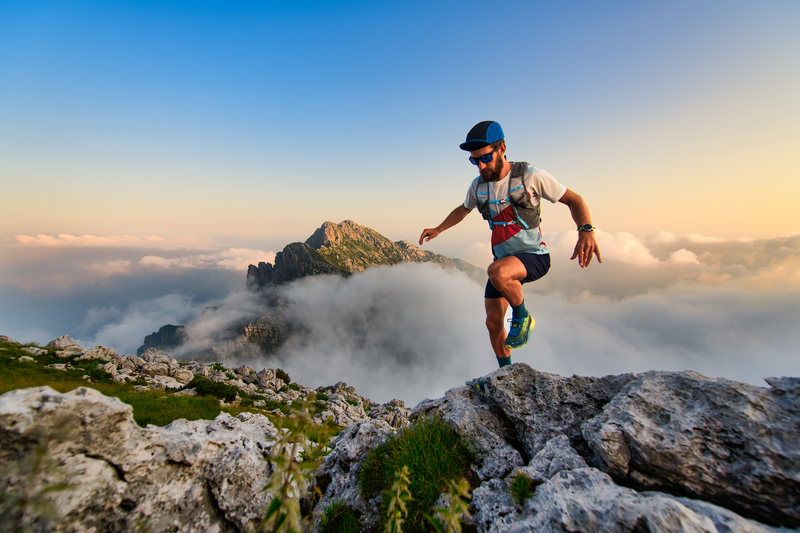
94% of researchers rate our articles as excellent or good
Learn more about the work of our research integrity team to safeguard the quality of each article we publish.
Find out more
ORIGINAL RESEARCH article
Front. Water , 28 June 2021
Sec. Water and Hydrocomplexity
Volume 3 - 2021 | https://doi.org/10.3389/frwa.2021.693172
Soil moisture plays an important role in the hydrologic water cycle. Relative to in-situ soil moisture measurements, remote sensing has been the only means of monitoring global scale soil moisture in near real-time over the past 40 years. Among these, soil moisture products from radiometry sensors operating at L-band, e.g., SMAP, SMOS, and SMOS-IC, are theoretically established to be more advantageous than previous C/X-band products. However, little effort has been made to investigate the inter-product differences of L-band soil moisture retrievals and provide insights into the optimal use of these products. In this regard, this study aims to identify the relative strengths and weaknesses of three L-band soil moisture products across diverse climate zones and land covers at the global scale using triple collocation analysis. Results show that SMOS-IC exhibits significantly improved soil moisture estimation skills, relative to the original SMOS product. This demonstrates the paramount importance of retrieval algorithm development in improving global soil moisture estimates—given both SMOS-IC and SMOS are using the same L-band brightness temperature information. Relative to SMOS-IC, SMAP is superior across 69% of global land surface in terms of error variances. However, SMOS-IC tends to outperform SMAP over temperate/arid regions including in the east of North America, South America, western Africa, northern China, and central Australia. Additionally, considerable performance degradation of all the L-band data products is observed over unvegetated areas. This may suggest that improving soil moisture retrieval accuracy over arid and semi-arid regions should be a key priority for future L-band soil moisture development, and model-based (e.g., GLDAS) soil moisture products appear to provide more accurate soil moisture estimates over these regions.
Soil moisture (SM) plays a critical role in hydrological and land-atmosphere coupling and therefore an accurate knowledge of its spatiotemporal dynamics is therefore essential for understanding related hydro-climatic processes (Koster et al., 2004; Brocca et al., 2014; Ford et al., 2014; Crow et al., 2018). Relative to in-situ observations, satellite remote sensing provides soil moisture retrievals with a global coverage, which makes it more suitable for large-scale hydrological and climate analyses (Massari et al., 2015; McColl et al., 2017; Koster et al., 2019). However, considerable uncertainties remain in SM remotely sensed retrievals (Karthikeyan et al., 2017; Beck et al., 2021), which forms the focus of the study reported here.
Satellite remote sensing of soil moisture is generally characterized by sensor types (i.e., active and passive) and microwave bands such as X-, C-, and L-bands used for the retrievals. Whereas, active microwave sensors transmit pulses of microwave energy to the land surface and measure the backscatter coefficient over vegetated regions (Mo et al., 1984; Wagner et al., 1999), the passive sensors observe microwave emission at various frequencies from the Earth's surface (Njoku and Kong, 1977; Jackson et al., 1982; Mo et al., 1982). The latter (i.e., passive) largely occupies the history of SM remote sensing compared to the active sensors (Kim et al., 2019). The first passive sensor for retrieving SM was SMMR (note that all undefined acronyms and abbreviations used in this study are listed in the Appendix) onboard the Nimbus-7 satellite launched in the late 1970s, which could observe brightness temperatures through multiple channels including C-band (6.6 GHz). Since then, the genealogy of passive microwave sensors has been expanded to X- (de Jeu et al., 2008; Fujii et al., 2009; Parinussa et al., 2014, 2015; Kang et al., 2020) and L-bands (Kerr et al., 2001; Entekhabi et al., 2010; Wigneron et al., 2021) over the last four decades.
