- 1Department of Agricultural Economics, Agricultural Sciences and Natural Resources University of Khuzestan, Mollasani, Iran
- 2Department of Agricultural Economics, Shiraz University, Shiraz, Iran
Today, with the decreased available water resources and the intensification of the conflicts between users of water resources, the cooperation or non-cooperation of users in water protection programs, especially in the agricultural sector, is of crucial importance. Therefore, the present study is aimed to provide a framework to evaluate the participatory behavior of farmers with optimal patterns of water allocation and to estimate the necessary budget to compensate for the reduction of water consumption in agricultural areas. To achieve this aim, the integration of the economic-environmental programming model with a top-down analytical approach and the agent-based model with a bottom-up analytical approach has been applied in the present study. Thus, the irrigation network of Jiroft Plain was chosen as the study area and a sample of farmers in this irrigation network was extracted for the analysis of the agent-based model using simple random method. It was found that different forms of insistence on the individual behavior of farmers make it difficult to participate in optimal water allocation patterns with simultaneously providing economic and environmental goals. As the degree of participation with the optimal model of water allocation with equal weight to economic and environmental goals, despite the implementation of the policy of giving facilities with a very low interest rate for the installation of new irrigation technologies, is estimated about 20%. Finally, the necessary budget for compensation policy for agricultural water storage was calculated based on the proposed framework, which can be considered a useful tool for policy makers in water resources management.
Introduction
Today, water resources scarcity has become a major limitation in the socio-economic development of societies and is considered as a threat to the livelihood of people in many parts of the world (Liu et al., 2017; Mirzaei et al., 2022b). With the increase in water consumption due to population growth, urbanization and economic growth, the awareness of stakeholders in environmental protection is also elevated (Sadegh and Kerachian, 2011; Kahil et al., 2015). The activities of stakeholders and users in considering nature are recognized as the main cause of the water resource crisis (AghaKouchak et al., 2015). The accurate assessment of water resources dynamics for the present and future, namely in semi-arid and arid regions, is not possible without considering human impacts (Vorosmarty et al., 2000; Alcamo et al., 2007). Thus, the evaluation and understanding the behavior of water beneficiaries and users has a great importance for recognition, development and improvement of decision-making processes in water resources management field (Anebagilu et al., 2021).
However, in past studies and conceptual frameworks, human systems have been considered mainly as a boundary condition with a linear thinking system toward integrated water resources management (IWRM), and their perception of the complex integrated dynamic relations between hydrological systems and human activities during long periods has not been adequate (Liu et al., 2008; Sivapalan et al., 2012; Pouladi et al., 2020). However, in order to make decisions about issues in which humans participate, such as water consumption management, factors such as identifying key stakeholders and behavioral rules and how stakeholders interact with each other and with the environment should be taken into account (Bonabeau, 2002). Therefore, the role of stakeholders and users of water resources as well as government institutions and water resource managers as decision makers in water allocation field is very important (Johansson et al., 2002; Orubu, 2006; Hanak and Lund, 2012; Farhadi et al., 2016; Hu et al., 2016). In recent years, several studies have investigated the dynamic role of stakeholder's activities in water resources management. These studies can be classified based on human water consumption (Lei et al., 2018), drought interactions with water extraction (Breyer et al., 2018), the relationship between floods and communities (Viglione et al., 2014; Ciullo et al., 2017) and water resources management in different sectors (Akhbari and Grigg, 2013; Farhadi et al., 2016; Hund et al., 2018; Pouladi et al., 2020; Mirzaei and Zibaei, 2021).
In general, mathematical programming models in different formats and considering technical, economic, hydrological and environmental aspects cannot alone be sufficient to manage water resources and evaluate the effects of adopting adaptive policies and strategies. Because in water resource management topic the discussion of participatory behavior of users in a prescriptive model or an optimal model is of great importance due to conflicting goals among users (Akhbari and Grigg, 2013; Mirzaei and Zibaei, 2021). Thus, in order to develop policies that are appropriate for stakeholders in water resources management field, it is necessary to link human behavior with mathematical programming models with different goals (Allred and Gary, 2019; Dessart et al., 2019; Granco et al., 2019).
