- 1Department of Water Management, Delft University of Technology, Delft, Netherlands
- 2Department of Water Resources and Ecosystems, IHE Delft Institute for Water Education, Delft, Netherlands
Ethiopia depends on rain-fed agriculture with limited use of irrigation for agricultural production. More than 90% of the food supply in the country comes from low productivity rain-fed smallholder agriculture. Since the livelihoods of many farmers depend on rainfed agriculture, this paper investigates how smallholders adapt to climate variability. Dhidhessa sub-basin of the Blue Nile river basin is home to many vulnerable immigrant smallholders from other parts of Ethiopia. Our study focuses on this sub-basin to understand how crop production and patterns have depended on rainfall. Secondary data on land cover and croplands, the number of households growing crops, crop yields, crop prices and area covered by three major crops (teff, maize, and sorghum) are analyzed over a period 2000–2019 and interpreted in light of a primary household survey of 135 farmers in the basin. Results show that almost 40% of the basin is under crop cultivation, and the area under cultivation has been growing 8.6‰ per year. Irrespective of rainfall variability, the number of households practicing crop cultivation has also been growing over the years. This means that more farmers are moving into the basin to cultivate. Analysis reveals that adaptation strategies are at play. Farmer decisions to grow which crops are sensitive to rainfall and their expectations of crop prices resulting from rainfall variability. Their decisions and crop prices are endogenous to the smallholder sociohydrology of the basin, leading more farmers to grow Teff relative to other crops in years of lower rainfall. These decisions are due to the lower sensitivity of Teff prices to rainfall variability and farmers' expectations of higher Teff prices relative to other crops as rainfall decreases. Such behavior also induces climate resilience, enabling farmers to respond to climate variability rather than migrating out of the basin. Moreover, it allows more farmers to migrate in and engage in crop cultivation within the basin. Such an adaptive strategy based on past experiences offers a way forward to incorporating adaptation mechanisms in sociohydrological models to simulate and assess water futures for similar basins worldwide.
Introduction
Agricultural development is fueled by economic growth, often ignoring the environmental degradation that it brings (den Besten et al., 2016; Pande and Savenije, 2016; Roobavannan et al., 2018). Due to the coevolution of human well-being with its agricultural water systems (Montanari, 2015; Roobavannan et al., 2017a), understanding the underlying interlinkages between the two is of utmost importance for sustainable agricultural development (Sivapalan et al., 2012; Montanari, 2015; Roobavannan et al., 2017b). One key challenge is the complexity induced by competing water demands by various users such as upstream vs. downstream water users, large vs. small holding farmers and the farmers vs. the environment (Gober and Wheater, 2014). This competition adversely affects those whose demands either have hidden or low economic value and have little or no influence on water resource policy, such as downstream users, smallholder farmers and the environment.
On the other hand, rainfall variability and other factors such as volatility in market prices drive farmers to choose the types of crops they grow (Ruben et al., 2000; Niles and Brown, 2017). Rain-fed agriculture is common in sub-Saharan African countries, mostly practiced by smallholder farmers (Bank, 1997; Strzepek and McCluskey, 2007), where crop yields depend on the strength of the rainfall seasons (Rockström et al., 2003; Jemberie et al., 2016; Villani et al., 2018). The income of smallholders is therefore linked to low productivity of crops grown and market volatility, and such dependence in turn results in low incomes for such farmers.
Ethiopia depends on rain-fed agriculture with limited use of irrigation for agricultural production (Awulachew et al., 2010). It is estimated that more than 90% of the food supply in the country comes from low productivity rain-fed smallholder agriculture, and hence rainfall is the single most important determinant of food supply and the country's economy (Mati, 2006; Manaswi and Thawait, 2014; Zewdie et al., 2020). Gebre et al. (2015) investigated the effects of climate on water availability in the Dhidhessa River basin and noted the effects of spatial and temporal variations in rainfall on agricultural production. Adgolign et al. (2015) reported that for effective planning and management of water resources, understanding the spatial and temporal fluctuations of water flows is critical. The authors recommended that a simulation approach might be the best approach to quantify water availability. However, there is limited literature on the dependence of farmers on rain-fed agriculture, effects of population, local re-adaptation to climate change variability and management in the Dhidhessa River Basin.
If such farmers mostly depend on rainfall, the key is to understand how they adapt to its variability. Here migration is often suggested as one adaptive response to climate variability (Andersson, 2014; Teweldebrihan et al., 2020). Yet smallholders are known to be efficient and resilient producers, with novel traditions to sustain their livelihoods under adversity (Debela et al., 2015; Belay et al., 2017; Dechassa et al., 2020).
This paper assesses how smallholder farmers adapt to anthropogenic and climate induced variability in the Dhidhessa river basin. In order to do so, the paper (i) interprets the evolution of crop coverage over time in the basin and (ii) understands how crop production and patterns depend on rainfall in the basin.
Study Area
The Dhidhessa River is a tributary of the Abbay River emanating from an elevation above 2,500 m near the Wacha and Vennio mountainous terrains in Ethiopia with a basin area of 16,567 km2 (Figure 1).
The climate of the Dhidhessa basin is traditionally characterized mostly as a Woina Dega (Sub-tropical) and Kolla (tropical) with heavy rainfall during Kiremt (winter) season (Bekele et al., 2021). The basin experiences variable rainfall, which ranges from a minimum of 121 mm to a maximum of 2,199 mm annually. Average rainfall amounts for the duration of dry, short and long rainy seasons are 151, 218, 916 mm, respectively (Tesemma et al., 2010). Overall annual rainfall decreases from the South-West (over 2,000 mm/year) to the North-East (about 1,000 mm/year), and about 70% of the rain falls between June and September (Conway, 2000; Dechassa et al., 2020).
The elevations in the basin are classified as highland (>2,500 m a.s.l), midland (1,500–2,500 m a.s.l) and lowland (<1,500 m a.s.l.) (Chimdessa et al., 2019). The temperature in the basin fluctuates depending on the topography of the sub-catchment area. The minimum average temperature in the sub-basin ranges between 7 and 17°C, and the maximum average temperature ranges between 21 and 37°C (Chimdessa et al., 2019; Tolessa et al., 2020). Studies made in recent years indicate that climate change, land and water degradation are mainly related to rainfall fluctuations and the traditional beliefs dominating in the community toward water (Adgolign et al., 2016; Chimdessa et al., 2019).
