- 1Interdisciplinary Centre for Water Research, Indian Institute of Science, Bengaluru, India
- 2Hydro-Remote Sensing (H-RSA) Group, Remote Sensing Division, Department of Civil Engineering, Indian Institute of Technology Bombay, Mumbai, India
- 3Department of Civil Engineering, Indian Institute of Technology Indore, Indore, India
- 4Department of Geography and Sustainable Development, University of St Andrews, St Andrews, United Kingdom
- 5Department of Earth and Environmental Sciences, Indian Institute of Science Education and Research, Bhopal, India
- 6Computer Science Department, Chandigarh University, Ajitgarh, India
- 7School of Environmental Sciences, Jawaharlal Nehru University, New Delhi, India
- 8Department of Civil Engineering, Indian Institute of Technology Kanpur, Kanpur, India
- 9Indian Institute of Technology, Bombay (IITB), Mumbai, India
- 10Rural Data Research and Analysis (RuDRA), Mumbai, India
- 11Department of Civil Engineering, Indian Institute of Technology Bombay, Mumbai, India
- 12CIIRC, Jyothy Institute of Technology, Bengaluru, India
- 13National Institute of Hydrology, Roorkee, India
- 14Department of Civil Engineering, Indian Institute of Technology Roorkee, Roorkee, India
- 15College of Life and Environmental Sciences, Exeter University, Exeter, United Kingdom
- 16School of Geographical Sciences, University of Bristol, Bristol, United Kingdom
- 17Department of Aerospace and Geodesy, Data Science in Earth Observation, Technical University of Munich, Munich, Germany
The Himalaya plays a vital role in regulating the freshwater availability for nearly a billion people living in the Indus, Ganga, and Brahmaputra River basins. Due to climate change and constantly evolving human-hydrosphere interactions, including land use/cover changes, groundwater extraction, reservoir or dam construction, water availability has undergone significant change, and is expected to change further in the future. Therefore, understanding the spatiotemporal evolution of the hydrological cycle over the Himalaya and its river basins has been one of the most critical exercises toward ensuring regional water security. However, due to the lack of extensive in-situ measurements, complex hydro-climatic environment, and limited collaborative efforts, large gaps in our understanding exist. Moreover, there are several significant issues with available studies, such as lack of consistent hydro-meteorological datasets, very few attempts at integrating different data types, limited spatiotemporal sampling of hydro-meteorological measurements, lack of open access to in-situ datasets, poorly accounted anthropogenic climate feedbacks, and limited understanding of the hydro-meteorological drivers over the region. These factors result in large uncertainties in our estimates of current and future water availability over the Himalaya, which constraints the development of sustainable water management strategies for its river catchments hampering our preparedness for the current and future changes in hydro-climate. To address these issues, a partnership development workshop entitled “Water sEcurity assessment in rIvers oriGinating from Himalaya (WEIGH),” was conducted between the 07th and 11th September 2020. Based on the intense discussions and deliberations among the participants, the most important and urgent research questions were identified. This white paper synthesizes the current understanding, highlights, and the most significant research gaps and research priorities for studying water availability in the Himalaya.
Water in the Himalaya Along Different Dimensions
The Himalaya consists of the highest mountains in the world, its orogeny leading to unique geomorphology has given rise to many complex processes such as river meandering, snow-glacier-permafrost interactions, Indian winter and summer monsoons (c.f. Figure 1; Bookhagen and Burbank, 2006; Dimri et al., 2015). The Himalaya is also known as the Third Pole as they contain a large amount of frozen water outside the polar region, which together with the Indian summer and winter monsoons (Dimri et al., 2016; Maharana et al., 2019), ensures that the rivers originating from the Himalaya plausibly never run dry (c.f. Figure 1; Bolch et al., 2019a; Immerzeel et al., 2020). Therefore, these river systems are crucial for supporting the agrarian societies and hydro-power needs of the downstream nations (Dame and Nüsser, 2011; Nüsser et al., 2012; Azam et al., 2021). Precipitation and temperature gradients across and along the Himalaya in conjunction with ridge-valley floor interactions lead to complex manifestation and physical orographic forcings (Bookhagen and Burbank, 2010; Thayyen and Dimri, 2018; Banerjee et al., 2020, 2021). The occurrence and frequency of snowfall replenishes the water storage over the Himalaya (Daloz et al., 2020). Downstream into the Indian subcontinent, the Indian summer monsoon precipitation becomes the dominant driver for water mass transport on and below the surface. It stays for a short time in surface water bodies and longer in sub-surface water bodies. However, groundwater exploitation over the northwest Indian region in recent decades is changing the residence time of groundwater and affecting water availability (Bookhagen and Burbank, 2010; Singh et al., 2019).
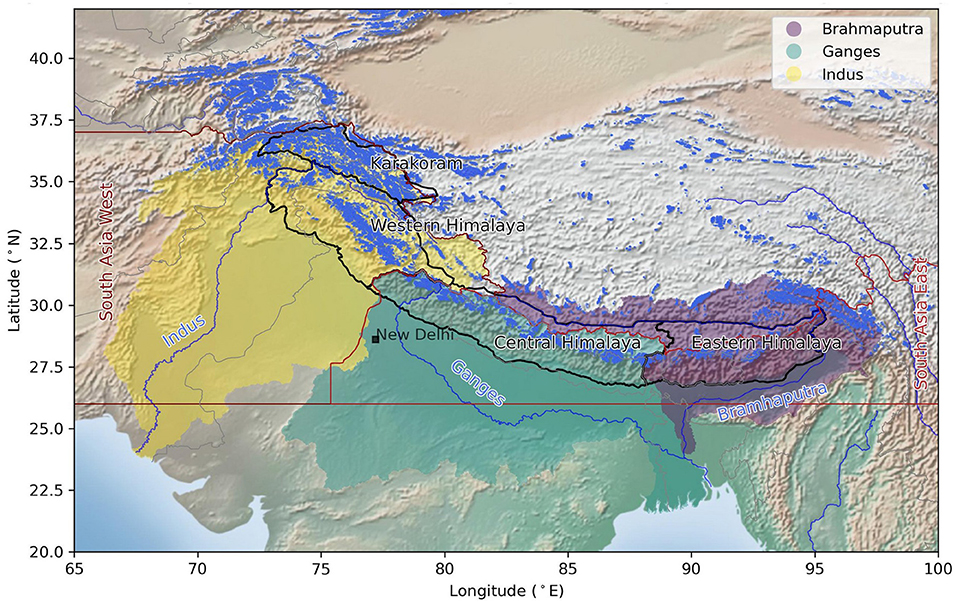
Figure 1. Map of the Himalayan River Basins—Indus, Ganga and Brahmaputra—feeding from the glaciers. The glacier cover (blue patches) is based on Randolph Glacier Inventory 6.0 (RGI Consortium, 2017). The background is the Stamen terrain map, the Himalayan region subdivision from Bolch et al. (2019a), river basin boundaries are based on the International Center for Integrated Mountain Development (ICIMOD, 2021), and river central lines are from the Global High-Resolution River Centerlines (USGS). RGI's first order regions 14 (South Asia West) and 15 (South Asia East) are also shown (red outlines).
The availability of water also varies both spatially and temporally. Water leaves the Himalayan river basins in the form of water vapor (driven by evapotranspiration) and river discharge. The majority of these river systems, such as the Indus, Ganga, and Brahmaputra, feed multiple nations (Figure 1), and thus the sharing of river discharge data is a politically sensitive issue (Molden et al., 2017). In addition, accurate evapotranspiration modeling requires an extensive network of weather stations, while only a limited number of weather stations are operational, especially near the glacierised regions where topographical challenges are inherent (Azam et al., 2018). Hence, remote sensing and gridded/reanalysis datasets becomes one of the preferred tools for estimating hydro-meteorological observables such as, air temperature, precipitation, evapotranspiration, but they come with their own challenges. For example, they may have a relatively coarse spatial resolution: the Tropical Rainfall Measuring Mission (TRMM; refer to Table A1 for abbreviations) precipitation products are at ~30 km grid (Hofmann-Wellenhof et al., 2007), and the fifth generation European Center for Medium-Range Weather Forecasts (ECMWF) atmospheric reanalysis ERA5/ERA5-Land data at ~30/~10 km grid (Hersbach et al., 2020). Also, several satellite observations are an indirect measure of a physical process and they often require calibration and validation against in-situ observations that are at times not available (Karimi and Bastiaanssen, 2015; Zhang et al., 2016). Further, the length of satellite era is relatively short, and time-series from many of the satellite missions cannot be considered as climate data (Vishwakarma et al., 2021). Satellite products require substantial post-processing to remove atmospheric effects and improve the signal to noise ratio. Due to these challenges, model simulated outputs at high spatiotemporal resolution and longer temporal coverage are also being employed to understand the past, the present, and the future of water availability in the Himalaya (Khanal et al., 2021). For example, the drivers of variability in precipitation have been investigated with the help of global and regional climate model outputs, but no conclusive pattern or trend can be identified (Immerzeel et al., 2010, 2020; Kaser et al., 2010; Lutz et al., 2016; Maharana and Dimri, 2016; Dimri et al., 2018; Huss and Hock, 2018; Maharana et al., 2019). Over the Hindu-Kush Karakoram Himalayan range, Palazzi et al. (2013) have shown decreased summer precipitation, whereas Kulkarni et al. (2013) and Javed et al. (2022) have shown increased winter precipitation. Dimri et al. (2022) explained the elevation dependent warming over the Karakoram region.
Modeling framework-based studies over the Himalaya show different impacts on different river basin systems (Ghimire et al., 2018; Nengker et al., 2018). Atmospheric changes associated with global warming can lead to alteration of the mountain hydrological cycle, in turn the streamflow patterns of the Himalayan rivers (Archer et al., 2010; Lutz et al., 2016; Armstrong et al., 2018). Available basin-wide studies show that glacier melt contributes the largest to total water discharge in the upper Indus basin (41%), whereas rainfall-runoff in the upper Ganga (66%) and Brahmaputra (59%) basins (Lutz et al., 2014). Glacier melt contribution is relatively lower in the upper Ganga (12%) and Bramhaputra (16%) basins. Snowmelt contribution is also lower in the upper Ganga (9%) and Bramhaputra (9%) basins, whereas 22% in the upper Indus basin (Lutz et al., 2014). Snowmelt contribution to the total discharge is significantly higher (or highest) in the headwaters of small/medium sub-basins in the Himalayan region, for example, in the Liddar Basin, western Himalaya (60%; Jeelani et al., 2017), Bhagirathi, upper Ganga Basin (60%; Rai et al., 2019), Chandra, a sub-basin of Chenab River (60%; Singh A. T. et al., 2021) etc. Groundwater also contributes significantly to the river discharge, but only noted in the Chandra basin (Singh A. T. et al., 2021). Nevertheless, the contribution from snow and glacier melt are expected to change in the future due to ongoing climate change (Prasch et al., 2013; Ragettli et al., 2016; Khadka et al., 2020). This is evident in the Baspa basin, a sub-basin of Satluj river, where Singh V. et al. (2021) applied a physical glacio-hydrological model model and noted an increase in snow melt contribution by 6% between 2000 and 2018.
Lutz et al. (2016) concluded that hydrological extremes would be frequent and stronger in a warmer climate, but the uncertainties are large. Based on stable isotope measurements of hydrogen and oxygen together with historical runoff records in the Indus River Basin, Karim and Veizer (2002) have shown a major loss of water to evapotranspiration, which is in line with the predictions in a warming climate (Banerjee and Azam, 2016; Kraaijenbrink et al., 2017). There are observational and modeling challenges over the Himalaya due to the paucity of observations (You et al., 2017).
In general, Himalayan glaciers have been retreating and losing mass during the last decades at a significant rate (Brun et al., 2017; Azam et al., 2018; Bolch et al., 2019a; King et al., 2019; Maurer et al., 2019; Bhattacharya et al., 2021). It is projected that river runoff in the larger catchments will increase up to 2050s but then decrease sharply outside the monsoon season owing to the decimated Himalayan glacier area and volume (Lutz et al., 2014; Rounce et al., 2020; Azam et al., 2021; Chandel and Ghosh, 2021). Therefore, we can expect to see an increase in extremes of water availability resulting in disasters such as glacial lake outburst flood (GLOF), avalanches, and droughts (Eckstein et al., 2017; Furian et al., 2021; Majeed et al., 2021; Shugar et al., 2021). GLOF is a sudden release of water or flash flood from a moraine- or ice-dammed glacial lake due to dam failure (Shugar et al., 2020; Veh et al., 2020; Sattar et al., 2021). GLOF could be triggered by a number of processes, including ice/debris/rock fall, avalanches, earthquakes, internal piping, etc. (Veh et al., 2020). GLOF mechanisms can be understood by simulating the GLOF chain sequences and flood routing modeling. Understanding these processes is critical for risk management and developing mitigation strategies for the downstream communities (Sattar et al., 2021).
A majority of the hydrometeorological and glacier-related processes (c.f. Figure 2), e.g., glacier mass balance, runoff, GLOF modeling studies rely on outputs from regional climate models, land surface models, and remote sensing datasets which suffer from high uncertainties over the Himalaya (Lutz et al., 2016; Hock et al., 2019; Gusain et al., 2020; Azam et al., 2021; Nepal et al., 2021). Furthermore, recent studies indicate that human activities, both agricultural and land use change, that are missing from models have significantly impacted the regional water budget (Budakoti et al., 2021; Chandel et al., 2021). Therefore, studies based on models can only provide a limited insight. Many attempts have been made to quantify the amount of water entering and leaving these river catchments and factors influencing their variability, but still our knowledge is limited (Lutz et al., 2016; Pritchard, 2019). Therefore, we need to obtain more data-driven inferences by including as many types of observations as possible.
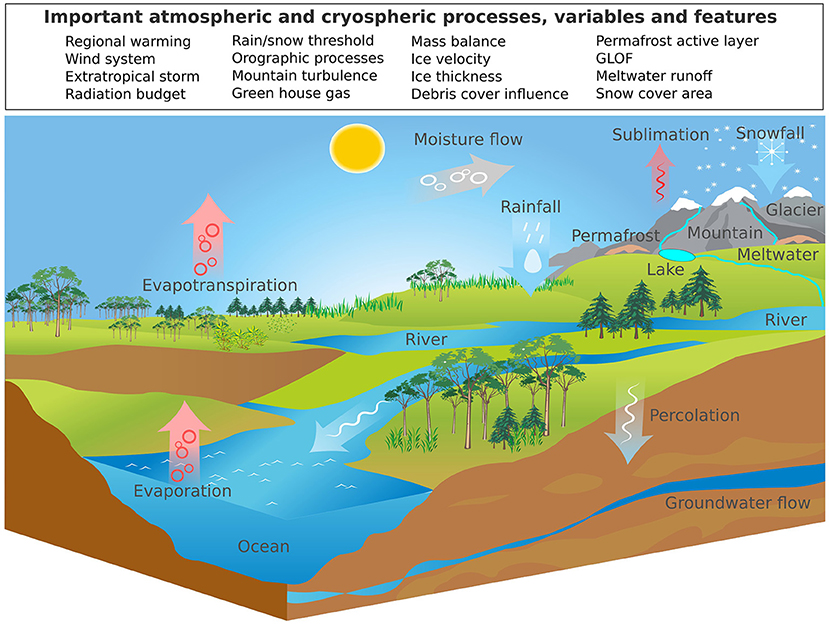
Figure 2. An illustration of simplified hydrological cycle showing the important cryospheric and atmospheric processes, variables and features. The figure is inspired from Azam et al. (2021). Background illustration credit: Nouri Atchabao/Vecteezy.
In the following sections, various techniques, observations, and methods that are being or can be employed to understand the water cycle in the Himalaya are discussed.
Estimating Glacier Mass Balance
An accurate assessment of glacier mass balance is vital for understanding the vulnerability of glaciers due to climate change and the rates of glacier denudation (Rabatel et al., 2011). The mass balance of Himalayan glaciers has been measured with different methods, from conventional (Østrem and Stanley, 1969), to glaciological, to remote sensing and geodetic (Brun et al., 2017), to various modeling techniques (Bolch et al., 2012; Shea et al., 2015; Azam et al., 2018).
In-situ Measurements
Glaciers respond to even small changes in temperature and precipitation (Oerlemans, 2001). Therefore, field observations of glacier mass balance at annual and seasonal scales are excellent climate records (Zemp et al., 2009; Trewin et al., 2021). Unfortunately, the high altitudes and harsh weather conditions hinder glaciological measurements in the Himalaya; therefore, existing in-situ studies mostly consider the easily accessible, small sized, and less debris-covered glaciers (Azam et al., 2018; Bolch et al., 2019a).
Glacier mass balance is the sum of all ablation (melt, sublimation, snowdrift, calving, etc.) and accumulation (snowfall, snowdrift, avalanches, etc.) processes (illustrated in Figure 2). These processes are glacier-wide and continuous in time, however, for practical reasons they are observed at point scale. Ablation is measured by installing an ablation stake network over the ablation area of the glaciers and assuming an ice density of 900 kg/m3 (Kaser et al., 2003; Huss, 2013). In contrast, snow accumulation is measured with the help of snow pits/cores and probing in the accumulation zone and measuring the density of snow along with the depth to the previous year's surface (Wagnon et al., 2007, 2013; Azam et al., 2016; Mandal et al., 2020; Soheb et al., 2020). These point-scale observations are integrated to estimate the glacier-wide mass balance using glacier hypsometry data obtained from satellite imagery or terrestrial sources (Cuffey and Paterson, 2010). Glacier hypsometry is generally obtained from a Digital Elevation Model (DEM) and the glacier outline (preferably from the recent ablation-season optical satellite images). Since the in-situ observations are important but limited in number to provide a comprehensive overview over the Himalaya, researchers rely on other proxies to estimate snowmelt and glacier runoff.
Due to the difficulties in field measurements in the Himalaya, only about 36 glaciers have glaciological mass balance measurements conducted for 1 year or more (Table 1). The majority of the measured glaciers are of small to medium size. Of all the 36 measured glaciers, most are located in the western Himalaya (n = 18), followed by the central Himalaya (n = 14), eastern Himalaya (n = 3), and Karakoram (n = 1). About ten glaciers have a series of measurements equal to or longer than 10 years (Table 1). The longest continuous series spans 17 years for the Chhota Shigri Glacier in the western Himalaya (−0.46 ± 0.40 m w.e. a−1 over 2002–2019; Mandal et al., 2020). The Naimona Nyi Glacier in the northern slope of central Himalaya has the longest record of annual mass balance but discontinuous (Yao et al., 2022).
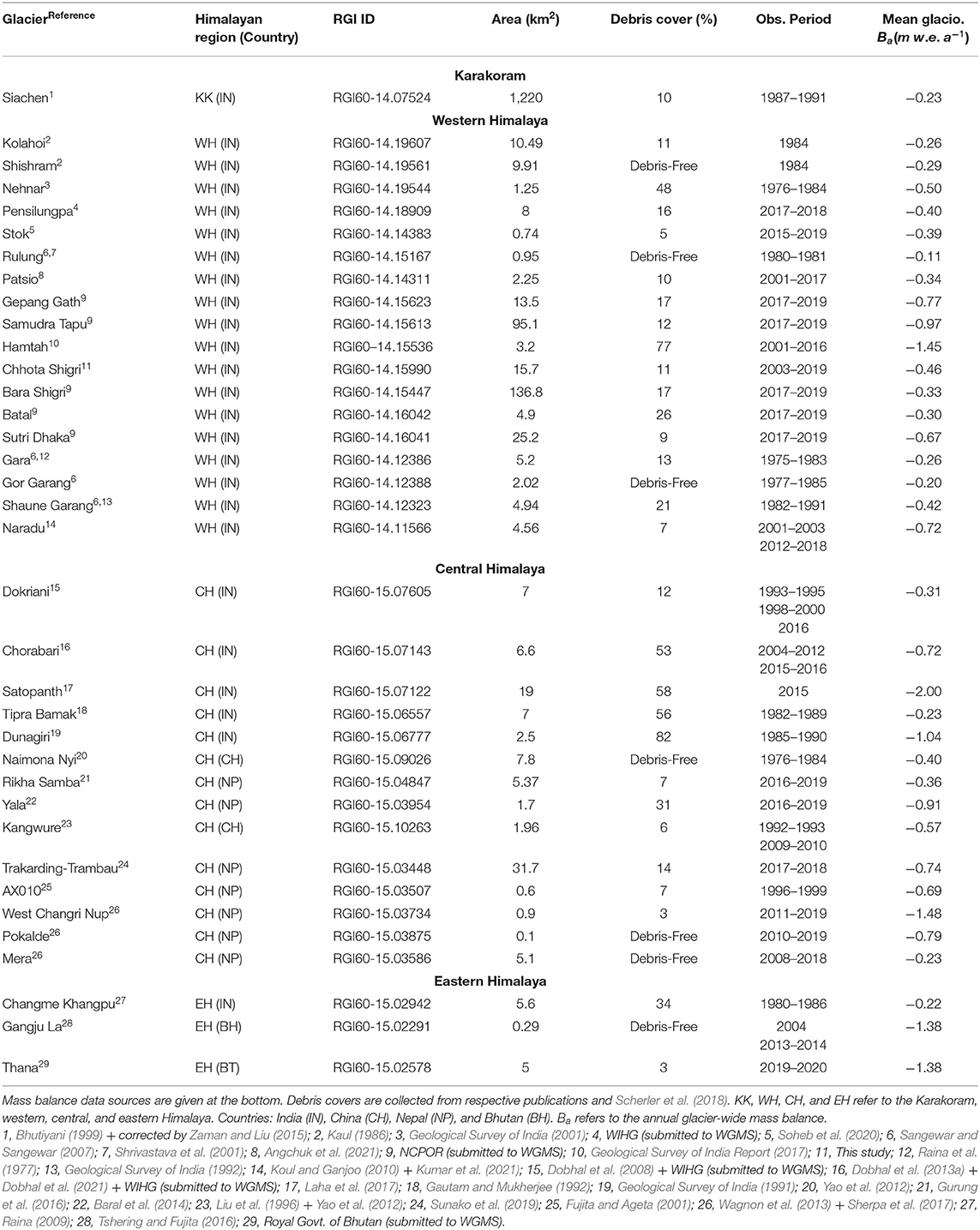
Table 1. Glaciological (in-situ) mass balance of Himalayan glaciers (only Siachen Glacier mass balance is based on hydrological method).