Researchers have presented different types of models to optimize the water resources allocation, which can be considered in three main groups (Guo et al., 2022). The first group is based on the analysis of the water resources system, which is either as rational allocation of water resources through dynamic system models or as optimal allocation of water resources through the maximization of economic advantages. However, in such systems, one-dimensional view is dominant and it is difficult to describe the interaction between internal elements in these systems (Schulze et al., 2017; Tong et al., 2018). The second group is based on systems engineering theory with the goal of sustainable use of water resources. In these models, optimization goals are not limited to economic benefits, but environmental, social and water quality goals have also been included (Noël and Cai, 2017; Mirzaei et al., 2022a). In general, both groups of models have a top-down process (Schlüter et al., 2017; Guo et al., 2022), which one cannot be optimistic about the success of water resources management without careful adjustment from a bottom to up process (Guo et al., 2022). Therefore, the third group of models is based on a bottom-up process and these types of models evaluate the interrelationship of agents (stakeholders) and the environment involved in water resources management (Jepsen et al., 2006; Groeneveld et al., 2017; Mirzaei and Zibaei, 2021; Guo et al., 2022).
Agent-based models that simulate the internal behavior of agents in a system can be appropriate models for analyzing and investigating the behavior of stakeholders and the decision-making process in water resources saving issue. These models evaluate the interaction effects between agents as well as their interaction effects with the environment. The first economic agent-based model was presented by Schelling (1971) in the field of analyzing agents' preferences for selecting a living place. Although the agent-based modeling was first used in computer simulations (An, 2012), in recent years, the agent-based model has been used in different studies. The agent-based model is used in the agricultural sector to analyze farmers' behavior according to economic goals (Guillem et al., 2015; Mirzaei and Zibaei, 2021), environmental (Heckbert et al., 2010), socio-hydrological (Pouladi et al., 2019) and political development (Happe et al., 2006; Brady et al., 2012; Granco et al., 2019). One of the strengths of agent-based model is to provide scientific formulas in the form of soft sentences that are understandable for all beneficiaries.
Various studies have been performed using the agent-based model in the field of the analysis of the participatory behavior of farmers with policies and plans for water resources saving (Akhbari and Grigg, 2013; Farhadi et al., 2016; Mirzaei and Zibaei, 2021; Guo et al., 2022). The main structure of the agent-based model of the present study is different from the study of Guo et al. (2022) and is consistent with the studies of Akhbari and Grigg (2013), Farhadi et al. (2016) and Mirzaei and Zibaei (2021). In these studies, the optimal model has extracted based on the top-down process, the participatory behavior of farmers based on the extractive patterns have investigated through the proposed agent-based model and in the present study, such approach was also used for agent-based analysis. However, the different aspect this study is the evaluation of the degree of compensation to farmers for water saving, which was only considered in the study of Guo et al. (2022) and this evaluation can help policy makers in accurate budget planning of water resources in different regions. Therefore, the current study can be considered comprehensive research due to the integration of studies in this field, which can be very useful in operationalizing water resources management policies.
To investigate the proposed model of this study, the farmers of Jiroft Plain irrigation network in Kerman province were considered. The location of this irrigation network is depicted in Figure 1. The Jiroft plain located in Kerman province is considered one of the main areas of agricultural production in Iran, and high amount of water in the Jazmurian wetland located downstream of this plain can make a considerable contribution to the reduction of fine dust in the province and even in Iran (Mirzaei and Zibaei, 2021). The extraction amount of surface and groundwater resources of this plain for agricultural sector is 188.4 and 882.6 million cubic meters, respectively, which has caused a decrease in the level of groundwater resources and Halil River (Regional Water Company of Kerman province in Iran, 2018). Therefore, it is of great importance to investigate the participatory behavior of farmers in this area with water saving policy.
Methodology
To achieve the aim of the present study, the integration of the a two-objective linear mathematical programming model with a top-down analytical approach and the agent-based model with a bottom-up analytical approach has been applied in the present study.
Mathematical programming model with economic-environmental objectives
The current study model to extract an optimal model is a two-objective linear mathematical programming model with the aim of maximizing farmers' gross margin and minimizing water consumption, where the main objectives and constraints are defined as followings:
Equations 1, 2 show the goal of maximizing the gross margin and minimizing the water consumed in the given irrigation network. Equations 3, 4 also show the constraints of the model, including the limitation of available land and capital for installing modern irrigation systems. The parameters of the model include trc,r, the income of each product unit (c) with irrigation technology (r) per hectare, tcc,r, the production cost of each product unit (c) with irrigation technology (r) per hectare, wdc, the net water demand of the product (c) per hectare, efr efficiency of irrigation technology (r), ir, the cost of installing irrigation technology (r) per hectare and the variables of the model include Xc,r, the decision variable of the area under cultivation (c) with irrigation technology (r), Z1, the gross margin variable, and Z2, the water consumption variable.