Methodology
Cropland Time Series
Remote sensing techniques are used to measure the crop coverage over time in the basin. Landsat images with minimum cloud cover and within the growing season are used to compute cropland proportions within the basin (Huang et al., 2017; Xiong et al., 2017). Google Earth Engine (GEE) is used to process the data (Gorelick et al., 2017; Shelestov et al., 2017).
A Classification and Regression Tree (CART) classifier is used to identify croplands over time. The CART classifier has been trained with multiple-time step images with 2015 selected for classifier training (Yang et al., 2016; Chen et al., 2017). The GFSAD 30 (Global Food Security-Support Analysis Data with 30 m resolution) crop map for 2015 (Xiong et al., 2017; Teluguntla et al., 2018) is used as a training reference. The GFSAD 30 crop map is a 0-1-2 map of crop existence, i.e., 0 - water, 1 - no crop, 2 - cropland, which was remapped into a 0-1 map, i.e., to 0 - no crop, 1 - cropland. The CART classifier's sample size for the study is 150,000, and the same number of sample points from 0-pixel and 1-pixel, i.e., 75,000 for each category, are used to train the classifier. As predictors of the classifier, for the same year of 2015, the “B2,” “B3,” “B4,” “B5,” “NDVI” bands were packed from Landsat 7, 32-day images (30 m resolution) within the main growing season (January - March; with three images one for each month), together with the ’elevation’ band from SRTM 30 (30 m resolution) DEM image (Teluguntla et al., 2018). Thus, CART classifier training includes 5*3 + 1 = 16 bands as the predictors (Figure 2). The predictors describe cropland's remote-sensing characteristics within the growing season, based on which the classifier is used to distinguish croplands from non-cropland pixels for years other than 2015 (Huang et al., 2017; Teluguntla et al., 2018).
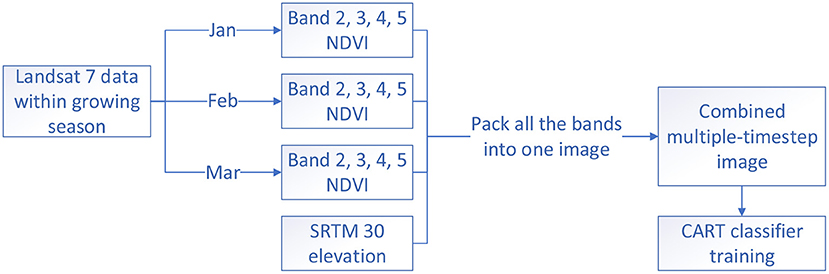
Figure 2. Multiple-time step images used as predictors to train the CART classifier for cropland identification.
The trained CART classifier is used to identify the distribution of croplands within the Dhidhessa river basin for 20 years from 2000 to 2019. For each year, the 16 bands mentioned above are used as predictors to identify crops.
Smallholder Agriculture System Dependence on Rainfall
Secondary Data and Analysis
The secondary data on agriculture, obtained from the Ethiopian Central Statistical Agency (CSA, 2012), is used to understand how agriculture in the basin depends on rainfall. The variables include the number of households (HH) growing crops in the basin, crop yields, crop prices and area covered by dominant crops in the basin. The three major crops grown (teff, maize, and sorghum) are selected for this study, accounting for nearly 80% of the crops grown in the area (CSA, 2017; Kabeta et al., 2019).
The daily rainfall data are obtained from the Ethiopian National Meteorological Agency (NMA, 2007), and the gauge locations are shown in Figure 1B. Its average over the basin is used to assess how crop yields, production, area, and prices, which are the key variables of the basin's agricultural system, vary with rainfall.
The data are employed to understand how farmers' decisions to grow crops of certain types are influenced by water availability and the expectation of prices at the end of the growing season.
Primary Data
Primary data was collected by the authors, while the secondary data was not but obtained from sources such as GEE and Ethiopian Agricultural Services. Additionally, primary data (farmer interviews) are used to validate key observations of the analysis based on secondary data. About 45 farmers were interviewed from each of three zones (namely Wollega, Benishangul, and Illibabor). Therefore, in total, 135 farmers were interviewed, selected randomly to represent the three zones of the Dhidhessa basin using the snowball sampling method (Goodman, 1961; Johnson, 2014).
The interview questions were focused on inquiring about the following: (i) why the number of HHs growing various crops varies with the rainfall, (ii) why the prices of crops change with annual rainfall, and; (iii) why the type of crops chosen by farmers varies with the rainfall and crop prices. The questionnaire used in this study is shown in Appendix A.
Results
Classified Cropland Time-Series
Figure 3A shows croplands identified by the CART classifier for 2015, which visually compares well with GFSAD 30 cropland map for the same year (shown in Figure 3B).
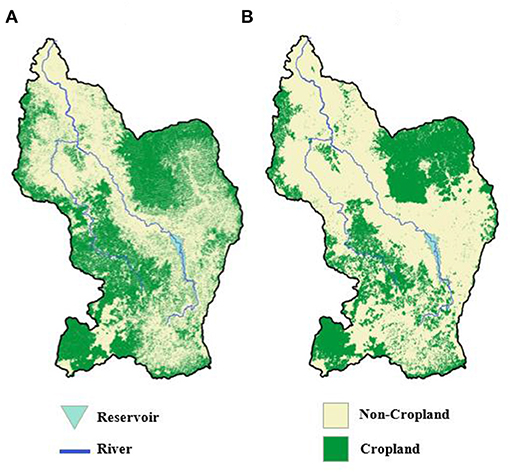
Figure 3. (A) Cropland map sample produced for the Dhidhessa River whole basin in 2015. (B) Cropland reference map of GFSAD 30 for Dhidhessa River whole basin for 2015. Green color means cropland while yellow color means non-cropland.
The training accuracy, defined as the proportion of correctly classified pixels within all pixels, is 0.82. The number on the diagonal lines of the confusion matrix show the number of points which has been correctly classified, and the confusion matrix here indicates that CART classifier has a very good performance.
Based on the croplands classified over time, Figure 4 plots the cropland proportions. Almost 40% of the basin is under crop cultivation currently. The results provide evidence of significant trends in the time series. The cropland proportions show an increasing trend for the whole basin area (8.6‰ per year, p-value < 0.001).
