- 1Institute of Food Safety, Animal Health and Environment (BIOR), Riga, Latvia
- 2Bioinformatics Research Unit, Rīga Stradiņš University, Riga, Latvia
- 3Department of Scientific Research, SIA net-OMICS, Riga, Latvia
Background: Biofilms, when formed on the surfaces of water pipes, can be responsible for a wide range of water quality and operational problems. We sought to assess the bacterial and free-living protozoa (FLP) diversity, in relation to the presence of Legionnaire's disease-causing bacteria Legionella pneumophila (L. pneumophila) in 45 biofilms of hot water distribution system pipes of apartment buildings in Riga, the capital city of Latvia.
Results: 16S rRNA amplicon sequencing (metataxonomics) revealed that each biofilm contained 224 rather evenly distributed bacterial genera and that most common and most abundant were two genera, completely opposites in terms of their oxygen requirements: the obligately anaerobic Thermodesulfovibrio and the strictly aerobic Phenylobacterium. Water temperature and north-south axis (i.e., different primary water sources) displayed the most significant effect on the inter-sample variations, allowing us to re-construct three sub-networks (modules) of co-occurring genera, one involving (potentially FLP-derived) Legionella spp. Pangenome-based functional profile predictions suggested that all three may be dominated by pathways related to the development and maintenance of biofilms, including quorum sensing and nutrient transport, as well as the utilization of various energy sources, such as carbon and nitrogen. In our 18S rRNA amplicon sequencing data, potential hosts of L. pneumophila were detected in 11 out of 12 biofilm samples analyzed, however, in many cases, their relative abundance was very low (<1%). By validating our findings using culture-based methods, we detected L. pneumophila (serogroups 2, 3, 6 and 9) in nine (20%) biofilms, whereas FLP (mostly Acanthamoeba, Vahlkampfidae and Vermamoeba spp.) were present in six (~13%) biofilms. In two biofilms, L. pneumophila and its potential hosts were detected simultaneously, using culture-based methods.
Conclusions: Overall, our study sheds light on the community diversity of hot water biofilms and predicts how several environmental factors, such as water temperature and source might shape it.
Background
Biofilms represent collections of microorganisms embedded within a slimy matrix, where they interact with each other, in order to better adapt themselves to different environmental factors and survive (Mahapatra et al., 2015). In fact, the majority of bacteria in water supply systems are known to occur in biofilms rather than in water phase (Mahapatra et al., 2015). Domestic water pipe biofilms can be responsible for a wide range of water quality and operational problems, as microorganisms in biofilms tend to display more resistance to antibiotics and disinfecting agents. Thus, biofilms represent a potential reservoir for pathogens, including several genera that belong to the bacterial phyla Proteobacteria (e.g., genera Pseudomonas, Klebsiella, Aeromonas), Actinobacteria, Firmicutes, Verrucomicrobia, Nitrospirae and Bacteroidetes (Liu et al., 2013), whereof Firmicutes are the dominant gram-positive bacteria (Wolf et al., 2004) and Proteobacteriare present the major phylum of gram-negative bacteria (Vaz-Moreira et al., 2017). Proteobacteria spp. include also the well-known respiratory pathogen—L. pneumophila—that can be transmitted to humans via inhalation of contaminated water droplets, causing the Legionnaires' disease (Abu Khweek and Amer, 2018).
Whether Legionella spp persist freely within biofilms growing to high numbers or within various free-living protozoan (FLP) hosts is still controversial and may be dynamic influenced by many poorly understood factors, such as water temperature, availability of bacterial prey or presence of potential FLP hosts, as well as the concentration of Legionella spp, as, when reaching high concentrations in their hosts, Legionella may be released in different forms, including free cells, cells within biofilm fragments, or secreted vesicles. Moreover, during its life cycle, L. pneumophila switch between various replicative, infectious and dormant forms, as an adaptation to environmental changes, thereof FLP hosts are mainly required for their replication. Warm water temperatures, stagnant water with excess nutrients, lack of chemical disinfectants, as well as certain surface materials are some of the conditions that promote the growth of and influence the complex interactions within biofilms, including their associated FLP, and consequently Legionella spp. (Academies, 2019).
The drinking water in Riga is supplied from both surface and groundwater sources. In the framework of the Riga Water and Environment Project (in 2001), primary chlorination has been largely replaced by ozonation and biofiltration (Juhna and Klavinš, 2001; Springe and Juhna, 2007), before distributing water to the end point users. Of note, chlorination is still used to some extent, but the concentration of chlorine has been reduced from 0.5 mg −1 to 0.2 mg l−1. Nevertheless, since 2016, high concentration chlorination (0.5 mg l−1) is being used for three consecutive days every second year to thoroughly disinfect the drinking water supply system. Hence, after leaving the water treatment plant, its microbial level should be within the limits set by the respective water authorities [https://www.rigasudens.lv/en/]. On the other hand, although such treatments substantially reduce the levels of microbes in water, the surviving microorganisms might grow again, if the conditions would be favorable. Therefore, by the time water reaches end point users, its quality may differ dramatically from that at the time of treatment, depending on e.g., the water flow rate, as well as pipe age and material of water distribution systems (Mahapatra et al., 2015). According to the data of the Center of Disease Control and Prevention in Latvia [https://www.spkc.gov.lv/en/], in the period from 2015 to 2019, there have been, on average, 31.2 confirmed human infection cases of Legionnaires' disease per year. In 2011 there were 30 legionellosis cases in Latvia. From those, four cases were likely to have been infected abroad, but, in 12 cases, Legionella spp. were found in the water-supply systems of the patients' heating units of the apartment houses or taps and showers of flats (Rozentale et al., 2011). In 2014, there was a study carried out on the seroprevalence against Legionella pneumophila among the Latvian blood donors (n = 2007). In total, 4.8% of samples were positive for L. pneumophila SG 1-6. The centralized hot water supply system was determined as one of the main risk factors, associated with this seropositivity (Valciņa et al., 2015). In another study, in 2014, in 27% of the dental practices, L. pneumophila have been found in the water taps or the dental unit waterline (Valciņa et al., 2014). Overall, from 2008 to 2017, Latvia has reported 231 community-acquired cases and zero healthcare-associated cases (Beauté et al., 2020).
In this study, we sought to assess the bacterial and FLP diversity and re-construct the possible co-occurrence networks within 45 biofilms of hot water pipes collected from several apartment buildings in Riga, the capital city of Latvia, with a focus on to the presence of L. pneumophila.
Methods
Sample Collection
Forty-five biofilm samples were collected from the inner surface of pipes in the hot water distribution systems of 43 (i.e., two buildings had replicates) apartment buildings in Riga (the capital of Latvia), during the repair works in May and June 2017. We also collected additional environmental information, i.e., water temperature in the respective pipe, the age and type of the pipe, as well as the age of the respective building, as summarized in Table 1 and Supplementary Table S1. All pipes were made from galvanized steel and the age of the pipe system ranged from 11 to 60 years, whereas the measured water temperature ranged from 37 to 57°C. All samples were collected from inhabited buildings and apartments, therefore, regular circulation of water in the sampled pipes can be assumed. Water temperature was measured at the time of sampling with a contact thermometer at the outer surface of the pipe. The age of the apartment buildings varied between 27 and 128 years and the floor of the building, from which the biofilm samples were collected, ranged from the basement to the 14th floor. Collected pipes were delivered to the laboratory in sealed bags within the same day to prevent their drying out, which might have compromised the viability of microorganisms. In the laboratory, the pipes were cut into five cm long pieces, using an angle grinder and the biofilm material was carefully removed with a scalpel from the inside of each pipe fragment.The collected material was gently ground with a sterile mortar and pestle to break up the larger pieces and thoroughly mix each sample before dividing into aliquots for separate analyses. The resulting material consisted of either moist powder or solid particles, which visually appeared to contain some amount of corrosion products (see Supplementary Figure S1).
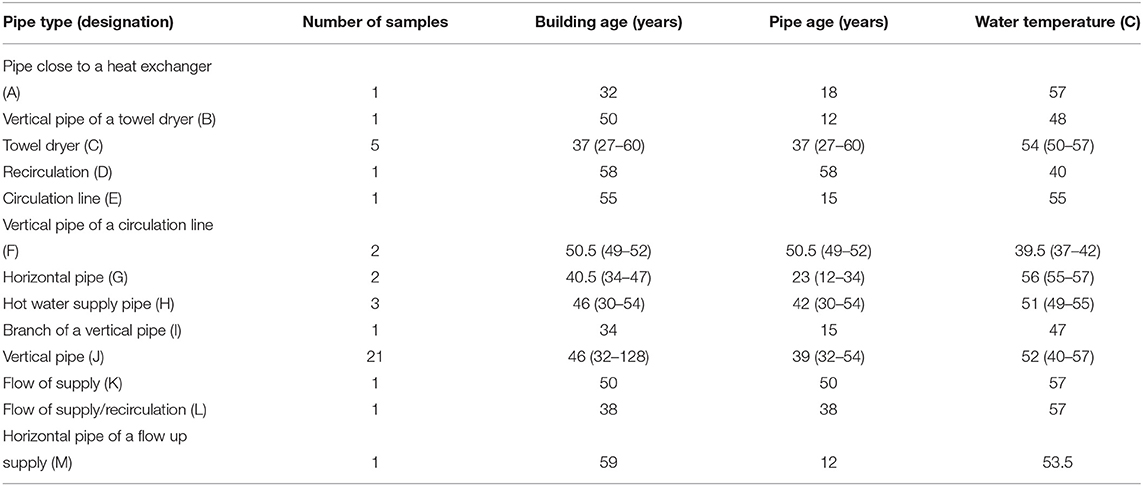
Table 1. Environmental information on the 45 biofilm samples collected from the inner surfaces of the pipes in the hot water distribution systems of apartment buildings in Riga (the capital of Latvia) during the repair works in May and June 2017.
Bacterial Analysis
The quantity of mesophilic aerobic and facultative anaerobic microorganisms was determined at 22 ± 1°C, according to the procedure of ISO 6222:1999 quality standard except that sterile water was used for dilution instead of peptone diluent. In brief, 1 g of each biofilm sample was diluted (1:10). Thereof, 1 ml was inoculated in yeast extract agar and incubated for 72 h. The numbers of L. pneumophila in the respective samples were determined according to the ISO 11731:2017 principles and normalized to 1 g of dry weight. In particular, 0.1 ml of the aforementioned dilution was inoculated on a basic medium, buffered charcoal yeast extract agar with L-cysteine, iron (III) pyrophosphate and selective supplements (BCYE+AB) (Liofilchem, Roseto degli Abruzzi, Italy). Incubation was done at 36 ± 1 °C for 10 days. Legionella spp.- like colonies were transferred to BCYE agar and BCYE-Cys agar (Liofilchem, Roseto degli Abruzzi, Italy), and the plates were incubated at 36 ± 1 °C for 2 days. Detection limit for Legionella spp. cultivation was calculated to be 100 CFU g−1. Species of Legionella spp.-like colonies was confirmed on autoflex speed MALDI-TOF mass spectrometer (Bruker Daltonics). L. pneumophila serogroups were determined using the ProlexTM Legionella pneumophila Serogroup 1 Latex Monoclonal Reagent and ProlexTM Legionella pneumophila Serogroups 2-14 Latex Polyclonal Reagents (Pro-Lab Diagnostics, Canada), according to manufacturer's recommendations.
Morphologic Identification of Free-Living Protozoa
Our culture method for FLP was as described in Vaerewijck et al. (2010). In order to permit the growth and multiplication of FLP, 15 ml of liquid Page amoeba saline (PAS) medium with two sterilized rice grains was added to each Petri plate containing 300 mg of undiluted biofilm sample. All plates were incubated for 4–5 days at 25°C. Thereafter, each Petri plate was examined under the light microscope (see Supplementary Figure S2 for a couple of examples). To further increase the biomass of FLP, additional 15 ml of PAS medium were added to each Petri plate, supplemented with 70μl liquid Peptone yeast-glucose (PYG) medium. Due to the low amount of PYG medium added, bacterial overgrowth could be avoided. This was followed by another round of incubation for 4–5 days at 25°C and a repeated examination under the light microscope for the presence of FLPs, which were then taxonomically classified based on morphological observations, as described in Vaerewijck et al. (2010).
