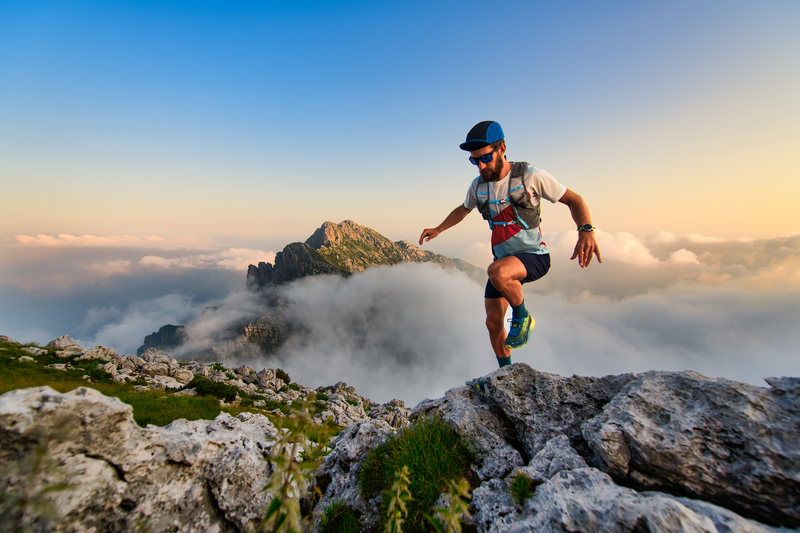
94% of researchers rate our articles as excellent or good
Learn more about the work of our research integrity team to safeguard the quality of each article we publish.
Find out more
MINI REVIEW article
Front. Water , 14 December 2021
Sec. Water and Critical Zone
Volume 3 - 2021 | https://doi.org/10.3389/frwa.2021.767910
This article is part of the Research Topic Critical Zone Geophysics View all 8 articles
Recent advances in measuring and modeling root water uptake along with refined electrical petrophysical models may help fill the existing gap in hydrological root model parametrization. In this paper, we discuss the choices to be made to combine root-zone hydrology and geoelectrical data with the aim of characterizing the active root zone. For each model and observation type we discuss sources of uncertainty and how they are commonly addressed in a stochastic inversion framework. We point out different degrees of integration in the existing hydrogeophysical approaches to parametrize models of root-zone hydrology. This paper aims at giving emphasis to stochastic approaches, in particular to Data Assimilation (DA) schemes, that are generally identified as the best way to combine geoelectrical data with Root Water Uptake (RWU) models. In addition, the study points out a more suitable objective function taken from the optimal transport theory that better captures complex geometry of root systems. Another pathway for improvement of geoelectrical data integration into RWU models using DA relies on the use of stem based methods as a leverage to introduce more extensive root knowledge into RWU macroscopic hydrological models.
The benefits of combining hydrological modeling with abundant information derived from different observations have been successfully demonstrated at least over the past 20 years. In order to support hydrological models of soil-plant interactions, observations are generally retrieved from plants (e.g., water potential measurements–Sus et al., 2014), from the atmosphere (e.g., remote sensing measurements–Gelsinari et al., 2020; Lei et al., 2020), and from the soil (e.g., soil moisture–Han et al., 2012; Gruber et al., 2015; Hu et al., 2017, 2019; Liu et al., 2017; Zhang et al., 2018). However, most of the studies neglect the root architectural traits due to the difficulty in collecting the relevant data. This is a major issue considering the fundamental role of roots in water regulation in the Earth Critical Zone (ECZ) (Newman et al., 2006; Srayeddin and Doussan, 2009). Existing studies combining observation of roots and root-system models are limited to laboratory experiments via X-ray or Magnetic Resonance Imaging (MRI; Koch et al., 2019), but this is not the scale suitable for field and real case applications.
The use of geophysical methods has been long advocated as a possible approach to image roots and root water uptake (e.g., Werban et al., 2008). In particular, geoelectrical methods offer a reliable tool to measure soil-roots interactions. These methods rely on Electrical Resistivity (ER), which is very sensitive to soil moisture (Michot et al., 2003) and salinity content (Kemna et al., 2002). In particular, time lapse measurements have demonstrated their effectiveness in characterizing processes inherent to water movement due to root water uptake (e.g., Garré et al., 2012). Alone, geoelectrical measurements are limited to the observation of state variable changes (moisture content, solute concentration, temperature). This requires that a link with the hydrological processes inducing these state variable changes be made in order to obtain useful information from the data. Also, advances in characterizing the root zone directly using stem-based methods (capacitive and Mise-à-la-masse) (Mary et al., 2020; Peruzzo et al., 2020) are potentially a useful tool in refining hydrological models of roots (Moreno et al., 2015; Cassiani et al., 2020; Cimpoiaşu et al., 2020; Garré et al., 2021).
Great progress has been made over the past decade to combine geophysical observation data with hydrological models (Camporese et al., 2010, 2011; Tso et al., 2020). Relatively few hydro-geophysical applications, though, have been focused on root system parametrization (Manoli et al., 2015; Cassiani et al., 2016; Mary et al., 2020; Rao et al., 2020). In a series of studies, (Kuhl et al., 2018), Kuhl et al. (2021) propose a novel coupled hydrogeophysical approach to estimate evapotranspiration (ET) and root depth from ER, and soil water content combining both soil water content from Time Domain Reflectometry (TDR) and ER measurements with a hydrological and plant model. The data integration using a coupled inversion showed the advantages of ER tomography for extrapolation beyond the local scale of soil water content sensors.
Despite these advancements, many uncertainties due to model errors, data errors, inversion errors, transformation from geophysical to hydrological quantities, and initial conditions need to be accounted for (Rubin and Hubbard, 2005). Stochastic methods provide a systematic framework for assessing or handling some of the complexities that arise in fusing disparate data sets. Their ability to include structural and parametric error distributions make them particularly attractive for application to the problem of dynamic parameter estimation (Linde et al., 2017; Tso et al., 2020).
