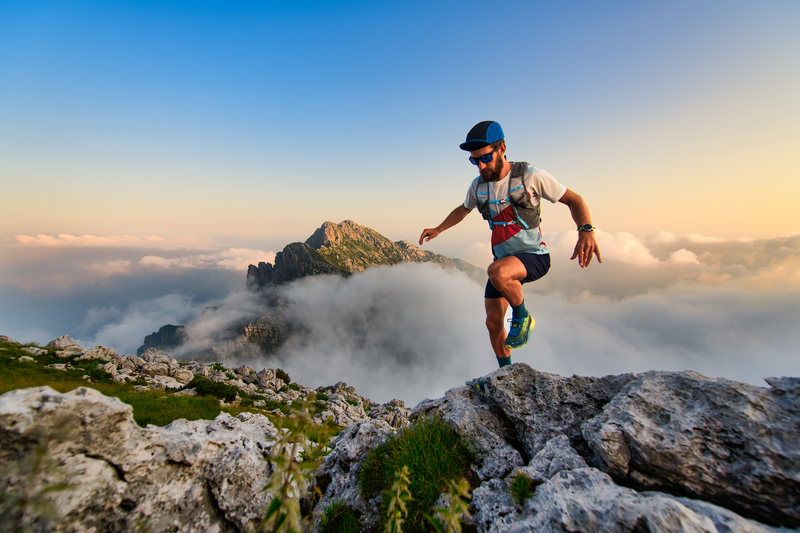
94% of researchers rate our articles as excellent or good
Learn more about the work of our research integrity team to safeguard the quality of each article we publish.
Find out more
ORIGINAL RESEARCH article
Front. Water , 22 February 2022
Sec. Environmental Water Quality
Volume 4 - 2022 | https://doi.org/10.3389/frwa.2022.832297
This article is part of the Research Topic Decentralized Wastewater Treatment and Resources Recovery View all 4 articles
Biofiltration processes help to remove trace organic chemicals (TOrCs) both in wastewater and drinking water treatment systems. However, the detailed TOrCs biotransformation mechanisms as well as the underlying drivers behind the variability of site specific transformation processes remain elusive. In this study, we used laboratory batch incubations to investigate the biotransformation of 51 TOrCs in eight bioactive filter materials of different origins treating a range of waters, from wastewater effluents to drinking water. Microscopy, 16S rRNA amplicon and whole metagenome sequencing for assessing associations between the biotransformation rate constants, microbial composition and genetic potential complemented chemical analysis. We observed strong differences in the mean global removal of TOrCs between the individual sand filters (−1.4–58%), which were mirrored in overall biomass, microbial community composition, and enzyme encoding genes. From the six investigated biomass markers, ATP turned out to be a major predictor of the mean global biotransformation rate, while compound specific biotransformations were correlated with the microbial community composition. High biomass ecosystems were indicated in our systems by a dominance of Nitrospirae, but individual TOrC biotransformation showed a correlation with rare taxa (<2%) such as Hydrogenophaga, or individual functions such as the enoyl-CoA hydratase/3-hydroxyacyl-CoA dehydrogenase encoding genes. In general, this study provides new insights into so far rarely addressed variability of TOrCs biotransformation. We propose potential novel biological indicators for the removal performance of TOrCs in biofiltration systems, highlighting the role of living biomass in predicting and normalizing the global transformation, and the role of the microbial community for the individual transformation of TOrCs in engineered and natural systems.
Trace organic chemicals (TOrCs) such as pharmaceuticals, personal care products and pesticides, have raised emerging concerns regarding their effects on the aquatic environment. These anthropogenic compounds usually enter the wastewater system, and may finally end up in the receiving water bodies, leading to their frequent detection in surface water, ground water and even drinking water at the concentration ranging from few ng·L−1 to several μg·L−1 (Yang et al., 2017; Montiel-León et al., 2018; Tröger et al., 2018). For example, the widely used artificial sweetener acesulfame has been detected worldwide in wastewater treatment plants (WWTPs) at 10–100 μg·L−1, and consequently, appears in rivers and groundwater with concentrations up to the double-digit μg·L−1 range (Castronovo et al., 2017). The effects of residual TOrCs on aquatic ecosystems and the human health have been studied in recent years (Vieno and Sillanpää, 2014; Yang et al., 2017; Shao et al., 2019).
In general, WWTPs provide the initial opportunity for removing TOrCs and preventing significant environmental exposure. However, conventional wastewater treatment processes are not originally designed to eliminate diverging TOrCs (Grandclément et al., 2017). WWTPs are an important source for the entry of TOrCs; therefore, engineering solutions to improve the removal of TOrCs are needed. Recently, advanced physico-chemical treatment options such as ozonation and activated carbon have been developed and applied at full scale (Kosek et al., 2020). In addition, membrane filtration options and advance oxidation processes are also able to efficiently remove TOrCs but are cost intensive (Kanaujiya et al., 2019). Conventional activated sludge (CAS) reduces the overall load of micropollutants by both sorption and biodegradation processes but many compounds are only partially removed or persistent (Fischer and Majewsky, 2014). It has been shown that some of these more persistent compounds are well-degraded in subsequent biofiltration systems (Paredes et al., 2016; Devault et al., 2021). For instance, the removal efficiencies of diclofenac by activated sludge treatment are often below 30% (Zhang et al., 2008), while in slow sand filtration systems the removal rate was 40 to almost 80% (Matamoros et al., 2007; Casas et al., 2015).
Recent studies have focused on the influences of various operation parameters to optimize TOrCs removal performance in biofiltration. So far, especially redox conditions (Oberleitner et al., 2020), carbon and nitrogen availability (Moe and Irvine, 2001; Zhang et al., 2019), hydraulic retention time (Priya and Philip, 2015), and the filter material (Paredes et al., 2016) have been reported to affect the efficiency of different biofiltration systems. These findings have contributed to our understanding of micropollutant biotransformation in engineered systems. However, all of the aforementioned operating conditions did not have a direct impact, they in fact affect the microbial communities and thereby the diversity, abundance and function of microorganisms.
In general, TOrCs at such low concentrations are not sufficient to serve as a sole carbon and energy source for microbial growth, and some studies raised the co-metabolism mechanisms to validate the high potential of microbial communities biotransforming TOrCs (Su et al., 2015; Shen et al., 2020).
To date, the identification of relevant species and enzymes at environmental TOrCs concentrations remains elusive. Therefore, to uncover the microbial “black box,” additional studies regarding the associations between the abundance of taxa, enzymes, pathways and TOrCs biotransformation are required. Johnson et al. (2015) for example, investigated the taxonomic biodiversity and biotransformation rates of 10 micropollutants in 10 full-scale WWTPs, and found biodiversity was positively associated with the collective removal rates of TOrCs. To explore the biodiversity in more detail, Wolff et al. (2018) divided the general microbial community into a core and a specialized community based on defined filter criteria and statistical selection, comparing their composition with micropollutant removal in five different biological wastewater treatment systems. The results demonstrated the significant correlation between the relative abundance of specialized community members and the removal rates of certain compounds. For example, the abundances of the genera Luteimonas, Roseibaca, and Phenylobacterium might be indicative for the degradation of metoprolol, 10,11-dihydro-10-hydrocarbamazepine and diclofenac under aerobic condition. Functional enzymes and degradation pathways are often studied in pure culture or individual compound biodegradation studies (Kjeldal et al., 2019), as the enzymatic reactions involving diverse microorganisms are quite intricate, making it hard to interpret the degradation pathways (Achermann et al., 2018; Zumstein and Helbling, 2019).
Despite these initial studies, the biotransformation mechanisms of various TOrCs in biological filtration systems on the microbial community level are rarely addressed so far, the majority of studies focused on their removal efficiencies and influencing factors from engineered aspects (Zearley and Summers, 2012; Carpenter and Helbling, 2017; Müller et al., 2017). To appropriately implement strategies for the stimulation of TOrCs removal in biofiltration systems, a better understanding of removal mechanisms, relevant microbes, and enzymes is needed.
In this study, biotransformation performance of eight different biological active sand filter materials from wastewater and water treatment plants for transforming 51 polar TOrCs were investigated experimentally. We coupled biotransformation rates with multiple microbial parameters, which involved the detailed analysis of degrading microorganisms, their functional genes and transformation pathways. The aim of this study is: (1) to assess and compare the transformation efficiencies of different types of biological active systems in a fully controlled laboratory setup, (2) to evaluate the influence of the microbial community composition on the biotransformations, (3) to suggest novel biological indicators for a more comprehensive evaluation of individual or global TOrCs removal efficiencies. For this purpose, we put the eight sand filters under strictly controlled laboratory conditions, thereby excluding any influence of the above-mentioned parameters (redox conditions, carbon and nitrogen availability, hydraulic retention time) on differences in biotransformation rates. Hence, we focused on the transformation potential of the microbial communities at identical conditions, which allowed us to directly link biotransformation rates with taxa, functional genes and biomass markers. We hypothesize that differences in biotransformation rates will be mirrored by the (a) microbial community, and their (b) enzymatic repertoire encoded in the metagenome. Moreover, we evaluated which role biomass plays in the normalization of global transformation performance. This may lead to the identification of biological parameters for the removal of TOrCs, individually or globally.
Fifty-one polar TOrCs which have been typically found in municipal wastewater were selected as target compounds in this study (Table 1), including pesticides, pharmaceuticals, and personal care products with different biodegradabilities in CAS treatment. For example, acyclovir, atenolol, caffeine, and ibuprofen can be relatively easily degraded (Prasse et al., 2011; Ferrando-Climent et al., 2012; Xu et al., 2017a; Chtourou et al., 2018), while carbamazepine and diclofenac are persistent and difficult to be biotransformed (Zhang et al., 2008; Kruglova et al., 2014), and some compounds such as emtricitabine and cetirizin have only been rarely studied so far. All TOrCs reference standards used were of analytical grade (Sigma-Aldrich) with a minimal purity of 98%.
