the CDF curve of the dominant alternative be positioned below and to the right of the analogous curve of the dominated alternative (Fathelrahman et al., 2014). SSD outperforms FSD in discriminatory power, and the SSD efficient set is a subset of the FSD efficient set.
It is reasonable to make a bunch of more discriminating versions of SD analysis that are based on more stringent assumptions about the risk attitudes of the DMs. The SERF analysis, which puts constraints on the absolute risk-aversion coefficient within an SSD analysis, has higher discriminatory power than FSD and SSD. For various risk attitudes, the SERF analysis employs utility-efficient alternatives. This strategy is claimed to have more discriminatory power since it takes into consideration the entire range of DM preferences (Hardaker et al., 2015).
Based on the hypothesis of preferring less risk to more by farmers at a given level of expected return (Schumann et al., 2004), we applied a negative exponential utility function in this study. A proper approximation of risk-averting behavior can be found in the negative exponential function (Adusumilli et al., 2020). Based on the suggested framework by Hardaker et al. (2015) for analyzing risky alternatives, a range of absolute risk-aversion coefficients (ra) is used to calculate CE values. While the coefficient of absolute risk aversion can be applied to outcomes assessed in terms of wealth or income (Anderson and Hardaker, 2003), the coefficient of relative risk aversion cannot. The ra measures a DM's risk-aversion degree. The DMs are categorized as risk-averse, risk-neutral, or risk-favoring, respectively, if ra > 0, ra = 0, or ra < 0. Hardaker et al. (2015) proposed the following formula for calculating ra values:
rr(w) is the relative risk-aversion coefficient in terms of wealth (w) which was set from 0 (risk-neutral farmer) to ~4 (extremely risk-averse farmer), based on Anderson and Dillon (1992). Wealth (w) was determined in this study using the respective net return means from barley, forage corn, wheat, and tomato under four different irrigation systems, namely, surface, drip, permanent sprinkler, and semi-permanent sprinkler. The ra values vary from 0 and 0.04, which translates to a range of rr coefficients of 0 and 4. As a result, for each irrigation option, the SERF approach used ra values ranging from 0 to 0.04 to calculate CE values for barley, forage corn, wheat, and tomato. The risk premiums for irrigation systems are then determined by subtracting CE values from the identical status-quo system at defined ra values, and the difference between CE values is recognized as the risk premium (Adusumilli et al., 2020).
The Study Area
The study area was the irrigation network of Doroodzan which is located in the north of the Bakhtegan basin on Kor River with a gross area of 78,553 ha. The most important water sources in this area are the regulated water of Doroodzan Dam and groundwater sources, which have decreased due to recent droughts. Regarding the increasing use of farmers in the arable areas of the Kor river basin to convert rain-fed agriculture into irrigated agriculture, the risk of water shortage in the downstream plains threatens the future of the region. On the one hand, providing the farmer's livelihood, and on the other hand, the reduction of available water and decreasing the river inflow to Bakhtegan lake, highlight the importance of irrigation technique improvements. More than 90% of the cultivation area in this region is allocated to wheat and barley crops in winter, and rice, tomato, and forage corn in summer. The study area's geographical location is depicted in Figure 2.
Results
Table 3 displays the mean and standard deviation of the simulated barley, forage corn, wheat, and tomato net returns for four alternative irrigation systems. In all selected crops, drip irrigation gives greater mean net returns per hectare, although the order of mean net returns per hectare for other irrigation systems changes from crop to crop.
SD was used to compare the performances of various irrigation systems for four different crops. The CDFs of different irrigation systems for barley, forage corn, wheat, and tomato are shown in Figure 3. FSD analysis was used to compare irrigation alternatives.