The two-objective model of the present study was solved using the augmented ε-constraint method and was extracted the set of optimal and efficient solutions. Among the Pareto efficient solutions, the best solution can be selected according to the opinions and views of the decision-makers and stakeholders, and the TOPSIS method has been used for this purpose.
Proposed agent-based model
In the present study, the optimal water allocation models extracted from the economic-environmental programming model are recognized as the environment of the agent-based model, and these models are shared with the farmers who are the agents of the model. Figure 3 shows the structure of the proposed agent-based model of the present study. As is shown in Figure 3, at first, farmers' decisions are based on comparing the amount of current water demand (WD) and the amount of allocated water through the model (AW). Thus, farmers with current water demand more than the allocated water reject the extractive model and farmers with current water demand less than or equal to the allocated water participate with the extractive model. Then, the effects of the social pressures of cooperative and no-cooperative farmers on each other are investigated and the changes in farmers' behavior are concluded. The study of Mirzaei and Zibaei (2021) was applied to calculate social pressures. In this way, to calculate the social pressure of no-cooperative farmers, the ratio of the population of no-cooperative farmers to the total population is used, and to calculate the social pressure of cooperative farmers, the ratio of the population of cooperative farmers to the total population is used (Mirzaei and Zibaei, 2021). In the third stage, the government's motivational policies and solutions are evaluated in order to encourage the cooperative farmers to continue their behavior and motivate no-cooperative farmers to change their behavior. For this purpose, it is necessary to extract the utility functions of farmers with and without the government's motivational solutions that utility function of farmers was considered as follows: (Akhbari and Grigg, 2013):
Values A, B, C and D according to incentive policies were obtained by using farmers-addressed questionnaire (Figure 2):
“A” is the percentage of total agricultural water demands that is much less than their demands and will not satisfy them at all if being allocated to them.
“B” is the MINIMUM percentage of total agricultural water demands that completely meets their demands and satisfies them if being allocated to them.
“C” is the MAXIMUM percentage of total agricultural water demands that completely satisfies them if being allocated to them (it can be less or more than %100).
“D” is the percentage of total agricultural water demands that is much more than their actual demands and if it is allocated to them, it may damage their crops and make them unsatisfied (it should be more than 100%).
Finally, the compensation policy for water saving is evaluated to accept the optimal patterns by all the farmers in the study area. The compensation per hectare is calculated by the product of the economic productivity of each cubic meter of water consumption with the amount of difference between the water current demand and the allocated optimum, and then the total amount of compensation to farmers for water saving and achieving the goals of optimal extraction model is estimated.
In order to solve the two-objective economic-environmental model of Jiroft plain irrigation network, the data of the price, yield, production cost and water cost per hectare have been obtained from the Jihad Agricultural Organization in the south of Kerman province. Also, the data related to the water demand of crops, the cost of installing modern irrigation systems and the amount of water supply in different channels of the irrigation network have been extracted from the regional water company of Kerman province. In the end, in order to solve the agent-based model, a sample of 82 farmers was extracted from the population of farmers of Jiroft irrigation network using a simple random sampling method and the relevant information was obtained through a questionnaire to solve the model. It is worth to mention that to calculate the sample size, information about the given population is required. In this analysis, the population consists of all the agricultural users in the Jiroft plain irrigation network, whose information was extracted from the general agricultural census of 2013 in terms of the villages of this region (Program and Budget Organization of Kerman Province, 2015).
Results and discussion
Results of economic-environmental programming model
In the present study, the irrigation network of Jiroft plain is considered as the studied area and optimal cultivation patterns with economic and environmental (saving and conservation of water resources) objectives with different importance weights through the combination of augmented-ε-constraint mathematical programming method and TOPSIS multi-criteria decision-making method were extracted (Table 1).
