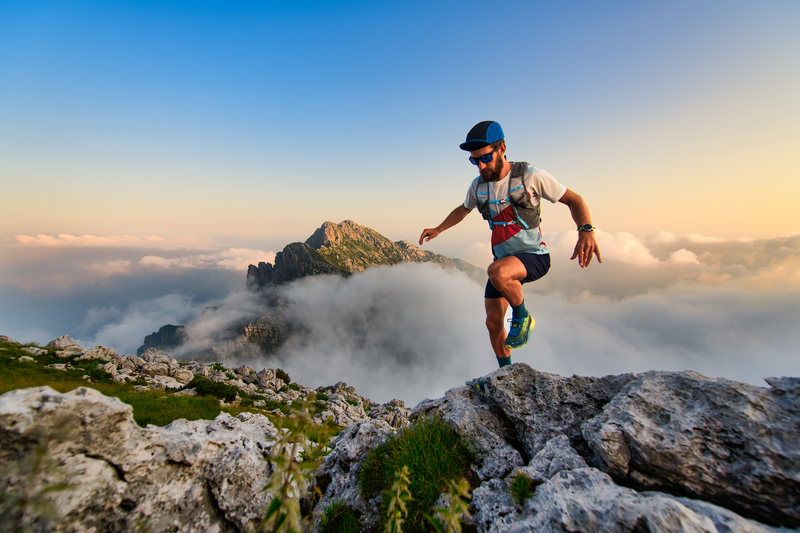
94% of researchers rate our articles as excellent or good
Learn more about the work of our research integrity team to safeguard the quality of each article we publish.
Find out more
REVIEW article
Front. Water , 07 April 2022
Sec. Water and Human Systems
Volume 4 - 2022 | https://doi.org/10.3389/frwa.2022.848686
This article is part of the Research Topic Limits and Permanence of Modern Interventions in the Water Cycle View all 8 articles
The application of distributed ledger technologies, including blockchain, is rapidly growing in governance, transport, supply chain, and logistics. Today, blockchain technology is promoted as the heart of Smart Cities. This study reviews the potential of blockchain application in water management systems. We surveyed the literature and organized the previous studies based on three main application topics: Smart Water Systems, Water Quality Monitoring, and Storm Water Management. Also, we addressed technical, organizational, social, and institutional challenges that may hinder the adoption of Blockchain technology. Water management systems need to have a long-term commitment plan, update their organizational policies, and acquire relevant knowledge and expertise before successfully adopting any distributed ledger technology.
The expansion of internet access, improved intelligent devices, and the advancement of information and communication technologies (ICT) have created opportunities for even more interconnection of physical and virtual domains (Caragliu et al., 2011; Shahid et al., 2018). Our modern life is entangled with an inter-networking of embedded devices, sensors, and computers known as the Internet of Things (IoT) that collect and distribute a large amount of data (Rose et al., 2015). The development of cloud platforms that use several servers to store data has improved IoT extensively (Tsague and Twala, 2018). This growing system of interconnected smart objects utilizing the Internet and supporting technologies is paving the way to create smart cities that could involve smart homes, connected automotive, digitized healthcare, smart environment control, effective monitoring of the quality of water, and much more (Shahid et al., 2018; Shilpi and Ahad, 2020). Nevertheless, The IoT sector faces data security and privacy challenges leading to device spoofing, false authentication, and less reliability in data sharing (Rose et al., 2015; Kumar and Mallick, 2018; Qatawneh et al., 2020).
Blockchain solutions are among emerging technology that is used to keep track of ownership of assets and record transactions in the form of distributed ledger, hence improving data security and privacy (Teeluck et al., 2021). Blockchain was the steppingstone for the creation of cryptocurrencies (Nakamoto, 2008). However, during the past two decades, the application of blockchains has expanded to manage smart assets and create and manage smart contracts (Christidis and Devetsikiotis, 2016).
In blockchains, all the data is saved into immutable blocks providing a secure peer-to-peer transaction without a third-party authentication (Brotsis et al., 2021). A blockchain is a series of time-stamped batches of information (i.e., blocks). Each block contains a unique string of alphanumeric characters of fixed length called hash, a hash of the previous block, an index, a time-stamp, the data, and a hash of the data. The hash of each block is calculated using the information from its previous block (Teeluck et al., 2021).
Unlike conventional methods of storing data, blockchain technology is decentralized, which means that the information is stored on a network of participating computers or nodes (Reyna et al., 2018; Teeluck et al., 2021). The blockchain is called public if participating in and contributing to the network's computing power does not require permission from other host members, and all nodes have equal rights. On the other hand, the blockchain is private if a centralized organization act as a certificate authority that can change the rules (including the consensus mechanism) and revert or modify the transactions. A consortium blockchain mixes the previous two types that only preselected trusted nodes participate in the consensus mechanism (Akram et al., 2020).
A new block is processed and added to the chain when a transaction occurs, known as mining (Reyna et al., 2018). Before any new block is added to the chain, it is required that participating nodes form a consensus about the authenticity of the new information. There are 12 different types of consensus mechanisms based on the application, and the Blockchain architecture and each network define its consensus mechanism (Teeluck et al., 2021).
In essence, the four main characteristics of blockchain technology differentiate it from most existing information systems designs (see Figure 1). These key characteristics include decentralization (non-localization), security, auditability, and smart execution (Saberi et al., 2019).
Figure 1. Steps in blockchain information and transactions (Saberi et al., 2019).
Blockchain is implemented in various sectors, some using major platforms such as Ethereum or Hyperledger and others smaller custom-made ones. For instance, the technology is used to store information about ownership, authenticity, and transactions related to high-value assets like diamonds; to trace and certify goods across supply chains (end-to-end tracking of containers and certifying origin of food ingredients and products); to provide a platform for energy trading; and to create smart contracts that facilitate transactions in real estate (Teeluck et al., 2021).
In the context of global water scarcity, the spatial distribution of water resources, and multi-scale water resource management, a distributed network approach like blockchain is advantageous to ensure trust in data reliability, data security, and data verification in public water transactions. Combining IoT, AI, and Blockchain as reinforcing technologies can increase public trust, encourage informed decision-making, and yield efficient optimization and water allocation (Lin et al., 2018). This study reviews the literature highlighting the application of smart systems, including IoT and blockchain technologies, in urban water management. We organized the reviewed publications into three main topics: Smart Water Systems, Water Quality Monitoring, and Stormwater Management. In the end, we provide a summary discussion of the benefits and challenges that water managers may face when implementing blockchain technology.
Depleting resources, complex regulations, and increasing demand for clean and affordable water are challenging current water management systems (Sriyono, 2020). Advanced digital technology is used to collect data on the distribution and consumption of water in urban settings, and IoT can be used to monitor the urban environment (Yang et al., 2015; Eggimann et al., 2017). The impacts of employing smart water systems can go beyond efficient management of resources. Innovative, efficient, and scalable solutions based on the integration of Blockchain and IoT for intelligent water and sanitation management in Africa can help nations achieve Sustainable Development Goals (Dogo et al., 2019b,c).