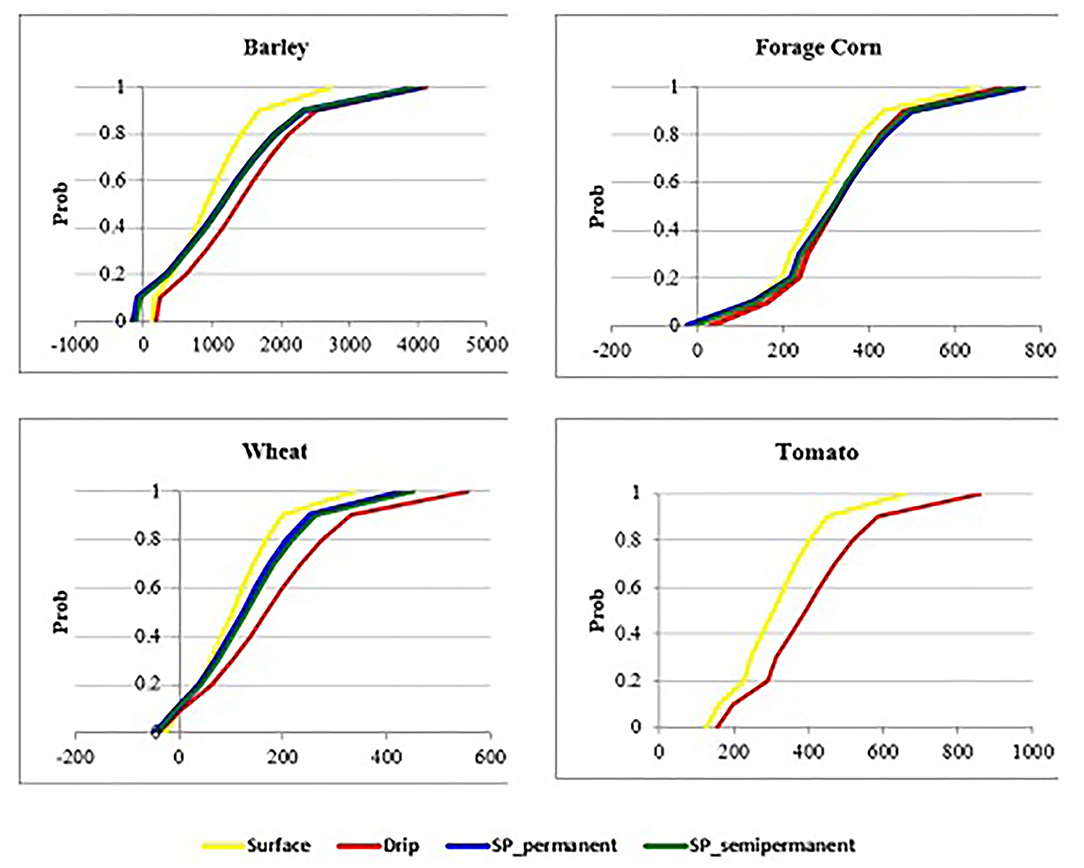
Figure 3. Cumulative distribution function of irrigation systems of Barley, Forage corn, Wheat, and Tomato.
Tables 4–7 present the results of SD testing for each irrigation system vs. others for barley, forage corn, wheat, and tomato, respectively. As can be seen, drip irrigation dominates all other systems by the FSD and SSD order for barley. Based on the SSD order comparison, the semi-permanent irrigation system dominates the permanent irrigation system. We observed that FSD and SSD order analysis is not able to compare other irrigation alternatives for barley. In the case of forage corn, drip irrigation dominates surface irrigation by FSD and SSD order while dominating permanent and semi-permanent sprinkler irrigation systems by SSD order. In addition, the semi-permanent irrigation system for forage corn dominates the permanent one by SSD. As the CDFs of the four irrigation systems for wheat indicate, the drip irrigation system is the furthest from the origin in comparison with the two sprinkler irrigation systems and does not intersect with them. Thus, drip irrigation dominates the permanent sprinkler and semi-permanent sprinkler in the first-degree sense. In the first-degree sense, however, it is impossible to discriminate between drip and surface systems for wheat. In addition, the SSD test revealed that drip irrigation surpassed various types of sprinkler irrigation for wheat. Overall, the SSD ranking of wheat irrigation systems shows that drip is preferred over semi-permanent sprinkler, which is then favored over permanent sprinklers. In terms of FSD and SSD for wheat, there is no evidence of predominance across surface irrigation methods. Considering the fact that tomatoes are grown in the Doroodzan region with only two systems of surface and drip irrigation, only these two systems were compared. Based on the results of SD testing for tomato, drip irrigation system dominates the surface system by FSD and SSD order for tomato.