By averaging all available in-situ mass balance records for 36 glaciers (240 annual mass balance data points), the mean mass balance for the period 1975–2020 becomes −0.60 m w.e. a−1 (standard deviation; SD: 0.56 m w.e.). This region-wide average is not much different from the previous estimate of −0.49 m w.e. a−1 (based on mass balance records from 24 glaciers) for the period 1975–2015 (Azam et al., 2018). This increased number of glacier measurements certainly improves the representativeness of the region-wide mass balance for the Himalaya. However, considering the topo-climatic heterogeneity across the Himalaya, 36 glaciers is a small sample size of roughly 95,000 total number of glaciers in the region.
Remote Sensing Based Estimates
Various remote sensing-based methods are available to estimate glacier mass balance at different spatiotemporal scale (Table 2). Among the available methods, the geodetic method is the most widely used, which uses multiple DEMs, from at least two different epochs, to obtain a volume change estimate (Bamber and Rivera, 2007; Gardelle et al., 2012, 2013; Brun et al., 2017; Hugonnet et al., 2021). These DEMs can be obtained from various remote sensing products like (a) satellite-based optical stereo images, (b) satellite-based radar interferometry, (c) aerial photogrammetry, images from drones, or laser scanning, and (d) Uncrewed Aerial Vehicles (UAV) based data through Structure from Motion techniques (SfM).
The DEMs obtained from different techniques have different accuracy, spatial coverage, spatial resolution and procurement cost. Considering the higher vertical errors in publicly available satellite-based DEMs, they are, in general, most useful for decadal-scale glacier mass balance estimations and less for the annual mass balance (Vijay and Braun, 2016, 2018). With the availability of new generation satellites that have better instruments, very high-resolution DEM data can be generated with reasonable accuracy but only for the past few years. It is to be noted that these products are only available on demand at a price, that too at irregular intervals. A few prominent remote sensing missions that have been used widely for geodetic mass balance studies in the Himalaya are: Advanced Spaceborne Thermal Emission and Reflection Radiometer (ASTER) mission (Brun et al., 2017; Bisset et al., 2020; Hugonnet et al., 2021), Satellite Pour l'Observation de la Terre (SPOT) (Berthier et al., 2007), Pléiades (Berthier et al., 2014; Wagnon et al., 2021), WorldView/GeoEye (Shean et al., 2020), etc.
A higher temporal resolution of mass balance estimates has been generated by using the laser altimetry-based Ice, Cloud, and Land Elevation Satellite (ICESat), ICESat-2 mission data (Kääb et al., 2012, 2015; Wang et al., 2021) and CryoSat-2 swath altimetry data (Jakob et al., 2021). However, ICESat based mass balance estimates have been shown to suffer from a bias due to the short satellite time span (Neckel et al., 2014; Brun et al., 2017; Wang et al., 2021).
Another widely used method is a simple empirical approach called Accumulation Area Ratio (AAR) and Equilibrium Line Altitude (ELA) method proposed by Kulkarni (1992). AAR is the ratio of the area of the accumulation zone to the area of the glacier (Cogley et al., 2011), whereas ELA is the line that divides the glacier into ablation and accumulation areas where accumulation equals ablation over a balance year (Braithwaite and Raper, 2009). This method has considerable uncertainty (Pratap et al., 2016; Tawde et al., 2016). This was first applied on the Gara Glacier (from 1977–1978 to 1982–1983) and the Gor-Garang Glacier (from 1976-77 to 1983-84), and then has been used to estimate the surface mass balance of Himalayan glaciers (e.g., Kulkarni et al., 2004; Mir et al., 2014 and Tak and Keshari, 2020). Tawde et al. (2016) developed an improved AAR method in which they used satellite data and a temperature-index model. They implemented a regression between the modeled AAR and field-based mass balance to estimate glacier-wide mass balance. This improved AAR method was first demonstrated over the Chhota Shigri Glacier and 12 other glaciers in the Chandra basin (from 2000 to 2009). Following the same methodology, Tawde et al. (2017) estimated the mass balance of 146 glaciers in the Chandra basin (from 1984 to 2012), however these had higher uncertainties.
In another development, Rabatel et al. (2005) estimated annual mass balance using variation between ELA at steady state of a glacier and satellite-derived ELA along with mass balance gradient across the ELA. They demonstrated this method over French alpine glaciers and showed that the mass balance of individual glaciers in a basin can be estimated reliably by using field-based observation of at least one representative glacier in the same basin. In the Himalaya, Chandrasekharan et al. (2018) and Garg et al. (2021) have used this method for estimating mass balance over some glaciers. This approach seems to have good potential for annual mass balance estimation. However, it needs to be tested rigorously for wider application.
Dumont et al. (2012) reported that the annual minimum albedo average on the whole glacier at the end of the ablation season is strongly correlated (r2 > 0.95) with the field-based annual mass balance of the Saint Sorlin Glacier in Saint Sorlin Glacier in France. Based on this correlation, the annual mass balance series of the glacier was reconstructed from the Moderate Resolution Imaging Spectroradiometer (MODIS)-derived albedo (from 2000 to 2009). Following this, Brun et al. (2015) have successfully tested this method for the Chhota Shigri Glacier in the western Himalaya. However, its efficacy was low when applied over the Mera Glacier in the central Himalaya due to the presence of cloud cover, short field-based mass balance data, and availability of fewer pixels for average albedo estimation. A similar approach was attempted by Sirguey et al. (2016) to estimate the annual as well as the seasonal mass balance of the Brewster Glacier in New Zealand, where they observed a strong correlation (r2 = 0.93) between the average of annual minimum albedo over the whole glacier and annual mass balance, as well as a good correlation (r2 = 0.86) between cumulative winter albedo and winter mass balance.
Of the techniques discussed above, the geodetic mass balance method based on DEM differencing has been the most widely used over Himalayan glaciers. One initial study for deriving geodetic mass balance in the Himalaya is by Berthier et al. (2007). The authors used SRTM v2 and SPOT5 DEMs for estimating mass balance of glaciers around the Chhota Shigri Glacier located in Lahaul-Spiti region from 1999 to 2004 and obtained mass loss rates of −0.7 to −0.85 m w.e. a−1. The authors present a method to co-register the two DEMs but did not consider properly the SRTM radar penetration into ice. The authors simply assumed the SRTM DEM which was acquired in February 2000 to be the representative for end of the ablation season in 1999 due to some penetration into snow. However, subsequent studies found that the penetration of the SRTM-C band radar beam can be up to few meters (Gardelle et al., 2012, 2013; Kääb et al., 2012). Vincent et al. (2013), therefore, revisited the results but found only a difference of less than 0.1 m w.e. a−1 with respect to Berthier et al. (2007).
In the last two decades several studies have been published investigating the glacier mass balance starting from 2000 to recent years based on DEM differencing in various regions of the Himalaya. One of the earliest studies is Gardelle et al. (2013) who investigated eight different regions from the Pamir, to the Himalaya, to Hengduan Shan for the period 1999 to 2011. The authors considered the penetration based on the comparison of the SRTM-C band to the SRTM-X band. They found the highest mass loss in the western Himalaya and slightly (although non-significant) positive values in the Karakoram. The glacier mass balance for the whole of High Mountain Asia (HMA) between 2000 and 2016 was investigated by Brun et al. (2017) while relying on stereo ASTER data only (and thus circumvent the radar penetration issue). They reported a moderate mass balance in the Himalayan region, with the Bhutan region having the highest negative mass balance and lowest in East Nepal (Table 3). The mass balance estimates for HMA were further refined by Shean et al. (2020) who used DEMs derived from ASTER and very high-resolution stereo images such as Worldview-2/3. They report a higher mass loss in the Himalayan region during 2000–2018 as compared to the previous studies, with the highest rates over the eastern Himalayan region (Table 3). Recently Hugonnet et al. (2021), further improved the processing of ASTER DEM and related mass balance estimates and provided not only an estimate for the period 2000 to 2019 for all glaciers on Earth but also for four subperiods therein. They found an increasing mass loss since 2000 with higher overall mass loss as compared to the previous studies (Table 3).
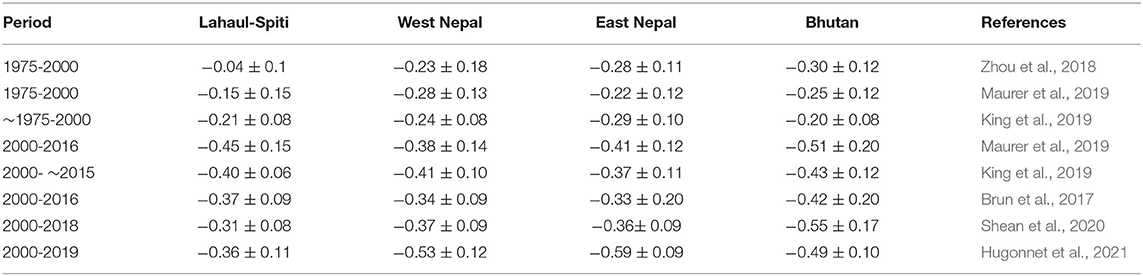
Table 3. Geodetic approach-based glacier mass balance estimates (m w.e. a−1) covering all of the Himalaya by various studies.
In order to better understand the long-term response of glaciers to climate change, longer and better data coverage is required. Declassified US spy stereo satellite images from the 1960s and 1970s provide an excellent opportunity in this regard. 1960s and 1970s high resolution (7–2 m) Corona KH-4 and KH-4B data were first used by Bolch et al. (2008, 2011) along with ASTER and Cartosat-1 data to investigate the mass balance evolution of the Mt Everest region in the Himalaya. 1970s Hexagon KH-9 metric camera data (8 m), first employed in HMA in the Tien Shan by Surazakov and Aizen (2010) and Pieczonka et al. (2013), was later used to improve the knowledge about glacier mass loss in the Himalaya and other regions of HMA as well. Zhou et al. (2018) applied these images along with the SRTM DEM for estimating the mass balance in some regions of the Tibetan Plateau and the Himalaya from 1975 to 2000. They reported a strong negative mass balance in most of the regions but only small negative values for Lahaul-Spiti (Table 3). This is in line with Mukherjee et al. (2018) who found similar insignificantly negative values for the region using 1971 Corona KH-4B and the SRTM DEM. Maurer et al. (2019) applied ~1975 Hexagon KH-9 and ASTER DEMs, while King et al. (2019) generated Hexagon KH-9 DEMs along with the 2000 SRTM and the ~2015 HMA DEM (Shean, 2017) to estimate the mass balance before and after 2000. Both studies found significant increase in mass loss after 2000 (Table 3).
King et al. (2020) used declassified imagery along with aerial images and Cartosat-1 data for up to 7 subperiods during 1962–2018 and reported increasing mass loss from −0.23 ± 0.13 m w.e. a−1 for 1962–1969 to −0.38 ± 0.13 m w.e. a−1 for 2009–2018. Bhattacharya et al. (2021) used various available stereo satellite data (in particular Corona, Hexagon and Pleiades data) to report mass changes for up to six subperiods within 1964 to 2019. The highest increase in mass loss was found for the Langtang region with −0.20 ± 0.09 m w.e. a−1 for 1964–1977 to −0.59 ± 0.14 m w.e. a−1 for 2017–2018.
Along with the aforementioned studies, there are several other studies were carried out in the Himalaya at individual glacier- or sub-region wise scale (e.g., Bhattacharya et al., 2016; Agarwal et al., 2017; Bhushan et al., 2017; King et al., 2017; Vijay and Braun, 2018; Gaddam et al., 2020). For a comprehensive review until 2017/18 we encourage readers to refer Azam et al. (2018) and Bolch et al. (2019a).
Glacier Melt and Mass Balance Modeling
Glacier surface mass balance and melt modeling has gained considerable attention in recent years, in part because of the availability of high-resolution gridded climate datasets (e.g., ERA5, ERA5-Land, HAR v2, CORDEX, etc.), and high-resolution satellite and DEM datasets (Azam et al., 2018). Also, the theoretical and physical understanding of various complex snow and glacier processes, such as influence of debris cover, long-term dynamic change, has improved significantly (Reid and Brock, 2010; Shea et al., 2015; Carenzo et al., 2016). Various surface melt and mass balance models have been implemented in the Himalayan region, such as hydrological models (Bhutiyani, 1999; Immerzeel et al., 2012), temperature-index model (Azam et al., 2014a), enhanced temperature-index model (Litt et al., 2019), albedo model (Brun et al., 2015), surface energy balance (SEB) model (Azam et al., 2014b), distributed SEB model (Arndt et al., 2021; Steiner et al., 2021; Srivastava and Azam, 2022), glacier dynamics model [Open Global Glacier Model (OGGM); Maussion et al., 2019], glacier evolution model [Python Glacier Evolution Model (PyGEM); Rounce et al., 2020]. However, the majority of the modeling studies use temperature-index models (Azam et al., 2021), with some modification for better representation (e.g., Pellicciotti et al., 2005). The temperature-index model is also known as the degree day model (DDM), because in them daily melt depth is computed by multiplying the number of positive degree days (often called cumulative positive degree days; CPDD) by the melt factor (Hock, 2003)This model is simple and can be applied over large areas/basins with limited data input requirements (Lutz et al., 2014; Azam et al., 2021). SEB models account directly for many of the physical processes that affect melt (e.g., surface melt, sublimation), but they require observations of several variables such as air/surface temperature, air pressure, short-wave and long-wave radiations, wind speed and humidity, etc. (Wagnon et al., 1999; Favier et al., 2004; Litt et al., 2019). Due to the scarcity of input data needed for SEB model, they are generally not suitable for modeling glacier mass balance over the Himalaya (Azam et al., 2014b).
Glacier mass balance models have been developed for a single glacier, for the entire basin as well as for the entire Himalayan region depending on the forcing and calibration data availability. For example, recently, Srivastava and Azam (2022) modeled the mass balance of Chhota Shigri (western Himalaya) and Dokriani Bamak glaciers (central Himalaya) for the past seven decades (1950–2020) using a temperature-index model forced with bias-corrected ERA5 data. Khadka et al. (2020) applied OGGM by integrating Glacio-hydrological Degree-day Model (GDM; Gupta et al., 2019) in the Koshi River basin (~3,000 km2 glacierised area) in the central Himalaya to estimate the glacier mass balance and the runoff. The model was forced with in-situ hydro-meteorological data and historical gridded data from the Climatic Research Unit (CRU). The model performance was good in simulating runoff (r > 0.8) and partitioning various runoff components. Rounce et al. (2020) applied the PyGEM model, forced with geodetic mass balance observations over the entire HMA region. Based on the model projection, they showed that by the end of the century, glaciers in HMA might lose between 29 ± 12% (RCP 2.6) and 67 ± 10% (RCP 8.5) of their total mass relative to 2015.
Glacier mass balance and melt models are extremely helpful to understand the glacier-scale to region-scale glacier mass balance behavior. However, in general, model outputs have large uncertainties because they are sensitive to the meteorological input variables and model parameters (Lutz et al., 2016; Rounce et al., 2020). Despite large uncertainty they have considerably improved our overall understanding of spatiotemporal variability in the Himalayan water resources.
Satellite Gravimetry
The Gravity Recovery and Climate Experiment (GRACE) mission and its successor GRACE Follow On (GRACE-FO, launched in 2018 after the end of GRACE in 2017) are a pair of identical satellites co-orbiting in the same plane (Tapley et al., 2019). The two satellites measure the change in the distance between them via a microwave link, which is a function of the gravity field of the Earth. The GRACE mission provided changes in the gravity field of the Earth at monthly and sub-monthly scale, which can be related to the surface mass changes (Tapley et al., 2019).
The gravity field can be recovered from the satellite mission either as blocks of masses (also called mascons) or as spherical harmonic coefficients (Tapley et al., 2019). The unconstrained spherical harmonic coefficients are noisy and require filtering prior to using GRACE data (Swenson and Wahr, 2006), but filtering introduces leakage and diminishes the signal amplitude (Vishwakarma et al., 2016, 2017). Metrics have been designed to understand the efficacy of filtering (Devaraju and Sneeuw, 2016), and methods have also been developed to account for leakage and the amplitude loss (Vishwakarma et al., 2018). It must be noted here that the mascon solutions are also filtered versions of the GRACE data, where filtering is performed with a regularization scheme that aims to minimize signal leakage with the help of prior information.
One of the most contentious issues with the use of GRACE-FO is its spatial resolution. A wide range of numbers have been advocated for indicating the spatial resolution, for example, Longuevergne et al. (2010) proposed a value of 200,000 km2 and Vishwakarma et al. (2018) indicated 63,000 km2 if the application can tolerate an error of 2 cm. It was also shown that the spatial resolution depends on the method used for filtering and leakage correction (Vishwakarma et al., 2018). For the spherical harmonic solutions Devaraju and Sneeuw (2016) devised the method of modulation transfer functions to identify the spatial resolution. However, for the case of mascons, the spatial resolution is believed to be at a spatial scale of 300 km. Despite these issues, it is known that GRACE-FO can observe variations in the gravity even at small spatial scales, if the gravity anomaly is large enough (Sneeuw and Sharifi, 2015). In this context, it has been shown that small spatial scale variations require dedicated modeling of the range acceleration signal obtained from the level 1-b data of the satellite mission (Weigelt, 2017; Ghobadi-Far et al., 2020).
The GRACE derived total water storage (TWS) anomaly includes changes in the soil moisture, canopy water, surface water, snow water, and groundwater (Tapley et al., 2019). Therefore, to arrive at one component, such as either ice mass balance or groundwater change, it is necessary to remove other storage components usually obtained from a model simulation that can have high uncertainty (Tiwari et al., 2009; Chen et al., 2015; Long et al., 2016). Therefore, using GRACE data for estimating mass changes over a glacier, which is much smaller than the spatial resolution of the observation, or in a groundwater aquifer is challenging (Wang Q. et al., 2018; Vishwakarma, 2020). Nevertheless, there have been some efforts in quantifying glacier mass change in the HMA using the point mass modeling approach, which gives an estimate for the whole region but with very high uncertainty. For example, Jacob et al. (2012) estimated the mass loss at a rate of −4 ± 20 Gt/yr from 2003 to 2010, while a recent study by Wouters et al. (2019) reported a trend of −13.5 ± 6 Gt/yr for the complete GRACE time series.
GRACE has been found more useful at studying basin-scale hydrology. While there are spatial and temporal resolution issues with GRACE data, it has proved useful in downstream regions where groundwater is being exploited rapidly (Chen et al., 2016). In addition, like any other remote sensing product, there is a need to validate the GRACE data with groundwater monitoring data from government agencies, farmers and stakeholders. In the past, the use of crowd sourced farmer data for groundwater management using the Mywell App has been successful in creating a high-resolution database for groundwater level data at the village scale (Maheshwari et al., 2014; Maheshwari, 2020). Preliminary results indicate that the locals can aid in better management of groundwater resources and can also augment rural database by providing crowd sourcing of data along with GRACE/GRACE-FO data.
Another geodetic technique that has recently emerged as a tool for monitoring water resources near glaciated regions is Global Navigation Satellite System (GNSS). It has been used for monitoring glacier mass change, lake water level, and surface deformation due to hydrological mass change (Dunse et al., 2012; Durand et al., 2019; Elliott and Freymueller, 2020; Koulali et al., 2022).
Global Navigation Satellite Systems
GNSS enable the most ubiquitous methods of geodetic positioning. It uses the known positions of the constellation of satellites to determine the position of the GNSS receiver at sub-cm accuracies. The precise positioning is based on the carrier phase pseudo range equation given by Hofmann-Wellenhof et al. (2007).
where φ is the carrier phase, ρ is the true range between the satellite and the receiver, λ is the wavelength of the carrier, N is the integer number of cycles, c is the velocity of light, δt is the clock error, T is the delay due to the troposphere, and I is that due to the ionosphere, M is the multipath error, S is the combined term of all other systematic errors like antenna phase center error and hardware delays in the satellite and the receiver, and ε is the random error associated with the phase measurement. The term ρ contains the position of the receiver and the satellite, and the terms T and M provide important environmental information.
In GNSS monitoring of glaciers, the position information from GNSS is sensitive to the movement of the glacier and surface elevation changes due to the load changes associated with glacier mass change. The tropospheric delay term T provides information concerning the precipitable water vapor (PWV), which is typically absorbed into weather models for forecasting. The multipath term M is the reflected GNSS signal. Since the GNSS uses a microwave L-band for sending signals, the reflected signal contains information concerning the backscattering properties of the surface. This has been successfully used for retrieving snow height information in glaciers and mountainous areas alike (Larson, 2016). Thus, GNSS can provide a variety of vital information for monitoring snow and glaciers.