Smart water systems are often defined within the concept of smart cities (D'amico et al., 2020). In a smart city, the physical infrastructure, the IT infrastructure, the social infrastructure, and the business infrastructure are collectively and intelligently connected (Chourabi et al., 2012; Bassoo et al., 2018). Smart water grid systems enhance consumption management, sustainability and compliance, and effective policymaking using integrated Blockchain and IoT technologies (Dogo et al., 2019c; Sriyono, 2020). Furthermore, the adaptation of smart grids helps urban water systems to identify and respond to sustainability and resiliency challenges, including drought and natural disasters, more effectively (Mutchek and Williams, 2014).
Depending on their needs, communities that use smart water technologies integrate various detecting devices and intelligent systems. In Singapore, the smart water grid is designed to track pressure, flow, and disinfectant levels in the distribution system. The data is transmitted through the cell network to a Computer Center to be analyzed in real-time. In case of detecting a problem, the Center alarms the utility and pinpoints the problem location to within 40 meters (Public Utilities Board Singapore, 2016). The East Bay Municipal Utility District (EBMUD) in San Francisco Bay Area has employed several programs that facilitate issue detection and reduce water use. They have tested and deployed advanced leak detection devices, set up smart irrigation controller rebates for consumers, and installed smart metering in conjunction with web-based tools that help users to detect leaks. Early leak detection and repairs resulted, on average 20% reduction in water use (Mutchek and Williams, 2014).
To successfully integrate water management and ICT, a smart water grid needs to configure a platform in both water and ICT networks; guarantee water resources; control water flows intelligently through bi-directional communication in water infrastructure; better manage assets in the water infrastructure; operate and maintain water infrastructure in an energy-efficient manner (Lee et al., 2014). Although the purpose of this review study initially was to find examples of successful and effective water management systems that had implemented DL platforms, we did not find a system that had all these characteristics. That makes the point that current water systems may have only partially benefitted from IoT and DL technologies. The benefits of Intelligent water management systems that use IoT sensors are described and evaluated in different contexts by various scholars. Managers can constantly manage and control both the operational aspects (asset management, leak management, water quality monitoring) and the customer end (automated meter reading and water conservation) using an integrated end-to-end platform that monitors the water distribution systems in real-time (Allen et al., 2012; Public Utilities Board Singapore, 2016; Rojek and Studzinski, 2019; Abdelhafidh et al., 2020).
In the context of water trading, Blockchain technology would increase transparency to auditors and regulators by recording trades and actions on the chain, preventing speculation. Also, implementing blockchain technology in water trading would streamline the counterparty data management process and save millions of dollars by creating a digital identity for organizations, digitizing water assets, and storing the digital assets on a shared ledger (Poberezhna, 2018). From the financial aspect, Blockchain technology facilitates collaboration among counterpart organizations and eliminates manual checks and reconciliation processes (Poberezhna, 2018).
In Australia, for instance, water authorities have partnered with the private sector, Civic Ledger, to improve the transparency and reliability of water market information. A pilot study aiming at assessing the feasibility of blockchain in agricultural water trading markets in Australia revealed that the main barriers to water market participation by irrigators include the complexity of trade process, lack of trust and price transparency in some closed systems, and lack of knowledge (Civic Ledger, 2020). The study found that implementing Blockchain technology to water trading in their case could immediately solve the issues related to authority, ownership, price, volume, and trade history because the market participants would have access to the essential supply chain information in real-time. The authorization issue would be solved by setting up water accounts in the Water Ledger platform where each water account was assigned an owner ID number, hence solving the ownership issue. Historical pricing data was gathered from the irrigators, and therefore by implementing the smart contracts, transparency in water pricing for the future would be resolved.
Smart contracts are effective tools to capture volume data as well. Combined with the historical volume data, water market volume information would be available in real-time going forward. Finally, access to trade history was solved by implementing smart contracts combined with the longitudinal trade data (Civic Ledger, 2020). Of course, the proposed solution design of Civic Ledger is tailored to the issues relevant to water trading in the agricultural sector.
Finding a systematic framework to guide a smart water network's real-world design and deployment is challenging and context-dependent (Li et al., 2020). In addition, evaluating the accuracy and efficiency of the data generated by the IoT and managed by distributed ledger platforms is essential for enhanced, and in some cases autonomous, decision-making in water management. Scientists have proposed various architectural designs to build intelligent water planning and managing systems that fit a specific application. For instance, Xiang et al. (2021) propose an Adaptive Intelligent Dynamic Water Resource Planning (AIDWRP), an AI technique, to effectively model sustainable water development. The goal is to help sustain urban areas' water environment and optimize systems' resource distribution. This computational model aims to reduce water supply costs subjected to a constraint to water requirement. The evaluation of the performance and accuracy of the model show that AI is an effective tool in managing and decision-making in urban water resources management (Xiang et al., 2021).
As another example, Wu et al. (2020) designed two predictive models for water quality based on integrated smart data-driven technologies. They collected raw water data from the urban water supply systems of Oslo and Bergen in Norway to predict biological water quality indicators in supply sources. Even though water supply systems are integrated with ubiquitous sensing technologies, very few appropriate microbial sensors are developed mainly due to the difficulty of measuring and collecting biological indicators of water quality (Wu et al., 2020).
The proposed models by Wu et al. (2020) both show significant predictive accuracy. Considering that water sources are affected by the seepage from agricultural, residential, and industrial users, effective quality control of water sources is essential to the safety planning of the water supply system. The outcome of Wu et al. (2020) proposed predictive models indicates that the Smart Data-Driven framework is an efficient way for future decision-making support of water quality control and risk management.
Some newly proposed architectural designs integrate IoT, Blockchain, Decision Support Systems, and AI technologies to ensure efficient water management. In a proposed design, Pahonṭu et al. (2020) create an experimental water distribution network integrated with a distributed architecture based on Blockchain and Hyperledger Fabric framework. They test the capabilities of their solution to evaluate the intervals for reading and writing data, reorganize the format of the data that is saved into the blockchain, and find a solution that fits the network's decision-making needs.
The major challenge regarding the large-scale adaptation of smart water networks is the lack of a standardized framework for designing and constructing such networks (Li et al., 2020). However, the performance of any proposed smart water system can be evaluated using two conceptual metrics of smartness and cyber wellness that measure system efficiency and security, respectively (Li et al., 2020). The smartness of the system is usually quantified by the time lag between the start input and terminal output and measured in minutes (Marchese and Linkov, 2017). Therefore, smartness aims to minimize the time delay between the system input and output, hence perfecting the real-time monitoring, sampling, transporting, processing, simulating, modeling, and controlling. The information security issue arises from the vulnerability of the IoT component where sensors and actuators across the water distribution network communicate data. Based on the International Telecommunication Union's definition, cyber wellness indicates the maximum data stored before a cyberattack while withstanding cyberattacks as long as possible at the same time (Li et al., 2020).