DNA Extraction and 16S/18S rRNA Amplicon Sequencing (Metataxonomics)
Microbial DNA was extracted from 250 mg of each biofilm sample using the DNeasy PowerSoil Kit (Qiagen, Hilden, Germany), following the manufacturer's instructions. As a negative extraction control, nuclease- and DNA-free water was used. Nuclease- and DNA-free water was used instead of a biofilm sample as a duplicate negative control of DNA extraction. These two negative controls were co-processed throughout the process of library preparation, sequencing and taxonomic assignment. Both negative controls performed as expected and no significant background contamination was observed.16S rRNA amplicon sequencing libraries were prepared according to Illumina's 16S Metagenomic Sequencing Library Preparation guide (document part #15044223 Rev. B), which uses primers S-D-Bact-0341-b-S-17 and S-D-Bact-0785-a-A-21 from Klindworth et al. (2013) to amplify a 464 bp long fragment of the V3-V4 regions of the bacterial 16S ribosomal RNA rRNA gene. All PCR reactions were supplemented with 1 mg ml−1 bovine serum albumin (BSA) to prevent inhibition. MinElute PCR Purification Kit (Qiagen) was used to remove BSA before purification with SPRI magnetic beads. Eukaryotic 18S rRNA amplicon sequencing libraries were prepared similarly except that different primers and PCR conditions were used. Forward primer Reuk454FWD1 (Stoeck et al., 2010) and reverse primer V4 (Bradley et al., 2016) with Illumina adapter overhangs were used to amplify the V4 hypervariable region of the 18S rRNA gene. PCR conditions for amplification of 18S rRNA gene fragment were as follows: 95°C for 5 min, 14 cycles of 98°C for 30 s, 57°C for 45 s and 72°C for 1 min, 21 cycle of 98°C for 30 s, 47°C for 45 s and 72°C for 1 min, with final extension at 72°C for 5 min. Only samples with detectable PCR product were further processed and sequenced. Amplicon sequencing was performed using the Illumina MiSeq platform and the MiSeq Reagent Kit v3 and MiSeq Reagent Kit v2 (Illumina, San Diego, United States), generating 2 x 300 bp and 2 x 250 bp paired-end (PE) reads, respectively (v2 kit was used only for the 18S rRNA amplicon libraries). The raw sequence data are available from the European Nucleotide Archive (ENA) database under the accession numbers PRJEB27134 (45 samples, for which 16S rRNA amplicon sequencing was performed) and PRJEB31264 (12 samples, for which 18S rRNA amplicon sequencing was performed).
16S/18S rRNA Amplicon Sequencing (Metataxonomic) Data Processing
16S rRNA amplicon sequencing (metataxonomic) data analysis was performed using the Illumina 16S Metagenomics workflow (v2.6.2.3.), available in the MiSeq Reporter software. The total number of sequencing reads ranged from 31,109 to 284,706 per sample (an average being 103,605 reads per sample). Sequences were clustered into OTUs based on 97% identity and taxonomic classification of the respective OTUs was performed, using an implementation of the Ribosomal Database Project (RDP) naive Bayesian classifier (Wang et al., 2007), against a curated version of the Greengenes taxonomic database. Analysis of 18S rRNA amplicon sequencing (metataxonomic) reads were performed in the CLC Genomics Workbench v11.0.1 (Qiagen). Briefly, adapter trimming, quality trimming and filtering was followed by down-sampling, open reference based OTU clustering against the PR2 v4.11.1 reference database (Guillou et al., 2012) at 97% similarity and including also chimera removal. When interpreting the results, the 0.1% minimum abundance threshold was considered, based on the known 0.1% carry-over contamination between Illumina MiSeq runs (Nearing et al., 2018).
Statistical Analysis
Biofilm community ecology analysis was performed using the R package vegan v2.5-2 (Jari Oksanen et al., 2018). First, raw sequence counts were normalized by rarefaction, as suggested in the package wiki1 as the community ecology analysis would require the numbers of taxonomic groups. Rarefaction was performed to the smallest number of sequences in our samples (n = 31,109) without replacement using the R package RAM and function OTU.rarefy (Chen, 2018). We calculated the alpha-diversity including the total number of different taxa in each sample (the richness; S), how evenly distributed these taxa are (Pielou's evenness; J'; constrained between 0 and 1, where 0 would indicate the presence of one dominant taxa) and the overall taxa diversity (the Shannon's diversity index; H'), which accounts for both the total number and evenness of the taxa. S and H' were calculated using the functions specnumber and diversity, respectively. J' was then calculated as J′ = H′/log(S). Pearson correlations were used to relate S, H', and J' to the 18 environmental factors (Table 1 and Supplementary Table S1). We also calculated the Rènyi's entropy profiles for each sample, reflecting a continuum of possible Shannon's diversity measurements, using the function renyi. Beta-diversity (i.e., the Bray-Curtis dissimilarities; BCD) was calculated using the function vegdist. To assess, which of the environmental factors (i.e., water temperature of the pipe, the age of the pipe or the floor of the building, from which the sample was collected; see Table 1 and Supplementary Table S1) has the strongest influence on this change in diversity of taxa between samples, we used the adonis function with 1,000 permutations to also determine the significance (p-Value) of these associations. In all cases, Benjamini-Hochberg (BH) (Benjamini and Hochberg, 1995) adjustment for multiple testing was used to calculate the false discovery rate (FDR).
Co-occurrence Network Analysis
We took a similar approach, as described in Org et al. (2017) to conduct bacterial co-occurrence network analysis. First, we normalized the genus abundances by calculating their respective proportions of the sample total sequence count, which were then arcsine-square root-transformed as a means of variance-stabilization and used as input to generate a co-occurrence network using the R package WGCNA (Langfelder and Horvath, 2008). We discarded all the genera that were present in less than 50% of our biofilm samples, leaving us with 272 “common” genera (out of 611 total genera identified) for further analysis. Although, clearly, also (or maybe in particular) taxa present in some samples may provide a very valuable information from the ecological point of view, the co-occurrence network analyses is based on correlations, hence including rare OTUs would create a “missing value” problem, requiring imputation, which may make sense for the so called “non-biological zeros” (technical and sampling zeros) (Jiang et al., 2021), however, may be less useful for “biological zeros” i.e., taxa that are non-existent in the particular samples. We re-constructed a signed network, where the minimal beta value satisfying the scale free topology criteria was chosen to be 6. For the dynamic tree cut function, we set deepSplit = 2 and minModuleSize = 5 as parameters. To relate the resulting genera groups (modules) to the environmental factors and the presence of L. pneumophila and its potential hosts (Table 1 and Supplementary Table S1), we calculated the Pearson's correlation between each environmental factor and the so-called module eigengenes (MEs), defined as the first principle component of a module, followed by BH (Benjamini and Hochberg, 1995) adjustment for multiple testing.
Functional Predictions
The functional composition of the modules was predicted using their pangenome-based functional profiles, as implemented within the PanFP tool (Jun et al., 2015), which utilizes all the available complete genomes of prokaryotes and their respective taxonomic classifications from the National Center for Biotechnology Information (NCBI) database [ftp://ftp.ncbi.nih.gov/genomes/] combined with the functional annotation from the KEGG Orthology (KO) database [https://www.genome.jp/kegg/ko.html]. Fisher's exact test was used to calculate the overlaps between the gene sets, i.e., module genes and genes annotated to the respective KO term, followed by the BH (Benjamini and Hochberg, 1995) adjustment for multiple testing.
Visualization
The overlaps between the occurrence of L. pneumophila in different hosts was visualized by Venn diagrams [http://bioinformatics.psb.ugent.be/webtools/Venn/].
Results
During the repair works in May and June 2017 in Riga, we were able to collect 45 biofilm samples (numbered one to 47) from the inner surfaces of hot water distribution system pipes of apartment buildings in Riga, supplemented by additional information on the environmental factors (e.g., water temperature, the age and type of the pipe), as summarized in Table 1 and Supplementary Table S1. Among others, we also documented the geographic coordinates—latitude and longitude—of the apartment buildings, which, in our case, were related to different primary water sources that supply drinking water to different parts of the city [https://www.rigasudens.lv/en/]. In particular, for the north part, natural groundwater is taken from the resource “Baltezers-Zaķumuiža” and supplemented with water from the lake “Mazais Baltezers.” Whereas, for the south part, the reservoir of Riga hydro-power plant on the River “Daugava” is used as the primary source of surface water.
Bacterial Community Diversity in Hot Water Pipe Biofilms, as Assessed by 16S rRNA Amplicon Sequencing
First, we explored the bacterial diversity of the 45 biofilms, using 16S rRNA amplicon sequencing. We analyzed the phylogenetic variation across the biofilms at different taxonomic levels (i.e., phylum, genus, and species).
Phylum-Level Analysis
Phylum-level analysis (Figure 1A and Supplementary Table S2) revealed that, in all samples, quantitatively, the most abundant phyla were Proteobacteria spp. (~36.3% of total sequences), followed by Firmicutes (~15.6%), Nitrospirae (~8.3%), Planctomycetes (~7.9%) and Actinobacteria (~3.4%) spp., supplemented by minor fractions of Bacteroidetes (~0.58%), Acidobacteria (~0.37%) and Cyanobacteria (~0.39%) spp. We also observed several thermophiles, such as Chloroflexi (~2.9%), Thermi (~1.49%) and Thermotogae (~1.38%) spp. in our biofilm samples.
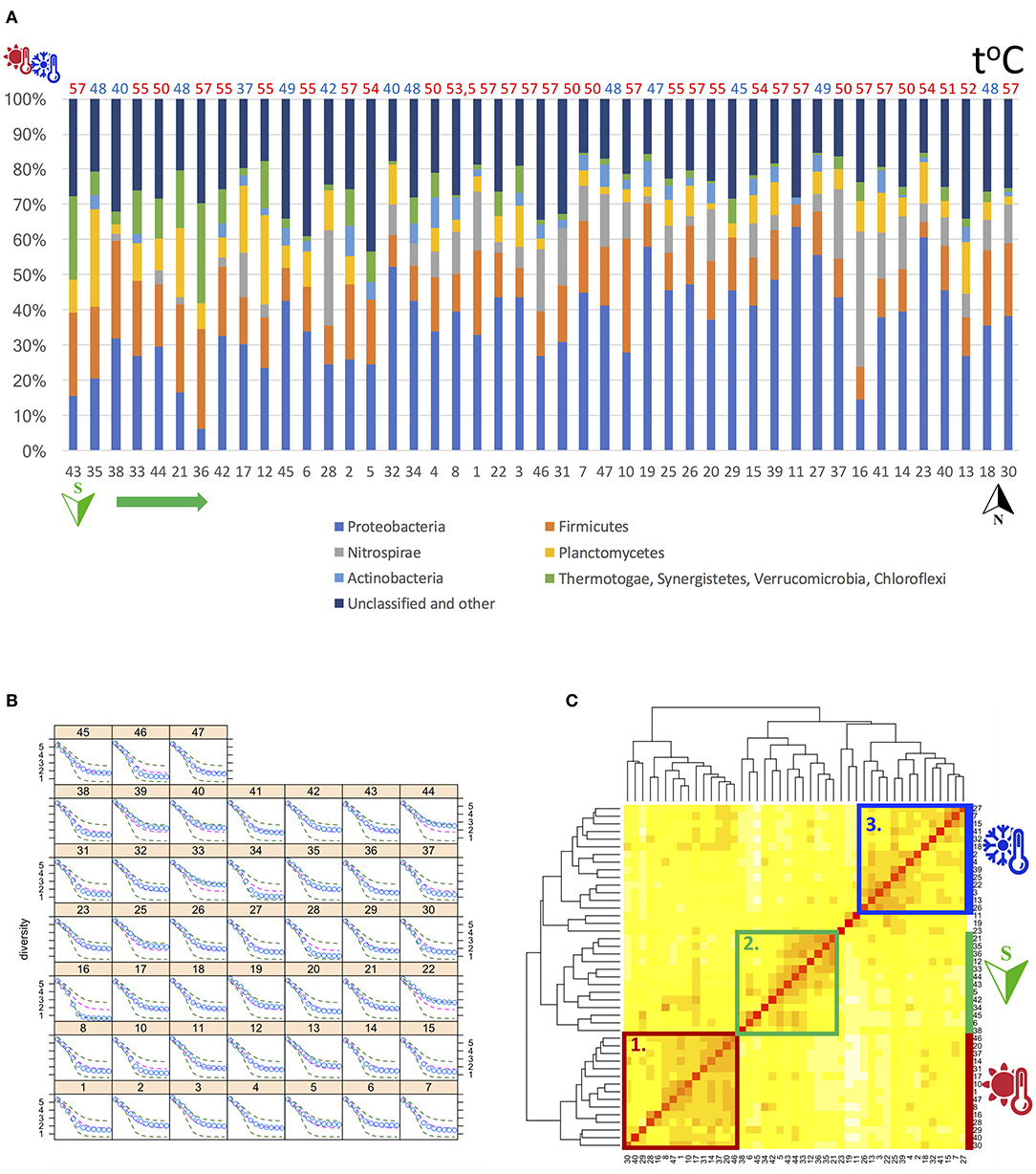
Figure 1. (A) Phylum-level analysis of the 16S rRNA amplicon sequencing data, showing the percentage of each particular phyla from the total number of sequences in each sample. The “other” category represents the sum of all classifications with less than 3.5% abundance. Samples are sorted by latitude (south to north) and the water temperature in the respective pipe is displayed above each bar (≥50°C in red and <50°C in blue). (B,C) Alpha and beta diversity analysis of the 16S rRNA amplicon sequencing (metataxonomic) data. (B) Renyi's entropy profiles of the 16S rRNA amplicon sequencing data reflecting a continuum of possible Shannon's diversity measurements (x-axis). Calculated using rarefied read counts. The plot uses Trellis graphics with a separate panel for each biofilm sample. The dots show the values for the respective sample, whereas the lines mark the extremes (in dark green) and median (in magenta) in the data set. (C) A Heatmap visualizing the relationships (clusters) among the 16S rRNA amplicon sequencing samples, based on the Bray-Curtis distance (beta diversity) calculations. It reflects the inter-sample diversity: 0 means that two samples have the same composition (i.e., share all the genera), whereas 1 means the two samples do not share any genera. Three large groups of biofilms, sharing similar composition are labeled 1. (in red), 2. (in green) and 3. (in blue). One of the groups (2. in green) was related to latitude, being dominated by samples from the southern part of Riga. Both other groups were related to water temperature. Of those, one (1. in red) was mainly dominated by water temperatures ≥50°C, whereas the other group (3. in blue) was dominated by water temperatures <50°C.