The observation strategies vary for the different types of variables that can be retrieved from GNSS positioning. For understanding loading due to glacier mass change, it is typical to have a continuously operating network of GNSS stations in and around the glacier (Jacobsen and Theakstone, 1997). Such networks can also provide PWV estimates, but to perform GNSS reflectometry with them, the sites with reasonably flat terrain have to be chosen (Larson, 2016). For monitoring glacier movement and shape, real-time kinematic (RTK) GNSS measurements can be conducted in periodic campaigns. DEMs can also be constructed to identify surface elevation changes of the glacier (Jacobsen and Theakstone, 1997). With the advent of low-cost GNSS receivers and antennas, more observations are being retrieved that are used for deriving novel inferences. For example, Koch et al. (2014) and Henkel et al. (2018) planted a low-cost GNSS antenna on a snow-free area in the summer months and estimated the winter snow depth from distorted GNSS signals. The low-cost GNSS receivers perform as good as the geodetic receivers (Odolinski and Teunissen, 2016), and therefore, they can be used for the densification of GNSS networks in the snow and glaciated areas.
Glacier Ice Thickness Modeling
Glacier ice thickness and ice reserves are estimated by in-situ measurements, power-law relations, physical models, and artificial neural networks (ANN) (Zemp et al., 2019; Haq et al., 2021; Millan et al., 2022). In-situ measurement of ice thickness such as borehole drilling, radio-echo sounding and ground-penetrating radar (GPR) is often costly, logistically demanding, and unsafe due to the rugged nature and harsh climatic conditions of the glaciated terrain (Saintenoy et al., 2013; Bohleber et al., 2017). The next approach is based on Volume Area Scaling (VAS) (Bahr, 1997). VAS is suitable for obtaining a general ice thickness pattern for most of the glaciers (Bahr et al., 2015), but requires calibration from a DEM and estimates of glacier extents (Möller and Schneider, 2010; Laumann and Nesje, 2017; Banerjee, 2020). It is to be noted that VAS cannot give information regarding the distribution of ice thickness. As a result, glaciologists have begun to rely on ice thickness models that use multi-temporal, multi-spectral and high spatial resolution satellite data to represent varying complexities of the glacial environment (Farinotti et al., 2021).
Types of Glacier Ice-Thickness Models
Recent work by the Ice Thickness Models Intercomparison eXperiment Phase 1 and Phase 2 (ITMIX1 and 2) evaluated all the models developed so far for the mountain glaciers. Several models exist that rely on different principles, viz., (1) using the shallow ice approximation (SIA) and an empirical relation between glacier elevation range and basal shear stress (Haeberli and Hoelzle, 1995) at the local scale; (2) flowline-based approach considering mass conservation and Glen's ice flow law (Glen, 1955); (3) two-dimensional consideration of the continuity equation (Morlighem et al., 2011). Apart from these, recent developments in data sciences have led to models that that do not rely on the physics of the problem, such as the Artificial Neural Network (ANN) approach (e.g., Clarke et al., 2009; Mey et al., 2015; Haq et al., 2021). Table 4 provides an up-to-date list of ice thickness models that have been used in the Himalaya.
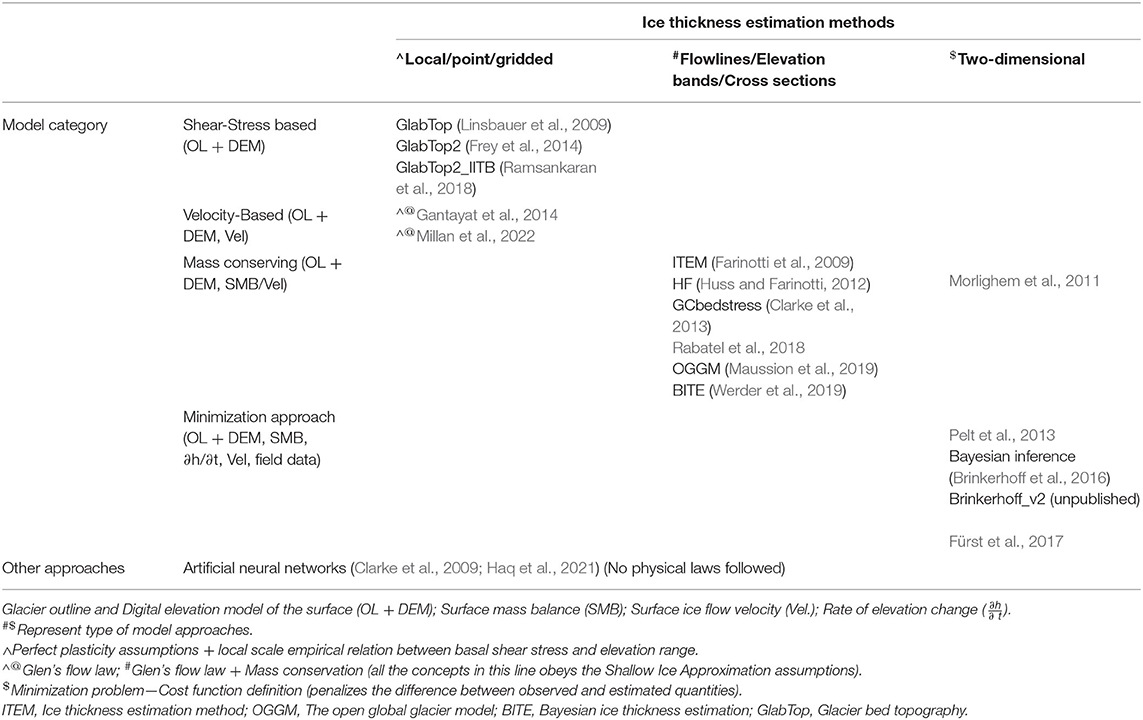
Table 4. List of model categories and mode of estimating ice thickness. Brackets within the model categories represent the remote sensing-based inputs used in the model.
Recent Advancements in Ice Thickness Modeling
The applicability of ice thickness models has evolved significantly due the abundance of satellite remote sensing datasets (e.g., LiDAR, Optical and SAR images) to the development of automated models like the Volume and Topography Automation (VOLTA; James and Carrivick, 2016), the Open Global Glacier Model (OGGM; Maussion et al., 2019), and ice velocity and slope-based methods (Millan et al., 2022). On the other hand, like the Glacier Thickness Database (GlaThiDa; Welty et al., 2020)—a global archive for in-situ ice thickness datasets and Global Glacier Thickness Initiative (G2TI; Farinotti et al., 2019)—have opened gateways for exploiting the maximum potential of the ice thickness models. Farinotti et al. (2021) have shown that calibrating the ice thickness models with field observations (even limited) and ensemble-approach is beneficial in terms of improving accuracy and robustness compared to individual model estimates. Millan et al. (2022) have recently modeled the ice thickness for all the glaciers on Earth using high-resolution ice velocity and topographic data based on the SIA approximation. The re-evaluated ice volume estimates by Millan et al. (2022) is the most comprehensive estimate because they rely on recent DEMs and satellite remote sensing image. The next best attempt was by Farinotti et al. (2019) that were based on at least a decade old dataset.
Recent Estimates of Ice Thickness Over the Himalaya
Studies related to ice thickness date to the 1960s (Raina, 2009), and field investigations are still ongoing in sporadic nature at accessible glaciers in the Himalaya. However, only a few glaciers are being surveyed using GPR (e.g., Azam et al., 2012; Vincent et al., 2016; Mishra et al., 2018; Pritchard et al., 2020). GPR survey-based ice thickness measurements are available for only around 21 glaciers and that too at different time intervals (Kulkarni et al., 2021). Therefore, VAS and ice thickness models with inputs from glacier inventories (e.g., GLIMS, RGI, etc.) and remote sensing paved the path to study glaciers at a relatively larger scale than with the GPR (e.g., Sattar et al., 2019; Pandit and Ramsankaran, 2020).
The VAS approaches were used to estimate glacier ice volume for the entire Hindu-Kush Himalaya range with the help of the RGI glacier outlines. However, the estimation of glacier ice volume varied from one study to the other due to a different set of parameters being used in different studies (Radić and Hock, 2010; Marzeion et al., 2012; Grinsted, 2013). Bolch et al. (2012), Frey et al. (2014), Linsbauer et al. (2016), and Farinotti et al. (2019) were able to estimate the total glacial volume of the HK region using ice thickness models for the year 2000. Bolch et al. (2012) reviewed VAS models and provided an updated estimate based on a shear-stress model. Similarly, Frey et al. (2014) and Linsbauer et al. (2016) also employed the shear-stress based model, while Farinotti et al. (2019) used an ensemble of ice thickness models to provide a consensus estimate of 2.87 ± 0.74 × 103 km3 and 0.88 ± 0.23 × 103 km3 of ice volume for RGI subregion 14 (South Asia West; Figure 1) and 15 (South Asia East) that covers the entire Hindu-Kush Karakoram Himalayan range). Surprisingly, the results of Millan et al. (2022) are 37% higher than the Farinotti et al. (2019) consensus ice volume estimates for RGI subregions (13, 14, and 15) that covers HMA. These difference between different studies reveal the inconsistency in the available ice thickness estimates for the glaciers in the HMA and in other regions as well. Hence, there is a need for developing new models with better strategies on utilizing variety of the available observations. Table 5 provides a summary of the estimated ice volume for the Himalayan region from various methods and sources.
Estimates of ice thickness is a crucial input for glacier models that are driven by climate models to project future glacier mass balance. Climate model projections of future glacier mass balance over the Himalaya produce consistent results and suggest that glaciers in the region will lose up to 90% of their mass by 2100 (Barsch and Jakob, 1998; Kraaijenbrink et al., 2017; Bolch et al., 2019a; Shannon et al., 2019). Based on these projections, there is considerable concern among policymakers and infrastructure planners about future water supplies to the wider region and the potential for increased glacier-related hazards impacting populations and infrastructures in the high mountain valleys (Huggel et al., 2020; Immerzeel et al., 2020).
Climate Models Based Mass Balance of Karakoram-Himalayan Glaciers
Various parts of the Earth system, such as oceans, atmosphere, cryosphere, land surface dynamics, and human systems, interact and influence each other. Therefore, Global Climate Models (GCMs) were developed to include all possible sub-systems, their interactions, and response to any change in the Earth system.
GCMs are computationally expensive and run at a coarse spatiotemporal resolutions, which reduces their efficacy at a regional scale. Testing and calibrating GCMs require high-resolution present-day climate data along with well-constrained information on past climates of critical regions worldwide, which includes changes in the glacier extent and mass balance. So far, the terrestrial cryosphere is represented in a highly simplified way in majority of the state-of-the-art global and regional climate models (RCMs) as they use static glacier masks, i.e., (i) no changes in ice extent, no feedback to the atmosphere; (ii) no consideration of water volume stored; (iii) either no or simplified runoff generation (see Figure 3; Kotlarski et al., 2010; Kumar et al., 2015, 2019). Therefore, a more sophisticated approach is necessary to overcome the poor representation of the glacier processes in climate models.
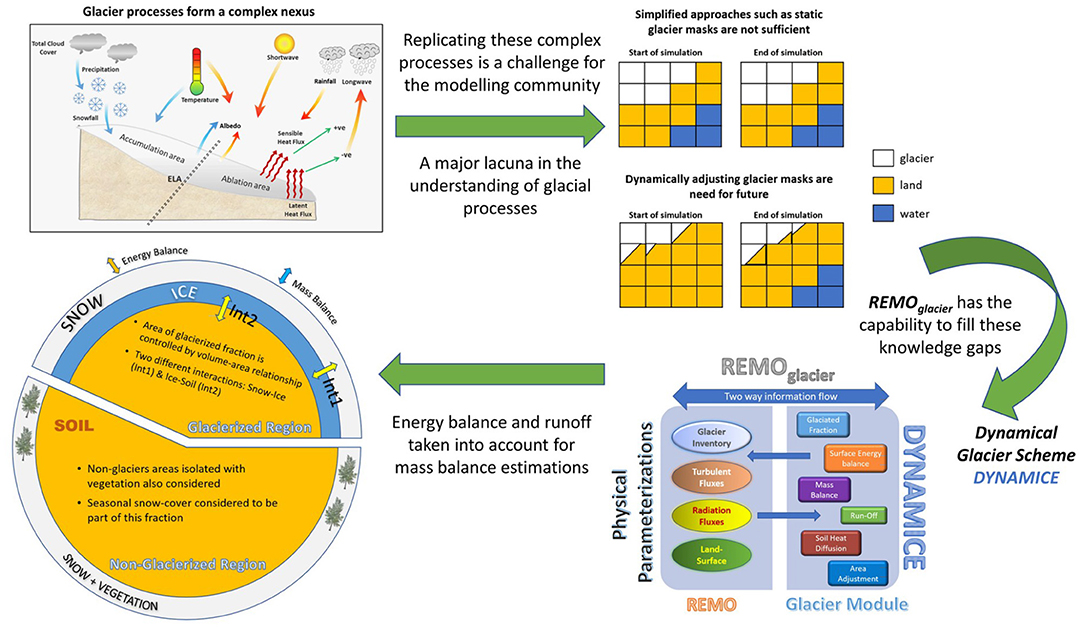
Figure 3. A schematic representation of the complex glacial processes involved in mass-balance estimations (top left). The difference in simplified (static glacier masks) and sophisticated (dynamical glacier masks) approaches (top right). Description of the dynamical glacier scheme DYNAMICE and the regional model dynamics of REMOglacier (bottom right). Different model dynamics and parameterisation schemes operate separately for glacierised and non-glacierised regions (bottom left).
RCMs, owing to their more nuanced spatiotemporal resolutions, act as better alternatives for regional studies. Within the framework of the Coordinated Regional Climate Downscaling Experiment (CORDEX), a regional earth system model comprising of REgional atmospheric MOdel (REMO), Max Planck Institute's Ocean Model (MPIOM), and a hydrological discharge (HD) model, coupled using OASIS coupler, has been developed for the CORDEX-SA (South-Asia) domain for climate change studies. This setup is called the Regional Earth System Model (RESM; Kumar et al., 2022). The proper description of cryospheric processes is essential for simulating the complete terrestrial water cycle in climate models, especially for the high mountain regions.
Owing to various socio-economic, topographical, and political constraints, attempts for a holistic study of Hindu-Kush Karakoram Himalayan glaciers have been very sporadic, undermining the fact that the region suffers from an acute shortage of quality observational datasets, both spatial and temporal. In RCM REMO, a dynamical glacier scheme (DGS) was implemented that has the unique ability to simulate the glacier mass balance for even dynamic fraction of each grid box depending on the accumulation and ablation conditions, while accounting for direct physical feedback mechanisms. The scheme represents surface glacier cover on a subgrid-scale and calculates the energy and mass balance of the glacierised part of a grid box (Kotlarski, 2007; Kotlarski et al., 2010; Kumar et al., 2015, 2019). This coupled model system is referred to as REMOglacier. It has been applied for the Himalaya to show computational effectiveness. Recently, several studies (e.g., Kumar et al., 2015, 2019; Javed et al., 2022) have started to assess the impact of large-scale and micro-climate on Himalayan glaciers, focusing on area and mass balance changes at a sub-grid scale.
Snow Cover, Snow Depth, and Snow Water Equivalent Variability Over the Himalaya
In addition to ice-related processes, snow cover has significant spatial and temporal variations over the Himalaya. Snow cover is regulated by seasons, topography, and hydrometeorology (Singh et al., 2014; Gurung et al., 2017; Rathore et al., 2018). Different techniques were proposed to estimate snow cover over different regions. Some of these techniques were adopted for snow cover monitoring over the Himalaya, and they were reported in Sood et al. (2020): normalized difference snow index (NDSI) (Sharma et al., 2012; Rathore et al., 2018), change detection (Singh S. et al., 2021), pan-sharpening (Singh et al., 2020), and snow cover mapping using snow depth maps (Gusain et al., 2016). Other than these techniques, snow cover mapping with interferometry coherence analysis using synthetic aperture radar (SAR) data (Kumar and Venkataraman, 2011) and, backscatter ratio-based technique (Thakur et al., 2013) have also been used over different sub-basins in the Himalayan region. The backscatter ratio from wet snow was calculated with the help of reference image from dry snow season. Different threshold values for different landcover values are reported for mapping the wet snow (Thakur et al., 2013). RISAT-1 data (~50 m resolution) in HV (vertical transmit and horizontal receive) polarization is used for deriving timeseries of snow cover area (SCA) maps over Beas and Bhagirathi river basins during 2013–2014 (Thakur et al., 2017). The accuracy of the SCA was 95% when compared with the maps prepared using optical data. These studies (Kumar and Venkataraman, 2011; Thakur et al., 2013, 2017) have demonstrated the potential of snow cover mapping using active microwave in Himalayan region. However, majority of the studies have focused mainly on using the optical data in the Indian Himalayan region for mapping snow cover and studying its variability (Sharma et al., 2012; Ahmad et al., 2020). The Himalayan snow cover does not have a uniform pattern or trend. In the upper parts of the Indus, the Ganga and the Brahmaputra basins, the snow cover area varies from 85 to 10% of the total area during ablation season (Singh et al., 2014). There is no trend in the mean snow cover over the Himalaya, however, at a regional scale, there is a statistically significant declining trend over small basins of Jhelum, Kosi, Manas, Gandaki, and Chandra (Gurung et al., 2017; Sahu and Gupta, 2020). For instance, in the low-elevation Ravi Basin, the snow cover decreases in mid-winter from 90 to 55 %, however, in the high-elevation Bhaga Basin, snow cover does not subside until April (Kulkarni et al., 2010). There is a shift in snow cover trends after 2010 and a deceleration in snow/ice cover shrinkage during recent years in the north-west Himalaya (Singh et al., 2018). However, due to large inter-annual fluctuation, snow depth depletion is not considered in snow cover variability, especially when the whole Himalayan range is examined (Gurung et al., 2011; Rathore et al., 2018).
The present synthesis shows that procuring a trend in snow cover changes is challenging for the Himalayan region due to the limited number of observations, substantial annual and inter-annual variability, and scarce in-situ snow depth area data (Kulkarni et al., 2021). However, overall, it is widely reported that depleting snow cover and its spatiotemporal changes are associated with changes in climatic variables over the Himalaya (Sood et al., 2020; Desinayak et al., 2022). Understanding variability of snow cover with respect to altitude and air temperature is vital for assessing availability of regional water resources and the impact of climate change in the Himalaya (Immerzeel et al., 2010; Shrestha et al., 2015). Moreover, the snow cover variation patterns, e.g., accumulation and ablation can potentially lead to imbalance in glacier mass balance and can trigger changes in water availability in different river basins (Kaser et al., 2010; Bolch et al., 2012).
Along with snow cover, snow depth (SD) and snow water equivalent (SWE) are also equally important in understanding regional hydrological cycle, and quantifying water availability in the Himalayan region. Annual snow water storage trends observed during 1987–2009 using Scanning Multichannel Microwave Radiometer (SMMR) data present a negative trend over Himalayan region (Smith and Bookhagen, 2018). The major contribution to SWE comes from mid-elevation range, i.e., 4,000–5,000 m a.s.l. in the Himalayan region and strongly affects the meltwater discharge. SWE in this elevation range has been shown to exhibit a strong negative trend (Smith and Bookhagen, 2018) along with shift in seasonality (Panday et al., 2011; Smith et al., 2017). Though exact mechanism behind these changes are not completely understood yet, few studies emphasized that aerosol contamination (Lau et al., 2010), changing precipitation patterns (Lutz et al., 2014), changes in western disturbances (Cannon et al., 2015), and evolving temperature dynamics could be the potential drivers behind SWE changes. Negative SWE trend can potentially impact the downstream water availability in the Himalayan region.
Monitoring of SD and SWE depends on the availability of a dense gauge network, but what we have is rather poor due to operational challenges. Remote sensing datasets, particularly microwave datasets [e.g., Advanced Scanning Microwave Radiometer for Earth observation (AMSR-E), Microwave Emission Model for Layered Snowpacks (MEMLS)] remains one of the most reliable techniques for obtaining SD and SWE in the Himalayan region. Various approaches consisting of non-linear models (Wang Y. et al., 2019), physically based models (Liu et al., 2014), data driven approaches (Xiao et al., 2018; Yang et al., 2021) and assimilation-based approaches (Stigter et al., 2017; Kwon et al., 2019) has been applied in different studies for estimating SD and SWE. However, correcting the radar penetration issue is one of the biggest challenges in such remote sensing datasets (Tiwari et al., 2016; Patil et al., 2020; Awasthi et al., 2021).
Rock Glaciers in the Himalaya
Rock glaciers are lobate or tongue-shaped assemblages of ice-rich angular debris that moves or slowly creeps downslope due to gravity (Owen and England, 1998; Haeberli et al., 2006; Janke and Bolch, 2022). The surface velocity of active rock glaciers varies between 0.1 m to a few meters per year. Rock glaciers contain significant amounts of ice and are climatically more resistant than glaciers due to the thick insulating debris (Jones et al., 2019a; Janke and Bolch, 2022). Even though the origin of the ice is debated they are commonly considered as permafrost landforms (Berthling, 2011). Rock glaciers are gaining more attention because of their importance in the mountain water cycle in particular with the expected reduction of the glacier water resources (Harrison et al., 2021; Janke and Bolch, 2022).
Rock glaciers are also an important component of the debris-transport system and their characteristics and occurrence is strongly influenced by the debris supply (Barsch and Jakob, 1998; Janke and Bolch, 2022). Rock glaciers can potentially transition from debris-covered glaciers especially in permafrost environments (Haeberli et al., 2006; Monnier and Kinnard, 2017; Jones et al., 2019b). Characteristics and evolution of debris-covered glaciers and their transition from clean -ice glaciers are also determined by debris supply. Hence, knowledge about the evolution of mountain slopes and geomorphological processes (such as valley-ridge scale interactions including rock slope failure and degradation of lateral moraines) that operate glacial and periglacial systems and how they impact the glaciers and rock glaciers are crucial. However, the ice-debris systems vary as the systems change in response to climate forcing. As a result, viewed from the land system perspective, a debris-ice land system incorporates numerous processes that respond to the climate in different ways over time.