The inevitable difficulties along the way do not change the value of these approaches for blending geophysical data with hydrological models, as: (i) geophysical data offer spatially extensive information, that can be also collected frequently in time; (ii) the “calibration” of hydrological models identify their own governing parameters on the basis of the (time-lapse) geophysical data, thus giving sense to the signals recorded by the geophysical measurements. Geophysical time lapse survey focused on ways to extract relevant hydrological information using time series analysis techniques (Binley et al., 2015). Relevant time scales for root hydrology range from single measurement to daily, seasonal or even continuous monitoring.
This review paper focuses on the combination of geoelectrical observations and hydrological modeling, with the aim of characterizing the active root zone. We discuss the approaches of model parametrization according to their degree of integration from simple trials to fully coupled inversion. An emphasis is given to Data Assimilation (DA) schemes as a stochastic estimation problem. Finally, the review paves possible ways of improving hydrogeophysical models based on the current state of the art of geoelectrical methods view data assimilation.
Many authors underlined the importance of identifying the best approach to describe RWU. Given the resolution of geoelectrical methods, macroscopic models (e.g., Muma et al., 2013; Vanderborght et al., 2021) describing RWU are often preferred to microscopic (or functional structural) ones (e.g., Javaux et al., 2008). These two types of models are usually based on solving a modified form of the 3D Richards equation to account for a certain root distribution and root/trunk xylem/stomatal hydraulic conductance (Volpe et al., 2013). The two approaches differ in their complexity in considering the inclusion of explicit root hydraulic features to simulate water flow toward individual roots (Doussan, 1998; Javaux et al., 2008). Currently, the link between hydraulic conductance and electrical properties of individual roots is not sufficiently known, limiting the use of combining geoelectrical measurements with microscopic models. In macroscopic models, RWU intervenes as a sink term in the soil water flow equation without solving for flow toward individual roots and thus the root system can be considered using a density formulation from empirical models (Vrugt et al., 2001). Examples of successful applications of macroscopic models are described by Couvreur et al. (2012), Manoli et al. (2014) and Camporese et al. (2015b).
Typical application of macroscopic RWU models assumed the root-zone averaged saturation (Manoli et al., 2014), making the hydrological model segments' length comparable with geophysical ones. Note that one way to simplify the description of microscopic water flow is to upscale hydraulic root architecture models to macroscopic representations of root hydraulics (Vanderborght et al., 2021). The resulting model has basically three macroscopic parameters defined at the soil element scale, or at the plant scale, rather than for each segment of the root system architecture and are more appropriate for comparison with geophysical segments.
The root parameterization employed in hydrological models defines the “state model” which is commonly described by a state vector xSW(t)(e.g., soil water content) with a Prior PDF function p(xSW). This prior PDF quantifies what we know about the state of the system given the prior distribution of the parameters and before considering the observed data.
Equation 1 describes the state vector at time t, F(.) is the evolution operator, m is a model parameter vector with a Prior distribution p(m), and ωt is an uncertainty model error (Linde, 2014; Ghorbanidehno et al., 2020). For instance, soil moisture dynamics are described by the Richards' equation, which is implicitly contained into F(.) and is highly non-linear. The model uncertainty ωt accounts for structural model errors (e.g., misrepresentation of physical processes), errors due to the discretization, parameter errors, initial condition errors, etc. In general, the actual value of each error is unknown, thus it is often considered as a random variable described by a Gaussian distribution.
One more pending issue in the application of RWU models is linked to the uncertainty and difficulty of measuring or predicting Root Length Density (RLD) with depth over time (Cai et al., 2018). Large uncertainties on the RLD definition are likely to lead to wrong estimates of model states. We discuss further how to reduce this uncertainty as a perspective in section Assimilation of Stem-Based Method Observation Data. What about the microscopic models? There isn't a lot mentioned about them.
Electrical resistivity tomography produces a set of injected electrical currents and corresponding voltages. Poisson's equation, which expresses the relationship between the measured voltage and the electrical conductivity of the medium, given the injected current, is used to forward model the electrical potential data (Binley and Kemna, 2005). Electrical conductivity is a property of a medium that quantifies how easily electrical charges are conducted and is linked to the soil properties according to specific petrophysical relationships. The most widely used is Archie's law (Archie, 1942), which empirically defines the dependence of the bulk soil electrical conductivity on porosity, pore water conductivity and soil saturation). Nevertheless, petrophysical relationships are site specific and need to be calibrated before serving as translation between ER and SMC. This implies that petrophysical relationships, such as Archie's law, have some parameters to be calibrated. A review of the existing methods and limitations is presented by Cimpoiaşu et al. (2020). Michot et al. (2016) showed that the petrophysical relationships in the root zone are biased due to the influence of the root system on the measured ER. In a synthetic study, Rao et al. (2019) evaluated how geometrical anisotropy of root networks impact the effective conductivity of the rhizosphere (the zone near the root). As petrophysical model uncertainty can be influenced by the presence of a root system, a better knowledge of root electrical properties would help define the formulation of a mixed root soil ER in order to refine the petrophysical relationships (Ehosioke et al., 2020).
The impact of roots on the impedance properties of the rhizosphere has been observed and modeled, even for relatively small fractional volumes (Rao et al., 2019). This impact can be direct and indirect. The former derives from the direct root-current iteration and depends on the large surface area and capacitance of roots, which, in turns, has been linked to root physiology and suberization (Weigand, 2017; Peruzzo et al., 2021; Tsukanov and Schwartz, 2021). The latter, indirect impact, is a combination of the root-induced changes in the physical, chemical, and biological properties of the rhizosphere, e.g., RWU, root exudation, solute uptake and rhizosphere microbiome changes (Landl et al., 2021).
The translation (or mapping) of soil and root electrical resistivity to SWC defines the so-called ‘observation model'. The observation model (i.e., predicted ER observations) is commonly described by a state vector yER(t) at assimilation time t with a prior PDF function p(yER(t)). HER(.) denotes the observation operator describing the Poisson's equation, xER(t) defines the ER values after petrophysical transformation of xSW(t), n is a parameter vector, and vt is a stochastic model error.
The term vtincorporates both measurement errors and observation model uncertainties, by taking into account the following criteria:
- Petrophysical model uncertainty should be considered in order to avoid the creation of unrepresentative hydrological models from geophysical inversions (Linde, 2014; Brunetti and Linde, 2018; Tso et al., 2019). The Archie's law parameters can be assumed as stochastic multivariate Gaussian distribution vectors (Botto et al., 2018) or as multivariate uniform distributions (Tso et al., 2020).