Table 1. Names, usage and abbreviations of the 51 TOrCs analyzed in this study and the statistical differences of their kbiol after normalization with ATP.
Six different sand materials from rapid sand filters of municipal WWTPs and two materials from slow sand filters used for water treatment were sampled and then stored at 4°C for ~3 months. The batch experiments were performed in triplicates with all eight sand filters under the same conditions: 20 g of sand material was added to each 250 mL bottle and 80 mL of treated wastewater from the WWTP Koblenz. All batches were aerated with 7 mg·L−1 oxygen and continuously shaken at 22°C and the speed of 100 rpm in the dark. To acclimate the microorganisms, all batches were equilibrated to the experimental conditions for ~24 h before the experiment started. The experiment was started by spiking the bottles with a mixture of 51 TOrCs at the initial concentration of 0.5 μg·L−1 for each compound, which was within the range of TOrCs concentration in actual wastewater (Luo et al., 2014).
Over 72 h incubation, water samples were withdrawn from each bottle at regular intervals resulting in eight time points (0, 1, 4, 8, 12, 24, 48, 72 h) for kbiol calculation (see below). Samples were immediately filtered using 0.45 μm regenerated cellulose membrane (Macherey-Nagel, Germany) and stored at 4°C for maximum 2 weeks until liquid chromatography tandem mass spectrometry (LC-MS/MS) analysis. At the end of the experiment, the remaining water was removed and the sand materials were collected and stored at −80°C for DNA extraction. In addition, each sand material was fixed 1:1 with ethanol for fluorescence in situ hybridization (FISH) analysis.
In contrast to the DNA based analysis and the monitoring of the compound removal (see below), the biomass measurements and the cell counting was performed as one measurement per filter material (n = 8). Loss on ignition (LOI, a measure for total organic matter content) was determined by drying 15 g of sand filter material at 105°C overnight, and then placing in a muffle furnace at 440°C for 4 h, weighing the samples at each step. ATP from 1 g sand filter material was measured by the single tube luminometer Sirius FB12 (Titertek Berthold, Germany) using the ATP Biomass Kit HS (BioThema, Sweden) according to the manufacturer's instructions with eight measurement points prior and after ATP standard addition. The sand samples were sent to Vermicon AG (Munich, Germany) for cell count measurement and FISH analysis. FISH counting was performed with the sand samples using fluorescence microscopy for following groups: Alphaproteobacteria, Betaproteobacteria, Gammaproteobacteria, Deltaproteobacteria, Epsilonproteobacteria, Actinobacteria, Firmicutes, Cytophaga-Flexibacer-Subphylum, Planctomycetes, Chloroflexi, Nitrospirae, TM7, Archaea. Probe sequences and hybridization conditions were taken from Daims et al. (2006). FISH protocol was performed following Snaidr et al. (1997) with following modifications for sample preparation: 10 g of sand samples were thoroughly vortexed for 5 min. After settlement of the samples at room temperature 200 μL of supernatant were transferred into a 2 mL vial and mixed with 1 mL of PBS buffer. Samples were vortexed, followed by centrifugation at 5,000 rpm for 1 min. Supernatant was extracted and transferred into new 2 ml vial, followed by centrifugation at 5,000 rpm for 5 min. Supernatant was discarded and remaining pellet was solved in 200 μL of 50% EtOH. In situ hybridizations were performed on the EtOH-fixed samples using the gene probe technology VIT® (vermicon identification technology) by Vermicon AG (Munich, Germany). At least 10 fields of view were counted per measurement using a Zeiss Axioscop 2 epifluorescence microscope equipped with fluorescence filter sets for the dyes FAM, Cy3, and 4,6-Diamidino-2-phenylindol-dihydrochloride (DAPI). More information can be obtained by request from Vermicon AG (Munich, Germany).
To determine the concentrations of the 51 spiked TOrCs, all filtered samples of the eight time points were used for LC-MS/MS analysis via an Agilent 1260-LC coupled to a SCIEX QTrap 5500-MS according to the method previously described by Falås et al. (2016). Along the sampled 72 h, biotransformation rate constants were estimated assuming first-order kinetics and negligible sorption according to Equation (1), whereby S is the TOrCs concentration (μg·L−1), XBiomass is the biomass concentration represented by the ATP (pmol·g−1), and kbiol is the normalized pseudo-first-order rate constant in g·(pmol·d)−1.
The DNA of the microorganisms was extracted from the sand filter material, and was used to analyze the present taxa by 16S rRNA amplicon sequencing, and by whole genome shotgun sequencing. Approximately 1.5 g of the frozen filter material from the end of the experiment (24 samples) was used for DNA extraction using the FastDNA SPIN Kit for soil (MP Biomedicals, Eschwege, Germany) according to the manufacturer's instructions. Concentrations and purity of the individual DNA extracts were measured by microspectrophotometry (NanoPhotometer P330, Implen). 16S rRNA gene amplification, library preparation and sequencing were performed by IMGM Laboratories GmbH (Planegg, Germany). In short, primer pair 341F (CCTACGGGNGGCWGCAG) and 805R (GACTACHVGGGTATCTAATCC) for V3-V4 hypervariable region was used for amplification of the 16S gene. Next, the purified PCR products were normalized to equimolar concentration with the SequalPrep Normalization Plate Kit (Thermo Fisher Scientific) and the DNA library was sequenced on the Illumina MiSeq next generation sequencing system (Illumina Inc.) in paired end mode with 2 × 250 bp. Whole metagenome sequencing was performed by IMGM Laboratories GmbH (Planegg, Germany). In brief, the extracted DNA samples were checked for quality and quantity by a 1% agarose gel (Midori Green-stained) electrophoresis and a Qubit dsDNA HS Assay Kit (Invitrogen). Prior to library preparation, the high molecular weight gDNA was sheared to target fragment size of 550 bp using a Covaris M220 Focused-ultrasonicator (Brighton, United Kingdom). In the following, DNA was prepared for sequencing using the NEBnext UltraTM II DNA library preparation kit for Illumina (New England Biolabs). Sequence data were generated on the Illumina HiSeq 2500 next generation sequencing system with the 2 × 250 bp paired end mode.
All 16S rRNA gene amplicons data were processed in R (v 3.6.0) (R Core Team, 2016) using the DADA2 (v 1.9.0) pipeline (Callahan et al., 2016). Quality trimming, denoising, error-correction, paired-end read merging, chimera removal, and dereplication steps were performed according to the default setting (Supplementary Table 1). The amplicon sequence variants (ASVs) were taxonomically classified with a naïve Bayesian classifier using the SILVA training dataset (v 137) (Quast et al., 2012). The ASV and taxonomy tables, along with associated sample metadata were imported into phyloseq (v 1.22.3) (McMurdie and Holmes, 2013) for community analysis. Whole metagenome sequencing data was processed using Trimmomatic (v 0.39) (Bolger et al., 2014), and the quality of the reads was checked with FastQC (v 0.11.8) (Andrews, 2010). The metagenome data was used for (a) validating the amplicon results with METAXA2 software (v 2.1.1) (Bengtsson-Palme et al., 2015); (b) calculating the metagenomic distances between the samples by making a gene-pool comparison/relatedness determination with the k-mer based weighted inner product, kWIP (Murray et al., 2017); (c) metagenome assembly and functional analysis. For these latter two analysis, we used MEGAHIT (v 1.2.9) (Li et al., 2015) to assemble the data, and QUAST (v 5.0.2) (Gurevich et al., 2013) to evaluate the assembly quality. For the subsequent functional analysis, SUPER-FOCUS (v 0.31) (Silva et al., 2016) was applied using the aligner DIAMOND (v 0.9.34) to obtain the enzyme commission (EC) catogories (Buchfink et al., 2015). In addition, taxonomic informed pathways classifications by sample groups were determined by HUMAnN2 (v 2.8.1) (Franzosa et al., 2018). Metagenome-assembled genomes (MAGs) were obtained by MetaBAT2 (v 2.15) (Kang et al., 2019) and manually refined after assessing the completeness (>70%) and contamination (<10%) by CheckM (v 1.0.13) (Parks et al., 2015). Taxonomic classification was conducted by GTDB-Tk (Chaumeil et al., 2020). Prodigal (v 2.6.3) (Hyatt et al., 2010) was used for open reading frames (ORFs) prediction. KofamKOALA (https://www.genome.jp/tools/kofamkoala/) (Aramaki et al., 2020) was used to obtain KO annotations for genes predicted by Prodigal. Xenobiotics metabolism was performed by the “Reconstruct Pathway” tool in KEGG mapper (https://www.genome.jp/kegg/mapper.html, accessed February 2021).