Using SERF based on a negative exponential utility function, the calculated CE values for the selected irrigation systems are plotted over a defined range of ra to facilitate rankings for DM's with varied risk attitudes. For relatively low degrees of risk aversion up to 0.02, the SERF analysis results for barley crops showed that drip irrigation is favored over all other irrigation methods, while the surface irrigation technique is chosen by more risk-averse farmers. This finding could be due to the high cost of drip irrigation equipment as well as the low expected yield and price of barley in the study area. For all levels of risk aversion, sprinkler irrigation systems are considerably less preferred in comparison with drip and surface. However, the semi-permanent irrigation system was more preferred among sprinkler systems by increasing the levels of risk aversion. At all degrees of risk aversion, the permanent sprinkler irrigation system was the least preferred method of irrigating barley, especially for more risk-averse farmers.
Forage corn as an important crop in the Doroodzan irrigation network by a high portion in cropping pattern is irrigated by a wide range of different systems including surface systems, drip, and various kinds of sprinkler systems. Thus, choosing an appropriate irrigation system for this crop is very important. The interview results showed that there is a wide range of ideas about the most appropriate irrigation system for forage corn. According to the SERF analysis, for all degrees of risk aversion, drip irrigation is the most recommended system. The second most preferred irrigation system for forage corn was the semi-permanent sprinkler system. However, with the increase in risk-aversion coefficient, the distance of the first and second most preferred systems to irrigate forage corn increases. The third most preferred system differed at various levels of risk aversion. For farmers who are less risk-averse, permanent sprinkler was the third preferred irrigation technique, while surface irrigation was the third-ranked irrigation option for farmers with a risk-aversion coefficient larger than 0.015. The permanent sprinkler irrigation system has a high energy demand, thus despite lower equipment costs, its reliability degrades for more risk-averse farmers. It is worth noting that there is no considerable difference between drip, permanent sprinkler, and semi-permanent sprinkler systems to irrigate forage corn for the risk-neutral farmer who maximizes profit. The other valuable result of SERF analysis for forage corn was related to surface irrigation. As shown in Figure 4, the rank of the surface irrigation system changed from the least preferred irrigation system to the second most preferred one by increasing the risk-aversion coefficient of the farmers.
Different irrigation systems a farmer could choose for the wheat crop were assessed by SERF analysis at different levels of risk aversion. SERF analysis showed that drip irrigation was the most preferred system for wheat except for extremely risk-averse farmers. As shown in Figure 4, the CE of the drip irrigation system is maximum up to the risk-aversion coefficient of 0.035. The second preferred irrigation system for wheat was the semi-permanent sprinkler for risk-aversion coefficients ranging from 0 to ~0.02, which could be due to the relatively lower cost and energy use of semi-permanent in comparison with other kinds of sprinkler systems. For risk-aversion level of 0.02 and higher, surface irrigation was the second preferred system for wheat, even though for lower risk-averse. The results of SERF analysis for tomato show that at all levels of risk aversion, farmers prefer drip irrigation to surface irrigation. This finding revealed that the trade-off between benefits from tomato yield improvement and the additional costs of the drip system has been able to justify the superiority of the drip irrigation system.