Dependence of Dhidhessa Agriculture on Rainfall
The number of households growing the three dominant crops, calculated based on secondary data, show an increasing trend over time (Figure 5). The total number of household grow crops in the basin is about 1214497 in 2001 and 3452125 in 2017 which shows an increasing trend over the years (Figure 5).
Figure 6 shows that the area fraction under the dominant crops is insensitive to rainfall variation over time. Hence, the area fraction is the agricultural area covered by respective crops as a fraction of the total cultivated land. The area fraction was calculated as the ratio of area cultivated for a crop and the total cultivated area. This corroborates with the evidence shown in Figure 5 that cropland areas follow an increasing trend over time due to more households moving into the basin.
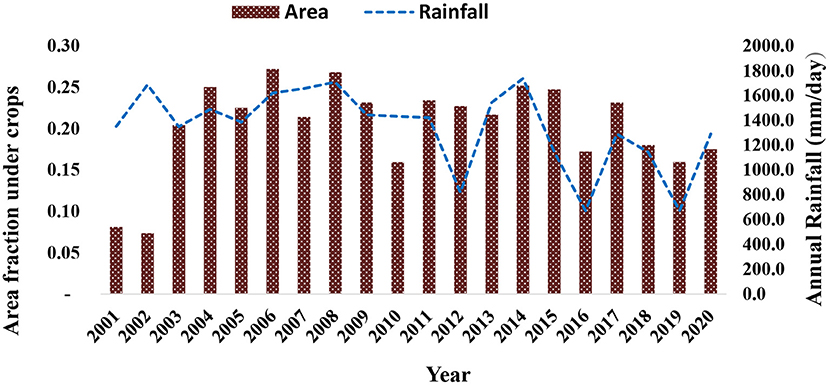
Figure 6. Area fraction of crops grown, as a fraction of total agricultural land in the basin. Also shown annual rainfall over the years.
Figure 7 shows that yields in the basin are highly sensitive to rainfall and increase when the basin receives more rainfall.
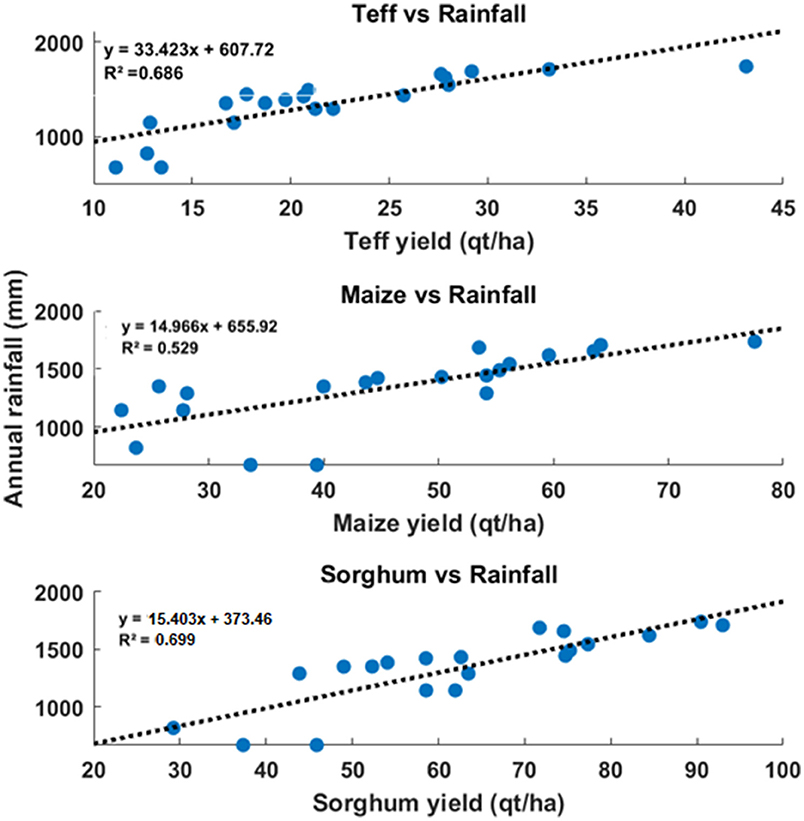
Figure 7. Scatter plots of the yields of dominants crops vs. annual rainfall amounts. Yields rise rainfall, suggesting rainfed agriculture.
Interviews with the farmers reveal that relatively larger farmers who also have livestock decide to leave their lands fallow, mainly used for grazing purposes, in years with good rainfall. They do this in anticipation of a fall in prices due to higher yields and production in good rainfall years. Figure 8 shows that prices of all the crops fall in good rainfall years. This means that crop yields are water-limited (and not irrigated by river water) to the extent that better rainfall improves yields by an amount that enables even fewer farmers to deliver higher total production. Additionally, farmer interviews also reveal that farmers substitute one crop for another when the rainfall increases. This supports the observation in Figure 9 that fewer farmers grow Teff relative to the other two crops as rainfall increases.
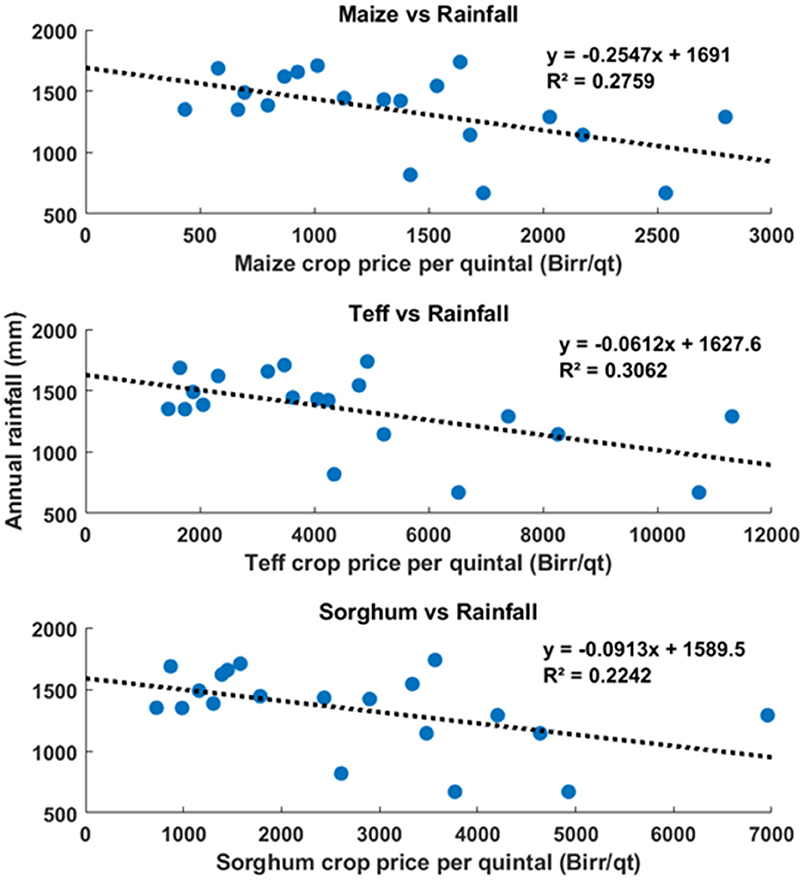
Figure 8. Scatter plots of the prices of dominants crops vs. annual rainfall amounts. In general crop prices fall in years with higher rainfall.