- Regarding the ERT data, Rossi et al. (2015) stated that the likelihood functions can be obtained from the measurement error PDFs defined as a zero-mean Gaussian measurement with a given variance.
- The uncertainty related to the mismatch between the spatial resolution of the geophysical parameters and the scale that characterizes the hydrogeological parameters should be considered.
The degree of integration of data with models may range from simple trial-and-error calibration (e.g., Binley et al., 2002) to fully coupled hydrogeophysical inversion and DA (e.g., Rajabi et al., 2018). Huisman et al. (2012) discussed whether the information content of the data is sufficient to obtain reliable estimates of model parameters. However, in general, the availability of spatially extensive (and time intensive) data greatly improves the model capability to identify the relevant governing hydraulic parameters, and/or unknown forcing conditions. There are several methods to compare quantitatively observed and simulated data such as moment analysis (e.g., Binley et al., 2002; Monego et al., 2010; Haaken et al., 2017) or the data-domain correlation approach of Johnson et al. (2009) that focuses on maximizing the correlation of hydrological and geophysical time series. Binley et al. (1996) introduced the concept of pixel breakthrough curves for analyzing time-lapse geophysical inversions of solute transport experiments. One may use such system responses to calibrate a hydrological model (e.g., Kemna et al., 2002; Crestani et al., 2015). For instance, Mary et al. (2020) used ERT and Mise-à-la-masse (MALM) data in order to constrain the initial values and boundary conditions of hydrological models. Cassiani et al. (2016) applied hydrological modeling for a quantitative interpretation of the ERT experiment on a plant-root system. The next degree of integration consists in incorporating additional knowledge during two sequential inversions, i.e., applied to the ERT measurements and the Richards' equation. This latter approach does not ensure an accurate quantitative description of the physical state, typically violating the mass balance (Manoli et al., 2015) as a result of resolution limitation in geophysical data. Conversely, a coupled inversion links a hydrological model directly with a forward model of the geophysical data, and the mismatch between measured and modeled geophysical response is minimized (Wagner et al., 2019; Wagner and Uhlemann, 2021). In doing so, the soil hydraulic parameters used in the hydrological model can be optimized, while error propagation is avoided (Rubin and Hubbard, 2005; Vereecken et al., 2006; Binley et al., 2010, 2015; Hinnell et al., 2010). Geolectrical inversion has known issues, typically due to the non-uniqueness of the solution in addition to incomplete or imperfect data due to practical limitations in collecting them. Advantages and drawbacks (structural errors in the hydrological conceptual model, dependency on known petrophysical relationships) of coupled inversions have been discussed by many authors (e.g., Hinnell et al., 2010; Camporese et al., 2015a; Slater and Binley, 2021; Yu et al., 2021). An emerging method for combining model predictions with observations is provided by the DA framework. The biggest advantage of DA methods over classical inversion is that they do not require inverted ER values, thus reducing the uncertainty in the estimation of the hydrological quantities, since no artifacts are inserted in the method by solving a classical inverse problem (see section Model Parametrization). Several studies show the promises of DA schemes for dynamic model parametrization in the context of soil-plant modeling (e.g., Han et al., 2012; Hu et al., 2017, 2019).
As a choice for the prior information we generally want to define minimally subjective Prior PDFs (see sections Root water uptake models, Formulating a Soil/Root Electrical Conductivity Model and the Petrophysical Relationship). A Gaussian prior will enforce smoothness via the prior covariance. Hence, Gaussian priors are not suitable for characterizing discontinuous (non-smooth) fields and we would prefer edge-preserving priors (Arridge et al., 2019). Note that prior information is also useful to constrain the deterministic inversion by setting the lower and upper bounds for each parameter (e.g., Kuhl et al., 2021). Prior knowledge can be estimated from onsite calibration measurement or from literature values.
The number of parameters governing a hydrological system can be high. A general rule of thumb is that parameters that are not being updated are assumed to be known, and literature values are used throughout the DA process. In order to decide which of the model and observation parameters need to be fixed, a global sensitivity analysis is often useful. To this end, Kuhl et al. (2021) quantified the sensitivity of each tested value by computing the root mean square error (RMSE) between the reference synthetic ER data and the perturbed ER data modeled. More advanced approaches can be used such as the Morris' method (1991) employed to determine the few most influential parameters among a large number of parameters (Hu et al., 2017).
Both for deterministic coupled hydrogeophysical inversion and DA methods, an objective function (or cost function) is defined, which expresses the misfit between observations and model predictions. This function can take different forms when considering the hydrogeophysical inversion (Rubin and Hubbard, 2005; Vereecken et al., 2006; Hinnell et al., 2010; Mboh et al., 2012).
To express the objective function for the variational approach of DA, we follow the nomenclature used by Ning et al. (2014). In the general case, we aim at estimating a time trajectory of the state vector x = [x0, x1…, xT] (with the time steps ranging from 1 to T and T being the assimilation window), by minimizing the cost function:
The cost function is explicitly expressed as the sum of three components to draw the similarities with classical inversion of hydrogeophysical data. Jb(x), Jq(x) and J0(x) stand respectively for the a priori, the model and the data term. Here, xbis a prior estimate of the true initial state commonly referred to as the background state. The notation represents the weighted quadratic norm, that is, eTP−1e, where T is the transpose operator, and P represents the covariances of the error e (same apply for and where Q and R represent the covariances of the error e for the model and the data weighted quadratic norm, respectively). In section Optimal Mass Transport Metric in Variational Data Assimilation we introduce a new objective function based on a different norm in order to improve the estimation of the misfit for RWU models.