All statistical analysis was performed in R environment (v 3.6.0). cor.test function was used to test the correlation coefficient r between the TOrCs biotransformation performance and different biomass indicators based on the Pearson method. Linear regression was used to model the global compound removal by ATP concentration. Residuals were tested for normality (Shapiro-Wilk normality test), and the distribution was inspected through QQ (quantile-quantile) plot (Supplementary Figure 1). A paired two-sample Mann-Whitney-Wilcoxon test (non-parametric) was used to identify significant differences between kbiol values of two sand groups. Kruskal-Wallis test was used to evaluate the differences of individual compound kbiol among all sand samples and between categories. The relationships between DADA2 and METAXA2 data were examined by Mantel tests. The microbial diversity indices were analyzed using the vegan package (v 2.5-6) (Oksanen et al., 2019). The species richness was determined by rarefying the amplicon dataset to the smallest sample (5,799 reads) through the “rrarefy” function (Supplementary Figure 2). Chao1 and Inverse Simpson index (performed on full dataset prior to rarefaction) were used to present community richness and alpha diversity, respectively. Community compositions were compared using Bray-Curtis dissimilarities on ASV abundances and presented using NMDS ordinations. Ordinations and heatmaps were done in the R package “ampvis2” (v 2.4.6) (Andersen et al., 2018). The analysis of correlations between TOrCs biotransformation rates and microorganisms or functional genes was based on Pearson correlations outlined in the Rhea script collection (Lagkouvardos et al., 2017). Before doing the correlation analysis, we tested the differences between each compound kbiol and zero (criterion 1), and set the minimum removal percentage to 10% (criterion 2). As a consequence, the kbiol of carbamazepine, fluconazole and xipamide were excluded from further analysis, since either criterion 1 or 2 was not met in any of the batches. Differential functional genes and pathway analyses were conducted by DESeq2 (v 1.29.5) (Love et al., 2014).
Physical, chemical and biological characteristics of eight sand filters are shown in Table 2. Materials of these filters included quartz gravel, quartz sand, anthracite and pumice. Loss on ignition, ATP, DNA concentration and cell counts served as biomass indicators. Biomass and organic matter content was high in Friedrichshafen, Eriskirchen and Wangen (>25 pmol/g ATP, >40 mg/g Loss on ignition), whereas the remaining five biofilters were low in biomass and organic matter, with the lowest measurements in the filter materials from drinking water systems (BWA and IFW; <1.5 pmol/g ATP, <9 mg/g Loss on ignition) (Table 2, Figure 1A). The biotransformation rate constants of TOrCs were calculated excluding six compounds (i.e., acridone, caffeine, ibuprofen, levetiracetam acid, ramiprilat and valsartan) which did not fit the first-order kinetics. Moreover, carbamazepine, fluconazole, and xipamide had non-significant kbiol and showed <10% removal in all sand samples. Wangen achieved the best overall TOrC parent compound removal with a global mean removal percentage of 58 ± 0.64%, followed by Friedrichshafen (49 ± 3.4%) and Eriskirchen (45 ± 1.2%) (Figure 1B). Hungen and Stuttgart showed similar global removal percentages of 28 and 26%, followed by Moos with 14%, respectively. In the drinking water filters IFW and BWA, there were almost no TOrCs degraded (removal below 0.5%). These differences in global transformation potential were highly correlated with biomass expressed as ATP concentration (Pearson r = 0.92, p < 0.001), followed by living cell counts (Pearson r = 0.76, p < 0.001), while other parameters showed no correlation (Table 2). The mean global kbiol could be predicted by ATP concentration [logarithmic: ln(ATP), LM, t = 8.3, adjusted R2 = 0.76, p < 0.001; or linear: LM, t = 10.9, adjusted R2 = 0.84, p < 0.001, Figure 1C]. The linear model showed a better fit, but the distribution of residuals was non-normal (Supplementary Figure 1C). In addition to kbiol, the overall average removal percentage showed a clear relationship with ATP concentration, this time on a natural logarithmic scale (LM, t = 13.9, adjusted R2 = 0.90, p < 0.001, Figure 1D). Therefore, we used ATP for XBiomass normalization (Equation 1) of kbiol for the clustering analysis and the following correlation analysis. According to the ATP biomass, the samples with high biomass (Eriskirchen, Friedrichshafen and Wangen, named as HBG) had a high general biotransformation ability, while the biotransformation potential of the samples with low biomass (Hungen, Moos and Stuttgart, named as LBG) was relatively low, which can be also seen across individual compounds (Figure 2A). After ATP normalization, however, the individual differences between filter materials and compounds became less pronounced (Figure 2B, except IFW). When comparing drinking water sand group (IFW and BWA, named as DW) with the other two groups, their kbiol (mean value of individual substances in each group) remained different (Mann-Whitney-Wilcoxon test, W = 1305, p = 0.02 for HBG; W = 1318, p = 0.01 for LBG). However, when testing for differences in biotransformation of LBG and HBG, there was no significant difference in the mean global kbiol between the two groups (Mann-Whitney-Wilcoxon test, W = 1305, p = 0.33). This indicated their mean biotransformation performance were comparable per biomass unit, independent of other parameters such as microbial community composition. Differences between the kbiol of individual compounds persisted when comparing seven sand samples (IFW was excluded: the values close or below zero caused superimposed signals when normalized, Figure 2B) or HBG and LBG. Overall, 19 compounds showed significant differences in seven sand samples, and 15 substances showed significantly differential kbiol between HBG and LBG (Kruskal-Wallis test, adjusted p < 0.05) (Table 1). For comparison, we found 35 and 38 substances to differ in their kbiol before normalization for the sand filter materials and HBG vs. LBG, respectively.
Table 2. Biological characterization of eight sand filter materials and correlation test between TOrCs removal and biomass indicators.
Figure 1. (A) ATP concentration in eight sand filter samples; (B) Average global removal of 51 TOrCs by eight sand filter materials in percentage and molar concentration; Regression (blue/red line) for predicting (C) mean global biotransformation rate constants (kbiol) and (D) average removal percentage of TOrCs from ATP concentration, gray shadow represents the 95% confidence interval.
Figure 2. (A) Non-normalized and (B) normalized biotransformation rate constants (kbiol) of 45 TOrCs by eight sand filter materials. The low ATP concentration in the IFW sand (0.76 pmol/g) made the normalized results appear superimposed. The kbiol values were scaled within appropriate range (−2, 2) for better visualization. Clustering used the Manhattan distance metric and Ward's minimum variance method (Ward.D2). Six TOrCs were excluded as they did not fit the first-order kinetics.
We investigated the microbial community composition of all incubations using 16S rRNA amplicon sequencing and metagenomics. For the amplicon data, all sequences were clustered into a total of 23147 bacterial ASVs after the filtering step, showing obvious differences among the sand filters at the phylum level (Figure 3A). Apart from a high abundance of Proteobacteria in all filter materials, Nitrospirae dominated in the HBG with mean read abundances of 27.6%, followed by Bacteroidetes (11.5%). In the LBG, Nitrospirae only accounted for 0.7%. Microbial community composition was further cross-validated by microscopy (FISH) and metagenome classifications (METAXA2) (Figure 3A). In particular, the community composition matrix that resulted from the metagenome (METAXA2) was highly correlated with the composition matrix generated (at much deeper resolution) with the ASV amplicon data (Mantel tests, r = 0.96, p = 0.001). The FISH results confirmed that the relative proportion of cells and their respective biomass (assuming the same cell size) follow the results of the DNA based data (with exceptions; e.g., for the Nitrospirae community in Stuttgart).
Figure 3. Taxonomic composition of eight sand filter materials. (A) Comparison of taxa from microscopy (FISH) vs. 16S amplicon (DADA2) vs. metagenome (METAXA2); (B) Relative abundance of microorganisms at the genus level.
At the genus level, a high degree of variation in the taxonomic composition between the sand samples was observed (Figure 3B, Supplementary Figure 3). In the HBG, there was a high amount of Nitrospira in Wangen and Eriskirchen with mean relative abundances of 45.2 and 39.0%, respectively. In Friedrichshafen (which was operated for post-denitrification), Nitrospira only accounted for 3.6% and other lineages became more abundant (e.g., Propionivibrio, Geobacter, Romboutsia, Legionella). In LBG, the three sand samples exhibited largely different microbial composition without sharing abundant genera. Stenotrophobacter (24.4%) was prominent in Hungen and Dokdonella (17.8%) dominated the Stuttgart filter materials. Pseudomonas (20.1%) and Janthinobacterium (17.3%) were dominant in Moos. In contrast to HBG and LBG, DW showed a stark dominance of Pseudomonas with a mean relative abundance of 60.1%, which also resulted in the lowest biodiversity indices (Table 2). In a multivariate analysis of the community composition (presented as ASV or as k-mers derived from the metagenome) we could recover a separation into HBG and LBG (Supplementary Figure 4, adonis: for ASV, R2 = 0.21, p = 0.001; for metagenomics k-mers (kWIP), R2 = 0.19, p = 0.001).
Finally, we confirmed that the community composition may be linked to the biotransformations observed in the systems using a Mantel test for the ASV matrix and the (normalized) kbiol values of the incubations (r = 0.50, p = 0.001). Subsequently, we were interested if there are any specific linkages between microbes and biotransformations. For this, Pearson's coefficients r were used to find hypothetical linkages between the kbiol and relative abundances of (a) microbial genera (Figure 4A) or (b) functional genes (Figure 4B, see paragraph below). In total, there were 62 genera (as a data reduction step, all ASV were collapsed to genera for this purpose) that showed either a significantly positive or negative correlation with the biotransformation rates of TOrCs (with a cutoff of abs(r) > 0.7, adjusted p < 0.05, observation > 9) (Figure 4A). All of these (except Pseudomonas) ranked below 2% relative abundance and can be regarded as rare taxa. Thirty genera showed highly positive correlations with more than one TOrC, such as Denitratisoma, Hydrogenophaga and Ideonella. Four genera correlated with single TOrCs, i.e., Litorilinea, Novosphingobium, Paludibaculum, Phaeodactylibacter were only positively associated with the biotransformation of diclofenac, acyclovir, sulfamethoxazole, and bezafibrate, respectively. Nitrospira, the dominant genus in the HBG, had no significant correlation with any compound (only when non-normalized kbiol values were considered we found multiple negative correlations). Examples for the negative correlation were Conexibacter, Ferruginibacter, Intestinibacter, Methylibium, Pseudomonas, and Terrimonas.