Genus-Level Analysis
We identified 611 OTUs, 272 of which were present in at least 50% of the biofilms and were considered as “core” for our biofilms (Supplementary Table S3). From these, 82 were present in all 45 biofilms, whereas 80 others were detected in only one sample each (Supplementary Figure S3). Quantiatively, 107 genus were represented by at least 0.1% of the total sequences, 48 (or ~45%) of these belonged to the phylum Proteobacteria (Supplementary Table S2).
Nevertheless, the most abundant genus was Thermodesulfovibrio (~7.5%) belonging to the phylum Nitrospira. It was followed by Phenylobacterium (~2.9%; phylum Proteobacteria), Moorella (~2.7%; phylum Firmicutes), Gemmata obscuriglobus (~2.6%; phylum Planctomycetes) and Rhodoplanes (~2.5%; phylum Proteobacteria). Legionella spp. (phylum Proteobacteria) was detected in 44/45 of biofilms (Supplementary Table S3), however its abundance was low, accounting, on average, only for 0.58% of total sequences (Supplementary Table S3).
Alpha Diversity Analysis
We next calculated several indices exploring the bacterial landscape within each biofilm (the so-called alpha diversity), including the richness (S), the Pielou's evenness (J'), the Shannon's diversity index (H') and the Rènyi's entropy profiles for each sample (see Materials and methods for definitions). Of note, it is well-known that the full spectrum of taxa in a sample is rarely saturated and increases with increasing sequencing depth (Weiss et al., 2017). Indeed, also in our data, both S and H' significantly positively correlated (R = 0.7, P = 1.3 x 10−8 and R = 0.4, P = 5.3 x 10−3, respectively), with the total number of sequences in each biofilm, only J' being sequence count independent (R = 0.24, P = 0.1; Supplementary Figure S4). Hence, we rarefied the raw sequence counts and repeated the analysis (Supplementary Table S4) and divided all indices into quantiles, creating four comparison groups (Supplementary Table S4) including the highest, above median, below median and the lowest diversity indices, respectively.
We also had two parallel sample pairs, collected from the same building: biofilms 7 vs. 47 and 27 vs. 37, allowing us to compare the biofilm diversity within one building. We observed that S ranged from 184 (biofilm 45) to 289 (biofilm 35), with a mean value of ~224. Biofilms 7 and 37 were in the lowest S group, whereas biofilms 47 and 27 were in the below median group (Supplementary Table S4), suggesting that genera richness can vary, even within the same building. J' ranged from 0.45 (biofilm 16) to 0.71 (biofilm 22), with a mean value of 0.62, indicating rather even distribution of genera, i.e., no trend toward a single dominating genus. H' ranged between 2.40 (biofilm 16) and 4 (biofilm 22), with a mean value of ~3.40. Biofilms 27 and 37 were both in the highest J' and H' groups, whereas biofilm 7 was in the below and above median group, respectively, but biofilm 47 was in the below median group in both cases (Supplementary Table S4), again suggesting that, even within the same building, biofilm landscapes may differ. When comparing the Rènyi's entropy profiles for each sample (Figure 1B), we observed that biofilms 22, 33 and 44 displayed higher diversities, as compared to all other biofilms, whereas biofilm 16 was less diverse, in line with the observations from H' and J' calculations. The diversity measurements for the two same-building sample pairs (i.e., biofilms 7 vs. 47 and 27 vs. 37) were similar. Finally, we explored, whether the alpha diversity measures (S, H', and J') demonstrated significant correlations with the 18 environment traits. H' and J' positively correlated (R = 0.3) with pipe age (P < 0.04), however, both correlations were not significant at FDR 5%.
Beta Diversity Analysis
We next calculated the beta diversity, i.e., the inter-sample distance of microbial composition or the Bray-Curtis distance (BCD; Figure 1C), which ranges between 0 and 1, where 0 means that two samples have the same composition (i.e., share all the genera), whereas 1 means the two biofilms do not share any genera. In our samples, the BCD ranged from 0.2 (biofilms 1 and 10, 20 and 46) to 0.9 (biofilms 11 and 29), with the mean value of 0.64, suggesting that our biofilms were rather dissimilar in their genera composition. Biofilms 19 and 11 had the highest median BCD measurements to all other biofilms—0.8 and 0.79, respectively, followed by biofilms 45 and 26, with a median BCD of 0.74 and 0.72, respectively. Overall, three large groups of biofilms, sharing similar composition, could be distinguished (Figure 1C). Water temperature (F = 4.1, P < 0.001) and latitude (i.e., different primary water sources) (F = 5.6, P < 0.001) were predicted to have the most significant effect (at FDR 5%) on the variations in BCD (Supplementary Table S5).
Bacterial Co-occurrence Sub-networks (Modules) and Their Relations to the Environment Traits
In order to identify sub-networks (modules) of bacteria occurring together (i.e., OTUs demonstrating similar abundance across the biofilm samples) and elucidate their relations to the environment traits (Table 1 and Supplementary Table S1), we used the weighted gene co-expression network analysis (WGCNA) (Langfelder and Horvath, 2008) to re-construct a network of co-occurring genera and identify modules within this network, similarly as previously described by Org et al. (2017). Of note, we considered only the 272 most “common” genera, present in at least 50% of our biofilms (Supplementary Table S3) and calculated the so called “hub” of each module, i.e., the most central genus (see the Module membership (MM) description below). This analysis yielded 10 modules (Figure 2A and Supplementary Table S6), each of which was given an arbitrary color name—black: (14 genera; Thermodesulfatator), blue (28 genera; Exiguobacterium), brown (24 genera; Blastochloris), green (22 genera; Meiothermus), magenta (13 genera; Ammonifex), pink (14 genera; Hydrogenophaga), purple (11 genera; Desulfosarcina), red (16 genera; Thioalkalivibrio), turquoise (51 genera; Hyphomicrobium) and yellow (24 genera; Alishewanella). The gray module unified 55 genera that could not be grouped in any particular community. Legionella spp. was part of the turquoise module (Supplementary Table S6).
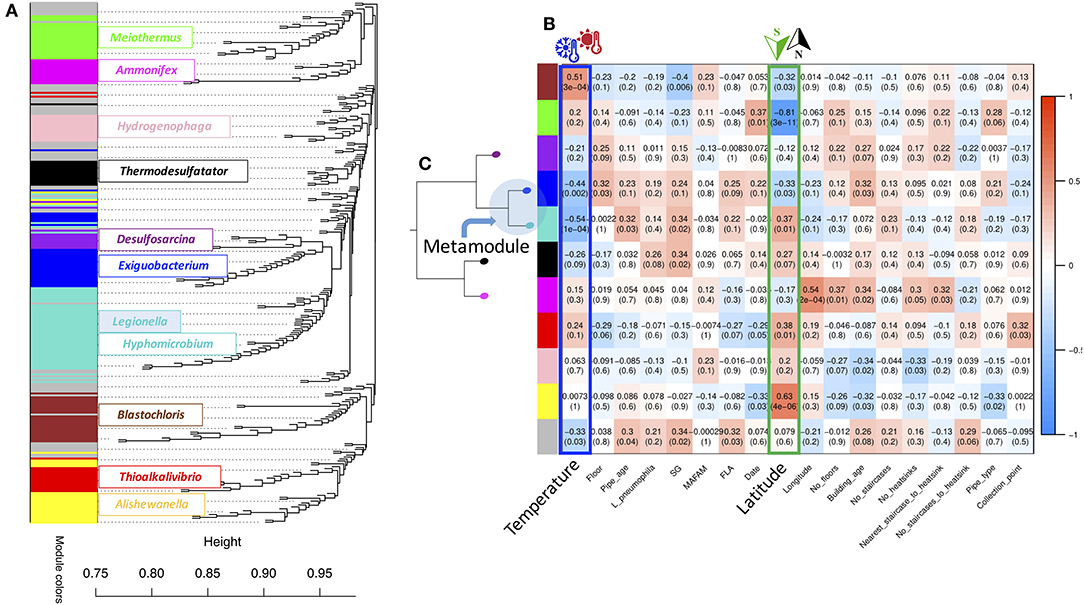
Figure 2. Bacterial co-occurrence network re-construction and module identification using weighted correlation network analysis (WGCNA; Langfelder and Horvath, 2008). This analysis yielded 10 modules (Supplementary Table S6), each of which was given an arbitrary color name-black, blue, brown, green, magenta, pink, purple, red, turquoise and yellow. (A) A clustering dendrogram of genera with assigned module colors and displaying the most central genus (as estimated using Module membership (MM) calculations). (B) A Heatmap visualizing the Pearson correlation strength and significance (nominal p-Values) of the 10 bacterial genera co-occurrence network modules associations with the 18 environmental traits (Table 1 and Supplementary Table S1). SG, serogroup; MAFAM, mesophilic aerobic and facultative anaerobic microorganisms; FLP, free-living protozoa. (C) A dendrogram of the relationships among the bacterial genera co-occurrence modules.
Next, we summarized each module by the module eigengene (ME), which is the first principal component of the module, capturing the majority of variation in genera abundance in that particular module. These module ME values were then correlated (Pearson's correlation test) with the environmental traits (Figure 2B and Supplementary Table S7). The Legionella spp.-containing turquoise module was the largest module (51 genera) and demonstrated highly significant negative (R = –0.54, P = 1.4 x 10−4, at FDR 5%) correlations with water temperature, which is in agreement with the mesophilic nature of Legionella spp. Less significant (at FDR 25%) positive correlations were observed with pipe age (R = 0.32, P = 0.03) and latitude, i.e., different source water (R = 0.37, P = 0.01). Another module demonstrating highly significant (at FDR 5%) negative correlations with water temperature (R = –0.44, P = 2.3 x 10−3) was the blue module. However, it was also negatively (R = –0.33, P < 0.03, at FDR 25%) correlated with latitude. In fact, the turquoise and blue modules were highly related to each other and part of the same meta-module (Figure 2C). In contrast, the brown module displayed the strongest and most significant positive correlation with water temperature (R = 0.51, P = 3.4 x 10−4, at FDR 5% (Figure 2B and Supplementary Table S7), and was negatively (R = –0.32, P = 0.03, at FDR 25%) correlating with latitude i.e., source water.