After the prediction step (Eqs. 1, 2), DA requires one additional step called update. This sequence is repeated recursively to estimate unknown states at multiple times of the system's evolution when observations are available. Note that this constitutes the main difference with single-step (also termed “batch”) geostatistical inversion where all the time steps are assimilated at once. Among DA approaches, we distinguish between Bayesian filters and Bayesian smoothers. While Bayesian filters (e.g., Kalman Filter, KF) in its basic form only computes estimates of the current state of the system given the history of measurements, Bayesian smoothers (e.g., Kalman Smoother, KS) can be used to reconstruct states that happened before the current time. Both filters and smoothers suffer from the computational cost related to the propagation over time of the covariance matrices. To overcome this limitation, the covariance matrices can be reduced by representing them with a number of random realizations generated from an appropriate distribution (Ensemble based methods). The Kalman filter can be extended to the Ensemble Kalman filter (EnKF) which allows for non-linear models but is limited by the Gaussian assumption. An alternative is the Particle Filter (PF) approach which extends the application of DA methods to non-Gaussian parameters. Many references report successful applications of both smoother and filter families to integrate geophysical observations (Pasetto et al., 2012; Manoli et al., 2015; Rossi et al., 2015) but also plant and atmospheric observations (Dong et al., 2016) into hydrological models.
DA algorithms rely on the state model, the observations and the observation models (petrophysical relationships) described already in sections Root Water Uptake Models and Formulating a Soil/Root Electrical Conductivity Model and the Petrophysical Relationship. For the specific case of improving the combination of geoelectrical data with hydrological root model we propose two pathways for improvement.
In section Definition of the Objective Function, we have seen that in most cases the discrepancy between measured and predicted data is expressed as the Euclidean L2 misfit function. Nevertheless, in reality, as the root system forms a network this norm is not optimal to capture its complex geometry. Recent studies show the potential of the optimal transport theory in defining a more suitable objective function based on the Wasserstein distance (Eq. 4, based on the formalism of Ning et al., 2014).
Equation 4 describes the minimization of the time trajectory of the state vector x = [x0, x1…, xT]. Compared to Eq. 3, only the term corresponding to the measurement error is still quantified by a quadratic norm, under the assumption that observational errors () are well-represented by additive (Gaussian) random noise. The other terms are based on the Wasserstein metric W.
Several studies report the advantages of using Wasserstein distance compared to Euclidean distance. For example, Ning et al. (2014) advocate the relevance of transportation metrics for quantifying non-random model error in variational DA for non-negative natural states and fluxes when Prior knowledge is not always reliably defined. Similarly, Métivier et al. (2016) use the Kantorovich–Rubinstein (KR) norm as an optimal transport distance to improve inversion of full waveform seismic tomography while Hernández and Liang (2018) propose a hybrid Bayesian and variational data assimilation method for high-resolution hydrologic forecasting.
Most of the studies cited above consider empirical functions describing the root system length or density needed for the macroscopic RWU model. Stem based geoelectrical methods such as electrical capacitance or MALM methods aim at retrieving the RLD via the distribution of current source density (CSD) (Dalton, 1995; Peruzzo et al., 2020). In order to reduce the solution's non-uniqueness we can use: (i) a simplified observation model approach which consists in informing the root-zone sink term used in macroscopic RWU model with the known RLD inferred from inverted CSD data (see e.g., Mary et al., 2019), (ii) a more accurate model approach with a forward model predicting the CSD based on the solution of the Poisson equation. This DA approach would allow an assimilation of either ERT or both ERT and CSD datasets, taking into account the associated measurement uncertainties.
Time lapse ERT measurements are the most informative tools to derive soil water movement and variation of soil water content due to root water uptake. Thus, it appears natural to cast geoelectrical monitoring data within a dynamical parametrization framework. This is especially straightforward considering the non-linearity and complexity of the soil-plant system and all the uncertainties that need to be accounted for. Improving our understanding of soil-plant interactions requires the development of coupled numerical hydrogeophysical models and data assimilation methods. Indeed, the governing parameters of soil-plant systems are likely non-Gaussian variables and the forward and translation models are highly non-linear. The parametrization strategy must then be adapted from coupled optimizers, specific DA schemes (Particle Filters) and/or improved error estimations (Wasserstein). Additionally, the recent advances in characterizing roots using geoelectrical methods such as stem based ones can be seen as a leverage to introduce more extensive root and soil knowledge into the hydrological model. We advocated the use of macroscopic RWU models which have the advantage of being easily informed by recent development in root imaging (CSD). Considering that one of the biggest challenges is to evaluate uncertainties in the model parameters, geophysical data and forcing data, we can conclude that there is an obvious advantage of the Bayesian approach over deterministic methods.
BM: writing-original draft. All authors contributed equally to the manuscript writing-review and editing.
The authors declare that the research was conducted in the absence of any commercial or financial relationships that could be construed as a potential conflict of interest.
All claims expressed in this article are solely those of the authors and do not necessarily represent those of their affiliated organizations, or those of the publisher, the editors and the reviewers. Any product that may be evaluated in this article, or claim that may be made by its manufacturer, is not guaranteed or endorsed by the publisher.
We wish to acknowledge support from the project ECZ-Dry: New technologies to monitor the Earth Critical Zone in water-limited ecosystems funded by Italy-Israel Scientific and Technological Cooperation Programme (Scientific Track 2018). BM acknowledges the financial support from European Union's Horizon 2020 research and innovation programme under a Marie Sklodowska-Curie grant agreement (Grant no. 842922).
ECZ, Earth Critical Zone; ET, Evapotranspiration; RWU, Root water uptake; ER(T), Electrical Resistivity (Tomography); SHP, Soil Hydraulic Properties; PF, Particle Filter; PP, Petrophysical Parameters; RP, Root Parameters; RLD, Root Length Density; EnKF, Ensemble Kalman Filter; KS, Kalman Smoother; ES, Ensemble Smoother; PDF, probability density function; OMT, Optimal Mass Transport; CSD, Current Source Density; MALM, Mise-à-la-masse; SWC, Soil Water Content.
Archie, G.. (1942). The electrical resistivity log as an aid in determining some reservoir characteristics. Trans. AIME 146, 54–62. doi: 10.2118/942054-G
Arridge, S., Maass, P., Öktem, O., and Schönlieb, C.-B. (2019). Solving inverse problems using data-driven models. Acta Numerica 28, 1–174. doi: 10.1017/S0962492919000059
Binley, A., Cassiani, G., and Deiana, R. (2010). Hydrogeophysics: opportunities and challenges. Bollettino di Geofisica Teorica ed Applicata 51. Available online at: https://eprints.lancs.ac.uk/id/eprint/50326/ (accessed November 29, 2021).