Water quality refers to water's physical, biological, and chemical characteristics. The important measurable water quality parameters include chemical oxygen demand, biochemical oxygen demand, pH, dissolved oxygen, turbidity, electrical conductivity, temperature, oxidation-reduction potential, salinity, total nitrogen, and total phosphorus (Bahadori and Smith, 2016; Kamaludin and Ismail, 2017). IoT framework enables the real-time monitoring of water quality indices facilitated by the speed of internet communication (Das and Jain, 2017; Kumar Jha et al., 2018). Automation of processes through IoT reduces the need for human resources and consequently limits human errors (Wiliem et al., 2006). Smart monitoring systems are adaptive and responsive, alerting users and decision-makers regarding sub-optimal or dangerous conditions in real-time. IoT-based water quality monitoring is cost-effective compared to traditional manual sampling methods (Spandana and Seshagiri Rao, 2018; Ighalo et al., 2021).
An IoT-based platform for water quality monitoring consists of microcontrollers, sensors, and communication units. A microcontroller is the processing unit in the system; the sensor unit is responsible for the actual data collection; and the communication unit transmits the data (Bai et al., 2020; Ighalo et al., 2021). The collected data is transferred to Cloud storage for further analysis or to a user-friendly PC/Smartphone interface through Wi-Fi modules (Lavanya and Raghavendra Babu, 2019; Shanthi et al., 2019). Some framework designs suggest using solar panels to provide the energy for running the system (Arvind et al., 2020). There are extensive proposed designs for an IoT based water quality monitoring system in the literature, focusing on portability (Tripathy et al., 2020), performance efficiency, and data reliability (Zin et al., 2019; Kumar et al., 2020), cost-effectiveness (Sarraf et al., 2020), and communication ability (Sithole et al., 2019a,b) of the module.
The application of IoT in monitoring water quality ranges from observing any chemical or physical change of water in rivers (Cianchi et al., 2000; Wang et al., 2013; Chowdury et al., 2019; Meshal et al., 2020) and watersheds (Hoos et al., 2019) to identify pollution levels in near real-time (Saravanan et al., 2018); to detection of hydrologic variability in estuarine and marine ecosystems used for early warning systems and rapid response to harmful algal bloom events (Glasgow et al., 2004). Developing countries like Nigeria use IoT to test the quality of surface water, groundwater, rainwater, and commercially available water to ensure access to safe drinking water in remote areas (Ighalo and Adeniyi, 2020).
The data generated from IoT integration into the water source management, treatment, and distribution systems can predict water quality, thus playing an important role in future risk control and decision support in urban water supply systems (Wu et al., 2020). The continuous monitoring of the water supply system creates time-series data that can further be examined to identify the impact of climate parameters, like monsoon in summer or wind speed, on the quality of water delivered to a specific geographical location (Tripathy et al., 2020). Some applications improve in-home conservation decision making where autonomous IoT enabled units can activate various filters used in homes depending on the change in the quality of water delivered to households (Almetwally et al., 2020) or send signals to households if health hazards are detected in the tap water (Sithole et al., 2019b).
Automated water quality monitoring in near real-time generates high-frequency, high-volume data. Anomaly in water quality data is inevitable and can be related to various factors, including sensor malfunction, database failure, and system deviations from its natural behavior (Rodriguez-Perez et al., 2020). Traditional methods of manual data anomaly detection and correction are complemented or replaced by more advanced rule-based, regression-based, and feature-based methods (Rodriguez-Perez et al., 2020). Machine learning (ML) and deep learning (DL) approaches are used in water quality anomaly detection tasks. Through a comprehensive literature review, Dogo et al. (2019a) argue that, in general, DL approaches tend to outperform traditional ML techniques in terms of feature learning accuracy and fewer false-positive rates. They propose a hybrid framework that mixes DL with extreme machine learning as a possible solution to detect anomalies in water quality data (Dogo et al., 2019a). One promising alternative is Artificial Neural Networks (ANNs) which do not require a priori knowledge of the underlying physical and environmental processes (Rodriguez-Perez et al., 2020). ANNs have the flexibility to train models using different learning methods to detect a broad range of anomaly types. For instance, Shi et al. (2018) proposed a wavelet-ANN-based model to detect and warn against water quality anomalies. They applied this approach as a case study to the Potomac River Basin in Virginia, USA, which successfully detected two anomaly events of TP variations from high-frequency online sensors. In Europe, Tinelli and Juran (2019) show how innovative sensing technology solutions, such as ANN, are used in combination with advanced pattern recognizers to ensure a real-time quality control for early chemical and/or bio-contamination detection and pre-emptive risk management purposes.
Stormwater runoff is the excess water not absorbed by saturated, sealed, or impermeable surfaces such as roads (Bassi et al., 2017). Surface runoff volumes and peak flows are significantly higher in urban areas because of the higher share of impermeable surfaces and the limited capacity of water management infrastructures (Bassi et al., 2017). In cities with combined sewage systems, heavy precipitation events damage physical infrastructure and create environmental and health issues when stormwater is mixed with sewage before heading to treatment facilities (Le Jallé et al., 2013). Furthermore, various non-point sources increasingly pollute urban water bodies. One example is commercial nurseries near urban areas that create a pollution problem during storm events. Experimental studies that measure runoff characteristics from commercial nurseries during typical irrigation events show that, on average, total nitrogen (TN) and phosphorus (TP) levels are like that of urban runoff. In contrast, total sediment (TS) is 2–4 times greater. However, during storm events, the average loading of TN, TP, and TS is approximately 35, 50, and 900 times higher than those of irrigation events, respectively (Yazdi et al., 2019).
Municipalities worldwide address the urban runoff issue using engineered (or gray) and green stormwater infrastructures. Gray infrastructures are engineered drainage and treatment systems. In contrast, green infrastructures (GI) are natural and artificial elements and processes used to manage wet weather impacts through improving ecological and hydrological functions (Bassi et al., 2017; Mcphillips and Matsler, 2018). An integrated approach to managing stormwater runoff uses new GI measures to mitigate the impact of runoff volume, speed, and pollution through natural filtration (Bassi et al., 2017). However, collecting data on the runoff characteristics and field observations during urban flooding are rarely available for model calibration and validation (Guan et al., 2021). In the context of climate change, higher severity and frequency of urban flooding are expected, requiring a new approach in short-term forecasting for emergency response and long-term planning for climate adaptation (Guan et al., 2021).
To improve our understanding of the fundamental urban runoff dynamics and associated processes like pollutant transport, we need to enhance our analytical capability and data support in urban flood modeling, using new approaches like using IoT in water system monitoring and the application of DL/ ML in flood prediction (Kabir et al., 2020; Nie et al., 2020; Guan et al., 2021). Digitization can also help improve urban planning processes that aim to reintegrate stormwater into urban water cycles, recognizing stormwater as a resource to increase the supply of water where it is needed the most (Le Jallé et al., 2013; Su et al., 2013).