The first optimal pattern (opt1) with the equal weight of economic and environmental objectives (50% of the importance of economic and environmental objectives) showed that by planting about 14,130 hectares of land and planting two crops of wheat (sprinkler irrigation) by 6% and cucumber (drip irrigation) by 94%, the gross margin is equal to 859.76 dollars/hectare is obtained from water consumption of 5,872.34 cubic meters/hectare that these changes can lead to the improvement of the water economic productivity from 0.084 to 0.146 dollars per cubic meter. Therefore, the farmer's gross margin will decrease by about 18% and water consumption by about 53% compared to the current conditions, which results into the improvement of the water economic productivity equal to 74%. The results of Table 1 showed that in the second optimal pattern (opt2) with a greater weight of the economic objective than the environmental objective (75% and 25% of the importance of the economic and environmental objectives, respectively), the farmer's gross margin will increase by about 27% and water consumption will decrease by about 22% compared to the current conditions, and these results improve the water economic productivity by about 63%. In order to achieve these objectives in the second optimal model, it is required that the 17,968 hectares of land be dedicated to the cultivation of two crops, wheat (sprinkler irrigation) by 5% and tomato (drip irrigation) by 95%. The results of the third optimal pattern (opt3) with a higher weight of the environmental objective compared to the economic objective (75 and 25% of the importance of the environmental and economic objectives, respectively) indicated that only 3,725 hectares of land, equivalent to 21% of the total land, are applied for agriculture crops plantation. This means that under water crisis conditions and when the importance of mitigating water consumption and its saving is three times more than the economic importance of farmers, it is required not to plant a large area of agricultural land. The results indicate that in order to achieve the objectives of the third optimal model, by cultivating about 3,725 hectares of land and planting two crops of wheat (sprinkler irrigation) by 24% and cucumber (drip irrigation) by 76%, the gross margin equal to 652.25 dollars/ hectare of water consumption equivalent to 4,456.35 cubic meters/hectare is achieved, and these changes can lead to the improvement of the water economic productivity from 0.084 to 0.146 dollars per cubic meter. In this model, gross margin and water consumption are reduced by 37 and 64%, respectively, compared to the current conditions. These changes can lead to a 74% improvement in the water economic productivity compared to the current conditions.
In general, the results showed that the cultivation pattern and the degree of use of modern irrigation technologies are not optimal in the current conditions, and, better conditions can be provided in the field of water resource management and improving the water economic productivity. However, in order to achieve the goals of optimal patterns and implement these patterns at the farms level, it is necessary to evaluate the participatory behavior of farmers with each of these patterns. Therefore, the calculation of improving water economic productivity and extracting the optimal cultivation pattern alone cannot result in the efficient management of water resources, and it is necessary for the government to implement various policies to encourage farmers to adopt the optimal patterns via analyzing the behavior and understanding the utility functions of farmers.
Results of agent-based model
First, by comparing the current water demand with the allocated water in each optimal extraction pattern, the cooperation and non-cooperation of 82 sample farmers in the irrigation network of Jiroft plain was estimated, and then the social impacts of the farmers on each other were investigated, the results of which are illustrated in Table 2.
Based on the results of Table 2, without considering social pressures, the cooperation rate with the first, second and third optimal patterns is about 13, 46, and 4%, respectively. After calculation of the social pressures, the cooperation rate for all patterns decreased to 0, 41, and 0%, respectively, which show the dominance of non-cooperative thinking in the Jiroft Plain irrigation network. Also, we can be optimistic for the implementation and operation of the second optimal pattern with a lower cost. In the second optimal pattern, the entire land is dedicated to the cultivation of crops and it is only necessary to encourage the farmers toward the optimal cultivation pattern and to adopt modern irrigation technologies. However, encouraging farmers to adopt the third pattern is difficult and expensive, as no farmer will participate with this pattern after considering the social pressures. In this pattern, only about 21% of land will be dedicated to crop cultivation, and as a result, encouraging some farmers to cultivate the crops, and persuading the active farmers to change the cultivation pattern and adoption of modern technologies will impose a much higher cost on the government. Therefore, in general, the implementation of optimal economic-environmental patterns will not be actualized considering current state of water consumption in the Jiroft plain irrigation network, and it is necessary for the government to provide solutions and policies to persuade more farmers to adopt the economic-environmental patterns. In this regard, in order to encourage farmers to adopt optimal patterns, the incentive policies of granting facilities with different interest rates for the installation and operation of modern irrigation technologies were evaluated (Figure 4).