Table 8 shows the estimated risk premiums for selected ra levels. The risk premium metric illustrates how much money is needed to get people to change their current method of irrigation. The premium for switching from another system to a drip irrigation system is estimated by differentiating CE of the drip system from others. For example, a risk-neutral farmer currently using a surface irrigation system will pay 120.57 thousand Rials to move to a drip irrigation system for barley crops. In other words, a risk-neutral farmer's least expected net return in drip irrigation system is 120.57 thousand Rials. However, the tendency to change the irrigation system from surface to drip decreases by increasing the risk-aversion coefficient of individuals, so the farmers with the highest risk-aversion coefficient prefer to stay in the surface irrigation system in the cases of wheat and barley. As shown in Table 8, farmers of forage corn and tomato with varying levels of risk aversion are willing to pay to switch from a surface irrigation system to a drip irrigation system. In fact, they expect more profitability in using the drip system for the cases of forage corn and tomato. In addition, farmers are willing to pay a premium to shift from sprinkler to drip systems for barley, wheat, and forage corn in all levels of risk aversion. Wheat farmers with risk-aversion coefficients up to 0.02 are willing to pay to turn the surface system into a drip system while, for risk-aversion coefficients higher than 0.03, they must receive a fee to be motivated to change the drip irrigation system.
Governments considering giving incentives for the adoption of modern irrigation systems should use the risk premiums determined in this way as a guide. These incentives are typically based on the expenses of installing those systems; but, when working with farmers who have varying levels of risk resilience, it is vital to understand their risk-taking behavior and determine the incentive amount that drives them to adopt new methods (Adusumilli et al., 2020).
Discussion
Using the SIMETAR add-ins of Excel and SERF analysis, this study investigated the profitability and risk efficiency of different irrigation systems for main crops in the Doroodzan region of the Bakhtegan Basin. The 5-year yield data were generated using simulation, surveys, and field data observations, and for each irrigation system, net return distributions were created. When comparing irrigation options using the FSD and SSD approaches, it was discovered that SD is unable to appraise the majority of systems. SD indicates that drip irrigation is dominant over all other systems for barley, over the surface, permanent and semi-permanent sprinklers for forage corn, and both kinds of sprinkler for wheat. However, SERF makes better discrimination between irrigation systems by the different levels of risk aversion. For a risk-neutral farmer, drip irrigation was found to be most preferred for all selected crops. This finding is consistent with Veisi et al. (2022) that stakeholders believe in the superiority of pressurized irrigation to traditional surface irrigation. However, by increasing the risk-aversion coefficient, surface irrigation systems' preference increases. This implies the fact that risk-averse farmers tend to remain on their usual farm practices systems because of their insufficient knowledge about the risk-efficient alternatives. Hence, policymakers and promoters should consider this group of farmers to inform and support them in adopting more efficient alternatives. This result is in line with Hristovska et al. (2012) and Bijttebier et al. (2018) that farmers' perceptions and information affect the adoption decision.
Estimation of risk premiums showed that willingness to pay amount varies depending on whether the farmer is risk-averse or risk-averse. Moreover, it clarifies that crop net returns have a significant influence on risk premiums; risk-neutral farmers would pay to transition from a surface to a drip irrigation system in barley and wheat, but risk-averse farmers would need to be compensated for their propensity to switch irrigation systems. The risk premiums for all crops and at all levels of ra are negative in the sense of both kinds of sprinkler systems. In other words, by comparing net returns, all farmers prefer to move from sprinkler to drip systems in the Doroodzan region. The important policy implications of these results regarding risk premiums can consider justifying subsidy allocation in a manner that induces more risk-efficient irrigation systems in the Bakhtegan Basin of Iran. Finally, as a trial to assess irrigation systems at different levels of risk aversion, this study suggested creating a regional attempt to encourage farmers to use a more efficient irrigation system in the uncertain world of decision-making.
Data Availability Statement
The raw data supporting the conclusions of this article will be made available by the authors, without undue reservation.