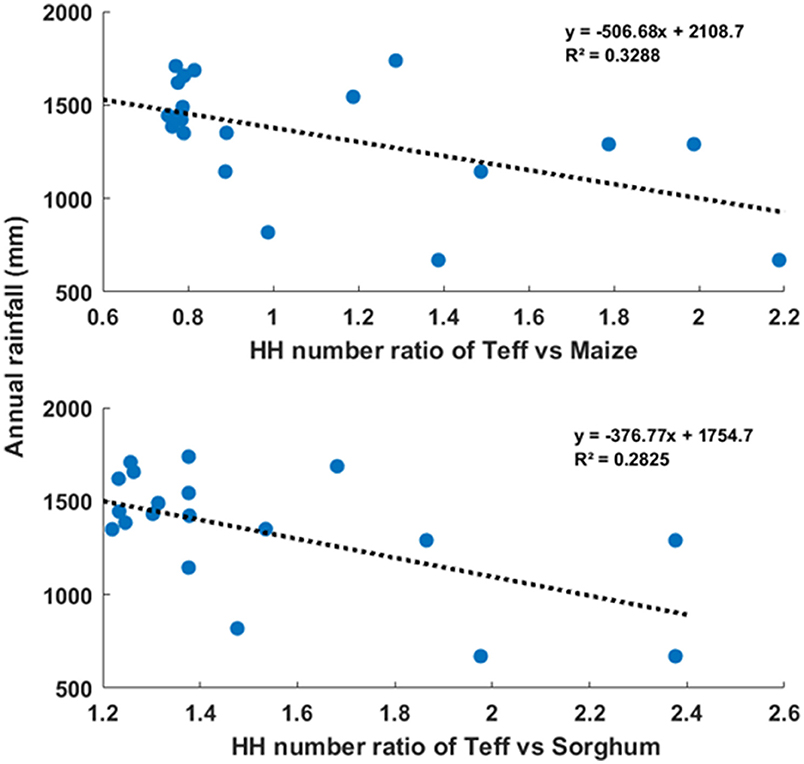
Figure 9. Scatter plots of household (HH) ratios of teff and maize, and teff and sorghum growers, vs. rainfall. Fewer households grow Teff relative to the other two crops when rainfall increase.
Discussion
The results demonstrate that farmer decision to grow which crops are sensitive to rainfall and their expectations of crop prices resulting from rainfall variability. The farmers' decisions and crop prices are endogenous in how cropland areas do not respond to rainfall variations, but instead, farmers shift from one crop to another. This demonstrates the sociohydrological complexity of the smallholder farming system (Pande and Savenije, 2016; Niles and Brown, 2017; Jovanovic et al., 2020; Lyu et al., 2020a; Pande et al., 2020). The interviews corroborate that the farmers decide on the types of crops to grow every season based on their prediction of rainfall using traditional rainfall prediction practices (Balehegn et al., 2019; Wedajo et al., 2019).
The interviews suggest that more farmers grow Teff in years when the expected amount of rainfall is low using the indigenous (traditional) knowledge in which farmers predict the supply will decrease and the price will rise due the demand of Teff consumers in the country. The farmers in the area mostly decide the type of crop to grow using the traditional expectation and past experience, therefore, they used to say “expecting is better than what you have gotten.” This is also corroborated by secondary data, as shown in Figure 9 above. Hence, the ratio of teff grower vs. maize and sorghum in relation to the annual rain fall varies in which the ratio of HH number grow teff vs. maize shows < 1 and ratio of teff grower vs. sorghum is about >1, respectively. Consequently, the less number of households start to grow Teff in good rainfall years (it does not matter whether Teff growers are more or less than Maize growers). This leads to an increase in the per-unit cost of producing Teff relative to other crops due to the rise of cultivation demand. The cost for teff is higher that maize and sorghum because the row sowing of teff cultivation needs more time and intensive labor cost (row-making and weeding due to its grain size) than other crops. This can be observed in Figure 10 that plots the per unit cultivation cost of Teff vs. other crops. Consequently, the price of seeds, human labor, and related costs of growing Teff is greater than of other crops. Yet these costs are offset by the higher price of Teff under poor rainfall conditions relative to the other two dominant crops, still making Teff an attractive option to grow as suggested. Indeed as Figure 11 shows, Teff prices relative to other crops are higher for seasons with lower rainfall.