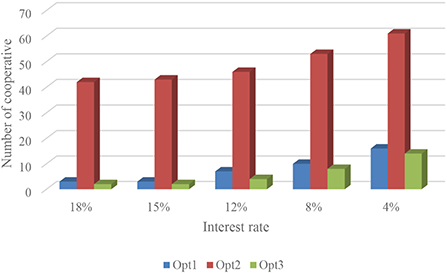
Figure 4. The amount of participation of farmers with optimal water allocation patterns by using the policy of granting facilities.
Granting facilities with an interest rate of 18% (equivalent to the major interest rate of bank loans in Iran), in order to install new irrigation technologies, can only encourage 3, 8 and 2 farmers to participate in the first, second and third optimal patterns, respectively. The reduction of the interest rate of loans granted to farmers will lead to an increase of cooperative farmers for all patterns. Thus, the interest rate of 4% (equivalent to the lowest interest rate of loans in Iran) compared to the interest rate of 18%, encourages 13, 19 and 12 farmers to accept the first, second and third optimal patterns, respectively. Therefore, according to the results obtained, it can be said that by granting facilities to farmers to install new irrigation technologies with the lowest interest rate of loans in Iran (4 percent rate), the cooperative rate of the present sample farmers in the first, second and third optimal patterns will be about 20, 74, and 17%, respectively. This finding means that can only hope for the implementation of the second optimal pattern and not completely (more than 70%) via the incentive policy of granting facilities with low interest rates.
Therefore, for water saving to be successful in the study area, the government should compensate the farmers for water saving, which of course will be the prerequisite for the adoption of this policy, the installation of smart meters in farms. Thus, the compensation cost per hectare is calculated by using the product of the water economic productivity in the current conditions with the difference between the water consumption and the optimal amount allocated, and then the total amount of compensation for each pattern was estimated (Table 3). It was found that the minimum and maximum calculation budget in the present study for saving water resources and adopting optimal economic-environmental patterns in the irrigation network level of Jiroft plain are about 4.15 and 12.06 million dollars, respectively.
Conclusion and suggestions
The present study presents a new structure to evaluate the operationalization of optimal patterns of water allocation at farms level. This study, by considering the top-down and bottom-up analytical processes simultaneously, can provide a new solution for saving water resources in different regions. As shown in the present study, improving the water economic productivity is not possible without persuading farmers. The results of the present study showed that even encouraging farmers to accept modern irrigation systems through granting facilities with an interest rate of 4% (the lowest interest rate of facilities in Iran) still cannot guarantee the use of optimal patterns at the regional level. This means that without spending money and compensation policy for water storage, we cannot be optimistic for efficient management of water resources in the study area. The calculations made in the study showed that the government should consider a budget of about 231 dollars to implement the most applicable optimal pattern in the irrigation network of Jiroft Plain (the model with the importance weight of 75 and 25% for economic and environmental objectives, respectively) per hectare to compensate the farmers for water saving, which is estimated at 4.15 million dollars for 17,968 hectares of the fields of this irrigation network. However, with this budget, we can hope for a 22% reduction in water consumption in the region. In this way, in order to save more water resources by farmers, the amount of compensation will be increased. Therefore, the main finding of the present study is that we cannot be optimistic for the success of optimal patterns of water allocation at the farms level without the analysis of the farmers' behavior and the government's motivational strategies. Hence, it is suggested that the analytical and computational structure of the present study be used for water resources management in different regions. In this study, the behavior participation of farmers and the amount of budget required to reduce water consumption have been discussed. But, it is suggested that in future studies, sustainability concepts related to the relationship between water, food, and energy should be pursued in the form of an agent-based model. It is also important to mention that since the agent-based model is a mental model, some of the answers did not match the real world and therefore the researchers had to discard some of the questionnaires. Thus, the status of farmers' utility functions should be carefully examined. In addition, due to different attitudes, subjective norms, and social pressure, the results of this study cannot be generalized to other regions. However, this issue does not reduce the value of the comprehensive framework introduced in this study.
Data availability statement
The original contributions presented in the study are included in the article/supplementary material, further inquiries can be directed to the corresponding author.
Ethics statement
Ethical review and approval was not required for the study on human participants in accordance with the local legislation and institutional requirements. Written informed consent from the participants was not required to participate in this study in accordance with the national legislation and the institutional requirements.