Figure 4. Heatmap showing correlations between biotransformation rate constants (kbiol) of 45 TOrCs and (A) 62 genera; green bars represent the genera for which we obtained at least one MAG; (B) 30 significantly differential biotransformation related functions between the high biomass and the low biomass group. Cutoff is p < 0.05, abs(r) > 0.7, observation > 9. Average clustering was based on Euclidean distances.
By comparison with the integrated enzyme database of KEGG, we identified 20409 EC numbers from the metagenome sequences. Principle component analysis (PCA) based on the relative abundance of annotated functions demonstrated, similar to the taxonomic patterns described above, distinct clustering of different sand samples (Figure 5). We can observe a separation between the HBG and LBG on axis 2 (PC2, 12%) with the exception of Friedrichshafen that shifted toward LBG, potentially caused by the low abundance of Nitrospira. PC1 (78%) distinguished two clusters of HBG, LBG and DW, which may due to the microbial structure differences between drinking water filters and wastewater filters. When comparing HBG with LBG, 1017 functions were over-represented in HBG and 1702 were over-represented in LBG, respectively (Supplementary Figure 5). From these, we selected those enzyme commission categories responsible for biocatalysis/biodegradation according to EAWAG-BBD database and analyzed their associations with TOrCs biotransformation. This resulted in 30 functions that showed a correlation with TOrCs biotransformation [with a cutoff of abs(r) > 0.7, adjusted p < 0.05, observation > 9] (Figure 4B). Notably, enoyl-CoA hydratase (EC 4.2.1.17)/3-hydroxyacyl-CoA dehydrogenase (EC 1.1.1.35) encoding genes were positively correlated with eight compounds (i.e., benzotriazole, carboxy acyclovir, ceterizin, DEET, lamotrigine, mecoprop, terbuthylazine, and torasemid). Both, dissimilatory sulfite reductase (EC 1.8.99.3) and nitrite reductase (EC 1.7.2.1) genes, which encode for universal and essential enzymes in the sulfur and nitrogen cycle, were also correlated to the biotransformation of carboxy acyclovir, clarithromycin, furosemide and mecoprop. Further, a few functions showed a correlation to only a single compound removal rate. For example, amidase (EC 3.5.1.4) encoding genes were found to be only correlated to the kbiol of carboxy acyclovir; threonine dehydratase (EC 4.3.1.19); acyl-CoA dehydrogenase, benzoate degradation ring-cleavage hydrolase, nitrate reductase (EC 1.7.2.1), S-formylglutathione hydrolase (EC 3.1.2.12) encoding genes were only correlated with the removal rate of atenolol.
Figure 5. Principle component analysis (PCA) of eight sand filter materials based on the differential functions identified by DESeq2. Square symbol represents the high biomass group, triangle symbol represents the low biomass group, the remaining samples indicated by circles represent IFW and BWA.
Overall, there were 163 significantly differential abundant pathways annotated by HUMAnN2 comparing the HBG with LBG (see Supplementary Figure 6 for an overview excluding biosynthesis pathways). In the HBG, sulfate reduction, aromatic biogenic amine degradation and pathways regarding carbohydrate degradation (i.e. starch, glycogen, stachyose, D–galactose, galactose degradation) were overrepresented, the involved microorganisms were identified to be mainly Nitrospirae. In the LBG, energy metabolism pathways, such as the TCA cycle, Calvin-Benson-Bassham cycle, NAD/NADP–NADH/NADPH cytosolic interconversion, or the octane oxidation pathway were overrepresented.
We further inspected MAGs that taxonomically matched the genera for which we found correlations with individual kbiol (see section above, Figure 4A: genera with green bars) in order to see if these statistically as potential relevant genera contain biotransformation pathways. We annotated 37 MAGs in the KEGG Mapper for pathway reconstruction and we estimated the completeness of the pathways individually (Figure 6). In the category of xenobiotics metabolism, MAGs classified to Hydrogenophaga showed the most complete pathways, especially in benzoate and furfural degradation. Steroid degradation pathway was prominent in Sphingorhabdus and Pseudomonas.
Figure 6. Heatmap showing the completeness of xenobiotics degradation pathways in 37 biotransformation-correlated MAGs. Pathways are identified by “Reconstruct Pathway” tool in KEGG Mapper. Average clustering was based on Euclidean distances.
Currently, parameters such as redox conditions or biodegradable dissolved organic carbon, are used to evaluate the removal efficiencies of TOrCs (Bertelkamp et al., 2016; Torresi et al., 2019; Oberleitner et al., 2020), but new indicators directly associated to degradation processes (e.g., microorganisms, functional genes, transformation products) can be expected to be additional suitable tools for the prediction of the biotransformation potential and controlling removal performance. Here, we investigated the biotransformation of diverging TOrCs by eight biological active sand filter materials from wastewater and drinking water treatment plants, for which the metagenomic analysis of the microbial communities provided novel insights into the biological potential of TOrC transformations.
We observed largely differing taxonomic and genetic profiles within each sand filter, accompanied by stark differences in the overall biotransformation performance (−1.4 vs. 58% removal) across all investigated TOrCs. The sand filter materials are influencing the biofilm formation and thus ATP and potentially microbial community composition, but they were not considered to have a direct association with (abiotical) TOrC transformations, for which their sorption effect can be ignored due to a) the polar substances and the rather low sorption affinity and b) the adsorption equilibrium from previous long-term exposure in the environment. This may point to the conclusion that functional microbial communities are the main predictor of TOrC transformations, however, most of these differences were eliminated after accounting for biomass, measured as ATP. Our results imply that the effect of biomass on the global TOrC transformation potential is foremost independent of the microbial community composition. We therefore had to partially reject our hypothesis that the microbial community composition is the major driver between global biotransformation potential in our experimental setup. However, on the other hand, the clear correlation of the compound matrix with the taxonomic matrix found by the Mantel test and later with single genera after normalization is evidence that although the global transformation potential is determined by biomass, the transformation of individual compounds is related to the taxonomic composition of the biological active sand filter system. After normalization, we could still identify significant differences for more than 15 different compounds (Table 1, e.g., clarithromycin, mecoprop, metoprolol, trimethoprim, furosemide, atenolol), which may be good candidates for a rather taxa or community specific degradation. Other studies already found indications that biomass is important for overall system performance, e.g., Liang et al. (2021) observed that the biomass increased with the running time of the reactors when also the TOrC removal increased, and Torresi et al. (2016) observed an enhanced TOrC removal with an increased biofilm thickness, although this feature was simultaneously attributed to increased diversity. In our study, however, alpha-diversity showed no such correlation (Table 2).
Our study indicates that we can predict the global transformation potential of (ex situ) materials from biofilters largely (explaining 76–84% of the kbiol variation, or 90% of the variation for compound removal) by measuring the living biomass as ATP concentration. Further studies will be needed to assess if the relationship between ATP and kbiol is linear or logarithmic [the two models were not significantly different (ANOVA, p = 0.24)]. Biomass is used as a linear parameter for TOrCs biotransformation kinetics (see Equation 1), and originally, the kbiol was determined by the absolute abundance of the functional degraders of the respective TOrC (Bekins et al., 1998). In mixed microbial communities, however, this relationship may have to be re-evaluated in the future.
When comparing frequently used biomass estimators for kbiol, ATP, a biomass parameter that is frequently used in microbiology, has not been considered for kbiol calculation of TOrC removal rates before. The biotransformation rate constants kbiol were previously normalized by attached or suspended biomass as dry weight (Casas et al., 2015; Mazioti et al., 2015; Torresi et al., 2016), total suspended solids (Achermann et al., 2018), or DNA concentration (Liang et al., 2021). However, most methods are accompanied by certain biases. For example, dead and dormant cells will strongly influence DNA measurements and cell counts; or the biofilm EPS will bias DNA measurement (through external DNA), dry weight, and total organic carbon measurement (LOI). In our study, living and total cells (biased only by the different cell volumes) were still the best predictors after ATP (Table 2). Our findings suggest that at least for biofiltration systems the global potential for the biotransformation of TOrCs is more dependent on ATP than on other biomass indicators. Hence, ATP could have a profound impact when comparing biotransformation results across studies and treatments.
While this conclusion will hopefully change the way we analyze TOrCs biotransformations, ATP itself has been frequently used as an important biomass indicator in drinking water biofiltration systems for natural organic matter (NOM) transformation (Pharand et al., 2014; Chen et al., 2016; Kirisits et al., 2019). This is not surprising since NOM is used as a substrate by the microbial communities in various environments such as soil, sediment, marine, and freshwater (Kolehmainen et al., 2007; Huang et al., 2011; Diem et al., 2013; Simon et al., 2013). However, TOrCs are not considered as substrates per se and their concentrations are by definition generally considered as too low for biomass maintenance and growth and therefore efficient degradation. Co-metabolism through promiscuity or mixed substrate use can be a mechanism that lead to TOrC removal (Rauch-Williams et al., 2010; Hellauer et al., 2019).