Author Contributions
MZ: conceptualization, resources, supervision, methodology, and writing—review and editing. DJ: conceptualization, methodology, software, formal analysis, investigation, and writing—original draft. All authors contributed to the article and approved the submitted version.
Conflict of Interest
The authors declare that the research was conducted in the absence of any commercial or financial relationships that could be construed as a potential conflict of interest.
Publisher's Note
All claims expressed in this article are solely those of the authors and do not necessarily represent those of their affiliated organizations, or those of the publisher, the editors and the reviewers. Any product that may be evaluated in this article, or claim that may be made by its manufacturer, is not guaranteed or endorsed by the publisher.
References
Adusumilli, N., Davis, S., and Fromme, D. (2016). Economic evaluation of using surge valves in furrow irrigation of row crops in Louisiana: a net present value approach. Agric. Water Manag. 174, 61–65. doi: 10.1016/j.agwat.2016.04.024
Adusumilli, N., Wang, H., Dodla, S., and Deliberto, M. (2020). Estimating risk premiums for adopting no-till and cover crops management practices in soybean production system using stochastic efficiency approach. Agric. Syst. 178, 102744. doi: 10.1016/j.agsy.2019.102744
Anderson, J. R., and Dillon, J. L. (1992). Risk Analysis in Dryland Farming Systems, No. 2. Food and Agriculture Organisation.
Anderson, J. R., and Hardaker, J. B. (2003). Risk aversion in economic decision making: pragmatic guides for consistent choice by natural resource managers. Risk Uncertain. Environ. Natural Resour Econom. 171–187. Available online at: https://hdl.handle.net/1959.11/6085
Balana, B. B., Bizimana, J. C., Richardson, J. W., Lefore, N., Adimassu, Z., and Herbst, B. K. (2020). Economic and food security effects of small-scale irrigation technologies in northern Ghana. Water Resour. Econ. 29, 100141. doi: 10.1016/j.wre.2019.03.001
Bernardo, D. J. (1988). The effect of spatial variability of irrigation applications on risk-efficient strategies. J. Agri. Appl. Econom. 20, 77–86.
Bijttebier, J., Ruysschaert, G., Hijbeek, R., Werner, M., Pronk, A. A., Zavattaro, L., et al. (2018). Adoption of non-inversion tillage across Europe: use of a behavioural approach in understanding decision making of farmers. Land Use Policy 78, 460–471. doi: 10.1016/j.landusepol.2018.05.044
Blanco-Gutiérrez, I., Varela-Ortega, C., and Purkey, D. R. (2013). Integrated assessment of policy interventions for promoting sustainable irrigation in semi-arid environments: a hydro-economic modeling approach. J. Environ. Manage. 128, 144–160. doi: 10.1016/j.jenvman.2013.04.037
Chouhan, S. S., Awasthi, M. K., and Nema, R. K. (2014). Maximizing water productivity and yields of wheat based on drip irrigation systems in clay loam soil. Int. J. Engg. Res. Technol. 3, 533–535. Available online at: https://www.ijert.org/research/maximizing-water-productivity-and-yields-of-wheat-based-on-drip-irrigation-systems-in-clay-loam-soil-IJERTV3IS070643.pdf
Daccache, A., Ciurana, J. S., Rodriguez Diaz, J. A., and Knox, J. W. (2014). Water and energy footprint of irrigated agriculture in the Mediterranean region. Environ. Res. Lett. 9, 124014. doi: 10.1088/1748-9326/9/12/124014
Daccache, A., Knox, J. W., Weatherhead, E. K., Daneshkhah, A., and Hess, T. M. (2015). Implementing precision irrigation in a humid climate - Recent experiences and on-going challenges. Agric. Water Manag. 147, 135–143. doi: 10.1016/j.agwat.2014.05.018
Deveci, O., Onkol, M., Unver, H. O., and Ozturk, Z. (2015). Design and development of a low-cost solar powered drip irrigation system using Systems Modeling Language. J. Clean. Prod. 102, 529–544. doi: 10.1016/j.jclepro.2015.04.124
Duenhas, L. H., and Saad, J. C. C. (2009). Economic viability and selection of irrigation systems using simulation and stochastic dominance. IRRIGA 14, 422–430. doi: 10.15809/irriga.2009v14n4p422-430
Epperson, J. E., Hook, H. E., and Mustafa, Y. R. (1992). Stochastic dominance analysis for more profitable and less risky irrigation of corn. J. Prod. Agri. 5, 243–247.