Successful stormwater governance mechanisms often combine command-and-control regulations with economic instruments to incentivize private property owners to implement effective measures against stormwater runoffs (Bassi et al., 2017). Digital technology, and specifically IoT and blockchain platforms, can be used to facilitate the development of an effective trading and mitigation framework, to help expand access to stormwater credit markets, to identify, measure, and track units of trading like volume and pollution level, and to automate the management of permanent or time-bound credits (Lathrop et al., 2012; Bassi et al., 2017).
IoT enables water systems to execute processes and communicate using a standard interface, orchestrate the management through new coordination applications, and provide tailored information services for a specific water distribution network community (Tadokoro et al., 2011; Robles et al., 2015; Syed et al., 2021). Access to real-time data from sensors and actuators improves the management of water infrastructures and asset utilization, resulting in cost savings (Robles et al., 2015; Napolitano et al., 2021).
Nevertheless, examples of IoT implementation show that it would not eliminate the need for third-party validation and oversight in water systems (Anjana et al., 2015; Perumal et al., 2015; Wadekar et al., 2016; Alabi et al., 2019). A potentially better alternative technology is a distributed ledger blockchain. Considering the novelty of this technology, blockchain is yet to be tested and evaluated in the context of different applications, including urban water management. For instance, Li et al. (2021) recently proposed a data-driven peer-to-peer blockchain to predict water consumption. Effective water consumption forecasts will improve production and supply planning, reduce operating costs, and improve social benefits (Li et al., 2021).
Like any other new technology, different Blockchain applications (e.g., cryptocurrencies, autonomous vehicles, and infrastructure and governance) face different maturity and adoption stages. However, there are common technical, organizational, social, and institutional challenges that any organization should address before it can achieve the objectives and full potential of Blockchain technology (Zhao et al., 2019; Feng et al., 2020; Bagloee et al., 2021). As Bagloee et al. (2021) suggest, the first issue is the scalability of Blockchain networks. As a data-intensive and computationally demanding solution, the requirements for data storage, bandwidth, computational speed, and power highly depend on the number of participating nodes and the quantity of data analyzed.
Cybersecurity is another technical concern. Although the recent incidents of unauthorized penetration to cryptocurrency exchange platforms are rare, the scope of such a threat is yet to be fully understood (Brotsis et al., 2021). Industries willing to adopt this technology need to consider standardization and interoperability across Blockchain platforms, especially if the system requires collaboration between competitors, suppliers, and other stakeholders. Organizations that adopt blockchain should be mindful of the platform's significant electricity use and energy costs due to its power-intensive computing nature. That might put Blockchain participants at odds with sustainability goals. Recent advancements in Blockchain-as-a-service where cloud computing is incorporated might be a solution. Finally, the technological advances have outpaced the sluggish regulation developments. A close collaboration of adopting industry, technology developers, and policymakers can help remove regulatory obstacles to Blockchain adoption (Bagloee et al., 2021).
Once it is decided that blockchain is a suitable and economically viable solution to implement, a distributed ledger system should be designed to improve water management operations, including quality control on water reserves, efficient systemic water management, water leakage detection, water quality, and safety monitoring, transparency of consumption, and prescriptive maintenance on infrastructure (Alabi et al., 2019). In general, the initial investments required for the adoption of blockchain-based water management systems are capital investments in equipment (e.g., computers and blockchain miners, sensors, smart meters, communication devices), service investments (e.g., remote sensing, blockchain mining services, cloud-base decision models), and knowledge and human capital investments (localized knowledge to monitor and manage the system).
The architectural design, then, includes a network of IoT devices embedded within the distribution system to measure water consumption, a public blockchain infrastructure, and smart contracts that represent the interests of different stakeholders and regulate the distribution of incentives amongst different users (see Figure 2). Water managers and end-users at the core of the network who directly benefit from the digitized water distribution system. The direct benefit of IoT and Blockchain in enhancing business processes such ablas accounting, billing, and distribution is discussed extensively (Pincheira et al., 2021). For instance, the cost of recordkeeping can be significantly reduced if real-time data regarding market shares are managed by smart contracts and saved on a Blockchain platform (Poberezhna, 2018). Digitized quality certificates and consumption information would be easily accessible to authorized participants in the blockchain network.
Figure 2. Blockchain-based water management system. The graph is developed by authors using icons embedded in Microsoft Office software.
Participating authorities and regulators can also monitor and audit business practices, transactions, and other financial documents in real-time. Business decisions can be made seamlessly through smart contracts based on predefined rules that govern the blockchain network. Data security is greatly improved compared to conventional centralized systems, and the inherent immutability of blockchain assures data integrity. Access to data can be restricted to authorized participants depending on whether a permission or permissionless architectural design is implemented. In water management systems that promote water savings, permissionless networks are more open and transparent and thus enable any stakeholder to put a value tag on those savings (Pincheira et al., 2021).
There are, however, legal limitations and ethical issues associated with blockchain technology before it reaches mainstream implementation. First, as an immutable and distributed ledger, blockchain does not conform to users' right to rectification and the right to be forgotten imposed by law to data software structures and public platforms (Teeluck et al., 2021). Also, there are problems with smart contracts in terms of conflict and dispute resolution. The legal system yet needs to find a way to assign responsibility in the case of conflict and decide upon the terms and conditions of the contract if disputes arise (Bottoni et al., 2020; Daniel and Ifejika Speranza, 2020; Düdder et al., 2021). In addition, the expansion of blockchain as a resource-intensive power-consuming process should raise concerns about the environmental impacts of the technology (Enescu et al., 2020; Teeluck et al., 2021). Finally, blockchain provides anonymity that could shield criminal activities such as money laundering, human trafficking, and terrorism financing.
Any blockchain application has three general technical and organizational components: the distributed ledger, the governance structure to use the ledger, and the ecosystem (i.e., stakeholders) that form the network (Van Wassenaer et al., 2021). Any attempt to implement blockchain technology at a large scale should succeed in a careful pilot program in which different technologies are tested, considering the role of interoperable blockchain solutions and standardization of information requirements in the water management industry (Sengupta and Kim, 2021). In this regard, it is important to identify critical information and data elements that have value to stakeholders, including end-user in water supply systems (Van Hilten et al., 2020; Sengupta and Kim, 2021). Managing the access to data and authorization criteria can be addressed via permissioned and permissionless blockchain solutions (Van Hilten et al., 2020).
To prepare for adopting blockchain solutions in water management systems, understanding the potential barriers is critical. Most importantly, successful blockchain implementation depends on overcoming intra-organizational barriers such as management's long-term commitment and support, lack of new organizational policies, and lack of knowledge and expertise (Saberi et al., 2019).