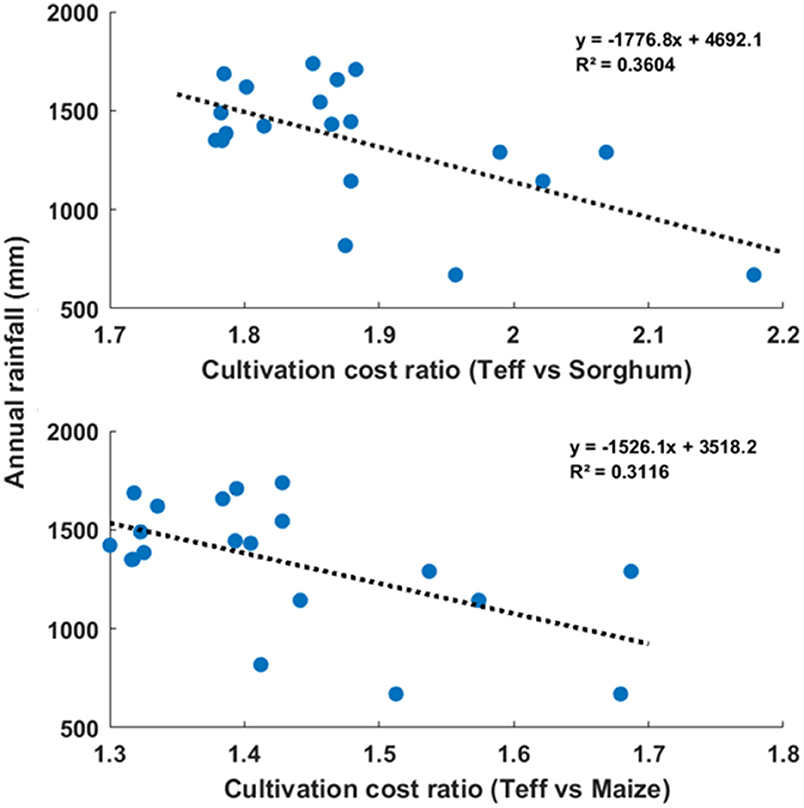
Figure 10. Scatter plots of cultivation cost ratios of teff and maize, and teff and sorghum, vs. rainfall. Cultivation cost of Teff relative to the other two crops falls when rainfall increases.
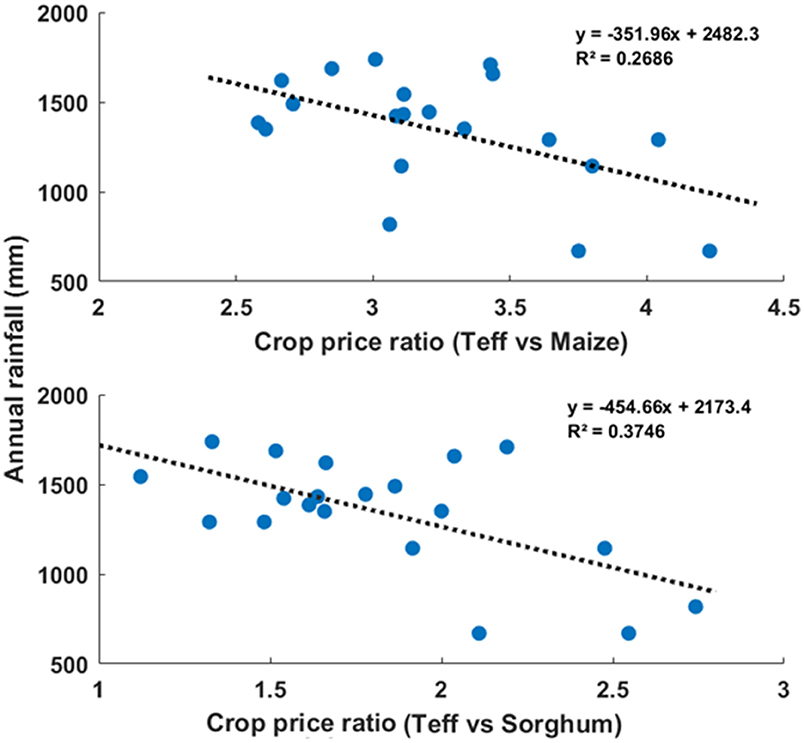
Figure 11. Scatter plots of price ratios of teff and maize, and teff and sorghum, vs. rainfall. Price of Teff relative to the other two crops falls when rainfall increases.
About 65% of interviewed farmers said they grow crops that fetch high prices when the rainfall is low. Only about 35% of interviewed farmers suggest that they grow crops when higher rains are expected. Others said they leave their lands for grazing during high rainfall seasons, driven by the expectation that livestock production income will be better than agricultural production (~51% respondents). This is also suggestive of the role that past experiences play (Baldassarre et al., 2013; Viglione et al., 2014; Fuchs et al., 2017; Leong, 2018) in farmers' decision-making to expect higher production levels and, therefore, lower prices for crops, during high rainfall seasons.
The interviews reveal that 81% of farmers make decisions, such as which crop to grow, based on indigenous knowledge. The remaining 19% use the advice from the agricultural extension experts. This may be the reason why the basin's farm system is sensitive to rainfall, but rainfall effects are endogenous. Farmers adapt their cropping patterns based on rainfall and price expectations, indicating that such adaptive measures are in place to make them more resilient to climate variability.
The adaptation strategy also enables them not to respond to climate variability by migrating out of the basin. Despite rainfall variability, households engaged in crop production have steadily increased in the basin. The total population number was 6,072,485 in 2001 and 12,884,927 in 2020 and the total number of growers increased from 1,214,497 in 2001 to 3,681,408 in 2010. For example the number of Teff growers in the basin was 375,003 in 2001 and increased to 1,028,804 in 2019 which is more than double in the time period. Similar increases are observed for other crops. This evidence of the critical role played by adaptation to climate variability is counterfactual to a pattern often alluded to that migration is the only response to climate variability. Lyu et al. (2020b) similarly found no links between rural to urban migration and climate variability in Jiangsu province China. Other studies (Akay et al., 2012; Hagedorn et al., 2013; Delango, 2019; Lyu et al., 2019) have made similar observations, though studies such as Heitmueller (2005) and Roobavannan et al. (2017a) have highlighted it as a response to change in water policy and consequent unemployment.
Such a response to climate variability indicates the extent to which crop production in the basin depends on rain and not so much on the river flows. Traditional practices have perhaps emerged due to long-term dependence on rainfall and its endogenous effects (Simelton et al., 2009; Panda et al., 2013; Mekuria, 2018). The number of households growing crops has been consistently increasing over time without any sensitivity to rain.