Espinosa-Tasón, J., Berbel, J., and Gutiérrez-Martín, C. (2020). Energized water: evolution of water-energy nexus in the Spanish irrigated agriculture, 1950–2017. Agric. Water Manag. 233, 106073. doi: 10.1016/j.agwat.2020.106073
Esteve, P., Varela-Ortega, C., Blanco-Gutiérrez, I., and Downing, T. E. (2015). A hydro-economic model for the assessment of climate change impacts and adaptation in irrigated agriculture. Ecol. Econ. 120, 49–58. doi: 10.1016/j.ecolecon.2015.09.017
Fan, Y., Liu, Y., DeLaune, P. B., Mubvumba, P., Park, S. C., and Bevers, S. J. (2020). Economic analysis of adopting no-till and cover crops in irrigated cotton production under risk. Agron. J. 112, 395–405. doi: 10.1002/agj2.20005
Fathelrahman, E., Basarir, A., Gheblawi, M., Sherif, S., and Ascough, J. (2014). Economic risk and efficiency assessment of fisheries in Abu-Dhabi, United Arab Emirates (UAE): a stochastic approach. Sustain. 6, 3878–3898. doi: 10.3390/su6063878
García-Prats, A., and Guillem-Picó, S. (2016). Adaptation of pressurized irrigation networks to new strategies of irrigation management: energy implications of low discharge and pulsed irrigation. Agric. Water Manag. 169, 52–60. doi: 10.1016/j.agwat.2016.02.023
Hadar, J., and Russell, W. R. (1969). Rules for ordering uncertain prospects. Am. Econ. Rev. 59, 25–34.
Hanoch, G., and Levy, H. (1975). “The efficiency analysis of choices involving risk,” in Stochastic Optimization Models in Finance, eds W. T. Ziemba and R. G. Vickson (Academic Press), 89–100.
Hardaker, J. B., and Lien, G. (2004). Stochastic efficiency analysis with risk aversion bounds: a comment. Aust. J. Agric. Resour. Econ. 54, 379–383. doi: 10.1111/j.1467-8489.2010.00498.x
Hardaker, J. B., Lien, G., Anderson, J. R., and Huirne, R. B. (2015). Coping With Risk in Agriculture: Applied Decision Analysis. Boston, MA: CABI.
Harris, T. R., and Mapp, H. P. (1986). A stochastic dominance comparison of water-conserving irrigation strategies. Am. J. Agri. Econom. 68, 298–305.
Hristovska, T., Watkins, K. B., and Anders, M. M. (2012). “An economic risk analysis of no-till management for the rice-soybean rotation system used in Arkansas,” in 2012 Annual Meeting (Birmingham, AL: Southern Agricultural Economics Association). doi: 10.22004/ag.econ.119676
Imran, M. A., Ali, A., Ashfaq, M., Hassan, S., Culas, R., and Ma, C. (2019). Impact of climate smart agriculture (CSA) through sustainable irrigation management on Resource use efficiency: a sustainable production alternative for cotton. Land Use Policy 88, 104113. doi: 10.1016/j.landusepol.2019.104113
Islam, K. M. N., Kenway, S. J., Renouf, M. A., Lam, K. L., and Wiedmann, T. (2021). A review of the water-related energy consumption of the food system in nexus studies. J. Clean. Prod. 279, 123414. doi: 10.1016/j.jclepro.2020.123414
Jabloun, M., and Sahli, A. (2012). WEAP-MABIA Tutorial 97. Federal Institute for Geosciences and Natural Resources, Hannover, Germany.