Author contributions
Design, conceptualization, supervision, and final writing: AM and HA. Methodology and data analysis: AM. All authors contributed to the article and approved the submitted version.
Conflict of interest
The authors declare that the research was conducted in the absence of any commercial or financial relationships that could be construed as a potential conflict of interest.
Publisher's note
All claims expressed in this article are solely those of the authors and do not necessarily represent those of their affiliated organizations, or those of the publisher, the editors and the reviewers. Any product that may be evaluated in this article, or claim that may be made by its manufacturer, is not guaranteed or endorsed by the publisher.
References
AghaKouchak, A., Feldman, D., Hoerling, M., Huxman, T., and Lund, J. (2015). Water and climate: Recognize anthropogenic drought. Nature 524, 409–411. doi: 10.1038/524409a
Akhbari, M., and Grigg, N. S. (2013). A framework for an agent-based model to manage water resources conflicts. Water Resour. Manag. 27, 4039–4052. doi: 10.1007/s11269-013-0394-0
Alcamo, J., Flörke, M., and Märker, M. (2007). Future long-term changes in global water resources driven by socio-economic and climatic changes. Hydrol. Sci. J., 52, 247–275. doi: 10.1623/hysj.52.2.247
Allred, S., and Gary, G. (2019). Riparian landowner decision-making in the context of flooding: an application of the theory of planned behavior. Environ. Syst. Decis. 39, 396–408. doi: 10.1007/s10669-019-09735-1
An, L. (2012). Modeling human decisions in coupled human and natural systems: Review of agent-based models. Ecol. Modell., 229, 25–36. doi: 10.1016/j.ecolmodel.2011.07.010
Anebagilu, P. K., Dietrich, J., Prado-Stuardo, L., Morales, B., Winter, E., Arumi, J. L., et al. (2021). Application of the theory of planned behavior with agent-based modeling for sustainable management of vegetative filter strips. J. Environ. Manage. 284, 112014. doi: 10.1016/j.jenvman.2021.112014
Bonabeau, E. (2002). Agent-based modelling, methods and techniques for simulating human systems. Proc. Natl. Acad. Sci. 99, 7280–7287. doi: 10.1073/pnas.082080899
Brady, M., Sahrbacher, C., Kellermann, K., and Happe, K. (2012). An agent-based approach to modeling impacts of agricultural policy on land use, biodiversity and ecosystem services. Landsc. Ecol. 27, 1363–1381. doi: 10.1007/s10980-012-9787-3
Breyer, B., Zipper, S. C., and Qiu, J. (2018). Socio-hydrological impacts of water conservation under anthropogenic drought in Austin, TX (USA). Water Resour. Res. 54, 3062–3080. doi: 10.1002/2017WR021155
Ciullo, A., Viglione, A., Castellarin, A., Crisci, M., and Baldassarre, D. G. (2017). Socio-hydrological modelling of flood-risk dynamics: comparing the resilience of green and technological systems. Hydrol. Sci. J.2, 880–891. doi: 10.1080/02626667.2016.1273527
Dessart, F. J., Barreiro-Hurl,é, J., and van Bavel, R. (2019). Behavioural factors affecting the adoption of sustainable farming practices: a policy-oriented review. Eur. Rev. Agric. Econ. 46, 417–471. doi: 10.1093/erae/jbz019
Farhadi, S., Nikoo, M. R., Rakhshandehroo, G. R., Akhbari, M., and Alizadeh, M. R. (2016). An agent-based-Nash modeling framework for sustainable groundwater management: a case study. Agric. Water Manag., 177, 348–358. doi: 10.1016/j.agwat.2016.08.018
Granco, G., Stamm, J. L. H., Bergtold, J. S., Daniels, M. D., Sanderson, M. R., Sheshukov, A. Y., et al. (2019). Evaluating environmental change and behavioral decision-making for sustainability policy using an agent-based model: a case study for the Smoky Hill River Watershed, Kansas. Sci. Total Environ. 695, 133769. doi: 10.1016/j.scitotenv.2019.133769
Groeneveld, J., Müller, B., Buchmann, C. M., Dressler, G., Guo, C., Hase, N., et al. (2017). Theoretical foundations of human decision-making in agent-based land use models–a review. Environ. Modell. Softw. 87, 39–48. doi: 10.1016/j.envsoft.2016.10.008