Furthermore, differences among partners in terms of organizational culture and information disclosure policies can lead to collaboration, communication, and coordination problems and therefore pose an inter-organizational barrier to adopting blockchain technology (Saberi et al., 2019). Access to technology, smart devices, and IT tools can also be challenging to water system stakeholders. Scalability, data storage, and computational power are system-related barriers that should be addressed before the technology is fully adopted (Ahram et al., 2017; Helmbrecht et al., 2017; Saberi et al., 2019). Entities that are not directly economically benefiting from the adoption of blockchain could pose external barriers. A fair distribution of costs and revenues determined by the association of the stakeholders and regulators would facilitate blockchain technology development via a consortium approach. Blockchain technology features like smart contracts can ensure that cost distribution is according to pre-determined rules, increasing transparency and assurance (Sengupta and Kim, 2021). Finally, a robust mechanism that secures information flow along the water supply system is necessary.
All authors listed have made a substantial, direct, and intellectual contribution to the work and approved it for publication.
This work was supported by the County of San Diego. This manuscript has been released as a report at the University of California Cooperative Extension's Agriculture Water Quality Research and Education Website (Nemati and Asgari, 2021).
The authors declare that the research was conducted in the absence of any commercial or financial relationships that could be construed as a potential conflict of interest.
All claims expressed in this article are solely those of the authors and do not necessarily represent those of their affiliated organizations, or those of the publisher, the editors and the reviewers. Any product that may be evaluated in this article, or claim that may be made by its manufacturer, is not guaranteed or endorsed by the publisher.
The Supplementary Material for this article can be found online at: https://www.frontiersin.org/articles/10.3389/frwa.2022.848686/full#supplementary-material
Abdelhafidh, M., Fourati, M., and Chaari Fourati, L. (2020). A genetic algorithm-based intelligent solution for water pipeline monitoring system in a transient state. Concurrency and Computation: Practice and Experience. doi: 10.1002/cpe.5959
Ahram, T., Sargolzaei, A., Sargolzaei, S., Daniels, J., and Amaba, B. (2017). Blockchain Technology Innovations. IEEE Technology & Engineering Management Conference (TEMSCON). Santa Clara, CA: IEEE. doi: 10.1109/TEMSCON.2017.7998367
Akram, S. V., Malik, P. K., Singh, R., Anita, G., and Tanwar, S. (2020). Adoption of blockchain technology in various realms: Opportunities and challenges. Wiley. doi: 10.1002/spy2.109
Alabi, M. O., Telukdarie, A., Van, N., and Rensburg, J. Industry 4.0: Innovative Solutions for The Water Industry. (2019).
Allen, M., Preis, A., Iqbal, M., and Whittle, A. J. (2012). Case study: a smart water grid in Singapore. Water Pract. Technol. 7. doi: 10.2166/wpt.2012.089
Almetwally, S. A. H., Hassan, M. K., and Mourad, M. H. (2020). Real time internet of things (IoT) based water quality management system. Procedia CIRP. 91, 478–485. doi: 10.1016/j.procir.2020.03.107
Anjana, S., Sahana, M. N., Ankith, S., Natarajan, K., Shobha, K. R., and Paventhan, A. (2015). An IoT based 6LoWPAN enabled Experiment for Water Management. Kolkata, India: IEEE ANTS. doi: 10.1109/ANTS.2015.7413654
Arvind, A., Paul, R., and Bhulania, P. (2020). Implementation of Water Quality Sensing System using Internet of Things. Noida, India: IEEE. doi: 10.1109/SPIN48934.2020.9070832
Bagloee, S. A., Heshmati, M., Dia, H., Ghaderi, H., Pettit, C., and Asadi, M. (2021). Blockchain: the operating system of smart cities. Cities. 112. doi: 10.1016/j.cities.2021.103104
Bahadori, A., and Smith, S. T. (2016). Dictionary of Environmental Engineering and Wastewater Treatment. Cham: Springer. doi: 10.1007/978-3-319-26261-1
Bai, Q., Wu, J., and Jin, C. (2020). The water quality online monitoring system based on wireless sensor network. 2020 13th International Symposium on Computational Intelligence and Design (ISCID). Hangzhou, China: IEEE. doi: 10.1109/ISCID51228.2020.00059
Bassi, A., Cuéllar, A., Pallaske, G., and Wuennenberg, L. (2017). Stormwater Markets: Concepts and Applications. Winnipeg: International Institute for Sustainable Development.
Bassoo, V., Ramnarain-Seetohul, V., Hurbungs, V., Fowdur, T. P., and Beeharry, Y. (2018). Big Data Analytics for Smart Cities. Internet of Things and Big Data Analytics Toward Next-Generation Intelligence. Cham: Springer. doi: 10.1007/978-3-319-60435-0_15
Bottoni, P., Gessa, N., Massa, G., Pareschi, R., Selim, H., and Arcuri, E. (2020). Intelligent smart contracts for innovative supply chain management. Front. Blockchain. 3. doi: 10.3389/fbloc.2020.535787
Brotsis, S., Limniotis, K., Bendiab, G., Kolokotronis, N., and Shiaeles, S. (2021). On the suitability of blockchain platforms for IoT applications: Architectures, security, privacy, and performance. Journal of Computer Networks. doi: 10.1016/j.comnet.2021.108005
Caragliu, A., Del Bo, C., and Nijkamp, P. (2011). Smart cities in Europe. J. Urban Technol. 18, 65–82. doi: 10.1080/10630732.2011.601117
Chourabi, H., Nam, T., Walker, S., Gil-Garcia, J. R., Mellouli, S., Nahon, K., et al. (2012). Understanding smart cities: An integrative framework. 45th Hawaii International Conference on System Sciences. Maui, HI: IEEE Computer Society. 2289–2297. doi: 10.1109/HICSS.2012.615
Chowdury, M. S. U., Emran, T. B., Ghosh, S., Pathak, A., Alam, M. M., Absar, N., et al. (2019). IoT based real-time river water quality monitoring system. Procedia Comp. Sci. 155, 161–168. doi: 10.1016/j.procs.2019.08.025
Christidis, K., and Devetsikiotis, M. (2016). Blockchains and Smart Contracts for the Internet of Things. Institute of Electrical and Electronics Engineers Inc. doi: 10.1109/ACCESS.2016.2566339
Cianchi, P., Marsili-Libelli, S., Burchi, A., and Burchielli, S. (2000). Integrated river quality management using internet technologies. Gent, Belgium: WATERMATEX.
Civic Ledger (2020). Improving Water Markets through New Digital Technologies. Townsville, QLD: Cooperative Research Centre for Developing Northern Australia Ltd.