In general, we divided the filters into three groups according to the clustering results, microbial community composition, and biomass estimates. The taxonomic composition between the HBG, LBG, DW, and also between single sand filters was different. HBG, though, shared Nitrospirae as highly abundant lineage (Figure 3), a diverse and widespread group of often autotrophic, nitrite-oxidizing bacteria (Koch et al., 2015). Previous studies found that biotransformation of certain TOrCs can be related to ammonia oxidation activity of nitrifying activated sludge and biofilms in WWTPs (Helbling et al., 2012; Rattier et al., 2014; Men et al., 2017; Xu et al., 2017b). Moreover, asulam, carbendazim, fenhexamid, mianserin, and ranitidine showed biotransformation (16–85%) by the isolate Nitrospira inopinata (Han et al., 2019). Metagenome data of rapid gravity sand filter microorganisms also suggested that Nitrospira may serve as keystone species that drives the microbial ecosystems by providing organic carbon compounds and enable heterotrophic ammonium and carbon cycling (Palomo et al., 2016). In our system, we found no direct statistical linkage between Nitrospira and the biotransformation rate constants, however, it is possible that at least in the investigated biofilters Nitrospirae lineages as autotrophic species enables the establishment of a high microbial biomass, and thus enables the TOrC transformations through other microbial members. This also matches the observations by Liang et al. (2021), which indicates that the biotransformation may rely on rare community members of TOrCs-specific degraders, while the community of their moving bed reactor followed a progressive succession toward a Nitrospirae based climax community. The hypothesized positive and negative correlations of (biomass normalized) TOrC biotransformation with microbial genera pointed to rare biosphere microorganisms (<2%), which exhibited most of the correlations (>98%) with individual TOrCs removal rates. Although these correlations require experimental verification to test for causal relationships, these correlation-based hypotheses are in line with previous reports indicating that a small fraction of highly-specialized microorganisms, accounting for <0.1% of the microbial communities in biofilms, may be responsible for the TOrC transformation (Falås et al., 2018). One of the highly correlating genera was Hydrogenophaga, which showed a putative linkage to the removal of amisulprid, clarithromycin, climbazole, flecainide, oxypurinol, sitagliptine, sulpirid, terbutryn and venlafaxine (the top left cluster in Figure 4A). This genus has also been previously reported to efficiently remove diclofenac, metoprolol, clarithromycin, erythromycin, atenolol and codeine (Kanaujiya et al., 2019).
In the drinking water sand filters DW, the biotransformation performance significantly differed from the wastewater filters. Here, Pseudomonas was found dominating DW, a common inhabitant of aquatic environments, including oligotrophic drinking water, lake water and surface water (Lopez et al., 2005; Huang et al., 2015; Nasreen et al., 2015). Although Pseudomonas has been reported to be able to degrade certain micropollutants (Li et al., 2010; Tezel et al., 2012; Devi et al., 2019), in our case, it also clustered in proximity to Hydrogenophaga, however, with more pronounced negative correlations with TOrCs.
The relative abundance of functional genes and encoded biotransformation pathways also correlated with individual compounds and resulted in a clearer clustering than the microbial lineages. Enoyl-CoA hydratase/3-hydroxyacyl-CoA dehydrogenase was overrepresented in the HBG and was positively correlated with the kbiol of eight TOrCs in our study. The enoyl-CoA hydratase is known to catalyze a β-oxidation substrate by adding hydroxyl groups and a proton to an unsaturated β-carbon of the molecule (Salgado et al., 2020). For instance, the degradation of ibuprofen can be initiated by enoyl-CoA hydratase with the introduction of hydroxyl groups, this enzyme was found to be upregulated during the biodegradation process (Almeida et al., 2013). Enoyl-CoA hydratase was also identified in steroid estrogen degradation by bacterium Serratia nematodiphila (Zhao et al., 2020). Furthermore, Cameron et al. (2019) reported enoyl-CoA hydratase contributed to biofilm formation and the antibiotic tolerance, which also supported our findings that the high biomass promoted the TOrCs removal. Therefore, we suggest that a high abundance of enoyl-CoA hydratase/3-hydroxyacyl-CoA dehydrogenase might indicate a favorable functional potential for TOrCs biotransformation. Dissimilatory sulfite reductase (EC 1.8.99.3) and nitrite reductase (EC 1.7.2.1) were found to be correlated to the biotransformation of carboxy acyclovir, clarithromycin, furosemide and mecoprop in our study. In previous studies, sulfite reductase was reported to catalyze the cleavage of isoxazole and piperazinyl rings (Jia et al., 2019), and nitrite reductase was correlated with the biotransformation rate constant of some compounds like sulfamethoxazole, erythromycin and trimethoprim (Torresi et al., 2018).
Our study was designed to compare the biological transformation potential of the filter materials ex situ under controlled conditions by applying laboratory scale transformation batch experiments. This comes with certain limitations and potential batch effects caused by, e.g., storage of sediments, which may have altered the natural microbial community of the sand filters to some degree. Our experiment also simplified the in situ systems, because factors such as engineering design, redox potential, hydraulic retention time, feed/famine cycles that can influence the bioactivity, were explicitly not considered here. Moreover, we mainly focused on filter systems used for post-treatment of conventionally treated wastewater, and thus our results may be more representative for these types of rapid sand filters that have already experienced long-term exposure to TOrCs. The selection of 51 polar compounds maybe not fully representative of the full spectrum of TOrCs, but they cover a typical range of biodegradable and persistent compounds that can be found in wastewater (Ahmad et al., 2019), and the historical exposure of sand filters avoids artifacts due to falsely adapted or artificial assembled microbial communities. Since the selected TOrCs are relatively polar compounds and for many of them organic carbon normalized distribution coefficients have been reported to be rather low (Ternes et al., 2004; Stein et al., 2008; Ramil et al., 2010), sorption was considered negligible and we assumed that the observed removal was mainly attributed to transformation processes. Future studies should be conducted to answer the question whether ATP can also account for differences in in situ full-scale filters under natural retention times and if for example the filter material choice can be used as an option to control the biomass. Moreover, it should be validated if autotrophic taxa, such as Nitrospirae, can indeed act as primary carbon and energy deliverer that support potential rare indicator taxa such as Hydrogenophaga for individual TOrC transformations. The identified correlations with enzymatic classes and taxa should be seen as putative links that need to be verified by future studies providing direct evidence. For example, additional metatranscriptome analysis on gene expression or stable isotope probing could provide us with a more detailed picture of TOrCs biotransformation mechanisms. Finally, our findings can be used as hypothesis for further looking into the details of TOrC biotransformation and its relationship with single taxa or whole communities.
To summarize, we investigated the biotransformation performance of 51 TOrCs in eight sand filters from wastewater and drinking water treatment plants and established associations between the microorganisms, functional genes and the TOrCs biotransformation, which is also of high practical relevance as it could support the optimization and control of these systems. We conclude that
1. after normalization to microbial biomass, there was no significant difference of the average kbiol between the main sand filter systems suggesting that biomass influences TOrC transformation globally;
2. the removal of individual compounds, however, was related to the taxonomic composition of the biological active sand filter system, indicated by individual kbiol correlation with single genera, and by the global correlation of the microbial community composition with the normalized kbiol matrix;
3. biotransformation of several TOrCs was rather correlated to rare biosphere lineages, e.g., Hydrogenophaga that had the most complete xenobiotics degradation pathways; on the enzymatic level, Enoyl-CoA hydratase/3-hydroxyacyl-CoA dehydrogenase, showed the broadest correlation with individual TOrC kbiol; hence, these may be examples of suitable indicators for assessing biotransformation potentials;
4. the calculation of the kbiol should be re-evaluated using a biomass marker for living cells (e.g., ATP) and traditional biomass estimators should not be used any more in transformation studies for normalization purposes.
All sequence data (amplicons, metagenome, assembled MAGs) has been deposited at INSDC (with ENA: https://www.ebi.ac.uk/ena) under the project accession number PRJEB43767. The ASV table of the amplicon data, and the DESeq2 output for enzyme categories and pathways from the metagenome data are deposited as csv files in the Supplementary Materials.
AW and DW designed and performed the experiment and the chemical analysis. LC, RL, and CW analyzed the molecular data and made the statistical analysis. LC performed the metagenome assembly. CW and LC developed and wrote the initial draft of the manuscript. All authors improved the manuscript sequentially by several rounds of review. All authors contributed to the article and approved the submitted version.
This work was supported by German Federal Ministry of Education and Research (BMBF), project OPTI (02WIL1388); Chinese Scholarship Council; International PhD Programme Environment, Resources and Sustainable Development scholarship (#Parthenope University of Naples); TUM Junior Fellow Funds.
The authors declare that the research was conducted in the absence of any commercial or financial relationships that could be construed as a potential conflict of interest.
All claims expressed in this article are solely those of the authors and do not necessarily represent those of their affiliated organizations, or those of the publisher, the editors and the reviewers. Any product that may be evaluated in this article, or claim that may be made by its manufacturer, is not guaranteed or endorsed by the publisher.
We would like to acknowledge the Leibniz-Rechenzentrum for providing computational support and Dr. Uwe Hübner for proof reading and a critical review of the manuscript. Dr. Claudia Beimfohr of Vermicon AG, Germany, is thanked for carrying out FISH analyses and cell count measurement.
The Supplementary Material for this article can be found online at: https://www.frontiersin.org/articles/10.3389/frwa.2022.832297/full#supplementary-material
Data Sheet 1. ASV abundance table.
Data Sheet 2. Differential enzyme categories abundance table.
Data Sheet 3. Differential pathway abundance table.
Data Sheet 4. TOrCs removal pattern over time.