Conclusion
This paper investigated how smallholders adapt to the change in climate and climate variability in the Dhidhessa river basin. Analysis of secondary and primary data on land cover, households engaged in crop production, crop yields, crop prices and area covered by three major crops (Teff, maize and sorghum) over a period of 20 years revealed that irrespective of rainfall variability, more farmers are moving in the basin because the area under crop cultivation, as well as the number of households practicing it, has grown over the years. Farmers' decisions to grow which crops were found to be sensitive to rainfall and their expectations of crop prices resulting from rainfall variability and climate change. Results from the trend indicate that the farmers are resilient to the change in climate and farmer decisions in choosing the type of crop respond to the rainfall variability and climate change over the years. Yields were found to be sensitive to rainfall to such an extent that even when fewer farmers grew crops in good rainfall years, the production was higher in spite of a smaller area under cultivation. Further, farmers grow more of Teff relative to other crops in lower rainfall years due to the lower sensitivity of Teff prices to rainfall variability and farmers' expectations of higher Teff prices relative to other crops in lower rainfall seasons. The behavior thus unraveled offers a way forward to incorporating adaptation mechanisms in sociohydrological models to simulate water futures in similar basins worldwide realistically.
Data Availability Statement
The original contributions presented in the study are included in the article/Supplementary Material, further inquiries can be directed to the corresponding author.
Author Contributions
The initial concept writing, analysis, review, and final write-up was done by MT and SP in which HL contributed on the analysis. MM contributed on the conceptualization and review of the paper. All authors contributed to the article and approved the submitted version.
Funding
The research grant from the International Foundation for Science (IFS) is acknowledged for the financial support (Grant Number I-2-W-6357-1). The support of IHE Delft and Delft University of Technology is acknowledged. The Schlumberger Foundation, Faculty of the Future, is warmly recognized for providing financial support.
Conflict of Interest
The authors declare that the research was conducted in the absence of any commercial or financial relationships that could be construed as a potential conflict of interest.
Publisher's Note
All claims expressed in this article are solely those of the authors and do not necessarily represent those of their affiliated organizations, or those of the publisher, the editors and the reviewers. Any product that may be evaluated in this article, or claim that may be made by its manufacturer, is not guaranteed or endorsed by the publisher.
Acknowledgments
Special thanks go to Mr. Bahru Fino; the Ministry of Agricultural extension are recognized for their help in providing secondary data.
Supplementary Material
The Supplementary Material for this article can be found online at: https://www.frontiersin.org/articles/10.3389/frwa.2021.735004/full#supplementary-material
References
Adgolign, T. B., Rao, G. S., and Abbulu, Y. (2016). WEAP modeling of surface water resources allocation in Didessa Sub-Basin, West Ethiopia. Sustain. Water Resour. Manage. 2, 55–70. doi: 10.1007/s40899-015-0041-4
Adgolign, T. B., Rao, G. V. R. S., and Abbulu, Y. (2015). Assessment of spatio-temporal occurrence of water resources in didissa sub-basin, West Ethiopia. Int. J. Civil Struct. Environ. Infrast. Eng. Res. Dev. 5, 105–120.
Akay, A., Bargain, O., and Zimmermann, K. F. (2012). Relative concerns of rural-to-urban migrants in China. J. Econ. Behav. Org. 81, 421–441. doi: 10.1016/j.jebo.2011.12.006
Andersson, L. (2014). Migration, Remittances and Household Welfare in Ethiopia. United Nations University-Maastricht Economic and Social Research Institute on Innovation and Technology (MERIT).
Awulachew, S. B., Erkossa, T., and Namara, R. (2010). Irrigation Potential in Ethiopia: Constraints and Opportunities for Enhancing the System. Ethiopia: International Water Management Institute.
Baldassarre, G. D., Viglione, A., Carr, G., Kuil, L., Salinas, J., and Blöschl, G. (2013). Socio-hydrology: conceptualising human-flood interactions. Hydrol. Earth Syst. Sci. 17, 3295–3303. doi: 10.5194/hess-17-3295-2013
Balehegn, M., Balehey, S., Fu, C., and Liang, W. (2019). Indigenous weather and climate forecasting knowledge among Afar pastoralists of north eastern Ethiopia: Role in adaptation to weather and climate variability. Pastoralism 9, 1–14. doi: 10.1186/s13570-019-0143-y
Bank, W. (1997). World Development Report 1997: The State in a Changing World. New York, NY: The World Bank.
Bekele, W. T., Haile, A. T., and Rientjes, T. (2021). Impact of climate change on the streamflow of the Arjo-Didessa catchment under RCP scenarios. J. Water Clim. Change. 12, 1–13. doi: 10.2166/wcc.2021.307
Belay, A., Recha, J. W., Woldeamanuel, T., and Morton, J. F. (2017). Smallholder farmers' adaptation to climate change and determinants of their adaptation decisions in the Central Rift Valley of Ethiopia. Agric. Food Secur. 6, 1–13. doi: 10.1186/s40066-017-0100-1
Chen, W., Xie, X., Wang, J., Pradhan, B., Hong, H., Bui, D. T., et al. (2017). A comparative study of logistic model tree, random forest, and classification and regression tree models for spatial prediction of landslide susceptibility. Catena 151, 147–160. doi: 10.1016/j.catena.2016.11.032
Chimdessa, K., Quraishi, S., Kebede, A., and Alamirew, T. (2019). Effect of land use land cover and climate change on river flow and soil loss in Didessa River Basin, South West Blue Nile, Ethiopia. Hydrology 6:2. doi: 10.3390/hydrology6010002
Conway, D. (2000). The climate and hydrology of the Upper Blue Nile River. Geogr. J. 166, 49–62. doi: 10.1111/j.1475-4959.2000.tb00006.x
CSA (2012). Ethiopia Demographic and Health Survey 2011. Addis Ababa, Ethiopia: Ethiopian Central Statistical Agency.
CSA (2017). The Federal Democratic Republic of Ethiopia. Addis Ababa: Central Statistical Agency. CSA.