Climate model projections resolve fundamental climatic and terrestrial processes only at broad scales; often around 100 km or so. Even downscaled products such as CORDEX-SA (South Asia) produce projections at around 25 ×25 km grids (e.g., Tangang et al., 2020). As a result, such projections at these spatial scales are challenging in resolving many of the geomorphological processes that operate in glacial systems, and which might affect their response to climate forcing.
For land surface models to enhance climate model projections of glacier mass balance and subsequent water supplies, they will need to more accurately account for paraglacial processes and be able to more accurately project the future evolution of debris-covered glaciers and rock glaciers to assess which glaciers will undergo the transition to debris-covered glaciers and rock glaciers, and which glaciers will not. The implications of such processes for future water supplies are likely profound. Recent research (Jones et al., 2021) has identified ~25,000 rock glaciers across the Himalaya. Most are located within the central Himalaya (~40%, n = 10,060), with ~30% situated in the eastern Himalaya and ~29% in the western Himalaya. The estimated areal coverage of rock glaciers across the area is 3,747 km2 (i.e., intact and relict), representing ~16% of that covered by glaciers (22,829 km2). Water volumes are estimated as 51.80 ± 10.36 km3, which translates to a rock glacier: glacier WVEQ (water volume equivalent) ratio in the central Himalaya of 1:17, with lower ratios in other regions. However, in some regions (e.g., in western Nepal), rock glacier WVEQ ratios reduce to between 1:3 and 1:5, suggesting strong regional contrasts in their significance. Also, rock glaciers are being mapped regionally, e.g., Bolch et al. (2022) identified 370 rock glaciers which cover an area of more than 10% of the total glacier area in the Poiqu basin in the Central Himalaya in Tibet. Pandey (2019) estimated 516 rock glaciers covering ~350 km2 area in Himachal Pradesh (northern India). Other forms of buried ice (i.e., ice-rich permafrost, ice-cored moraines, can also contain a significant amount of ice (Bolch et al., 2019b). No estimates have been made of the amount of ice in other non-glacial landforms, but it has been argued that this is likely to be substantial and should be accounted for better understanding the water resources in the Himalaya (Harrison et al., 2021; Janke and Bolch, 2022).
Permafrost in the Himalaya and Surrounding Areas
Permafrost is commonly defined as rock or soil with a temperature below 0°C for more than two consecutive years. Permafrost ground often contains ice lenses or ice located in pores of rock and soil. Hence, permafrost impacts hydrology but also slope stability. Thawing permafrost can destabilize mountain slopes leading to hazards and impacting geomorphological processes (Krautblatter et al., 2013; Gruber et al., 2017; Bolch et al., 2019a).
In the Himalaya, most of the existing studies about the permafrost are from remote sensing methods. In-situ studies confirming the permafrost presence in the Himalaya are still rare (Gruber et al., 2017; Wani et al., 2020). A surface temperature model suggests that the total permafrost area in the HKH (including the Hindu-Kush and Tibetan Plateau) is 16 times higher than the HKH glacierised area (Gruber, 2012; Gruber et al., 2017). Another study suggested that rock glaciers can be used as a proxy to infer the existence of permafrost (Schmid et al., 2015).
Cryospheric components —including snow, glacier and permafrost— release meltwater from seasonal to multi-century frozen storage and control the spatiotemporal changes in the river runoff. Therefore, in a warming climate, permafrost thawing is expected to have similar impacts on river runoff like snow and glaciers (Hewitt, 2014; Biskaborn et al., 2019; Wang C. et al., 2019). However, unlike glaciers, detailed studies on ice rich permafrost are not yet available in the Himalayan region. As a result, permafrost is not yet included in the hydrological modeling framework, and the impacts of permafrost thaw on the hydrological cycle remain unknown in the Himalayan region (Azam et al., 2021).
In some other parts of the Northern Hemisphere including the neighboring Tibetan Plateau, studies suggested permafrost degradation under recent climate change has resulted in hydrological changes, destabilization of rock glaciers, and frequent landslides (Zhang and Wu, 2012; Kargel et al., 2016; Rogger et al., 2017). These studies underscore the importance of including permafrost while designing frameworks for monitoring and assessing impact of climate change over catchments in the Himalaya (Azam et al., 2021). Understanding the volumetric and seasonal shifts in river runoff due to contribution of permafrost thaw would require a precise assessment of permafrost distribution, volume, and freeze/thaw processes (Azam et al., 2021). To fill these gaps, techniques should be developed that assess permafrost mass changes at large-scale by integrating time-varying changes of gravity with the GRACE satellites, digital elevation model differencing from multispectral images, and InSAR; while catchment-scale in-situ measurements can be used to validate satellite measurements (Azam et al., 2021). These in-situ data may include those from the automatic weather stations (Wani et al., 2020), geophysical surveys measuring electrical resistivity, data from ground penetrating radar, and temperature measurements from boreholes (Sjöberg et al., 2015; Wang S. et al., 2018; Wang C. et al., 2019).
Data Integration and Assimilation
Combining different datasets can help in improving the data quality and also in determining an unknown variable when the others are known. It has been shown that the effective resolution of a dataset can be improved by assimilating information at better spatial resolution (Reichle et al., 2001; Houborg et al., 2012; Peng et al., 2017; Miro and Famiglietti, 2018). Various data assimilation techniques have been devised and successfully implemented, such as improving operational weather forecast, predicting ocean dynamics, and modeling soil moisture content (Jackson et al., 1981; Peng et al., 2017) in streamflow estimates (Patil and Ramsankaran, 2017, 2018). Recently several studies have assimilated hydro-geodetic data and hydrological models to estimate, calibrate, or validate hydrological flux variables. For example, modeling river runoff with the help of hydro-geodetic approaches (Tourian et al., 2013; Sneeuw et al., 2014), estimating catchment-scale water budget using a Kalman filter framework (Pan and Wood, 2006; Lorenz et al., 2015), and calibration or/and validation of hydrological model outputs using GRACE datasets (Döll et al., 2014; Eicker et al., 2014). The ensemble Kalman filter approach has been used effectively to assimilate GRACE TWSC into a Land Surface Model (LSM) to improve model performance (Zaitchik et al., 2008; Houborg et al., 2012; Eicker et al., 2014). Several non-parametric methods have also been proposed to improve spatiotemporal knowledge of hydrological variables. For example, Sun (2013) predicted groundwater level changes by incorporating GRACE with hydro-meteorological variables in an ANN framework. Long et al. (2014) demonstrated the efficacy of ANN to predict TWSC from precipitation, soil moisture, and temperature, and Seyoum and Milewski (2017) used ANN to produce high-resolution TWS estimates. Recently Vishwakarma et al. (2021) used multivariate regression to improve the resolution of GRACE satellite products to half degree grids. Such higher spatial resolution datasets are required to better attribute spatiotemporal changes in water mass redistribution.
Data integration can also be carried out by using budget equations that rely on the conservation of mass or energy to define relations between various physical observables. These equations can be used to estimate one variable when others are known, and such a dataset is a derived dataset. For example, the water budget equation writes precipitation in a catchment as a sum of storage change, evapotranspiration, and runoff. If any three of these variables are known, then the fourth dataset can be estimated (Sneeuw et al., 2014; Lehmann et al., 2022). Such approaches have been used to estimate evapotranspiration, which, when compared to evapotranspiration from models, can provide an assessment of the accuracy of the model. It is to be noted that the efficacy of such an approach is limited by the accuracy of the variable with the highest uncertainty.
Major Gaps
The Himalaya has the largest glacierised area outside poles, and therefore, it requires a massive collaborative effort to measure a representative number of glaciers in the field. Working on Himalayan glaciers is challenging, and only about 36 glaciers have been studied so far for mass balance (Table 1), which constitute about 1% of the total glacierised area in the Himalaya (Bolch et al., 2012; Azam et al., 2018). Therefore, the biggest gap is the lack of glacier in-situ mass balance data from the region. Likewise, our current knowledge of the volume of water stored and ice thickness distributions in individual glaciers is also poor (c.f. Figure 4). Hence, one of the fundamental research questions in front of us is “How are climate change and glacier volume responses affecting the glacier movements of Himalayan glaciers?” So far, this is still under investigation and has been recently highlighted by Azam et al. (2021). In Figure 4 we show a schematic that dissects our current and required knowledge in the spatial and temporal domains. We are yet to obtain a comprehensive understanding of frozen water at various spatiotemporal scales.
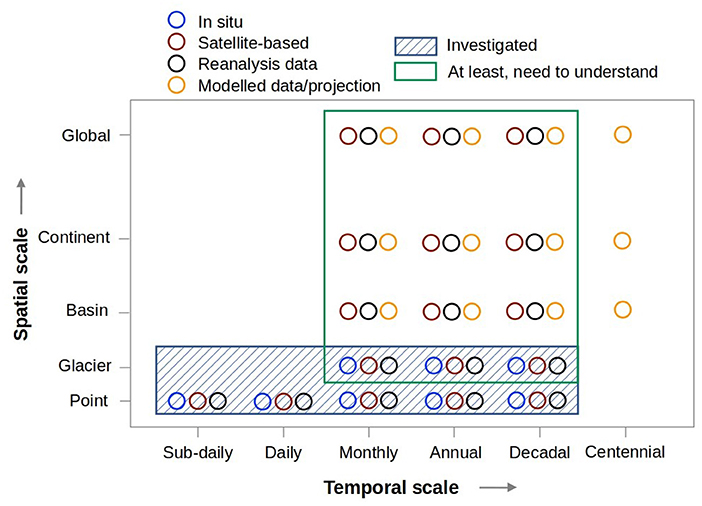
Figure 4. Spatiotemporal timeline of research investigation and understanding of the cryosphere and regional water resources in the Himalayan region. Relative data availability from various techniques/methods are also shown.
Another gap is the lack of in-situ meteorological datasets that are required to model glacier mass balance accurately. Temperature data from several reanalysis, gridded and model datasets (ERA5, ERA5-Land, APHRODITE, CRU, HAR, among others) are the best available options (Kumar et al., 2015, 2019; Kanda et al., 2020). However, precipitation values from these sources are either over or underestimated due to the unpredictability of precipitation in high altitude regions (e.g., Immerzeel et al., 2015; Wortmann et al., 2018). Also, information from the inaccessible areas and avalanche contribution to glacier mass balance is typically ignored (Laha et al., 2017).
The downstream countries would be affected the most by changes in the Himalayan water storage. There are several hydro-power projects being developed that would be severely affected by changes in water availability. Recently several water-related disasters were reported that were triggered by sudden release of large water volumes, e.g., the Chamoli disaster in 2021 (Shugar et al., 2021) and the Kedarnath disaster in 2013 (Dobhal et al., 2013b; Allen et al., 2016). Although the communities in the downstream countries recognize the need for improving the quantity/quality of the observation data, but still there is a lack of cooperation between surface water and groundwater management agencies (including trans-boundary, inter-and intra-government agencies). In such scenarios, data from remote sensing platforms (e.g., Altimeters, GRACE) can be used to understand the state of water use. However, due to poor spatiotemporal resolution of remote sensing data (c.f. Figure 4), there is a need for exploring data-integration or assimilation methods that may predict hydrometeorological variables of interest at various scales. It is also recommended to develop a framework for upscaling the use of crowd sourcing-based data and remote sensing data, along with observation data from government agencies to formulate management plans.
Challenges and Future Research Directions
We have made significant advances in our understanding of water resources variability in the Himalaya and downstream during the past few decades. These advances resulted from an improved understanding of the physical processes and dynamics and the advances in data collection and automation. However, we still need to overcome some of the challenges to direct future research.
Installing more weather stations and collecting long-term continuous in-situ data at different elevation over the Himalayan regions for a comprehensive spatiotemporal coverage is needed. Instrumentation and data collection schemes should be developed to monitor changes in glacier mass, snow hydrological processes and permafrost storage. In-situ meteorological observations are sub-daily, while glaciological measurements are usually seasonal or annual, but both are at point scale. Remote sensing can help us obtain spatially consistent, sub-monthly or monthly time-series data. Hence, there is an eminent need to develop frameworks that can assimilate/integrate both in-situ and multi-satellite remote sensing observations to create high resolution time-series estimating various hydro-glaciological processes.
Models are an invaluable tool for predicting future water availability and understanding the role of individual drivers. Current climate models lack in representing feedback processes involving human interferences, snow and glacier processes, and the corresponding basin runoff responses. For example, at the regional scale, coupled glacier-climate model setups such as REMOglacier have already shown good potential in replicating the observed glacier mass-balance variability. Furthermore, estimation of uncertainties in model outputs must be improved. Ensemble modeling, for example, has been shown to provide a realistic assessment of uncertainty range.
Geodetic observations can provide critical data for monitoring snow, glaciers, surface, and sub-surface water storage. The low-cost GNSS receivers can be deployed as they provide a wide variety of information on snow and glaciers. Particularly, a dense network of GNSS stations that can also perform GNSS reflectometry will be of immense value for snow and glacier monitoring in the Himalaya. The development of micro-electromechanical-system (MEMS) based gravimeters (Carbone et al., 2020) should make in-situ gravity measurements cheaper. It must be noted that terrestrial gravimetry is the most underutilized of all the geodetic techniques in glacier monitoring. Dedicated modeling of GRACE-FO data for retrieving Himalayan glacier mass change must be pursued to extract data for nearly two-decades. With the success of GRACE-FO, a number of future gravimetric missions are being proposed to ensure the continuous availability of temporal gravity data. Thus, investment in dedicated and integrated geodetic observations will open new vistas in the Himalayan glacier research.
Tracking changes in glacier mass balance, ice volume, permafrost storage, and snow hydrology over the Himalaya is challenging and we can minimize the above-mentioned gaps by capacity building and collaborations. Indian sub-continent hosts a large number of universities and institutions. Development of curriculum in this area of expertise in many of these institutions will increase the chances of minimizing the gaps. Also, capacity building from the secondary education level will prepare the upcoming generation to contribute significantly to this field. Collaboration and easy data sharing between institutions like India Meteorological Department (IMD), Indian Space Research Organization (ISRO), Defense Geoinformatics Research Establishment (Formerly Snow Avalanche and Study Establishment; SASE), Defense Research and Development Organization (DRDO), National Center for Polar and Oceanic Research (NCPOR), and other regional intergovernmental institutions such as the International Center for Integrated Mountain Development (ICIMOD) will also help immensely.
Data Availability Statement
The original contributions presented in the study are included in the article/supplementary material, further inquiries can be directed to the corresponding author.
Author Contributions
BDV, RR, and JB procured the funding for the workshop and organized it. Everyone was involved in discussions, contributed text relevant to their expertise and helped in revising the document. BDV, RR, MFA, and AM collated these contributions and continuously improved the manuscript. AM and SS prepared the Figure 1. BDV and AM prepared the Figures 2, 4. PK provided Figure 3. All authors contributed to the article and approved the submitted version.
Conflict of Interest
The authors declare that the research was conducted in the absence of any commercial or financial relationships that could be construed as a potential conflict of interest.
Publisher's Note
All claims expressed in this article are solely those of the authors and do not necessarily represent those of their affiliated organizations, or those of the publisher, the editors and the reviewers. Any product that may be evaluated in this article, or claim that may be made by its manufacturer, is not guaranteed or endorsed by the publisher.
Acknowledgments
We are grateful to UKIERI and DST for supporting us financially through UKIERI-DST Partnership Development Workshops. RR would like to thank the funding agency Ministry of Earth Science (MoES), Government of India for the project titled Estimating Mass balance of glaciers in the Bhaga Basin, Western Himalaya using GPR and Remote Sensing methods (Grant Ref. No: MoES/PAMC/H&C/107/2018-PC-II dated 27.07.2019).