Achermann, S., Bianco, V., Mansfeldt, C. B., Vogler, B., Kolvenbach, B. A., Corvini, P. F., et al. (2018). Biotransformation of sulfonamide antibiotics in activated sludge: the formation of pterin-conjugates leads to sustained risk. Environ. Sci. Technol. 52, 6265–6274. doi: 10.1021/acs.est.7b06716
Ahmad, J., Naeem, S., Ahmad, M., Usman, A. R., and Al-Wabel, M. I. (2019). A critical review on organic micropollutants contamination in wastewater and removal through carbon nanotubes. J. Environ. Manage. 246, 214–228. doi: 10.1016/j.jenvman.2019.05.152
Almeida, B., Kjeldal, H., Lolas, I., Knudsen, A. D., Carvalho, G., Nielsen, K. L., et al. (2013). Quantitative proteomic analysis of ibuprofen-degrading Patulibacter sp. strain I11. Biodegradation 24, 615–630. doi: 10.1007/s10532-012-9610-5
Andersen, K. S., Kirkegaard, R. H., Karst, S. M., and Albertsen, M. (2018). ampvis2: an R package to analyse and visualise 16S rRNA amplicon data. BioRxiv 299537. doi: 10.1101/299537
Andrews, S. (2010). FastQC: A Quality Control Tool for High Throughput Sequence Data. Available online at: http://www.bioinformatics.babraham.ac.uk/projects/fastqc/
Aramaki, T., Blanc-Mathieu, R., Endo, H., Ohkubo, K., Kanehisa, M., Goto, S., et al. (2020). KofamKOALA: KEGG ortholog assignment based on profile HMM and adaptive score threshold. Bioinformatics 36, 2251–2252. doi: 10.1093/bioinformatics/btz859
Bekins, B. A., Warren, E., and Godsy, E. M. (1998). A comparison of zero-order, first-order, and monod biotransformation models. Groundwater 36, 261–268. doi: 10.1111/j.1745-6584.1998.tb01091.x
Bengtsson-Palme, J., Hartmann, M., Eriksson, K. M., Pal, C., Thorell, K., Larsson, D. G. J., et al. (2015). METAXA2: improved identification and taxonomic classification of small and large subunit rRNA in metagenomic data. Mol. Ecol. Resour. 15, 1403–1414. doi: 10.1111/1755-0998.12399
Bertelkamp, C., van der Hoek, J. P., Schoutteten, K., Hulpiau, L., Vanhaecke, L., Bussche, J. V., et al. (2016). The effect of feed water dissolved organic carbon concentration and composition on organic micropollutant removal and microbial diversity in soil columns simulating river bank filtration. Chemosphere 144, 932–939. doi: 10.1016/j.chemosphere.2015.09.017
Bolger, A. M., Lohse, M., and Usadel, B. (2014). Trimmomatic: a flexible trimmer for Illumina sequence data. Bioinformatics 30, 2114–2120. doi: 10.1093/bioinformatics/btu170
Buchfink, B., Xie, C., and Huson, D. H. (2015). Fast and sensitive protein alignment using DIAMOND. Nat. Methods 12, 59–60. doi: 10.1038/nmeth.3176
Callahan, B. J., McMurdie, P. J., Rosen, M. J., Han, A. W., Johnson, A. J. A., and Holmes, S. P. (2016). DADA2: high-resolution sample inference from Illumina amplicon data. Nat. Methods 13, 581–583. doi: 10.1038/nmeth.3869
Cameron, L. C., Bonis, B., Phan, C. Q., Kent, L. A., Lee, A. K., and Hunter, R. C. (2019). A putative enoyl-CoA hydratase contributes to biofilm formation and the antibiotic tolerance of Achromobacter xylosoxidans. NPJ Biofilms Microbiomes 5, 1–7. doi: 10.1038/s41522-019-0093-6
Carpenter, C. M., and Helbling, D. E. (2017). Removal of micropollutants in biofilters: hydrodynamic effects on biofilm assembly and functioning. Water Res. 120, 211–221. doi: 10.1016/j.watres.2017.04.071
Casas, M. E., Chhetri, R. K., Ooi, G., Hansen, K. M., Litty, K., Christensson, M., et al. (2015). Biodegradation of pharmaceuticals in hospital wastewater by staged Moving Bed Biofilm Reactors (MBBR). Water Res. 83, 293–302. doi: 10.1016/j.watres.2015.06.042
Castronovo, S., Wick, A., Scheurer, M., Nödler, K., Schulz, M., and Ternes, T. A. (2017). Biodegradation of the artificial sweetener acesulfame in biological wastewater treatment and sandfilters. Water Res. 110, 342–353. doi: 10.1016/j.watres.2016.11.041
Chaumeil, P. A., Mussig, A. J., Hugenholtz, P., and Parks, D. H. (2020). GTDB-Tk: a toolkit to classify genomes with the Genome Taxonomy Database. Bioinformatics 36, 1925–1927. doi: 10.1093/bioinformatics/btz848
Chen, F., Peldszus, S., Elhadidy, A. M., Legge, R. L., Van Dyke, M. I., and Huck, P. M. (2016). Kinetics of natural organic matter (NOM) removal during drinking water biofiltration using different NOM characterization approaches. Water Res. 104, 361–370. doi: 10.1016/j.watres.2016.08.028
Chtourou, M., Mallek, M., Dalmau, M., Mamo, J., Santos-Clotas, E., Salah, A. B., et al. (2018). Triclosan, carbamazepine and caffeine removal by activated sludge system focusing on membrane bioreactor. Process Safety Environ. Protection 118, 1–9. doi: 10.1016/j.psep.2018.06.019
Daims, H., Lücker, S., and Wagner, M. (2006). Daime, a novel image analysis program for microbial ecology and biofilm research. Environ. Microbiol. 8, 200–213. doi: 10.1111/j.1462-2920.2005.00880.x
Devault, D. A., Amalric, L., Bristeau, S., Cruz, J., Tapie, N., Karolak, S., et al. (2021). Removal efficiency of emerging micropollutants in biofilter wastewater treatment plants in tropical areas. Environ. Sci. Pollution Res. 28, 10940–10966. doi: 10.1007/s11356-020-10868-z
Devi, R. S., Ramya, R., Kannan, K., Antony, A. R., and Kannan, V. R. (2019). Investigation of biodegradation potentials of high density polyethylene degrading marine bacteria isolated from the coastal regions of Tamil Nadu, India. Mar. Pollut. Bull. 138, 549–560. doi: 10.1016/j.marpolbul.2018.12.001
Diem, S., Von Rohr, M. R., Hering, J. G., Kohler, H. P. E., Schirmer, M., and Von Gunten, U. (2013). NOM degradation during river infiltration: effects of the climate variables temperature and discharge. Water Res. 47, 6585–6595. doi: 10.1016/j.watres.2013.08.028
Falås, P., Jewell, K. S., Hermes, N., Wick, A., Ternes, T. A., Joss, A., et al. (2018). Transformation, CO2 formation and uptake of four organic micropollutants by carrier-attached microorganisms. Water Res. 141, 405–416. doi: 10.1016/j.watres.2018.03.040
Falås, P., Wick, A., Castronovo, S., Habermacher, J., Ternes, T. A., and Joss, A. (2016). Tracing the limits of organic micropollutant removal in biological wastewater treatment. Water Res. 95, 240–249. doi: 10.1016/j.watres.2016.03.009
Ferrando-Climent, L., Collado, N., Buttiglieri, G., Gros, M., Rodriguez-Roda, I., Rodriguez-Mozaz, S., et al. (2012). Comprehensive study of ibuprofen and its metabolites in activated sludge batch experiments and aquatic environment. Sci. Total Environ. 438, 404–413. doi: 10.1016/j.scitotenv.2012.08.073
Fischer, K., and Majewsky, M. (2014). Cometabolic degradation of organic wastewater micropollutants by activated sludge and sludge-inherent microorganisms. Appl. Microbiol. Biotechnol. 98, 6583–6597. doi: 10.1007/s00253-014-5826-0
Franzosa, E. A., McIver, L. J., Rahnavard, G., Thompson, L. R., Schirmer, M., Weingart, G., et al. (2018). Species-level functional profiling of metagenomes and metatranscriptomes. Nat. Methods 15, 962–968. doi: 10.1038/s41592-018-0176-y
Grandclément, C., Seyssiecq, I., Piram, A., Wong-Wah-Chung, P., Vanot, G., Tiliacos, N., et al. (2017). From the conventional biological wastewater treatment to hybrid processes, the evaluation of organic micropollutant removal: a review. Water Res. 111, 297–317. doi: 10.1016/j.watres.2017.01.005
Gurevich, A., Saveliev, V., Vyahhi, N., and Tesler, G. (2013). QUAST: quality assessment tool for genome assemblies. Bioinformatics 29, 1072–1075. doi: 10.1093/bioinformatics/btt086
Han, P., Yu, Y., Zhou, L., Tian, Z., Li, Z., Hou, L., et al. (2019). Specific micropollutant biotransformation pattern by the comammox bacterium Nitrospira inopinata. Environ. Sci. Technol. 53, 8695–8705. doi: 10.1021/acs.est.9b01037
Helbling, D. E., Johnson, D. R., Honti, M., and Fenner, K. (2012). Micropollutant biotransformation kinetics associate with WWTP process parameters and microbial community characteristics. Environ. Sci. Technol. 46, 10579–10588. doi: 10.1021/es3019012