We further explored two metrics provided by WGCNA to prioritize genus within each module: genus significance (GS) and module membership (MM). GS is defined as the correlation between genus abundance and the respective environmental trait of interest (e.g., water temperature), thus, providing a measure of the relevance of that particular genus to the variation in that trait. MM of a genus indicates how strongly the abundance of a particular genus correlates with all the other genera within that module, i.e., how tightly a genus is “connected” to all other genera from that module. Highly “connected” or “hub” genera tend to have high MM values, whereas low MM values indicate orientation toward the periphery of the module. The three above mentioned modules—turquoise, blue and brown—displayed the highest correlations with water temperature (Supplementary Figure S5). In the turquoise module, the top genera most significantly (at FDR 5%) negatively correlating with water temperature (Supplementary Table S7) were Desulfovibrio (0.17% of total; R = –0.62, P = 5.2 x 10−6), Leptolyngbya (0.16% of total; R = –0.61, P = 5.9 x 10−6) and Hyphomicrobium (0.73% of total; R = –0.53, P = 1.5 x 10−4), the latter also being the “hub” genus of the module (MM = 0.82, P = 8.7 x 10−12, at FDR 5%).
Predicting the Functional Profiles of the Hot Water Pipe Biofilms
In order to also predict the potential functional implications of the analyzed biofilms, we performed a pangenome-based reconstruction of their putative genes/proteins and pathways, using the PanFP tool (Jun et al., 2015) and determined the over-represented pathways (at FDR 5%) in the three modules of interest (Figure 3 and Supplementary Table S8). As it can be inferred from Figure 3, the pathways significantly over-represented in all modules included “Biofilm formation,” the cell to cell communication system “Quorum sensing,” “Bacterial secretion system components,” and “Transporters,” as well as “Bacterial motility and chemotaxis” and “Lipopolysaccharide biosynthesis.” On top of that, we observed enrichment of various metabolic activities, including “Carbon fixation,” as well as “Sulfur,” “Nitrogen,” and “Methane metabolism.” At the same time, there were several pathways over-represented in only one or two of the three modules. For example, the turquoise module was enriched in “Legionellosis”-related genes/proteins, whereas the blue and brown modules demonstrated enrichment in pathways related to “Bacterial toxins” and “Cell growth” (Supplementary Table S8).
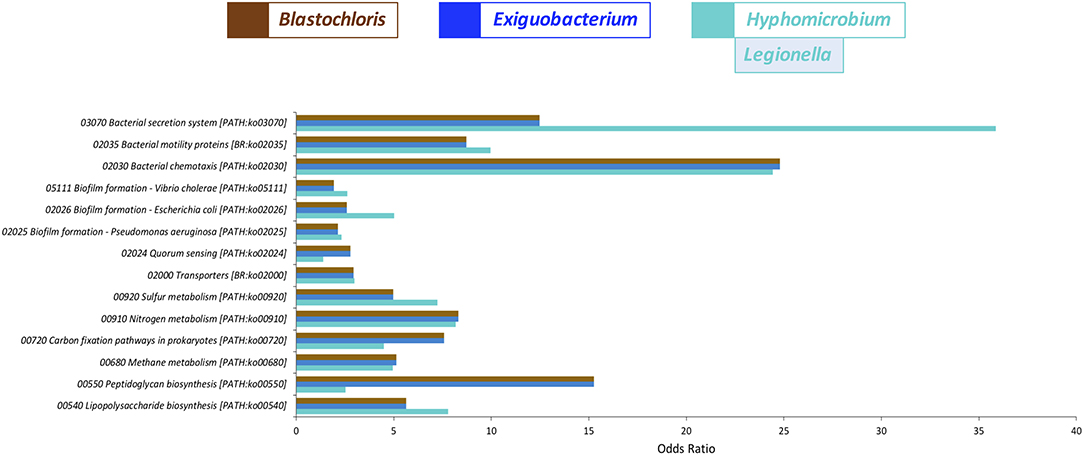
Figure 3. Pangenome-reconstructed functional protein enrichment analysis for the bacterial genera co-occurrence modules blue, brown and turquoise, displaying the most central genus of the module [as calculated by the measure of Module membership (MM)] in the figure legend. The x-axis demonstrates the Odds ratio of the Fisher's exact test, i.e., the odds for a genus to be part of the turquoise, blue or brown modules, respectively, while being annotated to the respective KO term (y-axis).
Eukaryotic Community Diversity in Hot Water Pipe Biofilms as Assessed by 18S rRNA Amplicon Sequencing
Finally, we also explored the eukaryotic community diversity in 12 of the 45 biofilms (only 12 samples produced detectable PCR amplicons that could be sequenced) using 18S rRNA amplicon sequencing. We identified 248 OTUs that could be classified as Eukaryota, according to the PR2 reference database (Guillou et al., 2012; Supplementary Table S10), thereof: Archaeplastida: 121, Opisthokonta: 44, Amoebozoa: 22, the SAR supergroup (Stramenopiles: 13, Alveolata: 6 and Rhizaria: 38), as well as Excavata: 2, Apusozoa: 1 and Eukaryota_X (Unclassified): 1 (Figure 4A). The Archaeplastida constitute a major group of eukaryotes, comprising the land plants, green and red algae, and a small group called the glaucophytes. The Opisthokonta, previously called the Fungi/Metazoa group, are a broad group of eukaryotes, including both the animal and fungus kingdoms. The Rhizaria are a species-rich super-group of mostly unicellular eukaryotes. The Amoebozoa and Alveolata unify amoeboid protists and protists, respectively. The Stramenopiles or Heterokonta includes mostly algae and parasitic oomycetes. Excavata contains a variety of free-living and symbiotic forms, and also includes some important parasites of humans. The Apusozoa comprises flagellate eukaryotes, occurring in soils and aquatic habitats, where they feed on bacteria (Guillou et al., 2012).
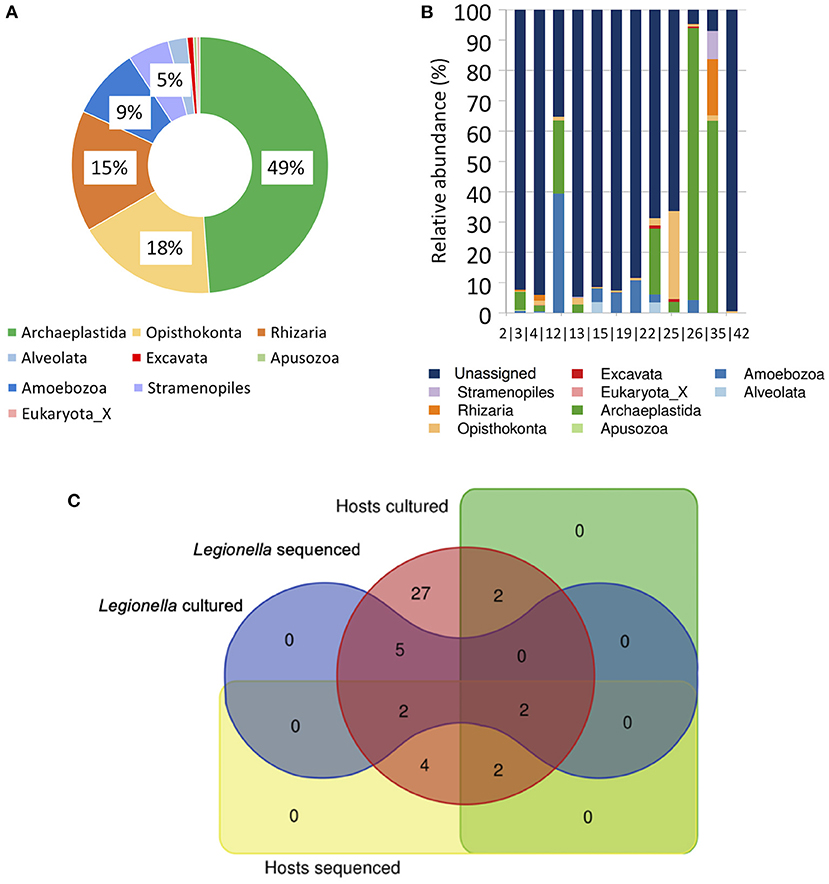
Figure 4. (A,B) Different major groups (kingdom-level groups or supergroups) of Eukaryota identified within the 18S rRNA amplicon sequencing (metataxonomic) data. (A) A pie chart, showing the proportions of each major group of all the operational taxonomic units (OTUs) identified. (B) A barplot, showing the proportions of each major group of all the OTUs for each sample separately. (C) A Venn diagram, showing the overlap between the presence of L. pneumophila and its potential hosts (Acanthamoeba, Filamoeba, Flamella, Vermamoeba and Vahlkampfia spp.) in the 16S/18S rRNA amplicon sequencing data vs. conventional culture-based assays.
Alpha Diversity Analysis
Like for the 16S rRNA amplicon sequencing data, we calculated several indices exploring the alpha diversity, such as S, J', H', and Rènyi's entropy profiles for each biofilm sample, considering all nine Eukaryotic super-groups together. S ranged from 3 (biofilm 42) to 86 (biofilm 35), with the mean value of ~20 per biofilm sample. J' ranged from 0.12 (biofilm 42) to 0.76 (biofilm 35), with the mean value of 0.44. H' ranged from 0.12 (biofilm 42) to 3.38 (biofilm 35), with the mean value of 1.26. We observed no significant correlations for these measures with the 18 environmental factors listed in Table 1 and Supplementary Table S1. When restricting our analysis to phagocytic free-living protists (i.e., excluding Opisthokonta such as Fungi and Metazoa, and Archeaplastida), the number of taxa decreased to 0 (biofilm 42) to 20 (biofilm 35), with the mean value of ~6 per biofilm sample. J' ranged from 0 (biofilm 42) to 0.8 (biofilm 2), with the mean value of 0.42. H' ranged from 0 (biofilm 42) to 2.14 (biofilm 35), with the mean value of 0.79.
Beta Diversity Analysis
We next calculated the BCD for the 18S rRNA amplicon sequencing data, again first considering all the nine Eukaryotic super-groups together. BCD ranged from 0.71 (biofilms 2 and 3) to 1 (e.g., biofilms 2 and 19, 2 and 42, 3 and 42, 4 and 19, 13 and 42, 19 and 42), with a mean value of 0.96, suggesting that the compared biofilms differed substantially in their Eukaryotic composition, in particular, biofilm 42 displayed a BCD of 1 to four other samples. For the phagocytic free-living protists, the BCD ranged from 0.7 (between biofilm samples 4 and 15) to 1.0 (e.g., between biofilm sample 42 and all the other samples, as well as between biofilm samples 2 and 3, 2 and 13, 2 and 19, 3 and 12, 3 and 35, 4 and 19), with a mean value of 0.96, again suggesting different compositions of phagocytic free-living protists across the biofilm samples.
Overall, the distribution of the different Eukaryotic groups was not uniform across the biofilm samples (Figure 4B): Archaeplastida strongly dominated two (biofilms 26 and 35) biofilm samples and was present abundantly in further two biofilms (biofilms 4 and 22). At the same time, it was not detectable in four other biofilm samples (biofilms 13, 15,19 and 42). Alveolata was mainly present in biofilm samples 13 (mainly Paraschneideria metamorphosa: 3.6% of total) and 22 (mainly Gregarines XX sp.: 3.5% of total), Amoebozoa was mainly detected in biofilm 4, as well as, in smaller amounts, in biofilm samples 19, 15 13, 26, 22, 2 and 3 and was dominated by Mb5C-lineage X sp. in six (50%) biofilms: 38.5% (4), 6.8% (15), as well as <1% of total sequences in biofilms 2, 3, 12 and 22. In five other biofilm samples, Echinamoeba exundans was detected: 9.4% (biofilm 19) and <1% in biofilm samples 2, 3, 13 and 22. Vermamoeba vermiformis was present in further four biofilm samples: >3.4% (13), >1.3% (22) and <1% in biofilm samples 3 and 12. Few representative sequences (<1%) of Vermamoeba and Echinamoeba were identified across several biofilm samples. Filamoeba was detected in five biofilms: 4.3% (biofilm 26) and <1% in biofilm samples 3, 13, 19, and 22 (Supplementary Table S10). Excavata (Vahlkampfiidae) were present in nine biofilms (75%), in four of those (biofilms 15,19, 22, and 25) >1%, whereas in five (biofilms 2, 3, 4, 13 and 26) <1% of total sequences. Finally, when comparing the co-occurrence of L. pneumophila and its potential hosts (Acanthamoeba, Filamoeba, Flamella, Vermamoeba and Vahlkampfia), as detected using conventional culture-based assays vs. sequencing (Figure 4C and Supplementary Table S10), we identified two biofilm samples (biofilms 15 and 22), where Legionella spp. and FLP were detected together using both methods.