Guillem, E. E., Murray-Rust, D., Robinson, D. T., Barnes, A., and Rounsevell, M. D. A. (2015). Modelling farmer decision-making to anticipate tradeoffs between provisioning ecosystem services and biodiversity. Agric. Syst. 137, 12–23. doi: 10.1016/j.agsy.2015.03.006
Guo, N., Shi, C., Yan, M., Gao, X., and Wu, F. (2022). Modeling agricultural water-saving compensation policy: an ABM approach and application. J. Clean. Prod. 344, 131035. doi: 10.1016/j.jclepro.2022.131035
Hanak, E., and Lund, J. R. (2012). Adapting California's water management to climate change. Clim. Change. 111, 17–44. doi: 10.1007/s10584-011-0241-3
Happe, K., Kellermann, K., and Balmann, A. (2006). Agent-based analysis of agricultural policies: an illustration of the agricultural policy simulator AgriPoliS its adaptation and behavior. Ecol. Soc. 11, 49. doi: 10.5751/ES-01741-110149
Heckbert, S., Baynes, T., and Reeson, A. (2010). Agent-based modeling in ecological economics. Ann. N. Y. Acad. Sci. 1185, 39–53. doi: 10.1111/j.1749-6632.2009.05286.x
Hu, Z., Wei, C., Yao, L., Li, C., and Zeng, Z. (2016). Integrating equality and stability to resolve water allocation issues with a multi-objective bi-level programming model. J. Water Resour. Plan. Manag. 142, 1–12. doi: 10.1061/(ASCE)WR.1943-5452.0000640
Hund, S. V., Allen, D. M., Morillas, L., and Johnson, M. S. (2018). Groundwater recharge indicator as tool for decision makers to increase socio-hydrological resilience to seasonal drought. J. Hydrol. 563, 1119–1134. doi: 10.1016/j.jhydrol.2018.05.069
Jepsen, M. R., Leisz, S., Rasmussen, K., Jakobsen, J., MØller-Jensen, L., and Christiansen, L. (2006). Agent-based modelling of shifting cultivation field patterns, Vietnam. Int. J. Geogr. Inf. Sci. 20, 1067–1085. doi: 10.1080/13658810600830848
Johansson, R. C., Tsur, Y., Roe, T. L., Doukkali, R., and Dinar, A. (2002). Pricing irrigation water: a review of theory and practice. Water Policy 4, 173–199. doi: 10.1016/S1366-7017(02)00026-0
Kahil, M. T., Dinar, A., and Albiac, J. (2015). Modelling water scarcity and droughts for policy adaptation to climate change in arid and semiarid regions. J. Hydrol. 522, 95–109. doi: 10.1016/j.jhydrol.2014.12.042
Lei, X., Zhao, J., Wang, D., and Sivapalan, M. (2018). A Budyko-type model for human water consumption. J. Hydrol. 567, 212–226. doi: 10.1016/j.jhydrol.2018.10.021
Liu, J., Yang, H., Gosllng, S. N., Kummu, M., Florke, M., Pfister, S., et al. (2017). Water scarcity assessments in the past, present, and future. Earths Future 5, 545–559. doi: 10.1002/2016EF000518
Liu, Y., Gupta, H., Springer, E., and Wagener, T. (2008). Linking science with environmental decision making: experiences from an integrated modeling approach to supporting sustainable water resources management. Environ. Model. Softw. 23, 846–858. doi: 10.1016/j.envsoft.2007.10.007
Mirzaei, A., Abdeshahi, A., Azarm, H., and Naghavi, S. (2022a). New design of water-energy-food-environment nexus for sustainable agricultural management. Stoch. Environ. Res. Risk Assess. 36, 1861–1874. doi: 10.1007/s00477-021-02131-9
Mirzaei, A., Azarm, H., and Naghavi, S. (2022b). Optimization of the cropping pattern under seasonal fluctuations of surface water using multistage stochastic programming. Water Supply 22, 5716–5728. doi: 10.2166/ws.2022.224
Mirzaei, A., and Zibaei, M. (2021). Water conflict management between agriculture and wetland under climate change: application of economic-hydrological-behavioral modelling. Water Resour. Manag. 35, 1–21. doi: 10.1007/s11269-020-02703-4
Noël, P. H., and Cai, X. (2017). On the role of individuals in models of coupled human and natural systems: lessons from a case study in the Republican River Basin. Environ. Model. Softw. 92, 1–16. doi: 10.1016/j.envsoft.2017.02.010
Orubu, C. O. (2006). Water resources, environment and sustainable development in Nigeria. J. Hum. Ecol. 19, 169–181. doi: 10.1080/09709274.2006.11905875
Program and Budget Organization of Kerman Province. (2015). The Results of the Agricultural General Census in Iran.