Debela, N., Mohammed, C., Bridle, K., Corkrey, R., and McNeil, D. (2015). Perception of climate change and its impact by smallholders in pastoral/agropastoral systems of Borana, South Ethiopia. Springerplus 4:236. doi: 10.1186/s40064-015-1012-9
Dechassa, C., Simane, B., and Alamerew, B. (2020). Analysis of farmers perceived and observed climate variability and change in Didessa sub-basin, Blue Nile River, Ethiopia. Afr. J. Agric. Res. 15, 149–164. doi: 10.5897/AJAR2019.14054
Delango, M. W. (2019). The causes and consequences of rural-urban migration: the case of Wolaita Sodo Town Merhal Sub-City. Budapest Int. Res. Crit. Inst. Hum. Soc. Sci. 2, 99–114. doi: 10.33258/birci.v2i4.609
den Besten, N. I., Pande, S., and Savenije, H. H. (2016). A socio-hydrological comparative assessment explaining regional variances in suicide rate amongst farmers in Maharashtra, India. Proc. Int. Assoc. Hydrol. Sci. 373, 115–118. doi: 10.5194/piahs-373-115-2016
Fuchs, S., Karagiorgos, K., Kitikidou, K., Maris, F., Paparrizos, S., and Thaler, T. (2017). Flood risk perception and adaptation capacity: a contribution to the socio-hydrology debate. Hydrol. Earth Syst. Sci. 21, 3183–3198. doi: 10.5194/hess-21-3183-2017
Gebre, S. L., Tadele, K., and Mariam, B. G. (2015). Potential impacts of climate change on the hydrology and water resources availability of Didessa Catchment, Blue Nile River Basin, Ethiopia. J. Geol. Geosci. 4, 1–7. doi: 10.4172/2329-6755.1000193
Gober, P., and Wheater, H. S. (2014). Socio-hydrology and the science–policy interface: a case study of the Saskatchewan River basin. Hydrol. Earth Syst. Sci. 18, 1413–1422. doi: 10.5194/hess-18-1413-2014
Gorelick, N., Hancher, M., Dixon, M., Ilyushchenko, S., Thau, D., and Moore, R. (2017). Google earth engine: planetary-scale geospatial analysis for everyone. Remote Sens. Environ. 202, 18–27. doi: 10.1016/j.rse.2017.06.031
Hagedorn, M., Karahan, F., Manovskii, I., and Mitman, K. (2013). Unemployment Benefits and Unemployment in the Great Recession: The Role of Macro Effects. Cambridge, MA: National Bureau of Economic Research.
Heitmueller, A. (2005). Unemployment benefits, risk aversion, and migration incentives. J. Popul. Econ. 18, 93–112. doi: 10.1007/s00148-004-0192-3
Huang, H., Chen, Y., Clinton, N., Wang, J., Wang, X., Liu, C., et al. (2017). Mapping major land cover dynamics in Beijing using all Landsat images in Google Earth Engine. Remote Sens. Environ. 202, 166–176. doi: 10.1016/j.rse.2017.02.021
Jemberie, M. A., Awass, A. A., Melesse, A. M., Ayele, G. T., and Demissie, S. S. (2016). “Seasonal rainfall–runoff variability analysis, Lake Tana Sub-Basin, Upper Blue Nile Basin, Ethiopia,” in Landscape Dynamics, Soils and Hydrological Processes in Varied Climates, eds A. Melesse, W. Abtew (Cham: Springer), 341–363.
Johnson, T. P. (2014). Snowball Sampling: Introduction. Wiley StatsRef: Statistics Reference Online.
Jovanovic, N., Musvoto, C., De Clercq, W., Pienaar, C., Petja, B., Zairi, A., et al. (2020). A comparative analysis of yield gaps and water productivity on smallholder farms in Ethiopia, South Africa and Tunisia. Irrig. Drain. 69, 70–87. doi: 10.1002/ird.2238
Kabeta, T., Haji, J., and Negash, R. (2019). Determinants of Smallholder Farmers Teff Market Supply, in Jimma Arjo District, Western Oromia Regional State, Ethiopia: A Two Stage Least Square Approach.
Leong, C. (2018). The role of narratives in sociohydrological models of flood behaviors. Water Resour. Res. 54, 3100–3121. doi: 10.1002/2017WR022036
Lyu, H., Dong, Z., and Pande, S. (2020a). Interlinkages between human agency, water use efficiency and sustainable food production. J. Hydrol. 582:124524. doi: 10.1016/j.jhydrol.2019.124524
Lyu, H., Dong, Z., Roobavannan, M., Kandasamy, J., and Pande, S. (2019). Rural unemployment pushes migrants to urban areas in Jiangsu Province, China. Palgrave Commun. 5, 1–12. doi: 10.1057/s41599-019-0302-1
Lyu, H., Dong, Z., Roobavannan, M., Kandasamy, J., and Pande, S. (2020b). Prospects of interventions to alleviate rural–urban migration in Jiangsu Province, China based on sensitivity and scenario analysis. Hydrol. Sci. J. 65, 2175–2184. doi: 10.1080/02626667.2020.1802030
Manaswi, C., and Thawait, A. (2014). Application of soil and water assessment tool for runoff modeling of Karam River basin in Madhya Pradesh. Int. J. Sci. Eng. Technol. 3, 529–532.
Mati, B. M. (2006). Overview of Water and Soil Nutrient Management Under Smallholder Rain-Fed Agriculture in East Africa. Vol. 105. IWMI.
Mekuria, W. (2018). The link between agricultural production and population dynamics in Ethiopia: a review. Adv. Plants Agric. Res. 8, 348–353. doi: 10.15406/apar.2018.08.00336
Montanari, A. (2015). Debates—Perspectives on socio-hydrology: introduction. Water Resour. Res. 51, 4768–4769. doi: 10.1002/2015WR017430
Niles, M. T., and Brown, M. E. (2017). A multi-country assessment of factors related to smallholder food security in varying rainfall conditions. Sci. Rep. 7, 1–11. doi: 10.1038/s41598-017-16282-9
Panda, A., Sharma, U., Ninan, K., and Patt, A. (2013). Adaptive capacity contributing to improved agricultural productivity at the household level: empirical findings highlighting the importance of crop insurance. Glob. Environ. Change 23, 782–790. doi: 10.1016/j.gloenvcha.2013.03.002
Pande, S., and Savenije, H. H. (2016). A sociohydrological model for smallholder farmers in Maharashtra, India. Water Resour. Res. 52, 1923–1947. doi: 10.1002/2015WR017841
Pande, S., Roobavannan, M., Kandasamy, J., Sivapalan, M., Hombing, D., Lyu, H., et al. (2020). A socio-hydrological perspective on the economics of water resources development and management. Oxford Res. Encycl. Environ. Sci. 1–30. doi: 10.1093/acrefore/9780199389414.013.657
Rockström, J., Barron, J., and Fox, P. (2003). Water productivity in rain-fed agriculture: challenges and opportunities for smallholder farmers in drought-prone tropical agroecosystems. Water Prod. Agric. Limits Oppor. Improv. 85199:8. doi: 10.1079/9780851996691.0145
Roobavannan, M., Kandasamy, J., Pande, S., Vigneswaran, S., and Sivapalan, M. (2017a). Allocating environmental water and impact on basin unemployment: role of a diversified economy. Ecol. Econ. 136, 178–188. doi: 10.1016/j.ecolecon.2017.02.006
Roobavannan, M., Kandasamy, J., Pande, S., Vigneswaran, S., and Sivapalan, M. (2017b). Role of sectoral transformation in the evolution of water management norms in agricultural catchments: a sociohydrologic modeling analysis. Water Res. Res. 53, 8344–8365. doi: 10.1002/2017WR020671
Roobavannan, M., van Emmerik, T. H. M., Elshafei, Y., Kandasamy, J., Sanderson, M. R., Vigneswaran, S., et al. (2018). Norms and values in sociohydrological models. Hydrol. Earth Syst. Sci. 22, 1337–1349. doi: 10.5194/hess-22-1337-2018
Ruben, R., Kruseman, G., Kuyvenhoven, A., and Brons, J. (2000). “Climate variability, risk-coping and agrarian policies: farm households' supply response under variable rainfall conditions,” in Report for NOP Project ‘Impact of Climate Change on Drylands (ICCD)’, Wageningen, The Netherlands.