Binley, A., Cassiani, G., Middleton, R., and Winship, P. (2002). Vadose zone flow model parametrisation using cross-borehole radar and resistivity imaging. J. Hydrol. 267, 147–159. doi: 10.1016/S0022-1694(02)00146-4
Binley, A., Henry-Poulter, S., and Shaw, B. (1996). Examination of solute transport in an undisturbed soil column using electrical resistance tomography. Water Resour. Res. 32, 763–769. doi: 10.1029/95WR02995
Binley, A., Hubbard, S. S., Huisman, J. A., Revil, A., Robinson, D. A., Singha, K., et al. (2015). The emergence of hydrogeophysics for improved understanding of subsurface processes over multiple scales. Water Resour. Res. 51, 3837–3866. doi: 10.1002/2015WR017016
Binley, A., and Kemna, A. (2005). “DC resistivity and induced polarization methods,” in Hydrogeophysics, eds Y. Rubin and S. S. Hubbard (Dordrecht: Springer Netherlands), 129–156.
Botto, A., Belluco, E., and Camporese, M. (2018). Multi-source data assimilation for physically based hydrological modeling of an experimental hillslope. Hydrol. Earth Syst. Sci. 22, 4251–4266. doi: 10.5194/hess-22-4251-2018
Brunetti, C., and Linde, N. (2018). Impact of petrophysical uncertainty on Bayesian hydrogeophysical inversion and model selection. Adv. Water Resour. 111, 346–359. doi: 10.1016/j.advwatres.2017.11.028
Cai, G., Vanderborght, J., Couvreur, V., Mboh, C. M., and Vereecken, H. (2018). Parameterization of root water uptake models considering dynamic root distributions and water uptake compensation. Vadose Zone J. 17:160125. doi: 10.2136/vzj2016.12.0125
Camporese, M., Cassiani, G., Deiana, R., and Salandin, P. (2011). Assessment of local hydraulic properties from electrical resistivity tomography monitoring of a three-dimensional synthetic tracer test experiment: ASSESSMENT OF LOCAL HYDRAULIC PROPERTIES FROM ERT. Water Resour. Res. 47. doi: 10.1029/2011WR010528
Camporese, M., Cassiani, G., Deiana, R., Salandin, P., and Binley, A. (2015a). Coupled and uncoupled hydrogeophysical inversions using ensemble Kalman filter assimilation of ERT-monitored tracer test data. Water Resour. Res. 51, 3277–3291. doi: 10.1002/2014WR016017
Camporese, M., Daly, E., and Paniconi, C. (2015b). Catchment-scale Richards equation-based modeling of evapotranspiration via boundary condition switching and root water uptake schemes. Water Resour. Res. 51, 5756–5771. doi: 10.1002/2015WR017139
Camporese, M., Paniconi, C., Putti, M., and Orlandini, S. (2010). Surface-subsurface flow modeling with path-based runoff routing, boundary condition-based coupling, and assimilation of multisource observation data: SURFACE-SUBSURFACE FLOW MODELING. Water Resour. Res. 46. doi: 10.1029/2008WR007536
Cassiani, G., Boaga, J., Barone, I., Perri, M. T., Deidda, G. P., Vignoli, G., et al. (2020). “Chapter 3–ground-based remote sensing of the shallow subsurface: geophysical methods for environmental applications,” in Developments in Earth Surface Processes, Vol. 23, eds P. Tarolli and S. M. Mudd (Amsterdam: Elsevier), 55–89.
Cassiani, G., Boaga, J., Rossi, M., Putti, M., Fadda, G., Majone, B., et al. (2016). Soil-plant interaction monitoring: Small scale example of an apple orchard in Trentino, North-Eastern Italy. Sci. Total Environ. 543, 851–861. doi: 10.1016/j.scitotenv.2015.03.113
Cimpoiaşu, M. O., Kuras, O., Pridmore, T., and Mooney, S. J. (2020). Potential of geoelectrical methods to monitor root zone processes and structure: a review. Geoderma 365:114232. doi: 10.1016/j.geoderma.2020.114232
Couvreur, V., Vanderborght, J., and Javaux, M. (2012). A simple three-dimensional macroscopic root water uptake model based on the hydraulic architecture approach. Hydrol. Earth Syst. Sci. 16, 2957–2971. doi: 10.5194/hess-16-2957-2012
Crestani, E., Camporese, M., and Salandin, P. (2015). Assessment of hydraulic conductivity distributions through assimilation of travel time data from ERT-monitored tracer tests. Adv. Water Resour. 84, 23–36. doi: 10.1016/j.advwatres.2015.07.022
Dalton, F. N.. (1995). In-situ root extent measurements by electrical capacitance methods. Plant Soil 173, 157–165. doi: 10.1007/BF00155527
Dong, Q., Zhan, C., Wang, H., Wang, F., and Zhu, M. (2016). A review on evapotranspiration data assimilation based on hydrological models. J. Geogr. Sci. 26, 230–242. doi: 10.1007/s11442-016-1265-4
Doussan, C.. (1998). Modelling of the hydraulic architecture of root systems: an integrated approach to water absorption-distribution of axial and radial conductances in maize. Ann. Bot. 81, 225–232. doi: 10.1006/anbo.1997.0541
Ehosioke, S., Nguyen, F., Rao, S., Kremer, T., Placencia-Gomez, E., Huisman, J. A., et al. (2020). Sensing the electrical properties of roots: a review. Vadose Zone J. 19:e20082. doi: 10.1002/vzj2.20082
Garré, S., Günther, T., Diels, J., and Vanderborght, J. (2012). Evaluating experimental design of ERT for soil moisture monitoring in contour hedgerow intercropping systems. Vadose Zone J. 11:vzj2011.0186. doi: 10.2136/vzj2011.0186
Garré, S., Hyndman, D., Mary, B., and Werban, U. (2021). Geophysics conquering new territories: the rise of “agrogeophysics.” Vadose Zone J. 20:e20115. doi: 10.1002/vzj2.20115