Identification and Quantification of Cultivable L. pneumophila and FLP in Biofilm Samples
We were particularly interested to detect the presence of L. pneumophila and its host FLP in our biofilms. Using cultivation methods, L. pneumophila was detected in nine (20%) biofilms, where its total numbers ranged from 100 to 5,600 colony forming units (CFU) g−1. However, only serogroups 2, 3, 6 and 9 were detected, serogroup 2 being the predominant one (in five or >55% of biofilms; Supplementary Table S1). In six (~13 %) biofilms, FLP were detected via microscopy, the most common genera being Acanthamoeba, Vahlkampfidae and Vermamoeba (Supplementary Table S1). However, only in two biofilm samples (15 and 22, the latter originating from the oldest—128 years old—building) FLP (from all three genera) were detected together with L. pneumophila (serogroup 9; 4,400 CFU g−1).
Discussion
In this study, in line with previous observations (Paranjape et al., 2020), we aimed to conduct a systems-level investigation to further explore the hypothesis that both, the presence of FLP hosts, as well as the composition of bacterial communities of domestic hot water pipes may affect the presence ofL. pneumophila and that certain environmental factors might demonstrate correlations with this community structure and sub-structure (modules) and functional capabilities, pointing to a potentially systemic effect and ecological affinities, resulting in complementary or competitive functionality, with potential consequences for the domestic water quality.
Clearly, the present study can be considered as mainly descriptive and has a number of limitations. As already recognized earlier, real-world biofilm samples are generally (an also in this case) obtained during repair works of networks, which poses challenges with regard to representative sampling, limited quantities of biomass available, appropriate controls, as well as comprehensive documentation of environmental variables (Fish et al., 2016). All our samples were collected exclusively from the right bank of the river “Daugava,” exclusively in summer and from hot water pipes only. We also lack data on bulk water (planctonic) microorganisms and water composition (nutrients/inorganics). We could not compare different pipe materials, a factor with demonstrated impact on microbial diversity in biofilms (Yu et al., 2010; Lu et al., 2014; Proctor et al., 2018), as all the pipes were made of galvanized steel. Moreover, we also lack information on pipe diameter and hydraulics, shear stress and biofilm stability/cohesion. The water temperatures we report were measured only at time of sampling, we lack information on the usage water temperatures, as well on the fluctuations (both short term such as day/night and long-term i.e., summer/winter) in water temperatures. Furthermore, consumer reports on water quality would need to be considered in future investigations. Of note, we also do lack functional data and structural analyses of the biofilms. Computationally, the usage of OTUs instead of recently introduced amplicon sequence variants (ASVs) (Callahan et al., 2017) as well as the usage or rarefaction as a method of normalization may be perceived as a further limitations. ASVs are intended to provide a better resolution than OTUs (Callahan et al., 2017) and the compositional nature of metataxonomic sequencing data may require customized normalization approaches (Marcos-Zambrano et al., 2021; Moreno-Indias et al., 2021). However, as with other novel approaches/open-source tools (Vilne et al., 2019), a rigorous assessment and benchmarking of ASVs vs. OTUs and rarefaction/proportion calculation vs. customized normalization approaches (Marcos-Zambrano et al., 2021) across different sites would be required, before integrating this approach into routine implementation of NGS analysis for monitoring and diagnostics testing, as there are still issues that need to be solved, such as the recently reported observation of potentially splitting a single genome into separate multiple bins that would inflate the observed diversity (Schloss, 2021), e.g., for L. pneumophila that typically has multiple copies of small subunit rRNA (https://rrndb.umms.med.umich.edu/). Finally, the usage of OTUs and rarefaction/proportion calculation allowed us to make direct comparisons with the previous literature, which has been largely using these conventional classification and normalization approaches.
There have been a number of previous investigations on water pipe biofilm diversity (Yu et al., 2010; Farhat et al., 2012; Lührig et al., 2015; Mahapatra et al., 2015; Ji et al., 2017, 2018; Bertelli et al., 2018; Fish and Boxall, 2018; Waak et al., 2019). However, the majority of these studies have been conducted through controlled laboratory experiments that may actually not replicate the real-world water pipe system microbiota accurately, hence, comparisons between different studies should be undertaken with care (Fish et al., 2016). In our study, the mean number of genera was ~224 per biofilm, and these were rather evenly distributed (J' 0.45–0.71), displaying no trend toward a single dominating genus. For example, Ji et al. (2017) and Waak et al. (2019) have reported 62,494 (H' of 2–6) and 41,815 OTUs, respectively. However, most probably, these include also the unclassified ones. Lu et al. (2014) reports (Lu et al., 2014) 125 OTUs, 16 of those with abundance >1%. Chan et al. (2019) has recently reported 732 to 1100 (J' 0.6–0.77 and H' of 4.1–5.4), Fish and Boxall (2018) 1,306 and Bertelli et al. (2018) 67 to 1038 OTUs. Of note, however, different types of pipes might accumulate different amounts of biofilm per area or length of pipe so the alpha and beta diversity measures could differ in a similar study depending on which parameter would be used for normalization. Hence, the exact numbers are difficult to compare due to the differences in experimental set-up, as well as due to differences in filtering and reporting strategies. Even when exploring the parallel biofilm sample pairs from the same building (biofilms 7 vs. 47 and 27 vs. 37) revealed only slight (191 vs. 217) or very slight (202 vs. 210) differences in the number of genera. Ji et al. (2017) has also identified a negative correlation between H' and temperature. We did not observe any significant (at FDR 5%) correlations of the alpha diversity measures (S, H', and J') and the 18 environment traits. This suggests that sequencing depth may actually have the strongest effect on the alpha diversity calculations of biofilms, as the number of observed microbial taxa is known to increase with increasing sequencing depth (Weiss et al., 2017). Indeed, before rarefaction, we observed that both S and H' significantly positively correlated (R = 0.7, P = 1.3 x 10−8 and R = 0.4, P = 5.3 x 10−3, respectively) with the total number of sequences in each biofilm sample.
Our beta diversity analysis revealed that the greatest contrast between our biofilm samples could be observed between those biofilms exposed (at least, at the time point of sampling) to different measured water temperature ranges (F = 4.1, P < 0.001) and samples collected in the northern vs. southern part of the sampling area (F = 5.6, P < 0.001; Supplementary Table S5), e.g., biofilms 19 and 11 both originated from pipes with the average measured water temperature of <50°C. At the group level, the 1. group unified samples from the lowest water temperature range, whereas the 2. group including exclusively samples from the southern part, where the river “Daugava” was the primary water source. In line with this, our co-occurrence network analysis (Figure 2 and Supplementary Table S6) identified three modules significantly correlating with water temperature (Supplementary Figure S5). Thereof, the Legionella spp. containing turquoise module unified genera the abundance of which increased with decreasing temperature and when moving toward north (Figure 2B). The blue module contained genera, the abundance of which increased with decreasing water temperature, as well as when moving toward south. In relation to water temperature, both modules were highly similar to each other, forming a so-called meta module (Figure 2C). The brown module, on the other hand, unified genera, the abundance of which increased with increasing water temperature and when moving toward south (Figure 2B). The variance in taxon abundance along the north-south axis (latitude) could be possibly explained by different primary water sources [https://www.rigasudens.lv/en/]. As, for the north part, natural groundwater is supplemented with surface water from the nearby lake. Whereas, for the south part, the surface water from the river “Daugava” is used as the primary water source. Water temperature and source are two well-known environmental factors that significantly affect the abundance and diversity of water pipe biofilms (Fish et al., 2016). However, follow-up studies should include a thorough characterization of the source water, in order to validate these correlations.
At phylum-level, we observed several thermophiles, not previously reported for the cold water systems, such as Chloroflexi, Thermi and Thermotogae. Otherwise, however, our findings (Figure 1A) are in line with a number of other systematic studies, exploring the microbial populations of water supply system biofilms (Yu et al., 2010; Baron et al., 2014; Mahapatra et al., 2015; Douterelo et al., 2018; Van Assche et al., 2018), as, in all our biofilm samples, quantitatively, the most abundant phyla were also Proteobacteria, Firmicutes, Nitrospirae and Bacteroidetes. Interestingly, it has been previously observed that Proteobacteria was predominant in water samples from households that did not complain about their drinking water quality, whereas those with consumer reports of red water and flowing water, containing elevated levels of iron and manganese, had markedly more sequences representing Nitrospira and Pedomicrobium (in 44/45 of our biofilm samples, on average 0.73% of total). Unfortunately, however, we do not have similar data on water quality, to make such comparisons.
We observed several genus-level differences, in comparison to cold-water biofilms (Douterelo et al., 2018), where the most abundant Proteobacteria genera were Massilia, Pseudomonas and Sphingomonas spp. (Douterelo et al., 2018), the latter two known as opportunistic pathogens (Baron et al., 2014). Pseudomonas spp., together with two other pathogen-containing genera—Acinetobacter and Klebsiella spp. have also been identified as the strongest biofilm producers in bacterial isolates from water pipes of kitchens (Mahapatra et al., 2015). We detected only marginal amounts of Pseudomonas (~0.15%) and Sphingomonas spp. (~0.14%), as well as another waterborne pathogen-containing genera (Baron et al., 2014)—Mycobacterium spp. (~0.13%). We did not identify (at >0.1% of total) Massilia, Klebsiella and Acinetobacter spp. (Supplementary Table S3). These differences could be possibly attributed to the measured water temperature, which was, at least, at the time point of sampling, on average, approximately ~52°C in this study. Previously, it has been reported that elevated water temperature (~51°C being the threshold) introduces significant changes with respect to both the phylogenetic composition and predicted functions of the microbiota at the tap (Ji et al., 2017). Moreover, previous investigations (Mahapatra et al., 2015; Douterelo et al., 2018) studied the process of biofilm formation and maturation using controlled laboratory experiments, whereas we analyzed mature biofilms (12 to 61 years old; mean = 36.9) in a field study. The differences in these biofilms could, among others, lie in the density of biomass, i.e., it is reasonable to assume that maturing biofilms would be lower in biomass, as compared to mature ones. It has been demonstrated that genera containing opportunistic pathogens were more common in low-biomass biofilms (Proctor et al., 2018). We also did not identify (at >0.1% of total) Mycobacterium, Nitrosomonas or Methylobacterium spp. (Supplementary Table S3), previously found to dominate chloraminated water distribution systems (Waak et al., 2019).
Interestingly, our genus-level analysis revealed that the two most abundant genera were actually opposites in terms of their oxygen requirements: obligately anaerobic Thermodesulfovibrio (~7.5%) vs. strictly aerobic Phenylobacterium (~2.9%) spp. Such a phenomenon could be explained by the fact that those bacteria located within the exterior surface of the biofilm usually have free access to oxygen, whereas, within the interior of a biofilm, the conditions are more anaerobic (Wang et al., 2017). Hence, it is reasonable to assume that Thermodesulfovibrio reside more within the exterior surface, while Phenylobacterium spp. might be located more toward the interior of a biofilm. Thermodesulfovibrio genus represents thermophilic bacteria found in fresh water hydrothermal environments, where they may oxidize hydrogen and other organic compounds through the reduction of sulfate (Sekiguchi et al., 2008). Recently, it has been identified in thermal water and mud in an Italian spa complex by 16S rRNA amplicon sequencing (Stefania Paduano, 2017), together with a couple of other genera, also present in our biofilms—Desulfomonile(~0.34%) and Geothermobacterium spp. (~0.14%). These similarities can be explained by the fact that both studies investigated real-world and hot water systems. Phenylobacterium genus is well known for its extremely limited nutritional spectrum, growing optimally only on xenobiotic compounds like herbicide chloridazon or analgesic drugs like antipyrin and pyramidon (Lingens et al., 1985). It has been previously reported to be associated with microalga Micrasterias crux-melitensis (Krohn-Molt et al., 2017), but has also been identified in drinking water biofilms (Lu et al., 2014). Hence, its presence could be explained by the fact that surface water is used as one of the water sources and we observe rather high proportions of Archaeplastida in several samples (Figure 4B). The third most abundant genus was an anaerobic and thermophilic bacteria—Moorella (~2.7%), often isolated from hot springs (Slobodkin et al., 1997), whereas the fourth most abundant genus, Gemmata (~2.6%) is interesting for its unusual ability to uptake proteins from the external milieu, i.e., perform “endocytosis” (Lonhienne et al., 2010). Hence, its functional role within a biofilm community merits further investigation.