Shelestov, A., Lavreniuk, M., Kussul, N., Novikov, A., and Skakun, S. (2017). Exploring Google Earth Engine platform for big data processing: classification of multi-temporal satellite imagery for crop mapping. Front. Earth Sci. 5:17. doi: 10.3389/feart.2017.00017
Simelton, E., Fraser, E. D., Termansen, M., Forster, P. M., and Dougill, A. J. (2009). Typologies of crop-drought vulnerability: an empirical analysis of the socio-economic factors that influence the sensitivity and resilience to drought of three major food crops in China (1961–2001). Environ. Sci. Policy 12, 438–452. doi: 10.1016/j.envsci.2008.11.005
Sivapalan, M., Savenije, H. H., and Blöschl, G. (2012). Socio-hydrology: a new science of people and water. Hydrol. Proces. 26, 1270–1276. doi: 10.1002/hyp.8426
Strzepek, K. M., and McCluskey, A. (2007). The Impacts of Climate Change on Regional Water Resources and Agriculture in Africa. Vol. 4290. World Bank Publications.
Teluguntla, P., Thenkabail, P. S., Oliphant, A., Xiong, J., Gumma, M. K., Congalton, R. G., et al. (2018). A 30-m landsat-derived cropland extent product of Australia and China using random forest machine learning algorithm on Google Earth Engine cloud computing platform. ISPRS J. Photogram. Remote Sens. 144, 325–340. doi: 10.1016/j.isprsjprs.2018.07.017
Tesemma, Z. K., Mohamed, Y. A., and Steenhuis, T. S. (2010). Trends in rainfall and runoff in the Blue Nile Basin: 1964–2003. Hydrol. Proces. 24, 3747–3758. doi: 10.1002/hyp.7893
Teweldebrihan, M. D., Pande, S., and McClain, M. (2020). The dynamics of farmer migration and resettlement in the Dhidhessa River Basin, Ethiopia. Hydrol. Sci. J. 65, 1–9. doi: 10.1080/02626667.2020.1789145
Tolessa, T., Dechassa, C., Simane, B., Alamerew, B., and Kidane, M. (2020). Land use/land cover dynamics in response to various driving forces in Didessa sub-basin, Ethiopia. GeoJournal 85, 747–760. doi: 10.1007/s10708-019-09990-4
Viglione, A., Di Baldassarre, G., Brandimarte, L., Kuil, L., Carr, G., Salinas, J. L., et al. (2014). Insights from socio-hydrology modelling on dealing with flood risk–roles of collective memory, risk-taking attitude and trust. J. Hydrol. 518, 71–82. doi: 10.1016/j.jhydrol.2014.01.018
Villani, L., Castelli, G., Hagos, E. Y., and Bresci, E. (2018). Water productivity analysis of sand dams irrigation farming in northern Ethiopia. J. Agric. Environ. Int. Dev. 112, 139–160.
Wedajo, G. K., Muleta, M. K., Gessesse, B., and Koriche, S. A. (2019). Spatiotemporal climate and vegetation greenness changes and their nexus for Dhidhessa River Basin, Ethiopia. Environ. Syst. Res. 8, 1–24. doi: 10.1186/s40068-019-0159-8
Xiong, J., Thenkabail, P. S., Gumma, M. K., Teluguntla, P., Poehnelt, J., Congalton, R. G., et al. (2017). Automated cropland mapping of continental Africa using Google Earth Engine cloud computing. ISPRS J. Photogram. Remote Sens. 126, 225–244. doi: 10.1016/j.isprsjprs.2017.01.019
Yang, T., Gao, X., Sorooshian, S., and Li, X. (2016). Simulating California reservoir operation using the classification and regression-tree algorithm combined with a shuffled cross-validation scheme. Water Resour. Res. 52, 1626–1651. doi: 10.1002/2015WR017394
Keywords: Dhidhessa river sub-basin, crop production, price volatility, adaptation strategy, climate resilience, smallholder sociohydrology, climate variability
Citation: Teweldebrihan MD, Lyu H, Pande S and McClain ME (2021) Smallholder Farmer's Adaptability to Anthropogenic and Climate-Induced Variability in the Dhidhessa River Sub-basin, Ethiopia. Front. Water 3:735004. doi: 10.3389/frwa.2021.735004
Received: 01 July 2021; Accepted: 22 September 2021;
Published: 25 October 2021.
Edited by:
Julie A. Winkler, Michigan State University, United StatesReviewed by:
Thulani Dube, Lupane State University, ZimbabweSerena Ceola, University of Bologna, Italy
Copyright © 2021 Teweldebrihan, Lyu, Pande and McClain. This is an open-access article distributed under the terms of the Creative Commons Attribution License (CC BY). The use, distribution or reproduction in other forums is permitted, provided the original author(s) and the copyright owner(s) are credited and that the original publication in this journal is cited, in accordance with accepted academic practice. No use, distribution or reproduction is permitted which does not comply with these terms.
*Correspondence: Meseret Dawit Teweldebrihan, bS5kLnRld2VsZGVicmloYW4mI3gwMDA0MDt0dWRlbGZ0Lm5s