Gelsinari, S., Doble, R., Daly, E., and Pauwels, V. R. N. (2020). Feasibility of improving groundwater modeling by assimilating evapotranspiration Rates. Water Resour. Res. 56:e2019WR025983. doi: 10.1029/2019WR025983
Ghorbanidehno, H., Kokkinaki, A., Lee, J., and Darve, E. (2020). Recent developments in fast and scalable inverse modeling and data assimilation methods in hydrology. J. Hydrol. 591:125266. doi: 10.1016/j.jhydrol.2020.125266
Gruber, A., Crow, W., Dorigo, W., and Wagner, W. (2015). The potential of 2D Kalman filtering for soil moisture data assimilation. Remote Sens. Environ. 171, 137–148. doi: 10.1016/j.rse.2015.10.019
Haaken, K., Deidda, G. P., Cassiani, G., Deiana, R., Putti, M., Paniconi, C., et al. (2017). Flow dynamics in hyper-saline aquifers: hydro-geophysical monitoring and modeling. Hydrol. Earth Syst. Sci. 21, 1439–1454. doi: 10.5194/hess-21-1439-2017
Han, E., Merwade, V., and Heathman, G. C. (2012). Application of data assimilation with the Root Zone Water Quality Model for soil moisture profile estimation in the upper Cedar Creek, Indiana. Hydrol. Process. 26, 1707–1719. doi: 10.1002/hyp.8292
Hernández, F., and Liang, X. (2018). Hybridizing Bayesian and variational data assimilation for high-resolution hydrologic forecasting. Hydrol. Earth Syst. Sci. 22, 5759–5779. doi: 10.5194/hess-22-5759-2018
Hinnell, A. C., Ferré, T. P. A., Vrugt, J. A., Huisman, J. A., Moysey, S., Rings, J., et al. (2010). Improved extraction of hydrologic information from geophysical data through coupled hydrogeophysical inversion. Water Resour. Res. 46. doi: 10.1029/2008WR007060
Hu, S., Shi, L., Huang, K., Zha, Y., Hu, X., Ye, H., et al. (2019). Improvement of sugarcane crop simulation by SWAP-WOFOST model via data assimilation. Field Crops Res. 232, 49–61. doi: 10.1016/j.fcr.2018.12.009
Hu, S., Shi, L., Zha, Y., Williams, M., and Lin, L. (2017). Simultaneous state-parameter estimation supports the evaluation of data assimilation performance and measurement design for soil-water-atmosphere-plant system. J. Hydrol. 555, 812–831. doi: 10.1016/j.jhydrol.2017.10.061
Huisman, J. A., Vrugt, J. A., and Ferre, T. P. A. (2012). Vadose zone model–data fusion: state of the art and future challenges. Vadose Zone J. 11:vzj2012.0140. doi: 10.2136/vzj2012.0140
Javaux, M., Schröder, T., Vanderborght, J., and Vereecken, H. (2008). Use of a three-dimensional detailed modeling approach for predicting root water uptake. Vadose Zone J. 7, 1079–1088. doi: 10.2136/vzj2007.0115
Johnson, T. C., Versteeg, R. J., Huang, H., and Routh, P. S. (2009). Data-domain correlation approach for joint hydrogeologic inversion of time-lapse hydrogeologic and geophysical data. GEOPHYSICS 74, F127–F140. doi: 10.1190/1.3237087
Kemna, A., Vanderborght, J., Kulessa, B., and Vereecken, H. (2002). Imaging and characterisation of subsurface solute transport using electrical resistivity tomography (ERT) and equivalent transport models. J. Hydrol. 267, 125–146. doi: 10.1016/S0022-1694(02)00145-2
Koch, A., Meunier, F., Vanderborght, J., Garré, S., Pohlmeier, A., and Javaux, M. (2019). Functional-structural root-system model validation using a soil MRI experiment. J. Exp. Bot. 70, 2797–2809. doi: 10.1093/jxb/erz060
Kuhl, A. S., Kendall, A. D., Dam, R. L., van Hamilton, S. K., and Hyndman, D. W. (2021). Root water uptake of biofuel crops revealed by coupled electrical resistivity and soil water content measurements. Vadose Zone J. 20:e20124. doi: 10.1002/vzj2.20124
Kuhl, A. S., Kendall, A. D., Van Dam, R. L., and Hyndman, D. W. (2018). Quantifying soil water and root dynamics using a coupled hydrogeophysical inversion. Vadose Zone J. 17:170154. doi: 10.2136/vzj2017.08.0154
Landl, M., Haupenthal, A., Leitner, D., Kroener, E., Vetterlein, D., Bol, R., et al. (2021). Simulating rhizodeposition patterns around growing and exuding root systems. In Silico Plants 3:diab028. doi: 10.1093/insilicoplants/diab028
Lei, F., Crow, W. T., Kustas, W. P., Dong, J., Yang, Y., Knipper, K. R., et al. (2020). Data assimilation of high-resolution thermal and radar remote sensing retrievals for soil moisture monitoring in a drip-irrigated vineyard. Remote Sens. Environ. 239:111622. doi: 10.1016/j.rse.2019.111622
Linde, N.. (2014). Falsification and corroboration of conceptual hydrological models using geophysical data. WIREs Water 1, 151–171. doi: 10.1002/wat2.1011
Linde, N., Ginsbourger, D., Irving, J., Nobile, F., and Doucet, A. (2017). On uncertainty quantification in hydrogeology and hydrogeophysics. Adv. Water Resour. 110, 166–181. doi: 10.1016/j.advwatres.2017.10.014
Liu, Y., Wang, W., and Hu, Y. (2017). Investigating the impact of surface soil moisture assimilation on state and parameter estimation in SWAT model based on the ensemble Kalman filter in upper Huai River basin. J. Hydrol. Hydromech. 65, 123–133. doi: 10.1515/johh-2017-0011
Manoli, G., Bonetti, S., Domec, J.-C., Putti, M., Katul, G., and Marani, M. (2014). Tree root systems competing for soil moisture in a 3D soil-plant model. Adv. Water Resour. 66, 32–42. doi: 10.1016/j.advwatres.2014.01.006
Manoli, G., Rossi, M., Pasetto, D., Deiana, R., Ferraris, S., Cassiani, G., et al. (2015). An iterative particle filter approach for coupled hydro-geophysical inversion of a controlled infiltration experiment. J. Comput. Physics 283, 37–51. doi: 10.1016/j.jcp.2014.11.035