References
Agarwal, V., Bolch, T., Syed, T. H., Pieczonka, T., Strozzi, T., and Nagaich, R. (2017). Area and mass changes of Siachen glacier (East Karakoram). J. Glaciol. 63, 148–163. doi: 10.1017/jog.2016.127
Ahmad, M., Alam, K., Tariq, S., and Blaschke, T. (2020). Contrasting changes in snow cover and its sensitivity to aerosol optical properties in Hindukush-Karakoram-Himalaya region. Sci. Total Environ. 699, 141058. doi: 10.1016/j.scitotenv.2020.141058
Allen, S. K., Rastner, P., Arora, M., Huggel, C., and Stoffel, M. (2016). Lake outburst and debris flow disaster at Kedarnath, June 2013: hydrometeorological triggering and topographic predisposition. Landslides 13, 1479–1491. doi: 10.1007/s10346-015-0584-3
Angchuk, T., Ramanathan, A., Bahuguna, I. M., Mandal, A., Soheb, M., Singh, V. B., et al. (2021). Annual and seasonal glaciological mass balance of Patsio Glacier, western Himalaya (India) from 2010 to 2017. J. Glaciol. 67, 1–10. doi: 10.1017/jog.2021.60
Archer, D. R., Forsythe, N., Fowler, H. J., and Shah, S. M. (2010). Sustainability of water resources management in the Indus Basin under changing climatic and socio economic conditions. Hydrol. Earth Syst. Sci. 14, 1669–1680. doi: 10.5194/hess-14-1669-2010
Armstrong, R. L., Rittger, K., Brodzik, M. J., Racoviteanu, A., Barrett, A. P., Khalsa, S.-J. S., et al. (2018). Runoff from glacier ice and seasonal snow in High Asia: separating melt water sources in river flow. Reg. Environ. Change 19, 1249–1261. doi: 10.1007/s10113-018-1429-0
Arndt, A., Scherer, D., and Schneider, C. (2021). Atmosphere driven mass-balance sensitivity of Halji Glacier, Himalayas. Atmosphere 12, 426. doi: 10.3390/atmos12040426
Awasthi, S., Kumar, S., Thakur, P. K., Jain, K., and Kumar, A. S. (2021). Snow depth retrieval in North-Western Himalayan region using pursuit-monostatic TanDEM-X datasets applying polarimetric synthetic aperture radar interferometry based inversion modelling. Int. J. Remote Sens. 42, 2872–2897. doi: 10.1080/01431161.2020.1862439
Azam, M. F., Azam, M. F., Kargel, J. S., Shea, J. M., Haritashya, U. K., Srivastava, S., et al. (2021). Glaciohydrology of the Himalaya-Karakoram. Science 373, eabf3668. doi: 10.1126/science.abf3668
Azam, M. F., Ramanathan, A., Wagnon, P., Vincent, C., Linda, A., Berthier, E., et al. (2016). Meteorological conditions, seasonal and annual mass balances of Chhota Shigri Glacier, western Himalaya, India. Ann. Glaciol. 57, 328–338. doi: 10.3189/2016AoG71A570
Azam, M. F., Wagnon, P., Berthier, E., Vincent, C., Fujita, K., and Kargel, J. S. (2018). Review of the status and mass changes of Himalayan-Karakoram glaciers. J. Glaciol. 64, 61–74. doi: 10.1017/jog.2017.86
Azam, M. F., Wagnon, P., Ramanathan, A., Vincent, C., Sharma, P., Arnaud, Y., et al. (2012). From balance to imbalance: a shift in the dynamic behaviour of Chhota Shigri glacier, western Himalaya, India From balance to imbalance: a shift in the dynamic behaviour of Chhota Shigri glacier, western Himalaya, India. J. Glaciol. 58, 315–324. doi: 10.3189/2012JoG11J123
Azam, M. F., Wagnon, P., Vincent, C., Ramanathan, A., Favier, V., Mandal, A., et al. (2014b). Processes governing the mass balance of Chhota Shigri Glacier (western Himalaya, India) assessed by point-scale surface energy balance measurements. Cryosphere 8, 2195–2217. doi: 10.5194/tc-8-2195-2014
Azam, M. F., Wagnon, P., Vincent, C., Ramanathan, A., Linda, A., and Singh, V. B. (2014a). Reconstruction of the annual mass balance of Chhota Shigri glacier, Western Himalaya, India, since 1969. Ann. Glaciol. 55, 69–80. doi: 10.3189/2014AoG66A104
Bahr, D.. (1997). Global distributions of glacier properties: a stochastic scaling paradigm. Water Resour. Res. 33, 1669–1679. doi: 10.1029/97WR00824
Bahr, D. B., Pfeffer, W. T., and Kaser, G. (2015). A review of volume-area scaling of glacier. Rev. Geophys. 53, 95–140. doi: 10.1002/2014RG000470
Bamber, J. L., and Rivera, A. (2007). A review of remote sensing methods for glacier mass balance determination. Glob. Planet. Change 59, 138–148. doi: 10.1016/j.gloplacha.2006.11.031
Banerjee, A.. (2020). Volume-area scaling for debris-covered glaciers. J. Glaciol. 66, 880–886. doi: 10.1017/jog.2020.69
Banerjee, A., and Azam, M. F. (2016). Temperature reconstruction from glacier length fluctuations in the Himalaya. Ann. Glaciol. 57, 189–198. doi: 10.3189/2016AoG71A047
Banerjee, A., Chen, R., Meadows, M. E., Sengupta, D., Pathak, S., Xia, Z., et al. (2021). Tracking 21st century climate dynamics of the Third Pole: an analysis of topo-climate impacts on snow cover in the central Himalaya using google earth engine. Int. J. Appl. Earth Obs. Geoinf. 103, 102490. doi: 10.1016/j.jag.2021.102490
Banerjee, A., Chen, R., Meadows, M. E., Singh, R. B., Mal, S., and Sengupta, D. (2020). An analysis of long-term rainfall trends and variability in the Uttarakhand Himalaya using google earth engine. Remote Sens. 12, 709. doi: 10.3390/rs12040709
Baral, P., Kayastha, R. B., Immerzeel, W. W., Pradhananga, N. S., Bhattarai, B. C., Shahi, S., et al. (2014). Preliminary results of mass-balance observations of Yala Glacier and analysis of temperature and precipitation gradients in Langtang Valley, Nepal. Ann. Glaciol. 55, 9–14. doi: 10.3189/2014AoG66A106
Barsch, D., and Jakob, M. (1998). Mass transport by active rockglaciers in the Khumbu Himalaya. Geomorphology 26, 215–222. doi: 10.1016/S0169-555X(98)00060-9
Berthier, E., Arnaud, Y., Kumar, R., Ahmad, S., Wagnon, P., and Chevallier, P. (2007). Remote sensing estimates of glacier mass balances in the Himachal Pradesh (Western Himalaya, India). Remote Sens. Environ. 108, 327–338. doi: 10.1016/j.rse.2006.11.017
Berthier, E., Vincent, C., Magnússon, E., Gunnlaugsson, Á. Pitte, P., Le Meur, E., et al. (2014). Glacier topography and elevation changes derived from Pléiades sub-meter stereo images. Cryosphere 8, 2275–2291. doi: 10.5194/tc-8-2275-2014
Berthling, I.. (2011). Beyond confusion: rock glaciers as cryo-conditioned landforms. Geomorphology 131, 98–106. doi: 10.1016/j.geomorph.2011.05.002
Bhattacharya, A., Bolch, T., Mukherjee, K., King, O., Menounos, B., Kapitsa, V., et al. (2021). High Mountain Asian glacier response to climate revealed by multi-temporal satellite observations since the 1960s. Nat. Commun. 12, 4133. doi: 10.1038/s41467-021-24180-y
Bhattacharya, A., Bolch, T., Mukherjee, K., Pieczonka, T., Kropáček, J., and Buchroithner, M. F. (2016). Overall recession and mass budget of Gangotri Glacier, Garhwal Himalayas, from 1965 to 2015 using remote sensing data. J. Glaciol. 63, 1115–1133. doi: 10.1017/jog.2016.96
Bhushan, S., Syed, T. H., Kulkarni, A. V., Gantayat, P., and Agarwal, V. (2017). Quantifying changes in the gangotri glacier of central himalaya: evidence for increasing mass loss and decreasing velocity. IEEE J. Sel. Top. Appl. Earth Obs. Remote Sens. 10, 5295–5306. doi: 10.1109/JSTARS.2017.2771215
Bhutiyani, M. R.. (1999). Mass-balance studies on Siachen Glacier in the Nubra valley, Karakoram Himalaya, India. J. Glaciol. 45, 112–118. doi: 10.3189/S0022143000003099
Biskaborn, B. K., Smith, S. L., Noetzli, J., Matthes, H., Vieira, G., Streletskiy, D. A., et al. (2019). Permafrost is warming at a global scale. Nat. Commun. 10, 264. doi: 10.1038/s41467-018-08240-4
Bisset, R. R., Dehecq, A., Goldberg, D. N., Huss, M., Bingham, R. G., and Gourmelen, N. (2020). Reversed surface-mass-balance gradients on himalayan debris-covered glaciers inferred from remote sensing. Remote Sens. 12, 1563. doi: 10.3390/rs12101563
Bohleber, P., Sold, L., Hardy, D. R., Schwikowski, M., Klenk, P., Fischer, A., et al. (2017). Ground-penetrating radar reveals ice thickness and undisturbed englacial layers at Kilimanjaro's Northern ice field. Cryosphere 11, 469–482. doi: 10.5194/tc-11-469-2017
Bolch, T., Buchroithner, M., Pieczonka, T., and Kunert, A. (2008). Planimetric and volumetric glacier changes in the Khumbu Himal, Nepal, since 1962 using Corona, Landsat TM and ASTER data. J. Glaciol. 54, 592–600. doi: 10.3189/002214308786570782
Bolch, T., Kulkarni, A. V., Kaab, A., Huggel, C., Paul, F., Cogley, J. G., et al. (2012). The state and fate of himalayan glaciers. Science 336, 310–314. doi: 10.1126/science.1215828
Bolch, T., Pieczonka, T., and Benn, D. I. (2011). Multi-decadal mass loss of glaciers in the Everest area (Nepal, Himalaya) derived from stereo imagery. Cryosphere 5, 349–358. doi: 10.5194/tc-5-349-2011
Bolch, T., Rohrbach, N., Kutuzov, S., Robson, B. A., and Osmonov, A. (2019b). Occurrence, evolution and ice content of ice-debris complexes in the Ak-Shiirak, Central Tien Shan revealed by geophysical and remotely-sensed investigations. Earth Surf. Process. Landforms 44, 128–143. doi: 10.1002/esp.4487
Bolch, T., Shea, J. M., Liu, S., Azam, F. M., Gao, Y., Gruber, S., et al. (2019a). “Status and Change of the Cryosphere in the Extended Hindu Kush Himalaya Region,” in The Hindu Kush Himalaya Assessment, eds P. Wester, A. Mishra, A. Mukherji, and A. Shrestha (Cham: Springer International Publishing), 209–255. doi: 10.1007/978-3-319-92288-1
Bolch, T., Yao, T., Bhattacharya, A., Hu, Y., King, O., Liu, L., et al. (2022). Earth observation to investigate occurrence, characteristics and changes of glaciers, glacial lakes and rock glaciers in the Poiqu river basin (central Himalaya). Remote Sens. 14, 1927. doi: 10.3390/rs14081927
Bookhagen, B., and Burbank, D. W. (2006). Topography, relief, and TRMM-derived rainfall variations along the Himalaya. Geophys. Res. Lett. 33, 1–5. doi: 10.1029/2006GL026037
Bookhagen, B., and Burbank, D. W. (2010). Toward a complete Himalayan hydrological budget: Spatiotemporal distribution of snowmelt and rainfall and their impact on river discharge. J. Geophys. Res. Earth Surf. 115, 1–25. doi: 10.1029/2009JF001426
Braithwaite, R. J., and Raper, S. C. B. (2009). Estimating equilibrium-line altitude (ELA) from glacier inventory data. Ann. Glaciol. 50, 127–132. doi: 10.3189/172756410790595930
Brinkerhoff, D. J., Aschwanden, A., and Truffer, M. (2016). Bayesian inference of subglacial topography using mass conservation. Front. Earth Sci. 4, 8. doi: 10.3389/feart.2016.00008
Brun, F., Berthier, E., Wagnon, P., Kaäb, A., and Treichler, D. (2017). A spatially resolved estimate of High Mountain Asia glacier mass balances from 2000 to 2016. Nat. Geosci. 11, 543. doi: 10.1038/s41561-018-0171-z
Brun, F., Dumont, M., Wagnon, P., Berthier, E., Azam, M. F., Shea, J. M., et al. (2015). Seasonal changes in surface albedo of Himalayan glaciers from MODIS data and links with the annual mass balance. Cryosphere 9, 341–355. doi: 10.5194/tc-9-341-2015
Budakoti, S., Chauhan, T., Murtugudde, R., Karmakar, S., and Ghosh, S. (2021). Feedback from vegetation to interannual variations of indian summer monsoon rainfall. Water Resour. Res. 57, 1–16. doi: 10.1029/2020WR028750
Cannon, F., Carvalho, L. M. V., Jones, C., and Bookhagen, B. (2015). Multi-annual variations in winter westerly disturbance activity affecting the Himalaya. Clim. Dynam. 44, 441–455. doi: 10.1007/s00382-014-2248-8
Carbone, D., Antoni-Micollier, L., Hammond, G., de Zeeuw - van Dalfsen, E., Rivalta, E., Bonadonna, C., et al. (2020). The NEWTON-g gravity imager: toward new paradigms for terrain gravimetry. Front. Earth Sci. 8, 573396. doi: 10.3389/feart.2020.573396
Carenzo, M., Pellicciotti, F., Mabillard, J., Reid, T., and Brock, B. W. (2016). An enhanced temperature index model for debris-covered glaciers accounting for thickness effect. Adv. Water Resourc. 94, 457–469. doi: 10.1016/j.advwatres.2016.05.001
Chandel, A. K., Khot, L. R., Molaei, B., Peters, R. T., Stöckle, C. O., and Jacoby, P. W. (2021). High-resolution spatiotemporal water use mapping of surface and direct-root-zone drip-irrigated grapevines using uas-based thermal and multispectral remote sensing. Remote Sens. 13, 1–17. doi: 10.3390/rs13050954
Chandel, V. S., and Ghosh, S. (2021). Components of himalayan river flows in a changing climate. Water Resourc. Res. 57, e2020WR027589. doi: 10.1029/2020WR027589
Chandrasekharan, A., Ramsankaran, R., and Pandit, A. (2018). Quantification of annual glacier surface mass balance for the Chhota Shigri Glacier, Western Himalayas, India using an equilibrium-line altitude (ELA) based approach. Int. J. Remote Sens. 39, 9092–9112. doi: 10.1080/01431161.2018.1506182
Chen, J., Famigliett, J. S., Scanlon, B. R., and Rodell, M. (2016). “Groundwater storage changes: present status from GRACE observations,” in Remote Sensing and Water Resources. Space Sciences Series of ISSI, vol 55, eds A. Cazenave, N. Champollion, J. Benveniste, and J. Chen (Cham: Springer). doi: 10.1007/978-3-319-32449-4_9
Chen, J. L., Wilson, C. R., Li, J., and Zhang, Z. (2015). Reducing leakage error in GRACE-observed long-term ice mass change: a case study in West Antarctica. J. Geod. 89, 925–940. doi: 10.1007/s00190-015-0824-2
Clarke, G. K. C., Anslow, F. S., Jarosch, A. H., Radi,ć, V., Menounos, B., Bolch, T., et al. (2013). Ice volume and subglacial topography for western Canadian glaciers from mass balance fields, thinning rates, and a bed stress model. J. Clim. 26, 4282–4303. doi: 10.1175/JCLI-D-12-00513.1
Clarke, G. K. C., Berthier, E., Schoof, C. G., and Jarosch, A. H. (2009). Neural networks applied to estimating subglacial topography and glacier volume. J. Clim. 22, 2146–2160. doi: 10.1175/2008JCLI2572.1
Cogley, J. G., Arendt, A. A., Bauder, A., Braithwaite, R. J., Hock, R., Jansson, P., et al. (2011). Glossary of Glacier Mass Balance and Related Terms, IHP-VII Technical Documents in Hydrology No. 86, IACS Contribution No. 2. Paris: UNESCO.
Cuffey, K., and Paterson, W. S. B. (2010). The Physics of Glaciers. Burlington, MA: Elsevier; Butterworth-Heineman.
Daloz, A. S., Mateling, M., L'ecuyer, T., Kulie, M., Wood, N. B., Durand, M., et al. (2020). How much snow falls in the world's mountains? A first look at mountain snowfall estimates in A-train observations and reanalyses. Cryosphere 14, 3195–3207. doi: 10.5194/tc-14-3195-2020
Dame, J., and Nüsser, M. (2011). Food security in high mountain regions: agricultural production and the impact of food subsidies in Ladakh, Northern India. Food Sec. 3, 179–194. doi: 10.1007/s12571-011-0127-2
Desinayak, N., Prasad, A. K., El-Askary, H., Kafatos, M., and Asrar, G. R. (2022). Snow cover variability and trend over the Hindu Kush Himalayan region using MODIS and SRTM data. Ann. Geophys. 40, 67–82. doi: 10.5194/angeo-40-67-2022
Devaraju, B., and Sneeuw, N. (2016). “On the spatial resolution of homogeneous isotropic filters on the sphere,” in International Association of Geodesy Symposia, eds N. Sneeuw, P. Novák, M. Crespi, and F. Sansò (Cham: Springer International Publishing), 145–153. doi: 10.1007/1345_2015_5
Dimri, A. P., Kumar, D., Choudhary, A., and Maharana, P. (2018). Future changes over the Himalayas: maximum and minimum temperature. Glob. Planet. Change 162, 212–234. doi: 10.1016/j.gloplacha.2018.01.015
Dimri, A. P., Niyogi, D., Barros, A. P., Ridley, J., Mohanty, U. C., Yasunari, T., et al. (2015). Western disturbances: a review. Rev. Geophys. 53, 225–246. doi: 10.1002/2014RG000460
Dimri, A. P., Palazzi, E., and Daloz, A. S. (2022). Elevation dependent precipitation and temperature changes over Indian Himalayan region. Clim. Dyn. 59, 1–21. doi: 10.1007/s00382-021-06113-z
Dimri, A. P., Thayyen, R. J., Kibler, K., Stanton, A., Jain, S. K., Tullos, D., et al. (2016). A review of atmospheric and land surface processes with emphasis on flood generation in the Southern Himalayan rivers. Sci. Total Environ. 556, 98–115. doi: 10.1016/j.scitotenv.2016.02.206
Dobhal, D. P., Gergan, J. T., and Thayyen, R. J. (2008). Mass balance studies of Dokriani Glacier from 1992 to 2000, Garhwal Himalaya, India. Bull. Glaciol. Res. 25, 9–17.
Dobhal, D. P., Gupta, A. K., Manish, M., and Khandelwal, D. D. (2013b). Kedarnath disaster: facts and plausible causes. Curr. Sci. 105, 171–174.
Dobhal, D. P., Mehta, M., and Srivastava, D. (2013a). Influence of debris cover on terminus retreat and mass changes of Chorabari Glacier, Garhwal region, central Himalaya, India. J. Glaciol. 59, 961–971. doi: 10.3189/2013JoG12J180
Dobhal, D. P., Pratap, B., Bhambri, R., and Mehta, M. (2021). Mass balance and morphological changes of Dokriani Glacier (1992–2013), Garhwal Himalaya, India. Quat. Sci. Adv. 4, 100033. doi: 10.1016/j.qsa.2021.100033
Döll, P., Schmied, H. M., Schuh, C., Portmann, F. T., and Eicker, A. (2014). Water resources research. Water Resour. Res. Res. 50, 5375–5377. doi: 10.1002/2014WR015595
Dumont, M., Gardelle, J., Sirguey, P., Guillot, A., Six, D., Rabatel, A., et al. (2012). Linking glacier annual mass balance and glacier albedo retrieved from MODIS data. Cryosphere 6, 1527–1539. doi: 10.5194/tc-6-1527-2012
Dunse, T., Schuler, T. V., Hagen, J. O., and Reijmer, C. H. (2012). Seasonal speed-up of two outlet glaciers of Austfonna, Svalbard, inferred from continuous GPS measurements. Cryosphere 6, 453–466. doi: 10.5194/tc-6-453-2012
Durand, M., Rivera, A., Geremia-Nievinski, F., Lenzano, M. G., Monico, J. G., Paredes, P., et al. (2019). GPS reflectometry study detecting snow height changes in the Southern Patagonia Icefield. Cold Reg. Sci. Technol. 166, 102840. doi: 10.1016/j.coldregions.2019.102840
Eckstein, D., Künzel, V., and Schäfer, L. (2017). Global climate Risk Index 2018. Bonn: Germanwatch.
Eicker, A., Schumacher, M., Kusche, J., Döll, P., and Schmied, H. M. (2014). Calibration/data assimilation approach for integrating GRACE data into the WaterGAP global hydrology model (WGHM) using an ensemble kalman filter: first results. Surv. Geophys. 35, 1285–1309. doi: 10.1007/s10712-014-9309-8
Elliott, J., and Freymueller, J. T. (2020). A block model of present-day kinematics of Alaska and western Canada. J. Geophys. Res. Solid Earth 125, e2019JB018378. doi: 10.1029/2019JB018378
Farinotti, D., Brinkerhoff, D. J., Fürst, J. J., Gantayat, P., Gillet-Chaulet, F., Huss, M., et al. (2021). Results from the ice thickness models intercomparison eXperiment phase 2 (ITMIX2). Front. Earth Sci. 8, 571923. doi: 10.3389/feart.2020.571923
Farinotti, D., Huss, M., Bauder, A., Funk, M., and Truffer, M. (2009). A method to estimate ice volume and ice thickness distribution of alpine glaciers. J. Glaciol. 55, 422–430. doi: 10.3189/002214309788816759
Farinotti, D., Huss, M., Fürst, J. J., Landmann, J., Machguth, H., Maussion, F., et al. (2019). A consensus estimate for the ice thickness distribution of all glaciers on earth. Nat. Geosci. 12, 168–173. doi: 10.1038/s41561-019-0300-3
Favier, V., Wagnon, P., Chazarin, J., Maisincho, L., and Coudrain, A. (2004). One-year measurements of surface heat budget on the ablation zone of Antizana Glacier 15, Ecuadorian Andes. J. Geophys. Res. 109, 1–15. doi: 10.1029/2003JD004359
Frey, H., Machguth, H., Huss, M., Huggel, C., Bajracharya, S. R., Bolch, T., et al. (2014). Estimating the volume of glaciers in the Himalayan – Karakoram region using different methods. Cryosphere 8, 2313–2333. doi: 10.5194/tc-8-2313-2014
Fujita, K., and Ageta, Y. (2001). Effect of summer accumulation on glacier mass balance on the Tibetan Plateau revealed by mass-balance model. J. Glaciol. 46, 244–252. doi: 10.3189/172756500781832945
Furian, W., Loibl, D., and Schneider, C. (2021). Future glacial lakes in High Mountain Asia: an inventory and assessment of hazard potential from surrounding slopes. J. Glaciol. 65, 453–467. doi: 10.1017/jog.2021.18
Fürst, J., Gillet-Chaulet, F., Benham, T. J., Dowdeswell, J. A., Grabiec, M., Navarro, F., et al. (2017). Application of a two-step approach for mapping ice thickness to various glacier types on Svalbard. Cryosphere 11, 2003–2032. doi: 10.5194/tc-11-2003-2017
Gaddam, V. K., Kulkarni, A. V., and Gupta, A. K. (2020). Assessment of the Baspa basin glaciers mass budget using different remote sensing methods and modeling techniques. Geocarto Int. 35, 296–316. doi: 10.1080/10106049.2018.1516247
Gantayat, P., Kulkarni, A. V., and Srinivasan, J. (2014). Estimation of ice thickness using surface velocities and slope: case study at Gangotri Glacier, India. J. Glaciol. 60, 277–282. doi: 10.3189/2014JoG13J078
Gardelle, J., Berthier, E., and Arnaud, Y. (2012). Impact of resolution and radar penetration on glacier elevation changes computed from DEM differencing. J. Glaciol. 58, 419–422. doi: 10.3189/2012JoG11J175
Gardelle, J., Berthier, E., Arnaud, Y., and Kaab, A. (2013). Region-wide glacier mass balances over the Pamir-Karakoram-Himalaya during 1999 – 2011. Cryosphere 7, 1263–1286. doi: 10.5194/tc-7-1263-2013
Garg, S., Shukla, A., Garg, P. K., Yousuf, B., Shukla, U. K., and Lotus, S. (2021). Revisiting the 24 year (1994-2018) record of glacier mass budget in the Suru sub-basin, western Himalaya: overall response and controlling factors. Sci. Total Environ. 800, 149533. doi: 10.1016/j.scitotenv.2021.149533
Gautam, C. K., and Mukherjee, B. K. (1992). Synthesis of Glaciological Studies on Tipra Bank Glacier, Bhyundar Ganga basin, District Chamoli, Uttar Pradesh (F.S. 1980–1988). Lucknow: Geological Survey of India
Geological Survey of India (1991). Annual General Report, Part 8, Vol. 124. Lucknow: Geological Survey of India.
Geological Survey of India (1992). Annual General Report. Part 8. Vol. 125. Lucknow: Geological Survey of India.
Geological Survey of India (2001). Glaciology of Indian Himalaya. Special Publication no 63. Lucknow: Geological Survey of India.
Geological Survey of India Report (2017). Long-Term Monitoring Of Mass Balance Of Hamtah Glacier, Lahaul & Spiti District, H.P. On Expedition Basis, eds K. Krishna, Z. Majeed, and S. Pramanik (GL/NR/HQ/2016/060). Lucknow: Geological Survey of India Report.
Ghimire, S., Choudhary, A., and Dimri, A. P. (2018). Assessment of the performance of CORDEX-South Asia experiments for monsoonal precipitation over the Himalayan region during present climate: part I. Clim. Dyn. 50, 2311–2334. doi: 10.1007/s00382-015-2747-2
Ghobadi-Far, K., Han, S. C., Allgeyer, S., Tregoning, P., Sauber, J., Behzadpour, S., et al. (2020). GRACE gravitational measurements of tsunamis after the 2004, 2010, and 2011 great earthquakes. J. Geod. 94, 65. doi: 10.1007/s00190-020-01395-3
Glen, J. W.. (1955). The creep of polycrystalline ice. Proc. R. Soc. Lon. Ser. A 228, 519–538. doi: 10.1098/rspa.1955.0066
Grinsted, A.. (2013). An estimate of global glacier volume. Cryosphere 7, 141–151. doi: 10.5194/tc-7-141-2013
Gruber, S.. (2012). Derivation and analysis of a high-resolution estimate of global permafrost zonation. Cryosphere 6, 221–233. doi: 10.5194/tc-6-221-2012
Gruber, S., Fleiner, R., Guegan, E., Panday, P., Schmid, M. O., Stumm, D., et al. (2017). Inferring permafrost and permafrost thaw in the mountains of the Hindu Kush Himalaya region. Cryosphere 11, 81–99. doi: 10.5194/tc-11-81-2017
Gupta, A., Kayastha, R. B., Ramanathan, AL., and Dimri, A. P. (2019). Comparison of hydrological regime of glacierized Marshyangdi and Tamor river basins of Nepal. Environ. Earth Sci. 78, 427. doi: 10.1007/s12665-019-8443-5
Gurung, D. R., Giriraj, A., Aung, K. S., Shrestha, B., and Kulkarni, A. V. (2011). Snow-Cover Mapping and Monitoring in the Hindu Kush-Himalayas. Kathmandu. Available online at: http://lib.riskreductionafrica.org/bitstream/handle/123456789/1125/snow-cover mapping and monitoring in the hindu.pdf?sequence=1
Gurung, D. R., Maharjan, S. B., Shrestha, A. B., Shrestha, M. S., Bajracharya, S. R., and Murthy, M. S. R. (2017). Climate and topographic controls on snow cover dynamics in the Hindu Kush Himalaya. Int. J. Climatol. 37, 3873–3882. doi: 10.1002/joc.4961
Gurung, S., Bhattrai, B. C., Kayastha, R. B., Stumm, D., Joshi, S., and Mool, P. K. (2016). Study of Annual Mass Balance (2011-2013) of Rikha Samba Glacier, Hidden Valley, Mustang, Nepal. AGU Fall Meeting Abstracts 11. Available online at: http://adsabs.harvard.edu/abs/2016AGUFM.C11C0799G (accessed July 18, 2019).