Jing, B., Shah, F., Xiao, E., Coulter, J. A., and Wu, W. (2020). Sprinkler irrigation increases grain yield of sunflower without enhancing the risk of root lodging in a dry semi-humid region. Agric. Water Manag. 239, 106270. doi: 10.1016/j.agwat.2020.106270
Karimi, V., Karami, E., and Keshavarz, M. (2018). Climate change and agriculture: Impacts and adaptive responses in Iran. J. Integr. Agri. 17, 1–15. doi: 10.1016/S2095-3119(17)61794-5
Khor, L. Y., and Feike, T. (2017). Economic sustainability of irrigation practices in arid cotton production. Water Resour. Econ. 20, 40–52. doi: 10.1016/j.wre.2017.10.004
Krishnan, R. S., Julie, E. G., Robinson, Y. H., Raja, S., Kumar, R., Huy, P., et al. (2020). Fuzzy logic based smart irrigation system using internet of things. J. Clean. Prod. 252, 119902. doi: 10.1016/j.jclepro.2019.119902
Liaghat, A. (2012). Analysis of Energy Consumption in Pressurized Irrigation Systems and Presentation of Methodology for Studying Energy Consumption in the Process of Design and Development of Systems. Tehran: Ministry of Agriculture-Jihad (MAJ).
Liu, Y., Langemeier, M. R., Small, I. M., Joseph, L., and Fry, W. E. (2017). Risk Management Strategies using Precision Agriculture Technology to Manage Potato Late Blight. Agronom. J. 109, 562–575. doi: 10.2134/agronj2016.07.0418
Mesgaran, M. B., and Azadi, P. (2018). “A national adaptation plan for water scarcity in Iran,” in Working paper 6, Stanford Iran 2040 Project (Stanford, CA: Stanford University).
Ministry of Agriculture Jihad. (2020). Available online at: https://maj.ir
Modarres Reza, Ali Sarhadi, and Donald H. Burn. (2016). “Changes of extreme drought and flood events in Iran.” Global and Planetary Change 144, 67–81.
Montazar, A., and Behbahani, S. M. (2007). Development of an optimised irrigation system selection model using analytical hierarchy process. Biosyst. Eng. 98, 155–165. doi: 10.1016/j.biosystemseng.2007.06.003
Mukherji, A., Falcon, T., Burke, J., de Fraiture, C., Faurès, J.-M., Füleki, B., et al. (2009). Revitalizing Asia's Irrigation: To Sustainably Meet Tomorrow's Food Needs. Colombo: International Water Management Institute; Rome: Food and Agriculture Organization of the United Nations.
NCCOI (2014). Third National Communication to UNFCCC. National Climate Change Office of Iran. Available online at: https://unfccc.int/sites/default/files/resource/ThirdNationalcommunicationIRAN.pdf
Okyere, C. Y., and Usman, M. A. (2021). The impact of irrigated agriculture on child nutrition outcomes in southern Ghana. Water Resour. Econ. 33, 100174. doi: 10.1016/j.wre.2020.100174
Özkan, S., Farquharson, R. J., Hill, J., and Malcolm, B. (2015). A stochastic analysis of the impact of input parameters on profit of Australian pasture-based dairy farms under variable carbon price scenarios. Environ. Sci. Policy 48, 163–171. doi: 10.1016/j.envsci.2014.12.012
Paydar, Z., and Qureshi, M. E. (2012). Irrigation water management in uncertain conditions—Application of Modern Portfolio Theory. Agric. Water Manag. 115, 47–54. doi: 10.1016/j.agwat.2012.08.004
Rodrigues, G. C., Paredes, P., Gonçalves, J. M., Alves, I., and Pereira, L. S. (2013). Comparing sprinkler and drip irrigation systems for full and deficit irrigated maize using multicriteria analysis and simulation modelling: Ranking for water saving vs. farm economic returns. Agric. Water Manag. 126, 85–96. doi: 10.1016/j.agwat.2013.05.005
Scheierling, S. M., Young, R. A., and Cardon, G. E. (2006). Public subsidies for water-conserving irrigation investments: hydrologic, agronomic, and economic assessment. Water Resour Res. 42, 1–11. doi: 10.1029/2004WR003809
Schumann, K. D., Richardson, J. W., Lien, G. D., and Hardaker, J. B. (2004). “Stochastic Efficiency Analysis Using Multiple Utility Functions,” in Presentation at the American Agricultural Economics Association Annual Meeting (Denver, CO).