Pouladi, P., Afshar, A., Afshar, M. H., Molajou, A., and Farahmand, H. (2019). Agent-based socio-hydrological modeling for restoration of Urmia Lake: application of theory of planned behavior. J. Hydrol. 576, 736–748. doi: 10.1016/j.jhydrol.2019.06.080
Pouladi, P., Afshar, A., Molajou, A., and Afshar, M. H. (2020). Socio-hydrological framework for investigating farmers' activities affecting the shrinkage of Urmia Lake; hybrid data mining and agent-based modelling. Hydrol. Sci. J. 65, 1249–1261. doi: 10.1080/02626667.2020.1749763
Regional Water Company of Kerman province in Iran. (2018). Jiroft: Water Resources Basic Studies Office.
Sadegh, M., and Kerachian, R. (2011). Water resources allocation using solution concepts of fuzzy cooperative games: fuzzy least core and fuzzy weak least core. Water Resour. Manag. 25, 2543–2573. doi: 10.1007/s11269-011-9826-x
Schelling, T. C. (1971). Dynamic models of segregation. J. Math. Sociol. 1, 143–186. doi: 10.1080/0022250X.1971.9989794
Schlüter, M., Baeza, A., Dressler, G., Frank, K., Groeneveld, J., Jager, W., et al. (2017). A framework for mapping and comparing behavioural theories in models of social-ecological systems. Ecol. Econ. 131, 21–35. doi: 10.1016/j.ecolecon.2016.08.008
Schulze, J., Müller, B., Groeneveld, J., and Grimm, V. (2017). Agent-based modelling of social-ecological systems: achievements, challenges, and a way forward. J. Artif. Soc. Soc. Simul. 20, 8. doi: 10.18564/jasss.3423
Sivapalan, M., Savenije, H. H., and Blöschl, G. (2012). Socio-hydrology: a new science of people and water. Hydrol. Process 26, 1270–1276. doi: 10.1002/hyp.8426
Tong, X., Nikolic, I., Dijkhuizen, B., van den Hoven, M., Minderhoud, M., Wäckerlin, N., et al. (2018). Behavior changes in post-consumer recycling: applying agent-based modelling in social experiment. J. Clean. Prod. 187, 1006–1013. doi: 10.1016/j.jclepro.2018.03.261
Viglione, A., Baldassarre, D. I., Brandimarte, G., Kuil, L., Carr, L., Salinas, G., et al. (2014). Insights from socio-hydrology modelling on dealing with flood risk–roles of collective memory, risk-taking attitude and trust. J. Hydrol. 518, 71–82. doi: 10.1016/j.jhydrol.2014.01.018
Keywords: water use conflict, farmers' behavior, economic-environmental programming model, agent-based model, agricultural water-saving
Citation: Mirzaei A and Azarm H (2022) Evaluation of farmers' participatory behavior and compensation policy for agricultural water-saving. Front. Water 4:1005821. doi: 10.3389/frwa.2022.1005821
Received: 28 July 2022; Accepted: 15 August 2022;
Published: 31 August 2022.
Edited by:
Negin Fallah Haghighi, Iranian Research Organization for Science and Technology, IranReviewed by:
Masoud Bijani, Tarbiat Modares University, IranSomayeh Naghavi, University of Jiroft, Iran
Ghasem Layani, Shahrekord University, Iran
Copyright © 2022 Mirzaei and Azarm. This is an open-access article distributed under the terms of the Creative Commons Attribution License (CC BY). The use, distribution or reproduction in other forums is permitted, provided the original author(s) and the copyright owner(s) are credited and that the original publication in this journal is cited, in accordance with accepted academic practice. No use, distribution or reproduction is permitted which does not comply with these terms.
*Correspondence: Abbas Mirzaei, YW1pcnphZWlAYXNucnVraC5hYy5pcg==