Hellauer, K., Mayerlen, S. M., Drewes, J. E., and Hübner, U. (2019). Biotransformation of trace organic chemicals in the presence of highly refractory dissolved organic carbon. Chemosphere 215, 33–39. doi: 10.1016/j.chemosphere.2018.09.166
Huang, G., Meng, F., Zheng, X., Wang, Y., Wang, Z., Liu, H., et al. (2011). Biodegradation behavior of natural organic matter (NOM) in a biological aerated filter (BAF) as a pretreatment for ultrafiltration (UF) of river water. Appl. Microbiol. Biotechnol. 90, 1795–1803. doi: 10.1007/s00253-011-3251-1
Huang, T., Guo, L., Zhang, H., Su, J., Wen, G., and Zhang, K. (2015). Nitrogen-removal efficiency of a novel aerobic denitrifying bacterium, Pseudomonas stutzeri strain ZF31, isolated from a drinking-water reservoir. Bioresour. Technol. 196, 209–216. doi: 10.1016/j.biortech.2015.07.059
Hyatt, D., Chen, G. L., and LoCascio, P. F. (2010). Prodigal: prokaryotic gene recognition and translation initiation site identification. BMC Bioinformatics 11, 119. doi: 10.1186/1471-2105-11-119
Jia, Y., Zhang, H., Khanal, S. K., Yin, L., and Lu, H. (2019). Insights into pharmaceuticals removal in an anaerobic sulfate-reducing bacteria sludge system. Water Res. 161, 191–201. doi: 10.1016/j.watres.2019.06.010
Johnson, D. R., Helbling, D. E., Lee, T. K., Park, J., Fenner, K., Kohler, H. P. E., et al. (2015). Association of biodiversity with the rates of micropollutant biotransformations among full-scale wastewater treatment plant communities. Appl. Environ. Microbiol. 81, 666–675. doi: 10.1128/AEM.03286-14
Kanaujiya, D. K., Paul, T., Sinharoy, A., and Pakshirajan, K. (2019). Biological treatment processes for the removal of organic micropollutants from wastewater: a review. Curr. Pollution Rep. 5, 112–128. doi: 10.1007/s40726-019-00110-x
Kang, D. D., Li, F., Kirton, E., Thomas, A., Egan, R., An, H., et al. (2019). MetaBAT 2: an adaptive binning algorithm for robust and efficient genome reconstruction from metagenome assemblies. PeerJ 7, e7359. doi: 10.7717/peerj.7359
Kirisits, M. J., Emelko, M. B., and Pinto, A. J. (2019). Applying biotechnology for drinking water biofiltration: advancing science and practice. Curr. Opin. Biotechnol. 57, 197–204. doi: 10.1016/j.copbio.2019.05.009
Kjeldal, H., Lolas, I. B. Y., Knudsen, A. D., Carvalho, G., Nielsen, K. L., Crespo, M. T. B., et al. (2019). Defining lower limits of biodegradation: atrazine degradation regulated by mass transfer and maintenance demand in Arthrobacter aurescens TC1. ISME J. 13, 2236–2251. doi: 10.1038/s41396-019-0430-z
Koch, H., Lücker, S., Albertsen, M., Kitzinger, K., Herbold, C., Spieck, E., et al. (2015). Expanded metabolic versatility of ubiquitous nitrite-oxidizing bacteria from the genus Nitrospira. Proc. Nat. Acad. Sci. U.S.A. 112, 11371–11376. doi: 10.1073/pnas.1506533112
Kolehmainen, R. E., Langwaldt, J. H., and Puhakka, J. A. (2007). Natural organic matter (NOM) removal and structural changes in the bacterial community during artificial groundwater recharge with humic lake water. Water Res. 41, 2715–2725. doi: 10.1016/j.watres.2007.02.042
Kosek, K., Luczkiewicz, A., Fudala-Ksiazek, S., Jankowska, K., Szopińska, M., Svahn, O., et al. (2020). Implementation of advanced micropollutants removal technologies in wastewater treatment plants (WWTPs)-examples and challenges based on selected EU countries. Environ. Sci. Policy 112, 213–226. doi: 10.1016/j.envsci.2020.06.011
Kruglova, A., Ahlgren, P., Korhonen, N., Rantanen, P., Mikola, A., and Vahala, R. (2014). Biodegradation of ibuprofen, diclofenac and carbamazepine in nitrifying activated sludge under 12°C temperature conditions. Sci. Total Environ. 499, 394–401. doi: 10.1016/j.scitotenv.2014.08.069
Lagkouvardos, I., Fischer, S., Kumar, N., and Clavel, T. (2017). Rhea: a transparent and modular R pipeline for microbial profiling based on 16S rRNA gene amplicons. PeerJ 5, e2836. doi: 10.7717/peerj.2836
Li, D., Liu, C. M., Luo, R., Sadakane, K., and Lam, T. W. (2015). MEGAHIT: an ultra-fast single-node solution for large and complex metagenomics assembly via succinct de Bruijn graph. Bioinformatics 31, 1674–1676. doi: 10.1093/bioinformatics/btv033
Li, H., Li, X., Duan, Y., Zhang, K. Q., and Yang, J. (2010). Biotransformation of nicotine by microorganism: the case of Pseudomonas spp. Appl. Microbiol. Biotechnol. 86, 11–17. doi: 10.1007/s00253-009-2427-4
Liang, C., de Jonge, N., Carvalho, P. N., Nielsen, J. L., and Bester, K. (2021). Biodegradation kinetics of organic micropollutants and microbial community dynamics in a moving bed biofilm reactor. Chem. Eng. J. 415, 128963. doi: 10.1016/j.cej.2021.128963
Lopez, L., Pozo, C., Rodelas, B., Calvo, C., Juarez, B., Martinez-Toledo, M. V., et al. (2005). Identification of bacteria isolated from an oligotrophic lake with pesticide removal capacities. Ecotoxicology 14, 299–312. doi: 10.1007/s10646-003-6367-y
Love, M. I., Huber, W., and Anders, S. (2014). Moderated estimation of fold change and dispersion for RNA-seq data with DESeq2. Genome Biol. 15, 550. doi: 10.1186/s13059-014-0550-8
Luo, Y., Guo, W., Ngo, H. H., Nghiem, L. D., Hai, F. I., Zhang, J., et al. (2014). A review on the occurrence of micropollutants in the aquatic environment and their fate and removal during wastewater treatment. Sci. Total Environ. 473, 619–641. doi: 10.1016/j.scitotenv.2013.12.065
Matamoros, V., Arias, C., Brix, H., and Bayona, J. M. (2007). Removal of pharmaceuticals and personal care products (PPCPs) from urban wastewater in a pilot vertical flow constructed wetland and a sand filter. Environ. Sci. Technol. 41, 8171–8177. doi: 10.1021/es071594+
Mazioti, A. A., Stasinakis, A. S., Pantazi, Y., and Andersen, H. R. (2015). Biodegradation of benzotriazoles and hydroxy-benzothiazole in wastewater by activated sludge and moving bed biofilm reactor systems. Bioresour. Technol. 192, 627–635. doi: 10.1016/j.biortech.2015.06.035
McMurdie, P. J., and Holmes, S. (2013). phyloseq: an R package for reproducible interactive analysis and graphics of microbiome census data. PLoS ONE 8, e61217. doi: 10.1371/journal.pone.0061217
Men, Y., Achermann, S., Helbling, D. E., Johnson, D. R., and Fenner, K. (2017). Relative contribution of ammonia oxidizing bacteria and other members of nitrifying activated sludge communities to micropollutant biotransformation. Water Res. 109, 217–226. doi: 10.1016/j.watres.2016.11.048
Moe, W. M., and Irvine, R. L. (2001). Effect of nitrogen limitation on performance of toluene degrading biofilters. Water Res. 35, 1407–1414. doi: 10.1016/S0043-1354(00)00417-6
Montiel-León, J. M., Duy, S. V., Munoz, G., Amyot, M., and Sauvé, S. (2018). Evaluation of on-line concentration coupled to liquid chromatography tandem mass spectrometry for the quantification of neonicotinoids and fipronil in surface water and tap water. Anal. Bioanal. Chem. 410, 2765–2779. doi: 10.1007/s00216-018-0957-2
Müller, J., Drewes, J. E., and Hübner, U. (2017). Sequential biofiltration–a novel approach for enhanced biological removal of trace organic chemicals from wastewater treatment plant effluent. Water Res. 127, 127–138. doi: 10.1016/j.watres.2017.10.009
Murray, K. D., Webers, C., Ong, C. S., Borevitz, J., and Warthmann, N. (2017). kWIP: The k-mer weighted inner product, a de novo estimator of genetic similarity. PLoS Comput. Biol. 13, e1005727. doi: 10.1371/journal.pcbi.1005727
Nasreen, M., Sarker, A., Malek, M. A., Ansaruzzaman, M. D., and Rahman, M. (2015). Prevalence and resistance pattern of Pseudomonas aeruginosa isolated from surface water. Adv Microbiol. 5, 74. doi: 10.4236/aim.2015.51008
Oberleitner, D., Schulz, W., Bergmann, A., and Achten, C. (2020). Impact of seasonality, redox conditions, travel distances and initial concentrations on micropollutant removal during riverbank filtration at four sites. Chemosphere 250, 126255. doi: 10.1016/j.chemosphere.2020.126255
Oksanen, J., Blanchet, F. G., Friendly, M., Kindt, R., Legendre, P., McGlinn, D., et al. (2019). vegan: Community Ecology Package. R Package version 2.5-6.