Siyal, A. W., Gerbens-Leenes, P. W., and Nonhebel, S. (2021). Energy and carbon footprints for irrigation water in the lower Indus basin in Pakistan, comparing water supply by gravity fed canal networks and groundwater pumping. J. Clean. Prod. 286, 489. doi: 10.1016/j.jclepro.2020.125489
Smith, R., and Baillie, J. (2009). “Defining precision irrigation: A new approach to irrigation management,” in Irrigation Australia 2009: Irrigation Australia Irrigation and Drainage Conference: Proceedings (Irrigation Australia Ltd), 1–6.
Srdjevic, B., and Obradovic, D. (1997). Reliability and risk in agricultural irrigation. IFAC Proc. 30, 97–102. doi: 10.1016/S1474-6670(17)41252-3
Veisi, H., Deihimfard, R., Shahmohammadi, A., and Hydarzadeh, Y. (2022). Application of the analytic hierarchy process (AHP) in a multi-criteria selection of agricultural irrigation systems. Agri. Water Manag. 267, 107619. doi: 10.1016/j.agwat.2022.107619
Yang, Q., Zhu, Y., and Wang, J. (2020). Adoption of drip fertigation system and technical efficiency of cherry tomato farmers in Southern China. J. Clean. Prod. 275, 123980. doi: 10.1016/j.jclepro.2020.123980
Yates, D., Sieber, J., Purkey, D., and Huber-Lee, A. (2005). WEAP21 - A demand-, priority-, and preference-driven water planning model. Part 1: Model characteristics. Water Int. 30, 487–500. doi: 10.1080/02508060508691893
Zabel, F., Putzenlechner, B., and Mauser, W. (2014). Global agricultural land resources - a high resolution suitability evaluation and its perspectives until 2100 under climate change conditions. PLoS ONE 9, e0107522. doi: 10.1371/journal.pone.0107522
Keywords: modern irrigation systems, risk aversion, certainty equivalent, risk premiums, stochastic dominance
Citation: Jahangirpour D and Zibaei M (2022) Farmers' Decision to Adoption of Modern Irrigation Systems Under Risk Condition: Application of Stochastic Efficiency With Respect to a Function Approach. Front. Water 4:931694. doi: 10.3389/frwa.2022.931694
Received: 29 April 2022; Accepted: 31 May 2022;
Published: 09 September 2022.
Edited by:
Masoud Bijani, Tarbiat Modares University, IranReviewed by:
Niloofar Ashktorab, Khuzestan University of Agricultural Sciences and Natural Resources, IranMostafa Mardani Najafabadi, Ramin Agriculture and Natural Resources University of Khouzestan, Iran
Abbas Mirzaei, Agricultural Sciences and Natural Resources University of Khuzestan, Iran
Copyright © 2022 Jahangirpour and Zibaei. This is an open-access article distributed under the terms of the Creative Commons Attribution License (CC BY). The use, distribution or reproduction in other forums is permitted, provided the original author(s) and the copyright owner(s) are credited and that the original publication in this journal is cited, in accordance with accepted academic practice. No use, distribution or reproduction is permitted which does not comply with these terms.
*Correspondence: Dorna Jahangirpour, djahangirpour@shirazu.ac.ir