D'amico, G., Taddeo, R., Shi, L., Yigitcanlar, T., and Ioppolo, G. (2020). Ecological indicators of smart urban metabolism: a review of the literature on international standards. Ecol. Indic. 118. doi: 10.1016/j.ecolind.2020.106808
Daniel, D., and Ifejika Speranza, C. (2020). The role of blockchain in documenting land users' rights: the canonical case of farmers in the vernacular land market. Front. Blockchain. 3. doi: 10.3389/fbloc.2020.00019
Das, B., and Jain, P. C. (2017). Real-Time Water Quality Monitoring System using Internet of Things. Jaipur, Malaviya: International Conference on Computer, Communications and Electronics (Comptelix). doi: 10.1109/COMPTELIX.2017.8003942
Dogo, E. M., Nwulu, N. I., Twala, B., and Aigbavboa, C. (2019a). A survey of machine learning methods applied to anomaly detection on drinking-water quality data. Urban Water J. 16, 235–248. doi: 10.1080/1573062X.2019.1637002
Dogo, E. M., Salami, A. F., Aigbavboa, C. O., and Nkonyana, T. (2019b). Taking Cloud Computing to the Extreme Edge: A Review of Mist Computing for Smart Cities and Industry 4.0 in Africa. Cham: Springer. doi: 10.1007/978-3-319-99061-3_7
Dogo, E. M., Salami, A. F., Nwulu, N. I., and Aigbavboa, C. O. (2019c). “Blockchain and Internet of Things-Based Technologies for Intelligent Water Management System”, in: Artificial Intelligence in IoT, Al-Turjman, F. (ed.). Cham: Springer International Publishing. doi: 10.1007/978-3-030-04110-6_7
Düdder, B., Fomin, V., Gürpinar, T., Henke, M., Iqbal, M., Janavičiene, V., Matulevičius, R., Straub, N., and Wu, H. (2021). Interdisciplinary blockchain education: utilizing blockchain technology from various perspectives. Front. Blockchain. 3. doi: 10.3389/fbloc.2020.578022
Eggimann, S., Mutzner, L., Wani, O., Schneider, M. Y., Spuhler, D., Moy De Vitry, M., et al. (2017). The potential of knowing more: a review of data-driven urban water management. Environ. Sci. Technol. 51, 2538–2553. doi: 10.1021/acs.est.6b04267
Enescu, F. M., Bizon, N., Onu, A., Răboacă, M. S., Thounthong, P., Mazare, A. G, et al. (2020). Implementing blockchain technology in irrigation systems that integrate photovoltaic energy generation systems. Sustainability. 12, 1540. doi: 10.3390/su12041540
Feng, H., Wang, X., Duan, Y., Zhang, J., and Zhang, X. (2020). Applying blockchain technology to improve agri-food traceability: A review of development methods, benefits and challenges. J Cleaner Product. 260, 121031. doi: 10.1016/j.jclepro.2020.121031
Glasgow, H. B., Burkholder, J. M., Reed, R. E., Lewitus, A. J., and Kleinman, J. E. (2004). Real-time remote monitoring of water quality: a review of current applications, and advancements in sensor, telemetry, and computing technologies. J. Exp. Mar. Biol. Ecol. 300, 409-448. doi: 10.1016/j.jembe.2004.02.022
Guan, M., Liang, Q., and Hou, J. (2021). Editorial: smart approaches to predict urban flooding: current advances and challenges. Front. Earth Sci. 9. doi: 10.3389/feart.2021.681751
Helmbrecht, J., Pastor, J., and Moya, C. (2017). Smart solution to improve water-energy nexus for water supply systems. Procedia Eng. 186, 101–109. doi: 10.1016/j.proeng.2017.03.215
Hoos, A. B., Wang, S. H., and Schwarz, G. E. (2019). Adapting a regional water-quality model for local application: a case study for Tennessee, USA. Environ Model Softw. 115, 187–199. doi: 10.1016/j.envsoft.2019.01.001
Ighalo, J. O., and Adeniyi, A. G. (2020). A comprehensive review of water quality monitoring and assessment in Nigeria. Chemosphere. 260, 127569. doi: 10.1016/j.chemosphere.2020.127569
Ighalo, J. O., Adeniyi, A. G., and Marques, G. (2021). Internet of Things for Water Quality Monitoring and Assessment: A Comprehensive Review. Artificial Intelligence for Sustainable Development: Theory, Practice and Future Applications. Cham: Springer. doi: 10.1007/978-3-030-51920-9_13
Kabir, S., Patidar, S., Xia, X., Liang, Q., Neal, J., and Pender, G. (2020). A deep convolutional neural network model for rapid prediction of fluvial flood inundation. J Hydrol. 590. doi: 10.1016/j.jhydrol.2020.125481
Kamaludin, K. H., and Ismail, W. (2017). Water Quality Monitoring with Internet of Things (IoT). Melaka, Malaysia: IEEE Conference on Systems, Process and Control (ICSPC 2017). doi: 10.1109/SPC.2017.8313015
Kumar Jha, M., Kumari Sah, R., Rashmitha, M. S., Sinha, R., Sujatha, B., and Suma, K. V. (2018). Smart Water Monitoring System for Real-time Water Quality and Usage Monitoring. International Conference on Inventive Research in Computing Applications. IEEE. doi: 10.1109/ICIRCA.2018.8597179
Kumar, D. S., Askarunisa, A., and Kumar, R. M. (2020). Embedded processor based automated assessment of quality of the water in an IoT background. Microprocess. Microsyst. 77, 103167. doi: 10.1016/j.micpro.2020.103167
Kumar, N. M., and Mallick, P. K. (2018). Blockchain technology for security issues and challenges in IoT. Procedia Computer Sci. 132, 1815–1823. doi: 10.1016/j.procs.2018.05.140
Lathrop, R. G., Auermuller, L., Haag, S., and Im, W. (2012). The stormwater management and planning tool: coastal water quality enhancement through the use of an internet-based geospatial tool. Coastal Manag. 40, 339–354. doi: 10.1080/08920753.2012.692309
Lavanya, N., and Raghavendra Babu, T. M. (2019). A survey on smart water quality monitoring system based on IoT. Int. J. Manag. Sci. 2, 153–156.
Le Jallé, C., Désille, D., and Burkhardt, G. (2013). Urban Stormwater Management in Developing Countries. NOVATECH.
Lee, S. W., Sarp, S., Jeon, D. J., and Kim, J. H. (2014). Smart water grid: the future water management platform. Desalin. Water Treat. 55, 339–346. doi: 10.1080/19443994.2014.917887
Li, H., Chen, X., Guo, Z., Xu, J., Shen, Y., and Gao, X. (2021). Data-driven peer-to-peer blockchain framework for water consumption management. Peer-to-Peer Networking and Applications. 14, 2887–2900. doi: 10.1007/s12083-021-01121-6
Li, J., Yang, X., and Sitzenfrei, R. (2020). Rethinking the framework of smart water system: a review. Water. 12. doi: 10.3390/w12020412
Lin, Y.-P., Petway, J., Lien, W.-Y., and Settele, J. (2018). Blockchain with artificial intelligence to efficiently manage water use under climate change. Environments. 5, 1–2. doi: 10.3390/environments5030034
Marchese, D., and Linkov, I. (2017). Can you be smart and resilient at the same time? Environ. Sci. Technol. 51, 5867–5868. doi: 10.1021/acs.est.7b01912
Mcphillips, L. E., and Matsler, A. M. (2018). Temporal evolution of green stormwater infrastructure strategies in three US cities. Front. Built Environ. 4. doi: 10.3389/fbuil.2018.00026
Meshal, A., Mikhael, M. N., and Mansour, H. A. (2020). Water quality monitoring system of the Nile River. Eng. Res. J. (ERJ). 1, 100–104.