Gusain, A., Ghosh, S., and Karmakar, S. (2020). Added value of CMIP6 over CMIP5 models in simulating Indian summer monsoon rainfall. Atmos. Res. 232, 104680. doi: 10.1016/j.atmosres.2019.104680
Gusain, H. S., Mishra, V. D., Arora, M. K., Mamgain, S., and Singh, D. K. (2016). Operational algorithm for generation of snow depth maps from discrete data in Indian Western Himalaya. Cold Reg. Sci. Technol. 126, 22–29. doi: 10.1016/j.coldregions.2016.02.012
Haeberli, W., Hallet, B., Arenson, L., Elconin, R., Humlum, O., Kääb, A., et al. (2006). Permafrost creep and rock glacier dynamics. Permafrost Periglac. Proc. 17, 189–214. doi: 10.1002/ppp.561
Haeberli, W., and Hoelzle, M. (1995). Application of inventory data for estimating characteristics of and regional climate-change effects on mountain glaciers: a pilot study with the European Alps. Ann. Glaciol. 21, 206–212. doi: 10.3189/S0260305500015834
Haq, M. A., Azam, M. F., and Vincent, C. (2021). Efficiency of artificial neural networks for glacier ice-thickness estimation: a case study in western Himalaya, India. J. Glaciol. 67, 671–684. doi: 10.1017/jog.2021.19
Harrison, S., Jones, D., Anderson, K., Shannon, S., and Betts, R. A. (2021). Is ice in the Himalayas more resilient to climate change than we thought? Geogr. Ann. Ser. A Phys. Geogr. 103, 1–7. doi: 10.1080/04353676.2021.1888202
Henkel, P., Koch, F., Appel, F., Bach, H., Prasch, M., Schmid, L., et al. (2018). Snow water equivalent of dry snow derived from GNSS Carrier Phases. IEEE Trans. Geosci. Remote Sens. 56, 3561–3572. doi: 10.1109/TGRS.2018.2802494
Hersbach, H., Bell, B., Berrisford, P., Hirahara, S., Horányi, A., Muñoz-Sabater, J., et al. (2020). The ERA5 global reanalysis. Q. J. R. Meteorol. Soc. 146, 1999–2049. doi: 10.1002/qj.3803
Hewitt, K.. (2014). “Glaciers of the Karakoram Himalaya,” in Encyclopedia of Snow, Ice and Glaciers (Dordrecht: Springer), 429–436. doi: 10.1007/978-94-007-6311-1
Hock, R.. (2003). Temperature index melt modelling in mountain areas. J. Hydrol. 282, 104–115. doi: 10.1016/S0022-1694(03)00257-9
Hock, R., Rasul, G., Adler, C., Cáceres, B., Gruber, S., Hirabayashi, Y., et al. (2019). “High Mountain areas,” in IPCC Special Report on the Ocean and Cryosphere in a Changing Climate, eds H. O. Pörtner, D. C. Roberts, V. Masson-Delmotte, P. Zhai, M. Tignor, E. Poloczanska, K. Mintenbeck, A. Alegría, M. Nicolai, A. Okem, J. Petzold, B. Rama, and N. M. Weyer (Cambridge; New York, NY: Cambridge University Press), 131–202. doi: 10.1017/9781009157964.004
Hofmann-Wellenhof, B., Lichtenegger, H., and Wasle, E. (2007). GNSS–Global Navigation Satellite Systems: GPS, GLONASS, Galileo, and More. Vienna: Springer Science & Business Media.
Houborg, R., Rodell, M., Li, B., Reichle, R., and Zaitchik, B. F. (2012). Drought indicators based on model-assimilated gravity recovery and climate experiment (GRACE) terrestrial water storage observations. Water Resour. Res. 48. doi: 10.1029/2011WR011291
Huggel, C., Carey, M., Emmer, A., Frey, H., Walker-Crawford, N., and Wallimann-Helmer, I. (2020). Anthropogenic climate change and glacier lake outburst flood risk: local and global drivers and responsibilities for the case of lake Palcacocha, Peru. Nat. Hazards Earth Syst. Sci. 20, 2175–2193. doi: 10.5194/nhess-20-2175-2020
Hugonnet, R., McNabb, R., Berthier, E., Menounos, B., Nuth, C., Girod, L., et al. (2021). Accelerated global glacier mass loss in the early twenty-first century. Nature 592, 726–731. doi: 10.1038/s41586-021-03436-z
Huss, M.. (2013). Density assumptions for converting geodetic glacier volume change to mass change. Cryosphere 7, 877–887, doi: 10.5194/tc-7-877-2013
Huss, M., and Farinotti, D. (2012). Distributed ice thickness and volume of all glaciers around the globe. J. Geophys. Res. Earth Surf. 117, 1–10. doi: 10.1029/2012JF002523
Huss, M., and Hock, R. (2018). Global-scale hydrological response to future glacier mass loss. Nat. Clim. Chang. 8, 135–140. doi: 10.1038/s41558-017-0049-x
ICIMOD (2021). Major River Basins in the Hindu Kush Himalaya (HKH) Region [Data set]. Kathmandu: ICIMOD.
Immerzeel, W. W., Beek, L. P. H. V., and Bierkens, M. F. P. (2010). Climate change will affect the asian water towers. Science 328, 1382–1385. doi: 10.1126/science.1183188
Immerzeel, W. W., Lutz, A. F., Andrade, M., Bahl, A., Biemans, H., Bolch, T., et al. (2020). Importance and vulnerability of the world's water towers. Nature 577, 364–369. doi: 10.1038/s41586-019-1822-y
Immerzeel, W. W., van Beek, L. P. H., Konz, M., Shrestha, A. B., and Bierkens, M. F. P. (2012). Hydrological response to climate change in a glacierized catchment in the Himalayas. Clim. Change 110, 721–736. doi: 10.1007/s10584-011-0143-4
Immerzeel, W. W., Wanders, N., Lutz, A. F., Shea, J. M., and Bierkens, M. F. P. (2015). Reconciling high-altitude precipitation in the upper Indus basin with glacier mass balances and runoff, Hydrol. Earth Syst. Sci. 19, 4673–4687. doi: 10.5194/hess-19-4673-2015
Jackson, T. J., Schugge, T. J., Nicks, A. D., Coleman, G. A., and Engman, E. T. (1981). Soil moisture updating and microwave remote sensing for hydrological simulation. Hydrol. Sci. Bull. 26, 305–319. doi: 10.1080/02626668109490889
Jacob, T., Wahr, J., Pfeffer, W. T., and Swenson, S. (2012). Recent contributions of glaciers and ice caps to sea level rise. Nature 482, 514–518. doi: 10.1038/nature10847
Jacobsen, F. M., and Theakstone, W. H. (1997). Monitoring glacier changes using a global positioning system in differential mode. Ann. Glaciol. 24, 314–319. doi: 10.3189/S0260305500012374
Jakob, L., Gourmelen, N., Ewart, M., and Plummer, S. (2021). Spatially and temporally resolved ice loss in High Mountain Asia and the Gulf of Alaska observed by CryoSat-2 swath altimetry between 2010 and 2019. Cryosphere 15, 1845–1862. doi: 10.5194/tc-15-1845-2021
James, W. H., and Carrivick, J. L. (2016). Automated modelling of spatially-distributed glacier ice thickness and volume. Comput. Geosci. 92, 90–103. doi: 10.1016/j.cageo.2016.04.007
Janke, J. R., and Bolch, T. (2022). “4.06 - Rock Glaciers,” in Treatise on Geomorphology, 2nd Edn, eds J. Jack, and F. Shroder (Oxford: Academic Press), 75–118. doi: 10.1016/B978-0-12-818234-5.00187-5
Javed, A., Kumar, P., Hodges, K. I., Sein, D. V., Dubey, A. K., and Tiwari, G. (2022). Does the recent revival of Western Disturbances govern the Karakoram Anomaly? J. Clim. 1–57. doi: 10.1175/JCLI-D-21-0129.1
Jeelani, G., Shah, R. A., Jacob, N., and Deshpande, R. D. (2017). Estimation of snow and glacier melt contribution to Liddar stream in a mountainous catchment, western Himalaya: an isotopic approach. Isotopes Environ. Health Stud. 53, 18–35. doi: 10.1080/10256016.2016.1186671
Jones, D. B., Harrison, S., and Anderson, K. (2019b). Mountain glacier-to-rock glacier transition. Glob. Planet. Change 181, 102999. doi: 10.1016/j.gloplacha.2019.102999
Jones, D. B., Harrison, S., Anderson, K., Shannon, S., and Betts, R. A. (2021). Rock glaciers represent hidden water stores in the Himalaya. Sci. Total Environ. 793, 145368. doi: 10.1016/j.scitotenv.2021.145368
Jones, D. B., Harrison, S., Anderson, K., and Whalley, W. B. (2019a). Rock glaciers and mountain hydrology: a review. Earth Sci. Rev. 193, 66–90. doi: 10.1016/j.earscirev.2019.04.001
Kääb, A., Berthier, E., Nuth, C., Gardelle, J., and Arnaud, Y. (2012). Contrasting patterns of early twenty-first-century glacier mass change in the Himalayas. Nature 488, 495–498. doi: 10.1038/nature11324
Kääb, A., Treichler, D., Nuth, C., and Berthier, E. (2015). Brief Communication: Contending estimates of 2003-2008 glacier mass balance over the Pamir-Karakoram-Himalaya. Cryosphere 9, 557–564. doi: 10.5194/tc-9-557-2015
Kanda, N., Negi, H. S., Rishi, M. S., and Kumar, A. (2020). Performance of various gridded temperature and precipitation datasets over northwest Himalayan region. Environ. Res. Commun. 2, eab9991. doi: 10.1088/2515-7620/ab9991
Kargel, J. S., Leonard, G. J., Shugar, D. H., Haritashya, U. K., Bevington, A., Fielding, E. J., et al. (2016). Geomorphic and geologic controls of geohazards induced by Nepal's 2015 Gorkha earthquake. Science 351, aac8353. doi: 10.1126/science.aac8353
Karim, A., and Veizer, J. (2002). Water balance of the Indus River Basin and moisture source in the Karakoram and western Himalayas: implications from hydrogen and oxygen isotopes in river water. J. Geophys. Res. 107, 1–12. doi: 10.1029/2000JD000253
Karimi, P., and Bastiaanssen, W. G. M. (2015). Spatial evapotranspiration, rainfall and land use data in water accounting - Part 1: review of the accuracy of the remote sensing data. Hydrol. Earth Syst. Sci. 19, 507–532. doi: 10.5194/hess-19-507-2015
Kaser, G., Fountain, A., and Jansson, P. (2003). A Manual for Monitoring the Mass Balance of Mountain Glaciers. IHP-VI Technical Documents in Hydrology, No. 59. Paris: UNESCO.
Kaser, G., Grosshauser, M., and Marzeion, B. (2010). Contribution potential of glaciers to water availability in different climate regimes. Proc. Natl. Acad. Sci. U.S.A. 107, 20223–20227. doi: 10.1073/pnas.1008162107
Khadka, M., Kayastha, R. B., and Kayastha, R. (2020). Future projection of cryospheric and hydrologic regimes in Koshi River basin, Central Himalaya, using coupled glacier dynamics and glacio-hydrological models. J. Glaciol. 66, 831–845. doi: 10.1017/jog.2020.51
Khanal, S., Lutz, A. f, Kraaijenbrink, P. D. A., van den Hurk, B., Yao, T., et al. (2021). Variable 21st century climate change response for rivers in high mountain asia at seasonal to decadal time scales. Water Resourc. Res. 57, e2020WR029266. doi: 10.1029/2020WR029266
King, O., Bhattacharya, A., Bhambri, R., and Bolch, T. (2019). Glacial lakes exacerbate Himalayan glacier mass loss. Sci Rep 9, 18145. doi: 10.1038/s41598-019-53733-x
King, O., Bhattacharya, A., Ghuffar, S., Tait, A., Guilford, S., Elmore, A. C., et al. (2020). Six decades of glacier mass changes around MT. everest are revealed by historical and contemporary images. One Earth 3, 608–620. doi: 10.1016/j.oneear.2020.10.019
King, O., Quincey, D. J., Carrivick, J. L., and Rowan, A. V. (2017). Spatial variability in mass loss of glaciers in the Everest region, central Himalayas, between 2000 and 2015. Cryosphere 11, 407–426. doi: 10.5194/tc-11-407-2017
Koch, F., Prasch, M., Schmid, L., Schweizer, J., and Mauser, W. (2014). Measuring snow liquid water content with low-cost gps receivers. Sensors 14, 20975–20999. doi: 10.3390/s141120975
Kotlarski, S.. (2007). A Subgrid Glacier Parameterisation for Use in Regional Climate Modelling. (Doctoral Dissertation) University of Hamburg, Hamburg. doi: 10.17617/2.994357
Kotlarski, S., Jacob, D., Podzun, R., and Paul, F. (2010). Representing glaciers in a regional climate model. Clim. Dyn. 34, 27–46. doi: 10.1007/s00382-009-0685-6
Koul, M. N., and Ganjoo, R. K. (2010). Impact of inter- and intra-annual variation in weather parameters on mass balance and equilibrium line altitude of Naradu Glacier (Himachal Pradesh), NW Himalaya, India. Clim. Change 99, 119–139. doi: 10.1007/s10584-009-9660-9
Koulali, A., Whitehouse, P. L., Clarke, P. J., van den Broeke, M. R., Nield, G. A., King, M. A., et al. (2022). GPS-observed elastic deformation due to surface mass balance variability in the Southern Antarctic Peninsula. Geophys. Res. Lett. 49, e2021GL097109. doi: 10.1029/2021GL097109
Kraaijenbrink, P. D. A., Bierkens, M. F. P., Lutz, A. F., and Immerzeel, W. W. (2017). Impact of a global temperature rise of 1.5 degrees celsius on Asia's glaciers. Nature 549, 257–260. doi: 10.1038/nature23878
Krautblatter, M., Funk, D., and Günzel, F. K. (2013). Why permafrost rocks become unstable: a rock-ice-mechanical model in time and space. Earth Surf. Process. Landf. 38, 876–887. doi: 10.1002/esp.3374
Kulkarni, A., Patwardhan, S., Kumar, K. K., Ashok, K., and Krishnan, R. (2013). Projected climate change in the Hindu Kush – Himalayan region by using the high-resolution regional climate model PRECIS. Mt. Res. Dev. 33, 142–151. doi: 10.1659/MRD-JOURNAL-D-11-00131.1
Kulkarni, A. V.. (1992). Mass balance of Himalayan glaciers using AAR and ELA methods. J. Glaciol. 38, 101–104. doi: 10.1017/S0022143000009631
Kulkarni, A. V., Rathore, B. P., and Alex, S. (2004). Monitoring of glacial mass balance in the Baspa basin using accumulation area ratio method. Curr. Sci. 86, 185–190.
Kulkarni, A. V., Rathore, B. P., Singh, S. K., and Ajai. (2010). Distribution of seasonal snow cover in central and western Himalaya. Ann. Glaciol. 51, 123–128. doi: 10.3189/172756410791386445
Kulkarni, A. V., Shirsat, T. S., Kulkarni, A., Negi, H. S., Bahuguna, I. M., and Thamban, M. (2021). State of Himalayan cryosphere and implications for water security. Water Secur. 14, 100101. doi: 10.1016/j.wasec.2021.100101
Kumar, P., Kotlarski, S., Moseley, C., Sieck, K., Frey, H., Stoffel, M., et al. (2015). Response of Karakoram-Himalayan glaciers to climate variability and climatic change: a regional climate model assessment. Geophys. Res. Lett. 42, 1818–1825. doi: 10.1002/2015GL063392
Kumar, P., Mishra, A. K., Dubey, A. K., Javed, A., Saharwardi, M.d,. S., et al. (2022). Regional earth system modelling framework for CORDEX-SA: an integrated model assessment for Indian summer monsoon rainfall. Clim Dyn. doi: 10.1007/s00382-022-06217-0
Kumar, P., Saharwardi, M. S., Banerjee, A., Azam, M. F., Dubey, A. K., and Murtugudde, R. (2019). Snowfall variability dictates glacier mass balance variability in Himalaya-Karakoram. Sci. Rep. 9, 18192. doi: 10.1038/s41598-019-54553-9
Kumar, R., Singh, S., Singh, A., Kumar, R., Singh, S., and Randhawa, S. S. (2021). Surface mass balance analysis at Naradu Glacier, Western Himalaya, India. Sci. Rep. 11, 12710. doi: 10.1038/s41598-021-91348-3
Kumar, V., and Venkataraman, G. (2011). SAR interferometric coherence analysis for snow cover mapping in the western Himalayan region. Int. J. Digit. Earth 4, 78–90. doi: 10.1080/17538940903521591
Kwon, Y., Forman, B. A., Ahmad, J. A., Kumar, S., and Yoon, Y. (2019). Exploring the utility of machine learning-based passive microwave brightness temperature data assimilation over terrestrial snow in high mountain Asia. Remote Sens. 11, 2265. doi: 10.3390/rs11192265
Laha, S., Kumari, R., Singh, S., Mishra, A., Sharma, T., Banerjee, A., et al. (2017). Evaluating the contribution of avalanching to the mass balance of Himalayan glaciers. Ann. Glaciol. 58, 110–118. doi: 10.1017/aog.2017.27
Larson, K. M.. (2016). GPS interferometric reflectometry: applications to surface soil moisture, snow depth, and vegetation water content in the western United States. Wiley Interdiscip. Rev. Water 3, 775–787. doi: 10.1002/wat2.1167
Lau, W. K. M., Kim, M. K., Kim, K. M., and Lee, W. S. (2010). Enhanced surface warming and accelerated snow melt in the Himalayas and Tibetan Plateau induced by absorbing aerosols. Environ. Res. Lett. 5, 025204. doi: 10.1088/1748-9326/5/2/025204
Laumann, T., and Nesje, A. (2017). Volume–area scaling parameterisation of Norwegian ice caps: a comparison of different approaches. Holocene 27, 164–171. doi: 10.1177/0959683616652712
Lehmann, F., Vishwakarma, B. D., and Bamber, J. (2022). How well are we able to close the water budget at the global scale? Hydrol. Earth Syst. Sci. 26, 35–54. doi: 10.5194/hess-26-35-2022
Linsbauer, A., Frey, H., Haeberli, W., Machguth, H., Azam, M. F., and Allen, S. (2016). Modelling glacier-bed overdeepenings and possible future lakes for the glaciers in the Himalaya-Karakoram region. Ann. Glaciol. 57, 119–130. doi: 10.3189/2016AoG71A627
Linsbauer, A., Paul, F., Hoelzle, M., Frey, H., and Haeberli, W (2009). “The Swiss Alps without glaciers – a GIS-based modelling approach for reconstruction of glacier beds,” in Proceedings of Geomorphometry 2009, ed R. S. Purves et al. (Zurich: Department of Geography, University of Zurich), 243–247. doi: 10.5167/uzh-27834
Litt, M., Shea, J., Wagnon, P., Steiner, J., Koch, I., Stigter, E., et al. (2019). Glacier ablation and temperature indexed melt models in the Nepalese Himalaya. Sci. Rep. 9, 5264. doi: 10.1038/s41598-019-41657-5
Liu, S., Xie, Z., Song, G., Ma, L., and Ageta, Y. (1996). Mass balance of Kangwure (flat-top) glacier on the north side of Mt. Xixiabangma, China. Bull. Gla. Res. 14, 37–43.
Liu, Y., Zhang, X., and Yu, H. (2014). Snow depth estimation using a lookup table method based on MEMLS. Gaojishu Tongxin Chin. High Technol. Lett. 24.