We used pangenome-based functional predictions to gain some insights on the functional capabilities of the three OTU co-occurrence modules of interest (turquoise, blue and brown), with potential consequences for the domestic water quality and the choice of control measures to minimize the biofilm growth. Functional predictions suggested the presence of genes/proteins related to pathways such as (Figure 3) “Biofilm formation” and “Quorum sensing” cell communication system, required to coordinate the community density within a biofilm (Abisado et al., 2018). Cell communication is tightly related to the secretion of and response to various signaling molecules (Abisado et al., 2018). Indeed, functional predictions suggested the presence of genes/proteins related to “Bacterial secretion system components” and “Transporters,” which are necessary for the transport of nutrients and waste products and hence for biofilm growth and maintenance (Wilking et al., 2013). Similarly, “Bacterial motility and chemotaxis,” another predicted pathway, based on the putative presence of functional genes/proteins, has been also recently demonstrated to play a key role in the development and maintenance of biofilms, as individual cells have been observed to actively move toward nutrients and search for the most favorable positions within a biofilm (Oliveira et al., 2016). On top of that, functional genes/proteins related to “Carbon fixation,” as well as “Sulfur,” “Nitrogen” and “Methane metabolism,” crucial for biofilm growth and maintenance (Fish et al., 2016), were also predicted as possibly present, implying that more complex and tailored control measures may be needed in order to minimize the biofilm growth, depending on the particular composition of the biofilm, as well as on the different environmental and other factors present, collectively shaping the metabolic response of the biofilm, which will tend to maintain its integrity.
Low abundance of Legionella spp. was detected in 44/45 of biofilms—on average, 0.58% of total sequences (Supplementary Table S3), however, there are studies which report even lower abundances of 0.003–0.3% in both chlorinated and unchlorinated water (Gomez-Alvarez et al., 2012; Liu et al., 2017; Bertelli et al., 2018). At the same time, in cooling towers, the leading source of Legionnaires' disease outbreaks, Legionella spp. abundances of 0.06-6.0% has been documented (Pereira et al., 2017). Legionella spp. was part of the turquoise module (Supplementary Table S6), unifying genera the abundance of which increased with decreasing water temperature and when moving toward north (Figure 2B) i.e., could be possibly water source dependent with increased abundance in groundwater. Water temperature is well-known to influence colonization of Legionella spp. and its presence in groundwater has also been documented (Costa et al., 2005). We did not identify (at >0.1% of total) any of the bacterial species that were found to support the adherence and growth of L. pneumophila using laboratory experiments, including Klebsiella pneumoniae, Flavobacterium spp., Empedobacter breve, Pseudomonas putida and fluorescens (Abu Khweek and Amer, 2018). On the other hand, we could detect several genera that can antagonize the persistence of L. pneumophila (Abu Khweek and Amer, 2018) in our samples: genus Sphingomonas and Pseudomonas (~0.15% in 45 and 44/45 samples, respectively), as well as Burkholderia and Acidovorax (<0.5% in 45 and 20/45 samples, respectively). Pseudomonas were part of the brown module, which demonstrated the opposite correlation pattern to the turquoise module (Figure 2B), in line with the previously demonstrated antagonistic interactions between Legionella and Pseudomonas spp. (Paranjape et al., 2020). In addition, we also found a couple of other genera with previously demonstrated anti-Legionella activity: Acinetobacter was also part of the brown module and Bacillus was part of the blue module (Corre et al., 2018).
Host-parasite interactions of Legionella spp. are considered to be crucial for the presence, growth and pathogenesis of these bacteria (Costa et al., 2005), and it utilizes an extensive host range spanning multiple phyla, including Amoebozoa, (amoebae), Excavata and Alveolata (ciliated protozoa) (Boamah et al., 2017). In our 18S rRNA amplicon sequencing data, potential hosts of L. pneumophila were detected in 11/12 biofilm samples analyzed (Supplementary Tables S10, S11), however, in many cases, the relative abundance was very low (<1%; Supplementary Table S10). For example, Echinamoeba thermarum (Amoebozoa) was detected in four biofilms. This amoeba species has been characterized as extremely thermophilic (Baumgartner et al., 2003) and as candidate host for L. pneumophila (Valster et al., 2010). Other confirmed hosts detected were Echinamoeba exundans (9.4%, in biofilm 19) (Fields et al., 1989), Vermamoeba vermiformis (0.5%, in biofilm 19) (Greub and Raoult, 2003) and Filamoeba sp. (4.3%, in 26) (Breiman et al., 1990). Rhizaria and Stramenopiles were represented by several members (especially, in biofilm 35) and they are known to be grazing on L. pneumophila (Amaro et al., 2015). Overall, co-occurrence of L. pneumophila and its potential hosts, was observed in 11 biofilms (Figure 4C and Supplementary Table S11), in two biofilms (15 and 22), using either sequencing or cultivation/microscopy or both of the detection methods, respectively. Interestingly, sample 22 also displayed one of the highest numbers of genera (n = 282), with the most even distribution of genera (J' = 0.71), i.e., the highest genera diversity H' (of 4). It was collected from a 42-year old circulation line (F) of a 53-year old building, where the measured approximate water temperature (at least, at the time point of sampling), was about <50°C, suggesting that the age of the building as well as its pipe system combined with lower temperatures might promote FLP-Legionella spp. interactions. However, as it can be observed in Figure 4B, a large number of sequences remained unassigned (i.e., displayed no similarity with sequences in the reference database), indicating a high abundance of taxa in our samples that were not well represented in the PR2 v4.11.1 reference database (Guillou et al., 2012). Dependence on the reference databases is a well-known major limitation of the sequence alignment-based approaches, used to assign taxa in sequencing studies (Chaudhary et al., 2015; Vilne et al., 2019), especially considering that the proportion of Protozoa/Amoeba that are most probably still not cultivable or not yet known may be large (Guillou et al., 2012).
The drinking water in Riga is supplied from both surface and groundwater sources. In the framework of the Riga Water and Environment Project (in 2001), primary chlorination has been largely replaced by ozonation and biofiltration (Juhna and Klavinš, 2001; Springe and Juhna, 2007), before distributing water to the end point users. Of note, chlorination is still used to some extent, but the concentration of chlorine has been reduced from 0.5 to 0.2 mg l−1. Nevertheless, since 2016, high concentration chlorination (0.5 mg l−1) is being used for three consecutive days every second year to thoroughly disinfect the drinking water supply system. Unfortunately, we lack data on biofilm composition before modifying water disinfection strategy from primary chlorination using high concentration of chlorine (0.5 mg −1) to a mixed approach, combining low concentration of chlorine (0.2 mg −1) with ozonation and biofiltration (except for the three consecutive days every second year when high concentration chlorination (0.5 mg l−1) is applied to thoroughly disinfect the drinking water supply system, introduced in 2016) (Juhna and Klavinš, 2001; Springe and Juhna, 2007). To the best of our knowledge, no identical studies have been described in the scientific literature, hence we are not able to explain our results in terms of this change. Nevertheless, some similar aspects can be found in other studies. For example, integration of ozonation and biofiltration has been studied in Vietnam where a pilot systems during a two-month period effectively removed dissolved organic carbon, reduced the trihalomethane (a harmful disinfection by-product) formation potential, and the concentration of Fe2+ and N-NH4+, which is an important aspect, considering that microorganisms in the water supply systems consume dissolved compounds for their metabolism (Nguyen et al., 2020). Furthermore, in a previous study, in Riga, where two different sampling plots at a distribution network were monitored over a period of 1 year, it was found that, after chlorination, bacterial growth in the water samples was limited by phosphorus and organic carbon content, as well as by the presence of nitrogen and iron (Nescerecka et al., 2018). Both water treatment methods are recommended by WHO, although several pathogens transmitted through drinking-water such as Legionella, Mycobacteria and Pseudomonas aeruginosa survive and grow in biofilms and they can be protected from chlorination (World Health Organization, 2017). It has been detected that 0.2 mg −1 of free chlorine cannot induce a 4-log reduction in L. pneumophila serogroup 1 (Cervero-Aragó et al., 2015), whereas turn ozonation can give significant log inactivation of this serogroup (Domingue et al., 1988). At the same time, there have been studies that do not detect Legionella spp. in biofilms of chloraminated systems in comparison to in biofilms from no-residual systems (Waak et al., 2018). There have also been some studies that do not confirm the effect of ozonation on the presence of Legionella (Blanc et al., 2005), and demonstrate that high water temperatures are more effective (Cervero-Aragó et al., 2015). In this regard, the Legionella spp. containing turquoise module demonstrated highly significant negative correlations with water temperature, supporting the recommendations by WHO for minimal hot water temperatures(>50°C). Other control measures to minimize the biofilm growth, recommended by WHO, include the optimization of organic carbon removal, restriction of water residence time within the distribution systems and maintenance of disinfectant residuals (World Health Organization, 2017).
Regarding the community profiling of the water treatment systems applying ozonation and biofiltration, in our study, we found that at the (phylum-level) bacterial communities contained the so called “core microbiome” of the water-phase that primarily consists of Alpha- and Beta- proteobacteria, and to a lesser extent of Gamma-proteobacteria, Nitrospirae, Planctomycetes, Acidobacteria, Bacteroidetes and Chloroflexi (Zhang and Liu, 2019). Core families among Beta-proteobacteria are Burkholderiaceae, Methylophilaceae, Comamonadaceae, and Rhodocyclaceae, whereas Sphingomonadaceae, Caulobacteraceae and Methylobacteriaceae are dominant in Alpha-proteobacteria. We not that the “core genera and species” and their relation to the disinfectant have not been defined, yet (Bautista-de Los Santos et al., 2016) quoted by Zhang and Liu (2019). In fact, it has been hypothesized that the “core genome” of the biofilm phase might not be easily definable, due to the spatially variable ecological niches and ecological succession (Zhang and Liu, 2019).
Taken together, this is the first study in Latvia, using next generation sequencing to exploring the biofilm communities of drinking water pipes. Clearly, further follow-up investigations should be conducted, including also the left side of “Daugava,” which receives cleaned river water as their primary source of water. The impact of source water also merits further investigations, in conjunction with other environmental factors, considering each apartment building with its heat exchangers as a closed system. In conclusion, our study confirms known correlations between the composition of the hot water pipe biofilms and several environmental factors such as water temperature and source and provides a resource for future studies in Latvia to understand how the environment shapes biofilms, the ecological relationships established within them and how this potentially relates to the household water quality and public health risk. We hope that our results may contribute to future changes in the regulations, such as the recommended minimal hot water temperatures or recommendations to exchange water pipes and mitigation of other distribution system-related risk factors.
Data Availability Statement
The raw sequence data are available from the European Nucleotide Archive (ENA) database under the accession numbers PRJEB27134 (45 samples, for which 16S rRNA amplicon sequencing was performed) and PRJEB31264 (12 samples, for which 18S rRNA amplicon sequencing was performed).
Author Contributions
OV and LG-I designed and supervised the research and wrote the manuscript. BV analyzed the data and wrote the manuscript. JĶ conducted the experiments, analyzed the data, and wrote the manuscript. AM, GK, and SM conducted the experiments. DP collected and processed sample data. All authors participated in revising and editing the manuscript, and have read and approved the final version of the manuscript.
Funding
This research was funded by the National Research Programme of Latvia Agricultural Resources for Sustainable Production on Qualitative and Healthy Foods in Latvia (AgroBioRes) project No. 5 Resistance of microorganisms and other biological and chemical risks research procedures development and application in the food chain (RISKS) (grant no. 10-4/VPP-7/5).
Conflict of Interest
BV is the CEO of SIA net-OMICS, a company providing bioinformatics analyses.
The remaining authors declare that the research was conducted in the absence of any commercial or financial relationships that could be construed as a potential conflict of interest.
Publisher's Note
All claims expressed in this article are solely those of the authors and do not necessarily represent those of their affiliated organizations, or those of the publisher, the editors and the reviewers. Any product that may be evaluated in this article, or claim that may be made by its manufacturer, is not guaranteed or endorsed by the publisher.
Acknowledgments
We would also like to thank the JSC RĪGAS SILTUMS in Latvia, in particular, Uǧis Osis, Artis Benefelds, and Gints Bērze, as well as all the technical workers, who assisted us with the organization and collected of pipe samples from the hot water distribution systems of apartment buildings in Riga during the repair works in May and June 2017. We also thank Valērijs Lopatovs from BIOR for preparing the pipe samples for downstream processing.