Mary, B., Peruzzo, L., Boaga, J., Cenni, N., Schmutz, M., Wu, Y., et al. (2020). Time-lapse monitoring of root water uptake using electrical resistivity tomography and mise-à-la-masse: a vineyard infiltration experiment. Soil 6, 95–114. doi: 10.5194/soil-6-95-2020
Mary, B., Vanella, D., Consoli, S., and Cassiani, G. (2019). Assessing the extent of citrus trees root apparatus under deficit irrigation via multi-method geo-electrical imaging. Sci. Rep. 9:9913. doi: 10.1038/s41598-019-46107-w
Mboh, C. M., Huisman, J. A., Van Gaelen, N., Rings, J., and Vereecken, H. (2012). Coupled hydrogeophysical inversion of electrical resistances and inflow measurements for topsoil hydraulic properties under constant head infiltration. Near Surf. Geophys. 10, 413–426. doi: 10.3997/1873-0604.2012009
Métivier, L., Brossier, R., Mérigot, Q., Oudet, E., and Virieux, J. (2016). An optimal transport approach for seismic tomography: application to 3D full waveform inversion. Inverse Probl. 32:115008. doi: 10.1088/0266-5611/32/11/115008
Michot, D., Benderitter, Y., Dorigny, A., Nicoullaud, B., King, D., and Tabbagh, A. (2003). Spatial and temporal monitoring of soil water content with an irrigated corn crop cover using surface electrical resistivity tomography: SOIL WATER STUDY USING ELECTRICAL RESISTIVITY. Water Resour. Res. 39. doi: 10.1029/2002WR001581
Michot, D., Thomas, Z., and Adam, I. (2016). Nonstationarity of the electrical resistivity and soil moisture relationship in a heterogeneous soil system: a case study. SOIL 2, 241–255. doi: 10.5194/soil-2-241-2016
Monego, M., Cassiani, G., Deiana, R., Putti, M., Passadore, G., and Altissimo, L. (2010). Tracer test in a shallow heterogeneous aquifer monitored via time-lapse surface ERT. Geophysics 75, WA61–WA73. doi: 10.1190/1.3474601
Moreno, Z., Arnon-Zur, A., and Furman, A. (2015). Hydro-geophysical monitoring of orchard root zone dynamics in semi-arid region. Irrig. Sci. 33, 303–318. doi: 10.1007/s00271-015-0467-3
Muma, M., Gumiere, S. J., Rousseau, A. N., Scudeler, C., and Paniconi, C. (2013). Implementation of a root water extraction module in CATHY: comparison of four empirical root-density distribution models. Proc. Environ. Sci. 19, 57–66. doi: 10.1016/j.proenv.2013.06.007
Newman, B. D., Wilcox, B. P., Archer, S. R., Breshears, D. D., Dahm, C. N., Duffy, C. J., et al. (2006). Ecohydrology of water-limited environments: a scientific vision: OPINION. Water Resour. Res. 42. doi: 10.1029/2005WR004141
Ning, L., Carli, F. P., Ebtehaj, A. M., Foufoula-Georgiou, E., and Georgiou, T. T. (2014). Coping with model error in variational data assimilation using optimal mass transport. Water Resour. Res. 50, 5817–5830. doi: 10.1002/2013WR014966
Pasetto, D., Camporese, M., and Putti, M. (2012). Ensemble Kalman filter versus particle filter for a physically-based coupled surface-subsurface model. Adv. Water Res. 47, 1–13. doi: 10.1016/j.advwatres.2012.06.009
Peruzzo, L., Chou, C., Wu, Y., Schmutz, M., Mary, B., Wagner, F. M., et al. (2020). Imaging of plant current pathways for non-invasive root Phenotyping using a newly developed electrical current source density approach. Plant Soil 450, 567–584. doi: 10.1007/s11104-020-04529-w
Peruzzo, L., Liu, X., Chou, C., Blancaflor, E. B., Zhao, H., Ma, X., et al. (2021). Three-channel electrical impedance spectroscopy for field-scale root phenotyping. Plant Phenome J. 4:e20021. doi: 10.1002/ppj2.20021
Rajabi, M. M., Ataie-Ashtiani, B., and Simmons, C. T. (2018). Model-data interaction in groundwater studies: review of methods, applications and future directions. J. Hydrol. 567, 457–477. doi: 10.1016/j.jhydrol.2018.09.053
Rao, S., Lesparre, N., Flores-Orozco, A., Wagner, F., Kemna, A., and Javaux, M. (2020). Imaging plant responses to water deficit using electrical resistivity tomography. Plant Soil 454, 261–281. doi: 10.1007/s11104-020-04653-7
Rao, S., Meunier, F., Ehosioke, S., Lesparre, N., Kemna, A., Nguyen, F., et al. (2019). Impact of maize roots on soil-root electrical conductivity: a simulation study. Vadose Zone J. 18:190037. doi: 10.2136/vzj2019.04.0037
Rossi, M., Manoli, G., Pasetto, D., Deiana, R., Ferraris, S., Strobbia, C., et al. (2015). Coupled inverse modeling of a controlled irrigation experiment using multiple hydro-geophysical data. Adv. Water Resour. 82, 150–165. doi: 10.1016/j.advwatres.2015.03.008
Rubin, Y., and Hubbard, S. S., (eds.). (2005). Hydrogeophysics. https://www.google.com/search?sxsrf=AOaemvK3O-dWBUYFUFZpTRITDSMVOT-uhg:1637595126321&q=Dordrecht&stick=H4sIAAAAAAAAAOPgE-LSz9U3SMpILi-3UOIAsbOSKwy1jDLKrfST83NyUpNLMvPz9POL0hPzMqsSQZxiq4zUxJTC_0sSiktSiYoWcGSw8CJWTpf8opSi1OSMkh2sjABToWIZWwAAAA&sa=X&ved=2ahUKEwja3Kiepaz0AhWWyjgGHSPjBf8QmxMoAXoECDwQAw
Slater, L., and Binley, A. (2021). Advancing hydrological process understanding from long-term resistivity monitoring systems. WIREs Water 8:e1513. doi: 10.1002/wat2.1513
Srayeddin, I., and Doussan, C. (2009). Estimation of the spatial variability of root water uptake of maize and sorghum at the field scale by electrical resistivity tomography. Plant Soil 319, 185–207. doi: 10.1007/s11104-008-9860-5