Long, D., Chen, X., Scanlon, B. R., Wada, Y., Hong, Y., Singh, V. P., et al. (2016). Have GRACE satellites overestimated groundwater depletion in the Northwest India Aquifer? Sci. Rep. 6, 24398. doi: 10.1038/srep24398
Long, D., Shen, Y., Sun, A., Hong, Y., Longuevergne, L., Yang, Y., et al. (2014). Drought and flood monitoring for a large karst plateau in Southwest China using extended GRACE data. Remote Sens. Environ. 155, 145–160. doi: 10.1016/j.rse.2014.08.006
Longuevergne, L., Scanlon, B. R., and Wilson, C. R. (2010). GRACE hydrological estimates for small basins: evaluating processing approaches on the High Plains aquifer, USA. Water Resour. Res. 46, 1–15. doi: 10.1029/2009WR008564
Lorenz, C., Tourian, M. J., Devaraju, B., Sneeuw, N., and Kunstmann, H. (2015). Basin-scale runoff prediction: an ensemble kalman filter framework based on global hydrometeorological data sets. Water Resour. Res. 51, 8450–8475. doi: 10.1002/2014WR016794
Lutz, A. F., Immerzeel, W. W., Kraaijenbrink, P. D. A., Shrestha, A. B., and Bierkens, M. F. P. (2016). Climate change impacts on the upper indus hydrology: Sources, shifts and extremes. PLoS ONE 11, e0165630. doi: 10.1371/journal.pone.0165630
Lutz, A. F., Immerzeel, W. W., Shrestha, A. B., and Bierkens, M. F. P. (2014). Consistent increase in high Asia's runoff due to increasing glacier melt and precipitation. Nat. Clim. Chang. 4, 587–592. doi: 10.1038/nclimate2237
Maharana, P., and Dimri, A. P. (2016). Study of intraseasonal variability of Indian summer monsoon using a regional climate model. Clim. Dyn. 46, 1043–1064. doi: 10.1007/s00382-015-2631-0
Maharana, P., Kumar, D., and Dimri, A. P. (2019). Assessment of coupled regional climate model (RegCM4.6–CLM4.5) for Indian summer monsoon. Clim. Dyn. 53, 6543–6558. doi: 10.1007/s00382-019-04947-2
Maheshwari, B.. (2020). “Participatory groundwater management: making the invisible resource visible and giving ownership of its sustainability to villagers,” in Success stories in agricultural water management research for development, in Christen, EW 2020 (Canberra, ACT: Australian Centre for International Agricultural Research), 67 pp.
Maheshwari, B., Varua, M., Ward, J., Packham, R., Chinnasamy, P., Dashora, Y., et al. (2014). The role of transdisciplinary approach and community participation in village scale groundwater management: insights from Gujarat and Rajasthan, India. Water 6, 3386–3408. doi: 10.3390/w6113386
Majeed, U., Rashid, I., Sattar, A., Allen, S., Stoffel, M., Nüsser, M., et al. (2021). Recession of Gya Glacier and the 2014 glacial lake outburst flood in the Trans-Himalayan region of Ladakh, India. Sci. Total Environ. 756, 144008. doi: 10.1016/j.scitotenv.2020.144008
Mandal, A., Ramanathan, A., Azam, M. F., Angchuk, T., Soheb, M., Kumar, N., et al. (2020). Understanding the interrelationships among mass balance, meteorology, discharge and surface velocity on Chhota Shigri Glacier over 2002-2019 using in situ measurements. J. Glaciol. 66, 727–741. doi: 10.1017/jog.2020.42
Marzeion, B., Jarosch, A. H., and Hofer, M. (2012). Past and future sea-level change from the surface mass balance of glaciers. Cryosphere 6, 1295–1322. doi: 10.5194/tc-6-1295-2012
Maurer, J. M., Schaefer, J. M., Rupper, S., and Corley, A. (2019). Acceleration of ice loss across the Himalayas over the past 40 years. Sci. Adv. 5, eaav7266. doi: 10.1126/sciadv.aav7266
Maussion, F., Butenko, A., Champollion, N., Dusch, M., Eis, J., Fourteau, K., et al. (2019). The open global glacier model (OGGM) v1.1. Geosci. Model Dev. 12, 909–931. doi: 10.5194/gmd-12-909-2019
Mey, J., Scherer, D., Zeilinger, G., and Strecker, M. R. (2015). Estimating the fill thickness and bedrock topography in intermontane valleys using artificial neural networks. J. Geophys. Res. Earth Surf. 120, 1301–1320. doi: 10.1002/2014JF003270
Millan, R., Mouginot, J., Rabatel, A., and Morlighem, M. (2022). Ice velocity and thickness of the world's glaciers. Nat. Geosci. 15, 124–129. doi: 10.1038/s41561-021-00885-z
Mir, R. A., Jain, S. K., Saraf, A. K., and Goswami, A. (2014). Detection of changes in glacier mass balance using satellite and meteorological data in tirungkhad basin located in Western himalaya. J. Indian Soc. Remote Sens. 42, 91–105. doi: 10.1007/s12524-013-0303-2
Miro, M. E., and Famiglietti, J. S. (2018). Downscaling GRACE remote sensing datasets to high-resolution groundwater storage change maps of California's Central Valley. Remote Sens. 10, 143. doi: 10.3390/rs10010143
Mishra, A., Negi, B. D. S., Banerjee, A., Nainwal, H. C., and Shankar, R. (2018). Estimation of ice thickness of the satopanth glacier, central himalaya using ground penetrating radar. Curr. Sci. 114, 785. doi: 10.18520/cs/v114/i04/785-791
Molden, D., Sharma, E., Shrestha, A. B., Chettri, N., Pradhan, N. S., and Kotru, R. (2017). Advancing regional and transboundary cooperation in the conflict-prone hindu Kush-Himalaya. Mt. Res. Dev. 37, 502–508. doi: 10.1659/MRD-JOURNAL-D-17-00108.1
Möller, M., and Schneider, C. (2010). Calibration of glacier volume – area relations from surface extent fluctuations and application to future glacier change. J. Glaciol. 56, 33–40. doi: 10.3189/002214310791190866
Monnier, S., and Kinnard, C. (2017). Pluri-decadal (1955-2014) evolution of glacier-rock glacier transitional landforms in the central Andes of Chile (30-33deg;S). Earth Surf. Dyn. 5, 493–509. doi: 10.5194/esurf-5-493-2017
Morlighem, M., Rignot, E., Seroussi, H., Larour, E., Ben Dhia, H., and Aubry, D. (2011). A mass conservation approach for mapping glacier ice thickness. Geophys. Res. Lett. 38, 1–6. doi: 10.1029/2011GL048659
Mukherjee, K., Bhattacharya, A., Pieczonka, T., Ghosh, S., and Bolch, T. (2018). Glacier mass budget and climate reanalysis data indicate a climatic shift around 2000 in Lahaul-Spiti, western Himalaya. Clim. Change 148, 219–233. doi: 10.1007/s10584-018-2185-3
Neckel, N., Kropáček, J., Bolch, T., and Hochschild, V. (2014). Glacier mass changes on the Tibetan Plateau 2003-2009 derived from ICESat laser altimetry measurements. Environ. Res. Lett. 9, 014009. doi: 10.1088/1748-9326/9/1/014009
Nengker, T., Choudhary, A., and Dimri, A. P. (2018). Assessment of the Performance of CORDEX-SA Experiments in Simulating Seasonal Mean Temperature Over the Himalayan Region for the Present Climate: Part I. Berlin: Springer. doi: 10.1007/s00382-017-3597-x
Nepal, S., Pradhananga, S., Kumar Shrestha, N., Kralisch, S., Shrestha, J. P., and Fink, M. (2021). Space-time variability in soil moisture droughts in the Himalayan region. Hydrol. Earth Syst. Sci. 25, 1761–1783. doi: 10.5194/hess-25-1761-2021
Nüsser, M., Schmidt, S., and Dame, J. (2012). Irrigation and development in the upper indus basin: characteristics and recent changes of a socio-hydrological system in central Ladakh, India. Md. Res. Dev. 32, 51–61. doi: 10.1659/MRD-JOURNAL-D-11-00091.1
Odolinski, R., and Teunissen, P. J. G. (2016). Single-frequency, dual-GNSS versus dual-frequency, single-GNSS: a low-cost and high-grade receivers GPS-BDS RTK analysis. J. Geod. 90, 1255–1278. doi: 10.1007/s00190-016-0921-x
Oerlemans, J.. (2001). Glaciers and Climate Change, eds J. Oerlemans (Rotterdam: AA Balkema Publishers).
Østrem, G., and Stanley, A. (1969). Glacier Mass Balance Measurements: a Manual for Field and Office Work. Ottawa, ON; Oslo: Department of Energy, Mines and Resources; Norwegian Water Re- sources and Electricity Board.
Owen, L. A., and England, J. (1998). Observations on rock glaciers in the Himalayas and Karakoram Mountains of northern Pakistan and India. Geomorphology 26, 199–213. doi: 10.1016/S0169-555X(98)00059-2
Palazzi, E., Hardenberg, J., and Von, Provenzale, A. (2013). Precipitation in the Hindu-Kush Karakoram Himalaya: observations and future scenarios. J. Geophys. Res. Atmos. 118, 85–100. doi: 10.1029/2012JD018697
Pan, M., and Wood, E. F. (2006). Data assimilation for estimating the terrestrial water budget using a constrained ensemble Kalman filter. J. Hydrometeorol. 7, 534–547. doi: 10.1175/JHM495.1
Panday, P. K., Frey, K. E., and Ghimire, B. (2011). Detection of the timing and duration of snowmelt in the Hindu Kush-Himalaya using QuikSCAT, 2000-2008. Environ. Res. Lett. 6, 024007. doi: 10.1088/1748-9326/6/2/024007
Pandey, P.. (2019). Inventory of rock glaciers in Himachal Himalaya, India using high-resolution Google Earth imagery. Geomorphology 340, 103–115. doi: 10.1016/j.geomorph.2019.05.001
Pandit, A., and Ramsankaran, R. (2020). Modeling ice thickness distribution and storage volume of glaciers in Chandra Basin, western Himalayas. J. Mount. Sci. 17, 2011–2022. doi: 10.1007/s11629-019-5718-y
Patil, A., Mohanty, S., and Singh, G. (2020). Snow depth and snow water equivalent retrieval using X-band PolInSAR data. Remote Sens. Lett. 11, 817–826. doi: 10.1080/2150704X.2020.1779373
Patil, A., and Ramsankaran, R. A. A. J. (2017). Improving streamflow simulations and forecasting performance of SWAT model by assimilating remotely sensed soil moisture observations. J. Hydrol. 555, 683–696. doi: 10.1016/j.jhydrol.2017.10.058
Patil, A., and Ramsankaran, R. A. A. J. (2018). Improved streamflow simulations by coupling soil moisture analytical relationship in EnKF based hydrological data assimilation framework. Adv. Water Resour. 121, 173–188. doi: 10.1016/j.advwatres.2018.08.010
Pellicciotti, F., Brock, B., Strasser, U., Burlando, P., Funk, M., and Corripio, J. (2005). An enhanced temperature-index glacier melt model including the shortwave radiation balance: development and testing for Haut Glacier d'Arolla, Switzerland. J. Glaciol. 51, 573–587. doi: 10.3189/172756505781829124
Pelt, W. J. J., Van Oerlemans, J., Reijmer, C. H., Pettersson, R., Pohjola, V. A., Isaksson, E., et al. (2013). An iterative inverse method to estimate basal topography and initialize ice flow models. Cryosphere 7, 987–1006. doi: 10.5194/tc-7-987-2013
Peng, J., Loew, A., Merlin, O., and Verhoest, N. E. C. (2017). A review of spatial downscaling of satellite remotely sensed soil moisture. Rev. Geophys. 55, 341–366. doi: 10.1002/2016RG000543
Pieczonka, T., Bolch, T., Wei, J., and Liu, S. (2013). Heterogeneous mass loss of glaciers in the aksu-tarim catchment (Central Tien Shan) revealed by 1976 KH-9 hexagon and 2009 SPOT-5 stereo imagery. Remote Sens. Environ. 130, 233–244. doi: 10.1016/j.rse.2012.11.020
Prasch, M., Mauser, W., and Weber, M. (2013). Quantifying present and future glacier melt-water contribution to runoff in a central Himalayan river basin. Cryosphere 7, 889–904. doi: 10.5194/tc-7-889-2013
Pratap, B., Dobhal, D., Bhambri, R., and Mehta, M. (2016). Four decades of glacier mass balance observations in the Indian Himalaya. Reg. Environ. Chang. 16, 643–658. doi: 10.1007/s10113-015-0791-4
Pritchard, H. D.. (2019). Asia's shrinking glaciers protect large populations from drought stress. Nature 569, 649–654. doi: 10.1038/s41586-019-1240-1
Pritchard, H. D., King, E. C., Goodger, D. J., McCarthy, M., Mayer, C., and Kayastha, R. (2020). Towards bedmap himalayas: development of an airborne ice-sounding radar for glacier thickness surveys in high-mountain Asia. Ann. Glaciol. 61, 35–45. doi: 10.1017/aog.2020.29
Rabatel, A., Castebrunet, H., Favier, V., Nicholson, L., and Kinnard, C. (2011). Glacier changes in the Pascua-Lama region, Chilean Andes (29?S): recent mass balance and 50 yr surface area variations. Cryosphere 5, 1029–1041. doi: 10.5194/tc-5-1029-2011
Rabatel, A., Dedieu, J., and Vincent, C. (2005). Using remote-sensing data to determine equilibrium-line altitute and mass-balance time series:validationon three French glacier, 1994-2002. J. Glaciol. 51, 539–546. doi: 10.3189/172756505781829106
Rabatel, A., Sanchez, O., Vincent, C., and Six, D. (2018). Estimation of glacier thickness from surface mass balance and ice flow velocities: a case study on argentière glacier, France. Front. Earth Sci. 6, 112. doi: 10.3389/feart.2018.00112
Radić, V., Bliss, A., Beedlow, A. C., Hock, R., Miles, E., and Cogley, J. G. (2014). Regional and global projections of twenty-first century glacier mass changes in response to climate scenarios from global climate models. Clim. Dyn. 42, 37–58. doi: 10.1007/s00382-013-1719-7
Radić, V., and Hock, R. (2010). Regional and global volumes of glaciers derived from statistical upscaling of glacier inventory data. J. Geophys. Res. Earth Surf. 115, F01010. doi: 10.1029/2009JF001373
Ragettli, S., Immerzeel, W. W., and Pellicciotti, F. (2016). Contrasting climate change impact on river flows from high-altitude catchments in the Himalayan and Andes Mountains. PNAS 113, 9222–9227. doi: 10.1073/pnas.1606526113
Rai, S. P., Singh, D., Jacob, N., Rawat, Y. S., Arora, M., and Kumar, B. (2019). Identifying contribution of snowmelt and glacier melt to the Bhagirathi River (Upper Ganga) near snout of the Gangotri Glacier using environmental isotopes. Catena 173, 339–351. doi: 10.1016/j.catena.2018.10.031
Raina, V. K.. (2009). Himalayan glaciers: a state-of-art review of glacial studies, glacial retreat and climate change. Himal. Glaciers State-Art Rev. Glacial Stud. Glacial Retreat Clim. Change, Ministry of Environment & Forests, Government of India, New Delhi
Raina, V. K., Kaul, M. K., and Singh, S. (1977). Mass-balance studies of Gara glacier. J. Glaciol. 18, 415–423. doi: 10.1017/S0022143000021092
Ramsankaran, R. A. A. J., Pandit, A., and Azam, M. F. (2018). Spatially distributed ice-thickness modelling for Chhota Shigri Glacier in western Himalayas, India. Int. J. Remote Sens. 39, 3320–3343. doi: 10.1080/01431161.2018.1441563
Rathore, B. P., Bahuguna, I. M., Singh, S. K., Brahmbhatt, R. M., Randhawa, S. S., Jani, P., et al. (2018). Trends of snow cover in Western and West-Central Himalayas during 2004-2014. Curr. Sci. 114, 800–807. doi: 10.18520/cs/v114/i04/800-807
Reichle, R. H., Entekhabi, D., and McLaughlin, D. B. (2001). Downscaling of radio brightness measurements for soil moisture estimation: a four-dimensional variational data assimilation approach. Water Resour. Res. 37, 2353–2364. doi: 10.1029/2001WR000475
Reid, T. D., and Brock, B. W. (2010). An energy-balance model for debris-covered glaciers including heat conduction through the debris layer. J. Glaciol. 56, 903–916. doi: 10.3189/002214310794457218
RGI Consortium (2017). Randolph Glacier Inventory—A Dataset of Global Glacier Outlines: Version 6.0: Technical Report, Global Land Ice Measurements from Space. Boulder, CO: Digital Media.
Rogger, M., Chirico, G. B., Hausmann, H., Krainer, K., Brückl, E., Stadler, P., et al. (2017). Impact of mountain permafrost on flow path and runoff response in a high alpine catchment. Water Resour. Res. 53, 1288–1308. doi: 10.1002/2016WR019341
Rounce, D. R., Hock, R., and Shean, D. E. (2020). Glacier mass change in high mountain asia through 2100 using the open-source python glacier evolution model (PyGEM). Front. Earth Sci. 7, 331. doi: 10.3389/feart.2019.00331
Sahu, R., and Gupta, R. D. (2020). Snow cover area analysis and its relation with climate variability in Chandra basin, Western Himalaya, during 2001–2017 using MODIS and ERA5 data. Environ. Monit. Assess. 192, 1–16. doi: 10.1007/s10661-020-08442-8
Saintenoy, A., Friedt, J. M., Booth, A. D., Tolle, F., Bernard, E., Laffly, D., et al. (2013). Deriving ice thickness, glacier volume and bedrock morphology of Austre Lovénbreen (Svalbard) using GPR. Near Surf. Geophys. 11, 253–261. doi: 10.3997/1873-0604.2012040
Sangewar, C. V., and Sangewar, M. A. (2007). Siddiqui, Thematic Compilation of Mass Balance Data on Glaciers of Satluj Catchment in Himachal Himalaya (Field Season: 2006-07). Lucknow: Geological Survey of India.
Sattar, A., Goswami, A., Kulkarni, A.nil,. V, Emmer, A., Haritashya, U. K., et al. (2021). Future glacial lake outburst flood (GLOF) hazard of the South Lhonak Lake, Sikkim Himalaya. Geomorphology 388, 107783. doi: 10.1016/j.geomorph.2021.107783
Sattar, A., Goswami, A., Kulkarni, A. V., and Das, P. (2019). Glacier-surface velocity derived ice volume and retreat assessment in the dhauliganga basin, central himalaya – a remote sensing and modeling based approach. Front. Earth Sci. 7, 105. doi: 10.3389/feart.2019.00105
Scherler, D., Wulf, H., and Gorelick, N. (2018). Global Assessment of supraglacial debris-cover extents. Geophys. Res. Lett. 11,798–805. doi: 10.1029/2018GL080158
Schmid, M. O., Baral, P., Gruber, S., Shahi, S., Shrestha, T., Stumm, D., et al. (2015). Assessment of permafrost distribution maps in the Hindu Kush Himalayan region using rock glaciers mapped in Google Earth. Cryosphere 9, 2089–2099. doi: 10.5194/tc-9-2089-2015
Seyoum, W. M., and Milewski, A. M. (2017). Improved methods for estimating local terrestrial water dynamics from GRACE in the Northern High Plains. Adv. Water Resour. 110, 279–290. doi: 10.1016/j.advwatres.2017.10.021
Shannon, S., Smith, R., Wiltshire, A., Payne, T., Huss, M., Betts, R., et al. (2019). Global glacier volume projections under high-end climate change scenarios. Cryosphere 13, 325–350. doi: 10.5194/tc-13-325-2019
Sharma, V., Mishra, V. D., and Joshi, P. K. (2012). Snow cover variation and streamflow simulation in a snow-fed river basin of the Northwest Himalaya. J. Mount. Sci. 9, 853–868. doi: 10.1007/s11629-012-2419-1
Shea, J. M., Immerzeel, W. W., Wagnon, P., Vincent, C., and Bajracharya, S. (2015). Modelling glacier change in the Everest region, Nepal Himalaya. The Cryosphere 9, 1105–1128. doi: 10.5194/tc-9-1105-2015
Shean, D. E.. (2017). High Mountain Asia 8-Meter DEM Mosaics Derived From Optical Imagery. Version 1.