Supplementary Material
The Supplementary Material for this article can be found online at: https://www.frontiersin.org/articles/10.3389/frwa.2021.799840/full#supplementary-material
Abbreviations
BH, Benjamini-Hochberg; BSA, bovine serum albumin; ENA, European Nucleotide Archive; FDR, false discovery rate; FLP, free-living protozoa; H', Shannon's diversity index; J', Pielou's evenness; KO, KEGG Orthology; L. pneumophila, Legionella pneumophila; NCBI, National Center for Biotechnology Information; OTU, operational taxonomic unit; PanFP, pangenome-based functional profiles; PAS, Page amoeba saline; PE, paired-end; PYG, Peptone yeast-glucose; rRNA, ribosomal RNA; S, number of different taxa in each sample; spp., ‘several species’; WGCNA, Weighted Correlation Network Analysis.
Footnote
1. ^Ecology analysis using vegan.
References
Abisado, R. G., Benomar, S., Klaus, J. R., Dandekar, A. A., and Chandler, J. R. (2018). Bacterial quorum sensing and microbial community interactions. MBio 9, e02331–e02317. doi: 10.1128/mBio.02331-17
Abu Khweek, A., and Amer, A. O. (2018). Factors mediating environmental biofilm formation by legionella pneumophila. Front. Cell Infect. Microbiol. 8:38. doi: 10.3389/fcimb.2018.00038
Academies, U. N. (2019). Management of Legionella in Water Systems. Technical report, National Academies of Sciences, Engineering, and Medicine, The National Academies Press: Washington, DC.
Amaro, F., Wang, W., Gilbert, J. A., Anderson, O. R., and Shuman, H. A. (2015). Diverse protist grazers select for virulence-related traits in legionella. ISME J. 9, 1607–1618. doi: 10.1038/ismej.2014.248
Baron, J. L., Vikram, A., Duda, S., Stout, J. E., and Bibby, K. (2014). Shift in the microbial ecology of a hospital hot water system following the introduction of an on-site monochloramine disinfection system. PLoS ONE 9:e102679. doi: 10.1371/journal.pone.0102679
Baumgartner, M., Yapi, A., Gröbner-Ferreira, R., and Stetter, K. O. (2003). Cultivation and properties of echinamoeba thermarum n. sp., an extremely thermophilic amoeba thriving in hot springs. Extremophiles 7, 267–274. doi: 10.1007/s00792-003-0319-6
Bautista-de Los Santos, Q. M., Schroeder, J. L., Blakemore, O., Moses, J., Haffey, M., Sloan, W., et al. (2016). The impact of sampling, pcr, and sequencing replication on discerning changes in drinking water bacterial community over diurnal time-scales. Water Res. 90, 216–224. doi: 10.1016/j.watres.2015.12.010
Beauté, J., Plachouras, D., Sandin, S., Giesecke, J., and Sparén, P. (2020). Healthcare-associated legionnaires' disease, europe, 2008-2017. Emerg. Infect. Dis. 26, 2309–2318. doi: 10.3201/eid2610.181889
Benjamini, Y., and Hochberg, Y. (1995). Controlling the false discovery rate: a practical and powerful approach to multiple testing. J. R. Stat. Soc. B. 57, 289–300. doi: 10.1111/j.2517-6161.1995.tb02031.x
Bertelli, C., Courtois, S., Rosikiewicz, M., Piriou, P., Aeby, S., Robert, S., et al. (2018). Reduced chlorine in drinking water distribution systems impacts bacterial biodiversity in biofilms. Front. Microbiol. 9:2520. doi: 10.3389/fmicb.2018.02520
Blanc, D. S., Carrara, P., Zanetti, G., and Francioli, P. (2005). Water disinfection with ozone, copper and silver ions, and temperature increase to control legionella: seven years of experience in a university teaching hospital. J. Hosp Infect. 60, 69–72. doi: 10.1016/j.jhin.2004.10.016
Boamah, D. K., Zhou, G., Ensminger, A. W., and O'Connor, T. J. (2017). From many hosts, one accidental pathogen: the diverse protozoan hosts of legionella. Front. Cell Infect. Microbiol. 7:477. doi: 10.3389/fcimb.2017.00477
Bradley, I. M., Pinto, A. J., and Guest, J. S. (2016). Design and evaluation of illumina miseq-compatible, 18s rrna gene-specific primers for improved characterization of mixed phototrophic communities. Appl. Environ. Microbiol. 82, 5878–5891. doi: 10.1128/AEM.01630-16
Breiman, R. F., Fields, B. S., Sanden, G. N., Volmer, L., Meier, A., and Spika, J. S. (1990). Association of shower use with legionnaires' disease. possible role of amoebae. JAMA 263, 2924–2926. doi: 10.1001/jama.263.21.2924
Callahan, B. J., McMurdie, P. J., and Holmes, S. P. (2017). Exact sequence variants should replace operational taxonomic units in marker-gene data analysis. ISME J. 11, 2639–2643. doi: 10.1038/ismej.2017.119
Cervero-Aragó, S., Rodríguez-Martínez, S., Puertas-Bennasar, A., and Araujo, R. M. (2015). Effect of common drinking water disinfectants, chlorine and heat, on free legionella and amoebae-associated legionella. PLoS ONE 10:e0134726. doi: 10.1371/journal.pone.0134726
Chan, S., Pullerits, K., Keucken, A., Persson, K. M., Paul, C. J., and Rådström, P. (2019). Bacterial release from pipe biofilm in a full-scale drinking water distribution system. NPJ Biofilms Microbiomes 5, 9. doi: 10.1038/s41522-019-0082-9
Chaudhary, N., Sharma, A. K., Agarwal, P., Gupta, A., and Sharma, V. K. (2015). 16s classifier: a tool for fast and accurate taxonomic classification of 16s rrna hypervariable regions in metagenomic datasets. PLoS ONE 10:e0116106. doi: 10.1371/journal.pone.0116106
Chen, W., and Simpson, J. L. C. (2018). Ram: R for Amplicon-Sequencing-Based Microbial-Ecology. R package version 1.2.1.7. Available online at: https://CRAN.R-project.org/package=RAM
Corre, M.-H., Delafont, V., Legrand, A., Berjeaud, J.-M., and Verdon, J. (2018). Exploiting the richness of environmental waterborne bacterial species to find natural, javax.xml.bind.jaxbelement@33d1fb3, competitors. Front. Microbiol. 9:3360. doi: 10.3389/fmicb.2018.03360
Costa, J., Tiago, I., da Costa, M. S., and Veríssimo, A. (2005). Presence and persistence of legionella spp. in groundwater. Appl. Environ. Microbiol. 71, 663–671. doi: 10.1128/AEM.71.2.663-671.2005
Domingue, E. L., Tyndall, R. L., Mayberry, W. R., and Pancorbo, O. C. (1988). Effects of three oxidizing biocides on legionella pneumophila serogroup 1. Appl. Environ. Microbiol. 54, 741–747. doi: 10.1128/aem.54.3.741-747.1988
Douterelo, I., Fish, K., and Boxall, J. (2018). Succession of bacterial and fungal communities within biofilms of a chlorinated drinking water distribution system. Water Res. 141, 74–85. doi: 10.1016/j.watres.2018.04.058
Farhat, M., Moletta-Denat, M., Frére, J., Onillon, S., Trouilhé, M.-C., and Robine, E. (2012). Effects of disinfection on legionella spp., eukarya, and biofilms in a hot water system. Appl. Environ. Microbiol. 78, 6850–6858. doi: 10.1128/AEM.00831-12
Fields, B. S., Sanden, G. N., Barbaree, J. M., Morrill, W. E., Wadowsky, R. M., White, E. H., et al. (1989). Intracellular multiplication of legionella pneumophila in amoebae isolated from hospital hot water tanks. Curr. Microbiol. 18, 131–137. doi: 10.1007/BF01570838
Fish, K. E., and Boxall, J. B. (2018). Biofilm microbiome (re) growth dynamics in drinking water distribution systems are impacted by chlorine concentration. Front. Microbiol. 9:2519. doi: 10.3389/fmicb.2018.02519
Fish, K. E., Osborn, A. M., and Boxall, J. (2016). Characterising and understanding the impact of microbial biofilms and the extracellular polymeric substance (eps) matrix in drinking water distribution systems. Environ. Sci. Water Res. Technol. 2, 614–630. doi: 10.1039/C6EW00039H
Gomez-Alvarez, V., Revetta, R. P., and Santo Domingo, J. W. (2012). Metagenomic analyses of drinking water receiving different disinfection treatments. Appl. Environ. Microbiol. 78, 6095–6102. doi: 10.1128/AEM.01018-12
Greub, G., and Raoult, D. (2003). Morphology of legionella pneumophila according to their location within hartmanella vermiformis. Res. Microbiol. 154, 619–621. doi: 10.1016/j.resmic.2003.08.003
Guillou, L., Bachar, D., Audic, S., Bass, D., Berney, C., Bittner, L., et al. (2012). The protist ribosomal reference database (pr2): a catalog of unicellular eukaryote small sub-unit rrna sequences with curated taxonomy. Nucleic Acids Res. 41, D597–D604. doi: 10.1093/nar/gks1160
Jari Oksanen, F., Blanchet, G., Friendly, M., Kindt, R., Legendre, P., McGlinn, D., et al. (2018). Vegan: Community Ecology Package. R package, version 2.5-2. Available online at: https://CRAN.R-project.org/package=vegan
Ji, P., Rhoads, W. J., Edwards, M. A., and Pruden, A. (2017). Impact of water heater temperature setting and water use frequency on the building plumbing microbiome. ISME J. 11, 1318–1330. doi: 10.1038/ismej.2017.14
Ji, P., Rhoads, W. J., Edwards, M. A., and Pruden, A. (2018). Effect of heat shock on hot water plumbing microbiota and legionella pneumophila control. Microbiome 6:30. doi: 10.1186/s40168-018-0406-7
Jiang, R., Li, W. V., and Li, J. J. (2021). mbimpute: an accurate and robust imputation method for microbiome data. Genome Biol. 22:192. doi: 10.1186/s13059-021-02400-4
Juhna, T., and Klavinš, M. (2001). Water-quality changes in latvia and riga 1980-2000: Possibilities and problems. Ambio 30, 306–315. doi: 10.1579/0044-7447-30.4.306
Jun, S.-R., Robeson, M. S., Hauser, L. J., Schadt, C. W., and Gorin, A. A. (2015). Panfp: pangenome-based functional profiles for microbial communities. BMC Res. Notes 8, 479. doi: 10.1186/s13104-015-1462-8
Klindworth, A., Pruesse, E., Schweer, T., Peplies, J., Quast, C., Horn, M., et al. (2013). Evaluation of general 16s ribosomal rna gene pcr primers for classical and next-generation sequencing-based diversity studies. Nucleic Acids Res. 41:e1. doi: 10.1093/nar/gks808
Krohn-Molt, I., Alawi, M., Förstner, K. U., Wiegandt, A., Burkhardt, L., Indenbirken, D., et al. (2017). Insights into microalga and bacteria interactions of selected phycosphere biofilms using metagenomic, transcriptomic, and proteomic approaches. Front. Microbiol. 8:1941. doi: 10.3389/fmicb.2017.01941
Langfelder, P., and Horvath, S. (2008). Wgcna: an r package for weighted correlation network analysis. BMC Bioinformatics 9:559. doi: 10.1186/1471-2105-9-559
Lingens, F., Blecher, R., Blecher, H., Blobel, F., Eberspächer, J., Fröhner, C., et al. (1985). Phenylobacterium immobile gen. nov., sp. nov., a gram-negative bacterium that degrades the herbicide chloridazon. Int. J. Syst. Evolut. Microbiol. 35, 26–39. doi: 10.1099/00207713-35-1-26
Liu, G., Tao, Y., Zhang, Y., Lut, M., Knibbe, W.-J., van der Wielen, P., et al. (2017). Hotspots for selected metal elements and microbes accumulation and the corresponding water quality deterioration potential in an unchlorinated drinking water distribution system. Water Res. 124, 435–445. doi: 10.1016/j.watres.2017.08.002
Liu, G., Verberk, J., and Van Dijk, J. (2013). Bacteriology of drinking water distribution systems: an integral and multidimensional review. Appl. Microbiol. Biotechnol. 97, 9265–9276. doi: 10.1007/s00253-013-5217-y