Mutchek, M., and Williams, E. (2014). Moving towards sustainable and resilient smart water grids. Challenges. 5, 123–137. doi: 10.3390/challe5010123
Nakamoto, S. (2008). Bitcoin: A Peer-to-Peer Electronic Cash System. Available online at: www.bitcoin.org; https://www.debr.io/article/21260-bitcoin-a-peer-to-peer-electronic-cash-system.
Napolitano, R., Reinhart, W., and Gevaudan, J. P. (2021). Smart cities built with smart materials. Sci. 371, 1200–1201. doi: 10.1126/science.abg4254
Nemati, M., and Asgari, M. (2021). Opportunities to Use Technological Advancements for Water Supply Management: Implications of Blockchain Technology. County of San Diego.
Nie, X., Fan, T., Wang, B., Li, Z., Shankar, A., and Manickam, A. (2020). Big data analytics and IoT in operation safety management in under water management. Computer Commun. 154, 188–196. doi: 10.1016/j.comcom.2020.02.052
Pahonṭu, B., Arsene, D., Predescu, A., and Mocanu, M. (2020). Application and Challenges of Blockchain Technology For Real-Time Operation In A Water Distribution System. 24th International Conference on System Theory, Control and Computing. doi: 10.1109/ICSTCC50638.2020.9259732
Perumal, T., Sulaiman, M. N., and Leong, C. Y. (2015). Internet of Things (IoT) enabled water monitoring system. IEEE 4th Global Conference on Consumer Electronics, 2 2016. https://www.google.com/search? client=firefox-b-d&q=Piscataway&stick=H4sIAAAAAAAAAOPgE-LQz9U3S CvIylICsyoKCou0tLKTrfTzi9IT8zKrEksy8_NQOFYZqYkphaWJRSWpRcWL WLkCMouTE0sSyxMrd7Ay7mJn4mAAAGgbwytXAAAA&sa=X&ved=2ahU KEwiy-raw2tb2AhVCmHIEHRYrDOAQmxMoAHoECCoQAg Piscataway, NJ: Institute of Electrical and Electronics Engineers Inc. p. 86–87. doi: 10.1109/GCCE.2015.7398710
Pincheira, M., Vecchio, M., Giaffreda, R., and Kanhere, S. S. (2021). Cost-effective IoT devices as trustworthy data sources for a blockchain-based water management system in precision agriculture. Comput. Electron. Agric. 180. doi: 10.1016/j.compag.2020.105889
Poberezhna, A. (2018). “Addressing Water Sustainability With Blockchain Technology and Green Finance,” in Transforming Climate Finance and Green Investment with Blockchains, Marke, A. (ed.). Amsterdam, Netherlands: Elsevier Inc. doi: 10.1016/B978-0-12-814447-3.00014-8
Public Utilities Board Singapore. (2016). Managing the water distribution network with a Smart Water Grid. Smart Water. 1, 4. doi: 10.1186/s40713-016-0004-4
Qatawneh, M., Almobaideen, W., and Abualghanam, O. (2020). Challenges of Blockchain Technology in Context Internet of Things: A Survey. doi: 10.5120/ijca2020920660
Reyna, A., Martín, C., Chen, J., Soler, E., and Díaz, M. (2018). On blockchain and its integration with IoT. Challenges and opportunities. Future Gener. Comput. Syst. 88, 173–190. doi: 10.1016/j.future.2018.05.046
Robles, T., Alcarria, R., Martín, D., Navarro, M., Calero, R., Iglesias, S., and López, M. (2015). An IoT based reference architecture for smart water management processes. J Wirel Mob Netw Ubiquitous. 6, 4–23.
Rodriguez-Perez, J., Leigh, C., Liquet, B., Kermorvant, C., Peterson, E., Sous, D., et al. (2020). Detecting technical anomalies in high-frequency water-quality data using artificial neural networks. Environ Sci Technol. 54:13719–30. doi: 10.1021/acs.est.0c04069
Rojek, I., and Studzinski, J. (2019). Detection and localization of water leaks in water nets supported by an ICT system with artificial intelligence methods as a way forward for smart cities. Sustainability. 11, 518. doi: 10.3390/su11020518
Rose, K., Eldridge, S., and Chapin, L. (2015). The Internet of Things: An Overview - Understanding the Issues and Challenges of a More Connected World. Internet Society. Available online at: https://www.internetsociety.org/
Saberi, S., Kouhizadeh, M., Sarkis, J., and Shen, L. (2019). Blockchain technology and its relationships to sustainable supply chain management. Int. J. Prod. Res. 57, 2117–2135. doi: 10.1080/00207543.2018.1533261
Saravanan, K., Anusuya, E., Kumar, R., and Son, L. H. (2018). Real-time water quality monitoring using Internet of Things in SCADA. Environ Monit Assess. 190, 556. doi: 10.1007/s10661-018-6914-x
Sarraf, R., Ojha, S., Biraris, D., and Bhangale, K. B. (2020). IoT based smart quality water management system. Int. J. Adv. Sci. Res. Eng. Trends 5, 12-16.
Sengupta, U., and Kim, H. M. (2021). Meeting changing customer requirements in food and agriculture through the application of blockchain technology. Front. Blockchain. 4. doi: 10.3389/fbloc.2021.613346
Shahid, A., Khalid, B., Shaukat, S., Ali, H., and Qadri, M. Y. (2018). Internet of Things Shaping Smart Cities: A Survey. Internet of Things and Big Data Analytics Toward Next-Generation Intelligence. doi: 10.1007/978-3-319-60435-0_14
Shanthi, S., Gopi, T., and Vidhyesh, C. (2019). IoT Based Water and Air Quality Monitoring System and Analysis. Int. J. Adv. Sci. Res. 14, 1405–1409.