Shean, D. E., Bhushan, S., Montesano, P., Rounce, D. R., Arendt, A., and Osmanoglu, B. (2020). A systematic, regional assessment of high mountain asia glacier mass balance. Front. Earth Sci. 7, 363. doi: 10.3389/feart.2019.00363
Sherpa, S. F., Wagnon, P., Brun, F., Berthier, E., Vincent, C., Lejeune, Y., et al. (2017). Contrasted surface mass balances of debris-free glaciers observed between the southern and the inner parts of the Everest region (2007–15). J. Glaciol. 63, 637–651. doi: 10.1017/jog.2017.30
Shrestha, M., Koike, T., Hirabayashi, Y., Xue, Y., Wang, L., Rasul, G., et al. (2015). Integrated simulation of snow and glacier melt in water and energy balance-based, distributed hydrological modeling framework at Hunza River Basin of Pakistan Karakoram region. J. Geophys. Res. Atmosph. 120, 4889–4919. doi: 10.1002/2014JD022666
Shrivastava, D., Sangewar, C. V., Kaul, M. K., and Jamwal, K. S. (2001). “Mass balance of Rulung Glacier- a Trans-Himalayan glacier, Indus basin, Ladakh,” in Proceeding of Symposium Snow, Ice and Glacier, March 1999 (Lucknow), 41–46
Shugar, D. H., Burr, A., Haritashya, U. K., Kargel, J. S., Watson, C. S., Kennedy, M. C., et al. (2020). Rapid worldwide growth of glacial lakes since 1990. Nat. Clim. Chang. 10, 939–945. doi: 10.1038/s41558-020-0855-4
Shugar, D. H., Jacquemart, M., Shean, D., Bhushan, S., Upadhyay, K., Sattar, A., et al. (2021). A massive rock and ice avalanche caused the 2021 disaster at Chamoli, Indian Himalaya. Science 373, 300–306. doi: 10.1126/science.abh4455
Singh, A. K., Tripathi, J. N., Kotlia, B. S., Singh, K. K., and Kumar, A. (2019). Monitoring groundwater fluctuations over India during Indian summer monsoon (ISM) and Northeast monsoon using GRACE satellite: Impact on agriculture. Quat. Int. 507, 342–351. doi: 10.1016/j.quaint.2018.10.036
Singh, A. T., Laluraj, C. M., Sharma, P., Redkar, B. L., Patel, L. K., Pratap, B., et al. (2021). Hydrograph apportionment of the Chandra River draining from a semi-arid region of the Upper Indus Basin, western Himalaya. Sci. Total Environ. 780, 146500. doi: 10.1016/j.scitotenv.2021.146500
Singh, D. K., Gusain, H. S., Mishra, V., and Gupta, N. (2018). Snow cover variability in North-West Himalaya during last decade. Arab. J. Geosci. 11, 579. doi: 10.1007/s12517-018-3926-3
Singh, S., Sood, V., Prashar, S., and Kaur, R. (2020). Response of topographic control on nearest-neighbor diffusion-based pan-sharpening using multispectral MODIS and AWiFS satellite dataset. Arab. J. Geosci. 13, 1–9. doi: 10.1007/s12517-020-05686-z
Singh, S., Sood, V., Taloor, A. K., Prashar, S., and Kaur, R. (2021). Qualitative and quantitative analysis of topographically derived CVA algorithms using MODIS and Landsat-8 data over Western Himalayas, India. Quat. Int. 575–576, 85–95. doi: 10.1016/j.quaint.2020.04.048
Singh, S. K., Rathore, B. P., Bahuguna, I. M., and Ajai. (2014). Snow cover variability in the Himalayan-Tibetan region. Int. J. Climatol. 34, 446–452. doi: 10.1002/joc.3697
Singh, V., Jain, S. K., and Shukla, S. (2021). Glacier change and glacier runoff variation in the Himalayan Baspa river basin. J. Hydrol. 593, 125918. doi: 10.1016/j.jhydrol.2020.125918
Sirguey, P., Still, H., Cullen, N. J., Dumont, M., Arnaud, Y., and Conway, J. P. (2016). Reconstructing the mass balance of Brewster Glacier, New Zealand, using MODIS-derived glacier-wide albedo. Cryospherea 10, 2465–2484. doi: 10.5194/tc-10-2465-2016
Sjöberg, Y., Marklund, P., Pettersson, R., and Lyon, S. W. (2015). Geophysical mapping of palsa peatland permafrost. Cryosphere 9, 465–478. doi: 10.5194/tc-9-465-2015
Smith, T., and Bookhagen, B. (2018). Changes in seasonal snow water equivalent distribution in high mountain Asia (1987 to 2009). Sci. Adv. 4, e1701550. doi: 10.1126/sciadv.1701550
Smith, T., Bookhagen, B., and Rheinwalt, A. (2017). Spatiotemporal patterns of High Mountain Asia's snowmelt season identified with an automated snowmelt detection algorithm, 1987-2016. Cryosphere 11, 2329–2343. doi: 10.5194/tc-11-2329-2017
Sneeuw, N., Lorenz, C., Devaraju, B., Tourian, M. J., Riegger, J., Kunstmann, H., et al. (2014). Estimating runoff using hydro-geodetic approaches. Surv. Geophys. 35, 1333–1359. doi: 10.1007/s10712-014-9300-4
Sneeuw, N., and Sharifi, M. A. (2015). “Rosborough Representation in Satellite Gravimetry,” in VIII Hotine-Marussi Symposium on Mathematical Geodesy, eds N. Sneeuw, P. Novák, M. Crespi, and F. Sansò (Cham: Springer), 109–114. doi: 10.1007/1345_2015_68
Soheb, M., Alagappan, R., Angchuk, T., Mandal, A., Kumar, N., and Lotus, S. (2020). Mass-balance observation, reconstruction and sensitivity of stok glacier, Ladakh region, India, between 1978 and 2019. J. Glaciol. 66, 1–16. doi: 10.1017/jog.2020.34
Sood, V., Singh, S., Taloor, A. K., Prashar, S., and Kaur, R. (2020). Monitoring and mapping of snow cover variability using topographically derived NDSI model over north Indian Himalayas during the period 2008–19. Appl. Comput. Geosci. 8, 100040. doi: 10.1016/j.acags.2020.100040
Srivastava, S., and Azam, M. F. (2022). Mass-, and energy-balance modelling, and sublimation losses on Dokriani Bamak and Chhota Shigri glaciers in Himalaya since 1979. Front. Water. 4, 874240. doi: 10.3389/frwa.2022.874240
Steiner, J. F., Kraaijenbrink, P. D. A., and Immerzeel, W. W. (2021). Distributed melt on a debris-covered glacier: field observations and melt modeling on the lirung glacier in the Himalaya. Front. Earth Sci. 9, 567. doi: 10.3389/feart.2021.678375
Stigter, E. M., Wanders, N., Saloranta, T. M., Shea, J. M., Bierkens, M. F. P., and Immerzeel, W. W. (2017). Assimilation of snow cover and snow depth into a snow model to estimate snow water equivalent and snowmelt runoff in a Himalayan catchment. Cryosphere 11, 1647–1664. doi: 10.5194/tc-11-1647-2017
Sun, A. Y.. (2013). Predicting groundwater level changes using GRACE data. Water Resour. Res. 49, 5900–5912. doi: 10.1002/wrcr.20421
Sunako, S., Fujita, K., Sakai, A., and Kayastha, R. B. (2019). Mass balance of Trambau Glacier, Rolwaling region, Nepal Himalaya: in-situ observations, long-term reconstruction and mass-balance sensitivity. J. Glaciol. 1–12. 65, 605–616. doi: 10.1017/jog.2019.37
Surazakov, A., and Aizen, V. B. (2010). Positional accuracy evaluation of declassified Hexagon KH-9 mapping camera imagery. Photogramm. Eng. Remote Sens. 76, 603–608. doi: 10.14358/PERS.76.5.603
Swenson, S., and Wahr, J. (2006). Post-processing removal of correlated errors in GRACE data. Geophys. Res. Lett. 33, 1–4. doi: 10.1029/2005GL025285
Tak, S., and Keshari, A. K. (2020). Investigating mass balance of Parvati glacier in Himalaya using satellite imagery based model. Sci. Rep. 10, 12211. doi: 10.1038/s41598-020-69203-8
Tangang, F., Chung, J. X., Juneng, L., Supari, S. E., Ngai, S. T., Jamaluddin, A. F., et al. (2020). Projected future changes in rainfall in Southeast Asia based on CORDEX–SEA multi-model simulations. Clim. Dyn. 55, 1247–1267. doi: 10.1007/s00382-020-05322-2
Tapley, B. D., Watkins, M. M., Flechtner, F., Reigber, C., Bettadpur, S., Rodell, M., et al. (2019). Contributions of GRACE to understanding climate change. Nat. Clim. Chang. 9, 358–369. doi: 10.1038/s41558-019-0456-2
Tawde, S. A., Kulkarni, A. V., and Bala, G. (2016). Estimation of glacier mass balance on a basin scale: an approach based on satellite-derived snowlines and a temperature index model. Curr. Sci. 111, 1977–1989. doi: 10.18520/cs/v111/i12/1977-1989
Tawde, S. A., Kulkarni, A. V., and Bala, G. (2017). An estimate of glacier mass balance for the Chandra basin, western Himalaya, for the period 1984-2012. Ann. Glaciol. 58, 99–109. doi: 10.1017/aog.2017.18
Thakur, P. K., Aggarwal, S. P., Arun, G., Sood, S., Senthil Kumar, A., Mani, S., et al. (2017). Estimation of snow cover area, snow physical properties and glacier classification in parts of western Himalayas using C-Band SAR data. J. Indian Soc. Remote. Sens. 45, 525–539. doi: 10.1007/s12524-016-0609-y
Thakur, P. K., Garg, P. K., Aggarwal, S. P., Garg, R. D., and Mani, S. (2013). Snow cover area mapping using synthetic aperture radar in Manali watershed of Beas river in the northwest Himalayas. J. Indian Soc. Remote. Sens. 41, 933–945. doi: 10.1007/s12524-012-0236-1
Thayyen, R. J., and Dimri, A. P. (2018). Factors controlling slope environmental lapse rate (SELR) of temperature in the monsoon and cold-arid glacio-hydrological regimes of the Himalaya. Front. Environ. Sci. 6, 42. doi: 10.3389/fenvs.2018.00042
Tiwari, S., Kar, S. C., and Bhatla, R. (2016). Interannual variability of snow water equivalent (SWE) over Western Himalayas. Pure Appl. Geophys. 173, 1317–1335. doi: 10.1007/s00024-015-1163-1
Tiwari, V. M., Wahr, J., and Swenson, S. (2009). Dwindling groundwater resources in northern India, from satellite gravity observations. Geophys. Res. Lett. 36, 1–5. doi: 10.1029/2009GL039401
Tourian, M. J., Sneeuw, N., and Bárdossy, A. (2013). A quantile function approach to discharge estimation from satellite altimetry (ENVISAT). Water Resour. Res. 49, 4174–4186. doi: 10.1002/wrcr.20348
Trewin, B., Cazenave, A., Howell, S., Huss, M., Isensee, K., Palmer, M. D., et al. (2021). Headline indicators for global climate monitoring. Bull. Am. Meteorol. Soc. 102, E20–E37. doi: 10.1175/BAMS-D-19-0196.1
Tshering, P., and Fujita, K. (2016). First in situ record of decadal glacier mass balance (2003–2014) from the Bhutan Himalaya. Ann. Glaciol. 57, 289–294. doi: 10.3189/2016AoG71A036
Veh, G., Korup, O., and Walz, A. (2020). Hazard from Himalayan glacier lake outburst floods. Proc. Natl. Acad. Sci. U.S.A. 117, 907–912. doi: 10.1073/pnas.1914898117
Vijay, S., and Braun, M. (2016). Elevation change rates of glaciers in the lahaul-spiti (Western Himalaya, India) during. Remote Sens. 8, 1038. doi: 10.3390/rs8121038
Vijay, S., and Braun, M. (2018). Early 21st century spatially detailed elevation changes of Jammu and Kashmir glaciers (Karakoram – Himalaya). Glob. Planet. Change 165, 137–146. doi: 10.1016/j.gloplacha.2018.03.014
Vincent, C., Ramanathan, Al., Wagnon, P., Dobhal, D. P., Linda, A., Berthier, E., et al. (2013). Balanced conditions or slight mass gain of glaciers in the Lahaul and Spiti region (northern India, Himalaya) during the nineties preceded recent mass loss. Cryosphere 7, 569–582. doi: 10.5194/tc-7-569-2013
Vincent, C., Wagnon, P., Shea, J. M., Immerzeel, W. W., Kraaijenbrink, P., Shrestha, D., et al. (2016). Reduced melt on debris-covered glaciers: investigations from Changri Nup Glacier, Nepal. Cryosphere 14, 1845–1858. doi: 10.5194/tc-10-1845-2016
Vishwakarma, B. D.. (2020). Monitoring droughts from GRACE. Front. Environ. Sci. 8, 584690. doi: 10.3389/fenvs.2020.584690
Vishwakarma, B. D., Bates, P., Sneeuw, N., Westaway, R. M., and Bamber, J. L. (2021). Re-assessing global water storage trends from GRACE time series. Environ. Res. Lett. 16, 034005. doi: 10.1088/1748-9326/abd4a9
Vishwakarma, B. D., Devaraju, B., and Sneeuw, N. (2016). Minimizing the effects of filtering on catchment scale GRACE solutions Bramha. J. Am. Water Resour. Assoc. 52, 5868–5890. doi: 10.1002/2016WR018960
Vishwakarma, B. D., Devaraju, B., and Sneeuw, N. (2018). What is the spatial resolution of GRACE satellite products for hydrology? Remote Sens. 10, 1–17. doi: 10.3390/rs10060852
Vishwakarma, B. D., Horwath, M., Devaraju, B., Groh, A., and Sneeuw, N. (2017). A data-driven approach for repairing the hydrological catchment signal damage due to filtering of GRACE products. Water Resour. Res. 53, 9824–9844. doi: 10.1002/2017WR021150
Wagnon, P., Brun, F., Khadka, A., Berthier, E., Shrestha, D., Vincent, C., et al. (2021). Reanalysing the 2007–19 glaciological mass-balance series of mera glacier, Nepal, Central Himalaya, using geodetic mass balance. J. Glaciol. 67, 117–125. doi: 10.1017/jog.2020.88
Wagnon, P., Linda, A., Arnaud, Y., Kumar, R., Sharma, P., Vincent, C., et al. (2007). Four years of mass balance on Chhota Shigri Glacier, Himachal Pradesh, India, a new benchmark glacier in the western himalaya four years of mass balance on Chhota Shigri Glacier, Himachal Pradesh, India, a new benchmark glacier in the western Himala. J. Glaciol. 53, 603. doi: 10.3189/002214307784409306
Wagnon, P., Ribstein, P., Francou, B., and Pouyaud, B. (1999). Annual cycle of energy balance of Zongo Glacier, Cordillera Real, Bolivia. J. Geophys. Res. 104, 3907–3923. doi: 10.1029/1998JD200011
Wagnon, P., Vincent, C., Arnaud, Y., Berthier, E., Vuillermoz, E., Gruber, S., et al. (2013). Seasonal and annual mass balances of Mera and Pokalde glaciers (Nepal Himalaya) since 2007. Cryosphere 7, 1769–1786. doi: 10.5194/tc-7-1769-2013
Wang, C., Wang, Z., Kong, Y., Zhang, F., Yang, K., and Zhang, T. (2019). Most of the northern hemisphere permafrost remains under climate change. Sci. Rep. 9, 3295. doi: 10.1038/s41598-019-39942-4
Wang, Q., Yi, S., and Sun, W. (2018). Consistent interannual changes in glacier mass balance and their relationship with climate variation on the periphery of the Tibetan Plateau. Geophys. J. Int. 214, 573–582. doi: 10.1093/gji/ggy164
Wang, Q., Yi, S., and Sun, W. (2021). Continuous estimates of glacier mass balance in high mountain asia based on ICESat-1,2 and GRACE/GRACE follow-on data. Geophys. Res. Lett. 48, e90954. doi: 10.1029/2020GL090954
Wang, S., Sheng, Y., Li, J., Wu, J., Cao, W., and Ma, S. (2018). An estimation of ground ice volumes in permafrost layers in Northeastern Qinghai-Tibet Plateau, China. Chin. Geogr. Sci. 28, 61–73. doi: 10.1007/s11769-018-0932-z
Wang, Y., Huang, X., Wang, J., Zhou, M., and Liang, T. (2019). AMSR2 snow depth downscaling algorithm based on a multifactor approach over the Tibetan Plateau, China. Remote Sens. of Environ. 231, 111268. doi: 10.1016/j.rse.2019.111268
Wani, J. M., Thayyen, R. J., Gruber, S., Ojha, C. S. P., and Stumm, D. (2020). Single-year thermal regime and inferred permafrost occurrence in the upper Ganglass catchment of the cold-arid Himalaya, Ladakh, India. Sci. Total Environ. 703, 134631. doi: 10.1016/j.scitotenv.2019.134631
Weigelt, M.. (2017). “The acceleration approach,” in Global Gravity Field Modeling from Satellite-to-Satellite Tracking Data, Lecture Notes in Earth System Sciences, eds M. Naeimi, and J. Flury (Cham: Springer), 97–126. doi: 10.1007/978-3-319-49941-3_4
Welty, E., Zemp, M., Navarro, F., Huss, M., Fürst, J. J., Gärtner-Roer, I., et al. (2020). Worldwide version-controlled database of glacier thickness observations. Earth Syst. Sci. Data 12, 3039–3055. doi: 10.5194/essd-12-3039-2020
Werder, M. A., Huss, M., Paul, F., Dehecq, A., and Farinotti, D. (2019). A Bayesian ice thickness estimation model for large-scale applications. J. Glaciol. 66, 137–152. doi: 10.1017/jog.2019.93
Wortmann, M., Bolch, T., Menz, C., Tong, J., and Krysanova, V. (2018). Comparison and correction of high-mountain precipitation data based on glacio-hydrological modeling in the Tarim River headwaters (High Asia). J Hydrometeorol. 19, 777–801. doi: 10.1175/JHM-D-17-0106.1
Wouters, B., Gardner, A. S., and Moholdt, G. (2019). Global glacier mass loss during the GRACE satellite mission (2002-2016). Front. Earth Sci. 7, 96. doi: 10.3389/feart.2019.00096
Xiao, X., Zhang, T., Zhong, X., Shao, W., and Li, X. (2018). Support vector regression snow-depth retrieval algorithm using passive microwave remote sensing data. Remote Sens. Environ. 210, 48–64. doi: 10.1016/j.rse.2018.03.008
Yang, J. W., Jiang, L. M., Lemmetyinen, J., Pan, J. M., Luojus, K., and Takala, M. (2021). Improving snow depth estimation by coupling HUT-optimized effective snow grain size parameters with the random forest approach. Remote Sens. Environ. 264, 112630. doi: 10.1016/j.rse.2021.112630
Yao, T., Bolch, T., Chen, D., Gao, J., Immerzeel, W., Piao, S., et al. (2022). The imbalance of the Asian water tower. Nat. Rev. Earth Environ. 1–15. doi: 10.1038/s43017-022-00299-4
Yao, T., Thompson, L., Yang, W., Yu, W., Gao, Y., Guo, X., et al. (2012). Different glacier status with atmospheric circulations in Tibetan Plateau and surroundings. Nat. Clim. Change 2, 663–667. doi: 10.1038/nclimate1580
You, Q., Ren, G., Zhang, Y., Ren, Y., Sun, X., Zhan, Y.-J., et al. (2017). An overview of studies of observed climate change in the Hindu Kush Himalayan (HKH) region. Adv. Clim. Chang. Res. 8, 141–147. doi: 10.1016/j.accre.2017.04.001
Zaitchik, B. F., Rodell, M., and Reichle, R. H. (2008). Assimilation of GRACE terrestrial water storage data into a land surface model: results for the Mississippi River basin. J. Hydrometeorol. 9, 535–548. doi: 10.1175/2007JHM951.1
Zaman, Q., and Liu, J. (2015). Mass balance of Siachen Glacier, Nubra valley, Karakoram Himalaya: facts or flaws? J. Glaciol. 61, 1012–1014. doi: 10.3189/2015JoG15J120
Zemp, M., Hoelzle, M., and Haeberli, W. (2009). Six decades of glacier mass-balance observations: a review of the worldwide monitoring network. Ann. Glaciol. 50, 101–111. doi: 10.3189/172756409787769591
Zemp, M., Huss, M., Thibert, E., Eckert, N., McNabb, R., Huber, J., et al. (2019). Global glacier mass changes and their contributions to sea-level rise from 1961 to 2016. Nature 568, 382–386. doi: 10.1038/s41586-019-1071-0
Zhang, K., Kimball, J. S., and Running, S. W. (2016). A review of remote sensing based actual evapotranspiration estimation. Wiley Interdiscip. Rev. Water 3, 834–853. doi: 10.1002/wat2.1168
Zhang, Z., and Wu, Q. (2012). Thermal hazards zonation and permafrost change over the Qinghai–Tibet Plateau. Nat. Haz. 61, 403–423. doi: 10.1007/s11069-011-9923-4
Zhou, Y., Li, Z., Li, J., Zhao, R., and Ding, X. (2018). Glacier mass balance in the Qinghai–Tibet Plateau and its surroundings from the mid-1970s to 2000 based on Hexagon KH-9 and SRTM DEMs. Remote Sens. Environ. 210, 96–112. doi: 10.1016/j.rse.2018.03.020
Appendix
Keywords: himalayan glaciology, himalayan hydroclimate, glacier mass balance, climate–change, third pole environment
Citation: Vishwakarma BD, Ramsankaran R, Azam MF, Bolch T, Mandal A, Srivastava S, Kumar P, Sahu R, Navinkumar PJ, Tanniru SR, Javed A, Soheb M, Dimri AP, Yadav M, Devaraju B, Chinnasamy P, Reddy MJ, Murugesan GP, Arora M, Jain SK, Ojha CSP, Harrison S and Bamber J (2022) Challenges in Understanding the Variability of the Cryosphere in the Himalaya and Its Impact on Regional Water Resources. Front. Water 4:909246. doi: 10.3389/frwa.2022.909246
Received: 31 March 2022; Accepted: 10 June 2022;
Published: 28 July 2022.
Edited by:
Riyaz Ahmad Mir, Geological Survey of India, IndiaReviewed by:
Vaibhav Garg, Indian Institute of Remote Sensing, IndiaMuhammad Shahid, National University of Sciences and Technology (NUST), Pakistan
Mohd Yawar Ali Khan, King Abdulaziz University, Saudi Arabia
Copyright © 2022 Vishwakarma, Ramsankaran, Azam, Bolch, Mandal, Srivastava, Kumar, Sahu, Navinkumar, Tanniru, Javed, Soheb, Dimri, Yadav, Devaraju, Chinnasamy, Reddy, Murugesan, Arora, Jain, Ojha, Harrison and Bamber. This is an open-access article distributed under the terms of the Creative Commons Attribution License (CC BY). The use, distribution or reproduction in other forums is permitted, provided the original author(s) and the copyright owner(s) are credited and that the original publication in this journal is cited, in accordance with accepted academic practice. No use, distribution or reproduction is permitted which does not comply with these terms.
*Correspondence: Bramha Dutt Vishwakarma, YnJhbWhhQGlpc2MuYWMuaW4=