Palomo, A., Fowler, S. J., Gülay, A., Rasmussen, S., Sicheritz-Ponten, T., and Smets, B. F. (2016). Metagenomic analysis of rapid gravity sand filter microbial communities suggests novel physiology of Nitrospira spp. ISME J. 10, 2569–2581. doi: 10.1038/ismej.2016.63
Paredes, L., Fernandez-Fontaina, E., Lema, J. M., Omil, F., and Carballa, M. (2016). Understanding the fate of organic micropollutants in sand and granular activated carbon biofiltration systems. Sci. Total Environ. 551, 640–648. doi: 10.1016/j.scitotenv.2016.02.008
Parks, D. H., Imelfort, M., Skennerton, C. T., Hugenholtz, P., and Tyson, G. W. (2015). CheckM: assessing the quality of microbial genomes recoveredfrom isolates, single cells, and metagenomes. Genome Res. 25, 1043–1055. doi: 10.1101/gr.186072.114
Pharand, L., Van Dyke, M. I., Anderson, W. B., and Huck, P. M. (2014). Assessment of biomass in drinking water biofilters by adenosine triphosphate. J. Am. Water Works Assoc. 106, E433–E444. doi: 10.5942/jawwa.2014.106.0107
Prasse, C., Wagner, M., Schulz, R., and Ternes, T. A. (2011). Biotransformation of the antiviral drugs acyclovir and penciclovir in activated sludge treatment. Environ. Sci. Technol. 45, 2761–2769. doi: 10.1021/es103732y
Priya, V. S., and Philip, L. (2015). Treatment of volatile organic compounds in pharmaceutical wastewater using submerged aerated biological filter. Chem. Eng. J. 266, 309–319. doi: 10.1016/j.cej.2014.12.048
Quast, C., Pruesse, E., Yilmaz, P., Gerken, J., Schweer, T., Yarza, P., et al. (2012). The SILVA ribosomal RNA gene database project: improved data processing and web-based tools. Nucleic Acids Res. 41, D590–D596. doi: 10.1093/nar/gks1219
R Core Team (2016). R: A Language and Environment for Statistical Computing. Vienna. Available online at: https://www.R-project.org/
Ramil, M., El Aref, T., Fink, G., Scheurer, M., and Ternes, T. A. (2010). Fate of beta blockers in aquatic-sediment systems: sorption and biotransformation. Environ. Sci. Technol. 44, 962–970. doi: 10.1021/es9027452
Rattier, M., Reungoat, J., Keller, J., and Gernjak, W. (2014). Removal of micropollutants during tertiary wastewater treatment by biofiltration: role of nitrifiers and removal mechanisms. Water Res. 54, 89–99. doi: 10.1016/j.watres.2014.01.030
Rauch-Williams, T., Hoppe-Jones, C., and Drewes, J. E. (2010). The role of organic matter in the removal of emerging trace organic chemicals during managed aquifer recharge. Water Res. 44, 449–460. doi: 10.1016/j.watres.2009.08.027
Salgado, R., Brito, D., Noronha, J. P., Almeida, B., Bronze, M. R., Oehmen, A., et al. (2020). Metabolite identification of ibuprofen biodegradation by Patulibacter medicamentivorans under aerobic conditions. Environ. Technol. 41, 450–465. doi: 10.1080/09593330.2018.1502362
Shao, Y., Chen, Z., Hollert, H., Zhou, S., Deutschmann, B., and Seiler, T. B. (2019). Toxicity of 10 organic micropollutants and their mixture: Implications for aquatic risk assessment. Sci. Total Environ. 666, 1273–1282. doi: 10.1016/j.scitotenv.2019.02.047
Shen, J., Du, Z., Li, J., and Cheng, F. (2020). Co-metabolism for enhanced phenol degradation and bioelectricity generation in microbial fuel cell. Bioelectrochemistry 134, 107527. doi: 10.1016/j.bioelechem.2020.107527
Silva, G. G. Z., Green, K. T., Dutilh, B. E., and Edwards, R. A. (2016). SUPER-FOCUS: a tool for agile functional analysis of shotgun metagenomic data. Bioinformatics 32, 354–361. doi: 10.1093/bioinformatics/btv584
Simon, F. X., Rudé, E., Llorens, J., and Baig, S. (2013). Study on the removal of biodegradable NOM from seawater using biofiltration. Desalination 316, 8–16. doi: 10.1016/j.desal.2013.01.023
Snaidr, J., Amann, R., Huber, I., Ludwig, W., and Schleifer, K. H. (1997). Phylogenetic analysis and in situ identification of bacteria in activated sludge. Appl. Environ. Microbiol. 63, 2884–2896. doi: 10.1128/aem.63.7.2884-2896.1997
Stein, K., Ramil, M., Fink, G., Sander, M., and Ternes, T. A. (2008). Analysis and sorption of psychoactive drugs onto sediment. Environ. Sci. Technol. 42, 6415–6423. doi: 10.1021/es702959a
Su, L., Aga, D., Chandran, K., and Khunjar, W. O. (2015). Factors impacting biotransformation kinetics of trace organic compounds in lab-scale activated sludge systems performing nitrification and denitrification. J. Hazard. Mater. 282, 116–124. doi: 10.1016/j.jhazmat.2014.08.007
Ternes, T. A., Joss, A., and Siegrist, H. (2004). Peer reviewed: scrutinizing pharmaceuticals and personal care products in wastewater treatment. Environ. Sci. Technol. 38, 392A−399A. doi: 10.1021/es040639t
Tezel, U., Tandukar, M., Martinez, R. J., Sobecky, P. A., and Pavlostathis, S. G. (2012). Aerobic biotransformation of n-tetradecylbenzyldimethylammonium chloride by an enriched Pseudomonas spp. community. Environ. Sci. Technol. 46, 8714–8722. doi: 10.1021/es300518c
Torresi, E., Fowler, S. J., Polesel, F., Bester, K., Andersen, H. R., Smets, B. F., et al. (2016). Biofilm thickness influences biodiversity in nitrifying MBBRs—Implications on micropollutant removal. Environ. Sci. Technol. 50, 9279–9288. doi: 10.1021/acs.est.6b02007
Torresi, E., Gülay, A., Polesel, F., Jensen, M. M., Christensson, M., Smets, B. F., et al. (2018). Reactor staging influences microbial community composition and diversity of denitrifying MBBRs-implications on pharmaceutical removal. Water Res. 138, 333–345. doi: 10.1016/j.watres.2018.03.014
Torresi, E., Tang, K., Deng, J., Sund, C., Smets, B. F., Christensson, M., et al. (2019). Removal of micropollutants during biological phosphorus removal: Impact of redox conditions in MBBR. Sci. Total Environ. 663, 496–506. doi: 10.1016/j.scitotenv.2019.01.283
Tröger, R., Klöckner, P., Ahrens, L., and Wiberg, K. (2018). Micropollutants in drinking water from source to tap-method development and application of a multiresidue screening method. Sci. Total Environ. 627, 1404–1432. doi: 10.1016/j.scitotenv.2018.01.277
Vieno, N., and Sillanpää, M. (2014). Fate of diclofenac in municipal wastewater treatment plant—a review. Environ. Int. 69, 28–39. doi: 10.1016/j.envint.2014.03.021
Wolff, D., Krah, D., Dötsch, A., Ghattas, A. K., Wick, A., and Ternes, T. A. (2018). Insights into the variability of microbial community composition and micropollutant degradation in diverse biological wastewater treatment systems. Water Res. 143, 313–324. doi: 10.1016/j.watres.2018.06.033
Xu, Y., Yuan, Z., and Ni, B. J. (2017a). Biotransformation of acyclovir by an enriched nitrifying culture. Chemosphere 170, 25–32. doi: 10.1016/j.chemosphere.2016.12.014
Xu, Y., Yuan, Z., and Ni, B. J. (2017b). Impact of ammonium availability on atenolol biotransformation during nitrification. ACS Sustain. Chem. Eng. 5, 7137–7144. doi: 10.1021/acssuschemeng.7b01319
Yang, Y. Y., Toor, G. S., Wilson, P. C., and Williams, C. F. (2017). Micropollutants in groundwater from septic systems: transformations, transport mechanisms, and human health risk assessment. Water Res. 123, 258–267. doi: 10.1016/j.watres.2017.06.054
Zearley, T. L., and Summers, R. S. (2012). Removal of trace organic micropollutants by drinking water biological filters. Environ. Sci. Technol. 46, 9412–9419. doi: 10.1021/es301428e
Zhang, L., Carvalho, P. N., Bollmann, U. E., Brix, H., and Bester, K. (2019). Enhanced removal of pharmaceuticals in a biofilter: effects of manipulating co-degradation by carbon feeding. Chemosphere 236, 124303. doi: 10.1016/j.chemosphere.2019.07.034
Zhang, Y., Geißen, S. U., and Gal, C. (2008). Carbamazepine and diclofenac: removal in wastewater treatment plants and occurrence in water bodies. Chemosphere 73, 1151–1161. doi: 10.1016/j.chemosphere.2008.07.086
Zhao, X., Wang, Y., Xu, X., Tian, K., Zhou, D., Meng, F., et al. (2020). Genomics analysis of the steroid estrogen-degrading bacterium Serratia nematodiphila DH-S01. Biotechnol. Biotechnol. Equip. 34, 430–440. doi: 10.1080/13102818.2020.1764388
Keywords: sand filters, metagenome, micropollutants, biotransformation, ATP
Citation: Cao L, Wolff D, Liguori R, Wurzbacher C and Wick A (2022) Microbial Biomass, Composition, and Functions Are Responsible for the Differential Removal of Trace Organic Chemicals in Biofiltration Systems: A Batch Study. Front. Water 4:832297. doi: 10.3389/frwa.2022.832297
Received: 09 December 2021; Accepted: 28 January 2022;
Published: 22 February 2022.
Edited by:
Nhamo Chaukura, Sol Plaatje University, South AfricaReviewed by:
Yun Zhou, Huazhong Agricultural University, ChinaCopyright © 2022 Cao, Wolff, Liguori, Wurzbacher and Wick. This is an open-access article distributed under the terms of the Creative Commons Attribution License (CC BY). The use, distribution or reproduction in other forums is permitted, provided the original author(s) and the copyright owner(s) are credited and that the original publication in this journal is cited, in accordance with accepted academic practice. No use, distribution or reproduction is permitted which does not comply with these terms.
*Correspondence: Christian Wurzbacher, Y2hyaXN0aWFuQHd1cnpiYWNoZXIuY2M=
Disclaimer: All claims expressed in this article are solely those of the authors and do not necessarily represent those of their affiliated organizations, or those of the publisher, the editors and the reviewers. Any product that may be evaluated in this article or claim that may be made by its manufacturer is not guaranteed or endorsed by the publisher.
Research integrity at Frontiers
Learn more about the work of our research integrity team to safeguard the quality of each article we publish.