Sus, O., Poyatos, R., Barba, J., Carvalhais, N., Llorens, P., Williams, M., et al. (2014). Time variable hydraulic parameters improve the performance of a mechanistic stand transpiration model. A case study of Mediterranean Scots pine sap flow data assimilation. Agric. For. Meteorol. 198–199, 168–180. doi: 10.1016/j.agrformet.2014.08.009
Tso, C.-H. M., Johnson, T. C., Song, X., Chen, X., Kuras, O., Wilkinson, P., et al. (2020). Integrated hydrogeophysical modelling and data assimilation for geoelectrical leak detection. J. Contam. Hydrol. 234:103679. doi: 10.1016/j.jconhyd.2020.103679
Tso, C. M., Kuras, O., and Binley, A. (2019). On the field estimation of moisture content using electrical geophysics: the impact of petrophysical model uncertainty. Water Resour. Res. 55, 7196–7211. doi: 10.1029/2019WR024964
Tsukanov, K., and Schwartz, N. (2021). Modeling plant roots spectral induced polarization signature. Geophys. Res. Lett. 48:e2020GL090184. doi: 10.1029/2020GL090184
Vanderborght, J., Couvreur, V., Meunier, F., Schnepf, A., Vereecken, H., Bouda, M., et al. (2021). From hydraulic root architecture models to macroscopic representations of root hydraulics in soil water flow and land surface models. Hydrol. Earth Syst. Sci. 25, 4835–4860. doi: 10.5194/hess-25-4835-2021
Vereecken, H., Binley, A., Cassiani, G., Revil, A., and Titov, K., (eds.). (2006). Applied Hydrogeophysics. Dordrecht: Springer Netherlands. Available online at: https://www.springer.com/gp/book/9781402049101 (accessed November 29, 2021).
Volpe, V., Marani, M., Albertson, J. D., and Katul, G. (2013). Root controls on water redistribution and carbon uptake in the soil–plant system under current and future climate. Adv. Water Resour. 60, 110–120. doi: 10.1016/j.advwatres.2013.07.008
Vrugt, J. A., Wijk, M. T., van Hopmans, J. W., and Šimunek, J. (2001). One-, two-, and three-dimensional root water uptake functions for transient modeling. Water Resour. Res. 37, 2457–2470. doi: 10.1029/2000WR000027
Wagner, F. M., Mollaret, C., Günther, T., Kemna, A., and Hauck, C. (2019). Quantitative imaging of water, ice and air in permafrost systems through petrophysical joint inversion of seismic refraction and electrical resistivity data. Geophys. J. Int. 219, 1866–1875. doi: 10.1093/gji/ggz402
Wagner, F. M., and Uhlemann, S. (2021). An overview of multimethod imaging approaches in environmental geophysics. Adv. Geophys. 62, 1–72. doi: 10.1016/bs.agph.2021.06.001
Weigand, M.. (2017). Monitoring Structural and Physiological Properties of Crop Roots Using Spectral Electrical Impedance Tomography. Bonn: Zenodo.
Werban, U., Attia al Hagrey, S., and Rabbel, W. (2008). Monitoring of root-zone water content in the laboratory by 2D geoelectrical tomography. Z. Pflanzenernähr. Bodenk. 171, 927–935. doi: 10.1002/jpln.200700145
Yu, Y., Weihermüller, L., Klotzsche, A., Lärm, L., Vereecken, H., and Huisman, J. A. (2021). Sequential and coupled inversion of horizontal borehole ground penetrating radar data to estimate soil hydraulic properties at the field scale. J. Hydrol. 596:126010. doi: 10.1016/j.jhydrol.2021.126010
Zhang, H., Kurtz, W., Kollet, S., Vereecken, H., and Franssen, H.-J. H. (2018). Comparison of different assimilation methodologies of groundwater levels to improve predictions of root zone soil moisture with an integrated terrestrial system model. Adv. Water Resour. 111, 224–238. doi: 10.1016/j.advwatres.2017.11.003
Keywords: hydrogeophysics, inversion, root water uptake, soil-plant modeling, geoelectrical imaging, data assimilation, Wasserstein distance
Citation: Mary B, Peruzzo L, Iván V, Facca E, Manoli G, Putti M, Camporese M, Wu Y and Cassiani G (2021) Combining Models of Root-Zone Hydrology and Geoelectrical Measurements: Recent Advances and Future Prospects. Front. Water 3:767910. doi: 10.3389/frwa.2021.767910
Received: 31 August 2021; Accepted: 08 November 2021;
Published: 14 December 2021.
Edited by:
Sylvain Pasquet, UMR7154 Institut de Physique du Globe de Paris (IPGP), FranceReviewed by:
Alex Furman, Technion Israel Institute of Technology, IsraelCopyright © 2021 Mary, Peruzzo, Iván, Facca, Manoli, Putti, Camporese, Wu and Cassiani. This is an open-access article distributed under the terms of the Creative Commons Attribution License (CC BY). The use, distribution or reproduction in other forums is permitted, provided the original author(s) and the copyright owner(s) are credited and that the original publication in this journal is cited, in accordance with accepted academic practice. No use, distribution or reproduction is permitted which does not comply with these terms.
*Correspondence: Benjamin Mary, YmVuamFtaW4ubWFyeUB1bmlwZC5pdA==
Disclaimer: All claims expressed in this article are solely those of the authors and do not necessarily represent those of their affiliated organizations, or those of the publisher, the editors and the reviewers. Any product that may be evaluated in this article or claim that may be made by its manufacturer is not guaranteed or endorsed by the publisher.
Research integrity at Frontiers
Learn more about the work of our research integrity team to safeguard the quality of each article we publish.