Shi, B., Wang, P., Jiang, J., and Liu, R. (2018). Applying high-frequency surrogate measurements and a wavelet-ANN model to provide early warnings of rapid surface water quality anomalies. Sci. Total Environ. 610, 1390–1399. doi: 10.1016/j.scitotenv.2017.08.232
Shilpi, and Ahad, M. (2020). Blockchain technology and smart cities - a review. EAI Endorsed Trans. Energy Web. 4. doi: 10.4108/eai.13-7-2018.163846
Sithole, M. P. P., Nwulu, N. I., and Dogo, E. M. (2019a). Dataset for a wireless sensor network based drinking-water quality monitoring and notification system. Data in Brief. 27. doi: 10.1016/j.dib.2019.104813
Sithole, M. P. P., Nwulu, N. I., and Dogo, E. M. (2019b). Development of a wireless sensor network based water quality monitoring and notification system. Malatya, Turkey: 2019 International Artificial Intelligence and Data Processing Symposium (IDAP), 21-22 Sept. doi: 10.1109/IDAP.2019.8875987
Spandana, K., and Seshagiri Rao, V. R. (2018). Internet of Things (Iot) Based Smart Water Quality Monitoring System. Int. J. Eng. Technol. doi: 10.14419/ijet.v7i3.6.14985
Sriyono, E. (2020). Digitizing water management: toward the innovative use of blockchain technologies to address sustainability. Cogent Eng. 7. doi: 10.1080/23311916.2020.1769366
Su, X., Shao, G., Vause, J., and Tang, L. (2013). An integrated system for urban environmental monitoring and management based on the Environmental Internet of Things. Int. J. Sustain. Dev. World Ecol. 20, 205–209. doi: 10.1080/13504509.2013.782580
Syed, A. S., Sierra-Sosa, D., Kumar, A., and Elmaghraby, A. (2021). IoT in smart cities: a survey of technologies, practices and challenges. Smart Cities. 4, 429–475. doi: 10.3390/smartcities4020024
Tadokoro, H., Onishi, M., Kageyama, K., Kurisu, H., and Takahashi, S. (2011). Smart Water Management and Usage Systems for Society and Environment. Hitachi Review.
Teeluck, R., Durjan, S., and Bassoo, V. (2021). Blockchain Technology and Emerging Communications Applications. Security and Privacy Applications for Smart City Development. Cham: Springer. doi: 10.1007/978-3-030-53149-2_11
Tinelli, S., and Juran, I. (2019). Artificial intelligence-based monitoring system of water quality parameters for early detection of non-specific bio-contamination in water distribution systems. Water Supply. 19, 1785–1792. doi: 10.2166/ws.2019.057
Tripathy, A. K., Das, T. K., and Chowdhary, C. L. (2020). “Monitoring Quality of Tap Water in Cities Using IoT,” in Emerging Technologies for Agriculture and Environment, Subramanian, B., Chen, S.-S., and Reddy, K. R. (eds.). Singapore: Springer. doi: 10.1007/978-981-13-7968-0_8
Tsague, H. D., and Twala, B. (2018). Practical Techniques for Securing the Internet of Things (IoT) Against Side Channel Attacks. Internet of Things and Big Data Analytics Toward Next-Generation Intelligence. doi: 10.1007/978-3-319-60435-0_18
Van Hilten, M., Ongena, G., and Ravesteijn, P. (2020). Blockchain for organic food traceability: case studies on drivers and challenges. Front. Blockchain. 3. doi: 10.3389/fbloc.2020.567175
Van Wassenaer, L., Verdouw, C., and Wolfert, S. (2021). What blockchain are we talking about? An analytical framework for understanding blockchain applications in agriculture and food. Front. Blockchain. 4. doi: 10.3389/fbloc.2021.653128
Wadekar, S., Vakare, V., Prajapati, R., Yadav, S., and Yadav, V. (2016). Smart Water Management Using IOT. IEEE Access. doi: 10.1109/WECON.2016.7993425
Wang, S., Zhang, Z., Ye, Z., Wang, X., Lin, X., and Chen, S. (2013). Application of environmental internet of things on water quality management of urban scenic river. Int. J. Sustain. Dev. World Ecol. 20, 216–222. doi: 10.1080/13504509.2013.785040
Wiliem, L., Yarlagadda, P. K. D. V., and Zhou, S. (2006). Development of internet based real-time water condition monitoring system. Lulea, Sweden: 19th International Congress and Exhibition on Condition Monitoring and Diagnostic Engineering Management (COMADEM 2006) p. 119–129.
Wu, D., Wang, H., and Seidu, R. (2020). Smart data driven quality prediction for urban water source management. Future Gener. Comput. Syst. 107, 418–432. doi: 10.1016/j.future.2020.02.022
Xiang, X., Li, Q., Khan, S., and Khalaf, O. I. (2021). Urban water resource management for sustainable environment planning using artificial intelligence techniques. Environ. Impact Assess. 86. doi: 10.1016/j.eiar.2020.106515
Yang, J., Li, Y., Zhang, N. F., Yang, J. F., Kuang, K., Hu, Y. H., and Qi, W. G. (2015). Analysis of Urban Residential Water Consumption Based on Smart Meters and Fuzzy Clustering. 2015 IEEE International Conference on Computer and Information Technology; Ubiquitous Computing and Communications; Dependable, Autonomic and Secure Computing; Pervasive Intelligence and Computing. doi: 10.1109/CIT/IUCC/DASC/PICOM.2015.192
Yazdi, M. N., Sample, D. J., Scott, D., Owen, J. S., Ketabchy, M., and Alamdari, N. (2019). Water quality characterization of storm and irrigation runoff from a container nursery. Sci Total Environ, 667, 166–178. doi: 10.1016/j.scitotenv.2019.02.326
Zhao, G., Liu, S., Lopez, C., Lu, H., Elgueta, S., Chen, H., and Boshkoska, B. M. (2019). Blockchain technology in agri-food value chain management: A synthesis of applications, challenges and future research directions. Comp. Indust. 109, 83–99. doi: 10.1016/j.compind.2019.04.002
Keywords: blockchain, IoT, water quality, stormwater, urban, smart cities
Citation: Asgari M and Nemati M (2022) Application of Distributed Ledger Platforms in Smart Water Systems—A Literature Review. Front. Water 4:848686. doi: 10.3389/frwa.2022.848686
Received: 04 January 2022; Accepted: 18 March 2022;
Published: 07 April 2022.
Edited by:
Yizi Shang, China Institute of Water Resources and Hydropower Research, ChinaReviewed by:
Katarzyna Pietrucha-Urbanik, Rzeszów University of Technology, PolandCopyright © 2022 Asgari and Nemati. This is an open-access article distributed under the terms of the Creative Commons Attribution License (CC BY). The use, distribution or reproduction in other forums is permitted, provided the original author(s) and the copyright owner(s) are credited and that the original publication in this journal is cited, in accordance with accepted academic practice. No use, distribution or reproduction is permitted which does not comply with these terms.
*Correspondence: Mehdi Nemati, bWVoZGluQHVjci5lZHU=
Disclaimer: All claims expressed in this article are solely those of the authors and do not necessarily represent those of their affiliated organizations, or those of the publisher, the editors and the reviewers. Any product that may be evaluated in this article or claim that may be made by its manufacturer is not guaranteed or endorsed by the publisher.
Research integrity at Frontiers
Learn more about the work of our research integrity team to safeguard the quality of each article we publish.