Lonhienne, T. G., Sagulenko, E., Webb, R. I., Lee, K.-C., Franke, J., Devos, D. P., et al. (2010). Endocytosis-like protein uptake in the bacterium gemmata obscuriglobus. Proc. Natl. Acad. Sci. U.S.A. 107, 12883–12888. doi: 10.1073/pnas.1001085107
Lu, J., Buse, H., Gomez-Alvarez, V., Struewing, I., Santo Domingo, J., and Ashbolt, N. (2014). Impact of drinking water conditions and copper materials on downstream biofilm microbial communities and l egionella pneumophila colonization. J. Appl. Microbiol. 117, 905–918. doi: 10.1111/jam.12578
Lührig, K., Canbäck, B., Paul, C. J., Johansson, T., Persson, K. M., and Rådström, P. (2015). Bacterial community analysis of drinking water biofilms in southern sweden. Microbes Environ. 30, 99–107. doi: 10.1264/jsme2.ME14123
Mahapatra, A., Padhi, N., Mahapatra, D., Bhatt, M., Sahoo, D., Jena, S., et al. (2015). Study of biofilm in bacteria from water pipelines. J. Clin. Diagn. Res. 9:DC09. doi: 10.7860/JCDR/2015/12415.5715
Marcos-Zambrano, L. J., Karaduzovic-Hadziabdic, K., Loncar Turukalo, T., Przymus, P., Trajkovik, V., Aasmets, O., et al. (2021). Applications of machine learning in human microbiome studies: a review on feature selection, biomarker identification, disease prediction and treatment. Front. Microbiol. 12:634511. doi: 10.3389/fmicb.2021.634511
Moreno-Indias, I., Lahti, L., Nedyalkova, M., Elbere, I., Roshchupkin, G., Adilovic, M., et al. (2021). Statistical and machine learning techniques in human microbiome studies: contemporary challenges and solutions. Front. Microbiol. 12:635781. doi: 10.3389/fmicb.2021.635781
Nearing, J. T., Douglas, G. M., Comeau, A. M., and Langille, M. G. (2018). Denoising the denoisers: an independent evaluation of microbiome sequence error-correction approaches. PeerJ 6:e5364. doi: 10.7717/peerj.5364
Nescerecka, A., Juhna, T., and Hammes, F. (2018). Identifying the underlying causes of biological instability in a full-scale drinking water supply system. Water Res. 135, 11–21. doi: 10.1016/j.watres.2018.02.006
Nguyen, P. D., Le, T. D. Q., Nguyen, N. H., Tran, K. T., Nguyen, M. T., and Huynh, K. A. (2020). Reducing disinfection byproduct precursors and chlorine consuming substances by a special integration of biofiltration and ozonation: a pilot study. J. Water Process Eng. 37:101419. doi: 10.1016/j.jwpe.2020.101419
Oliveira, N. M., Foster, K. R., and Durham, W. M. (2016). Single-cell twitching chemotaxis in developing biofilms. Proc. Natl. Acad. Sci. U.S.A. 113, 6532–6537. doi: 10.1073/pnas.1600760113
Org, E., Blum, Y., Kasela, S., Mehrabian, M., Kuusisto, J., Kangas, A. J., et al. (2017). Relationships between gut microbiota, plasma metabolites, and metabolic syndrome traits in the metsim cohort. Genome Biol. 18, 70. doi: 10.1186/s13059-017-1194-2
Paranjape, K., Bédard, m., Whyte, L. G., Ronholm, J., Prévost, M., and Faucher, S. P. (2020). Presence of legionella spp. in cooling towers: the role of microbial diversity, pseudomonas, and continuous chlorine application. Water Res. 169:115252. doi: 10.1016/j.watres.2019.115252
Pereira, R. P., Peplies, J., Höfle, M. G., and Brettar, I. (2017). Bacterial community dynamics in a cooling tower with emphasis on pathogenic bacteria and legionella species using universal and genus-specific deep sequencing. Water Res. 122, 363–376. doi: 10.1016/j.watres.2017.06.011
Proctor, C. R., Reimann, M., Vriens, B., and Hammes, F. (2018). Biofilms in shower hoses. Water Res. 131, 274–286. doi: 10.1016/j.watres.2017.12.027
Rozentale, B., Bormane, A., Perevosčikovs, J., Lucenko, I., and Brila, A. (2011). Increase of cases of legionellosis in latvia, 2011. Euro Surveill. 16:pii=20009. doi: 10.2807/ese.16.45.20009-en
Schloss, P. D. (2021). Amplicon sequence variants artificially split bacterial genomes into separate clusters. mSphere. 6:e0019121. doi: 10.1128/mSphere.00191-21
Sekiguchi, Y., Muramatsu, M., Imachi, H., Narihiro, T., Ohashi, A., Harada, H., et al. (2008). Thermodesulfovibrio aggregans sp. nov. and thermodesulfovibrio thiophilus sp. nov., anaerobic, thermophilic, sulfate-reducing bacteria isolated from thermophilic methanogenic sludge, and emended description of the genus thermodesulfovibrio. Int. J. Syst. Evolut. Microbiol. 58, 2541–2548. doi: 10.1099/ijs.0.2008/000893-0
Slobodkin, A., Reysenbach, A. L., Mayer, F., and Wiegel, J. (1997). Isolation and characterization of the homoacetogenic thermophilic bacterium moorella glycerini sp. nov. Int. J. Syst. Bacteriol. 47, 969–974. doi: 10.1099/00207713-47-4-969
Springe, G., and Juhna, T. (2007). “Water supply and sanitation in riga: development, present, and future,” in Environmental History of Water: Global Views on Community Water Supply and Sanitation, eds P. S. Juuti, T. S. Katko, and H. S. Vuorinen (New York, NY: Wiley), 401–410.
Stefania, P., Valeriani, F., Romano-Spica, V., Bargellini, A., Borella, P., and Marchesi, I. (2017). Microbial biodiversity of thermal water and mud in an italian spa by metagenomics: a pilot study. Water Supply. 18, 1456–1465. doi: 10.2166/ws.2017.209
Stoeck, T., Bass, D., Nebel, M., Christen, R., Jones, M. D. M., Breiner, H.-W., et al. (2010). Multiple marker parallel tag environmental dna sequencing reveals a highly complex eukaryotic community in marine anoxic water. Mol. Ecol. 19 (Suppl. 1):21–31. doi: 10.1111/j.1365-294X.2009.04480.x
Vaerewijck, M., Sabbe, K., Van Hende, J., Baré, J., and Houf, K. (2010). Sampling strategy, occurrence and diversity of free-living protozoa in domestic refrigerators. J. Appl. Microbiol. 109, 1566–1578. doi: 10.1111/j.1365-2672.2010.04783.x
Valciņa, O., Pūle, D., Lucenko, I., Krastiņa, D., Šteingolde, a., Krūmiņa, A., et al. (2015). Legionella pneumophila seropositivity-associated factors in latvian blood donors. Int. J. Environ. Res. Public Health 13:ijerph13010058. doi: 10.3390/ijerph13010058
Valciņa, O., Pūle, D., Makarova, S., Bērzinš, A., and Krūmiņa, A. (2014). “Occurrence of Legionella Pneumophila in Hot Potable Water in Latvia Medical Basic Sciences,” in 7th International Conference Research and Conservation of Biological Diversity in Baltic Region (Daugavpils: Riga Stradins University), 25–27.
Valster, R. M., Wullings, B. A., and van der Kooij, D. (2010). Detection of protozoan hosts for legionella pneumophila in engineered water systems by using a biofilm batch test. Appl. Environ. Microbiol. 76, 7144–7153. doi: 10.1128/AEM.00926-10
Van Assche, A., Crauwels, S., De Brabanter, J., Willems, K. A., and Lievens, B. (2018). Characterization of the bacterial community composition in water of drinking water production and distribution systems in flanders, belgium. Microbiologyopen 8:e726. doi: 10.1002/mbo3.726
Vaz-Moreira, I., Nunes, O. C., and Manaia, C. M. (2017). Ubiquitous and persistent proteobacteria and other gram-negative bacteria in drinking water. Sci. Total Environ. 586, 1141–1149. doi: 10.1016/j.scitotenv.2017.02.104
Vilne, B., Meistere, I., Grantiņa-Ieviņa, L., and Ķibilds, J. (2019). Machine learning approaches for epidemiological investigations of food-borne disease outbreaks. Front. Microbiol. 10:1722. doi: 10.3389/fmicb.2019.01722
Waak, M. B., Hozalski, R. M., Hallé, C., and LaPara, T. M. (2019). Comparison of the microbiomes of two drinking water distribution systems with and without residual chloramine disinfection. Microbiome 7, 87. doi: 10.1186/s40168-019-0707-5
Waak, M. B., LaPara, T. M., Hallé, C., and Hozalski, R. M. (2018). Occurrence of legionella spp. in water-main biofilms from two drinking water distribution systems. Environ. Sci. Technol. 52, 7630–7639. doi: 10.1021/acs.est.8b01170
Wang, J. C., Cordero, J., Sun, Y., Aranke, M., Wolcott, R., Colmer-Hamood, J. A., et al. (2017). Planktonic growth of pseudomonas aeruginosa around a dual-species biofilm supports the growth of fusobacterium nucleatum within that biofilm. Int. J. Otolaryngol. 2017:3037191. doi: 10.1155/2017/3037191
Wang, Q., Garrity, G. M., Tiedje, J. M., and Cole, J. R. (2007). Naive bayesian classifier for rapid assignment of rrna sequences into the new bacterial taxonomy. Appl. Environ. Microbiol. 73, 5261–5267. doi: 10.1128/AEM.00062-07
Weiss, S., Xu, Z. Z., Peddada, S., Amir, A., Bittinger, K., Gonzalez, A., et al. (2017). Normalization and microbial differential abundance strategies depend upon data characteristics. Microbiome 5, 27. doi: 10.1186/s40168-017-0237-y
Wilking, J. N., Zaburdaev, V., De Volder, M., Losick, R., Brenner, M. P., and Weitz, D. A. (2013). Liquid transport facilitated by channels in bacillus subtilis biofilms. Proc. Natl. Acad. Sci. U.S.A. 110, 848–852. doi: 10.1073/pnas.1216376110
Wolf, M., Müller, T., Dandekar, T., and Pollack, J. D. (2004). Phylogeny of firmicutes with special reference to mycoplasma (mollicutes) as inferred from phosphoglycerate kinase amino acid sequence data. Int. J. Syst. Evol. Microbiol. 54, 871–875. doi: 10.1099/ijs.0.02868-0
World Health Organization (2017). Guidelines for Drinking-Water Quality: First Addendum to the Fourth Edition. Retrieved from: https://www.who.int/publications/i/item/9789241549950 (accesesed April 24, 2021).
Yu, J., Kim, D., and Lee, T. (2010). Microbial diversity in biofilms on water distribution pipes of different materials. Water Sci. Technol. 61, 163–171. doi: 10.2166/wst.2010.813
Keywords: biofilms, 16S/18S rRNA amplicon sequencing, metataxonomics, hot water supply systems, bacterial diversity, free-living protozoa, Legionella pneumophila, co-occurrence networks
Citation: Vilne B, Grantiņa-Ieviņa L, Ķibilds J, Mališevs A, Konvisers G, Makarova S, Pūle D and Valciņa O (2021) Investigating Bacterial and Free-Living Protozoa Diversity in Biofilms of Hot Water Pipes of Apartment Buildings in the City of Riga (Latvia). Front. Water 3:799840. doi: 10.3389/frwa.2021.799840
Received: 22 October 2021; Accepted: 22 November 2021;
Published: 15 December 2021.
Edited by:
Mario Carere, National Institute of Health (ISS), ItalyReviewed by:
Laura Gomez Valero, Institut Pasteur, FranceZhiya Sheng, University of Alberta, Canada
Copyright © 2021 Vilne, Grantiņa-Ieviņa, Ķibilds, Mališevs, Konvisers, Makarova, Pūle and Valciņa. This is an open-access article distributed under the terms of the Creative Commons Attribution License (CC BY). The use, distribution or reproduction in other forums is permitted, provided the original author(s) and the copyright owner(s) are credited and that the original publication in this journal is cited, in accordance with accepted academic practice. No use, distribution or reproduction is permitted which does not comply with these terms.
*Correspondence: Baiba Vilne, YmFpYmEudmlsbmUmI3gwMDA0MDtyc3UubHY=; Lelde Grantiņa-Ieviņa, bGVsZGUuZ3JhbnRpbmEtaWV2aW5hJiN4MDAwNDA7Ymlvci5sdg==; Juris Ķibilds, anVyaXMua2liaWxkcyYjeDAwMDQwO2Jpb3IubHY=