While the L-band SM products (i.e., SMAP, SMOS-IC, and SMOS) are relatively new, recent validation studies using in-situ data as reference have reported that the L-band SM products generally outperform X- and C-band retrievals. For example, Cui et al. (2018) showed that SMAP and SMOS are consistently better than AMSR2 and FengYun-3B over two dense networks of ground stations. Ma et al. (2019) presented L-band SM products (SMAP and SMOS-IC) are generally better than a X-band derived product (AMSR2-LPRM) using global ground-based observations distributed over varied climate zones. Zhang et al. (2021) showed that the temporal correlation of SMAP and SMOS-IC against references is better than that of ASCAT except for tropical regions. This is because L-band is theoretically more sensitive to SM dynamics and has a better penetration capacity through vegetation cover (Jackson, 1993; Wigneron et al., 1995; Njoku and Entekhabi, 1996).
Due to the point-footprint scale differences, traditional in-situ-based validation is subject to significant spatial representative error, and dense soil moisture networks that are representative of footprint scale soil moisture dynamics are limited (Miralles et al., 2010; Crow et al., 2012; Chen et al., 2016). Triple collocation (TC) is a large-scale validation technique by which error variances and data-truth correlation coefficients of three independent datasets can be estimated without a reference (i.e., truth; Stoffelen, 1998; McColl et al., 2014). TC has been extensively used and tested for validating various remote sensing- and model-based SM products (Scipal et al., 2010; Miyaoka et al., 2017; Chen et al., 2018; Zhang et al., 2021). However, the three L-band SM products (i.e., SMAP, SMOS-IC, and SMOS) have not been simultaneously considered and compared through triple collocation analyses (TCA). This is mainly due to efforts not to violate the assumption of zero error-correlation, a key assumption implicit within TC (Gruber et al., 2016b). Generally, to fulfill the assumption of zero error cross-correlation, it is common to define the triplet used in TC with independent datasets as possible (e.g., active + passive + model). In this aspect, products resulting from the same sensor type and/or same band tend to be not preferred in TC. Consequently, simultaneous consideration on the three L-band products for TC has received relatively little attention, creating a need to fill this gap for data users and developers to identify product strengths and weaknesses. As shown in a large number of validation studies mentioned above, a SM product is not absolutely superior in all spaces and times because of retrieval conditions present (e.g., RFI and vegetation density; Zhou et al., 2019) and limited parameterizations of physical conditions. Consequently, various products tend to exhibit complementarity in their performances. In addition, the performance complementarity represented by error variances and error covariances play an important role in merging multiple datasets for achieving improvements in the merged dataset (Bates and Granger, 1969; Gruber et al., 2017; Kim S. et al., 2020).
Our contributions through this study are as follows. First, we compared anomaly time series of the three L-band SM products in terms of error variance and squared data-truth Pearson correlation coefficients via TC analyses using three separate data triplet combinations. Second, the assessments for various climatic and land cover conditions were classified to further investigate their performance in space. We believe our TC-based validation study can provide usage guidelines and directions for further improvements of the L-band soil moisture products. The rest of this paper is structured as follows. In section Materials and Methods, we first describe the datasets used in this study and their data preprocessing. The triple collocation analyses along with associated data triplets are also detailed. This is followed by the results and discussion in section Results and Discussion and we conclude in section Summary and Conclusions with a summary of key findings drawn.
The comparison of the three passive L-band soil moisture datasets was conducted for a 5-year period from April 1st, 2015 to March 31st, 2020. Table 1 shows a summary of main differences of the three retrieval algorithms, SMAP, SMOS-IC, and SMOS. The summary is based on the key references, including O'Neill et al. (2016) for SMAP; Fernandez-Moran et al. (2017) and Wigneron et al. (2021) for SMOS-IC; Kerr et al. (2012), Al Bitar et al. (2017), and Wigneron et al. (2017) for SMOS, through which readers can refer to further details. In addition to the three passive L-band soil moisture datasets compared, two additional SM products, ASCAT and the surface soil moisture of GLDAS-CLS, were used for constructing TC triplets as independent SM inputs. The details of the datasets used for this study are summarized in Table 2.
In order to filter out unreliable satellite SM retrievals, the following preprocessing is applied. For SMAP, we use pixels where the percentages of the open water area were <10%; vegetation water content (VWC) <5 kg/m2; the fraction of the frozen condition (land surface temperature <273.15 K) <10%; recommended by the retrieval quality flag (Das et al., 2013). For SMOS-IC, the scene flag (SF) and the root-mean-square error of brightness temperature (TB-RMSE) are used for the filtering, by which we masked pixels with SF >1 or TB-RMSE >8 K. Here, the former indicates complex topography and conditions with frozen, urbanization, ice, and water bodies; the latter implies being under considerable radio frequency interference (RFI). For SMOS, we use pixels where the RFI probability was <25%; SM data quality index <0.06; global quality index <10 according to the recommendations in Bengoa and Zapata (2009) and Al-Yaari et al. (2014). For ASCAT, pixels are used if they have <10% probability of snow, frozen ground, and 50% of estimated retrieval error as recommended by Chen et al. (2018). Note that SM data from the morning overpass of the satellites were used by considering that morning is more favorable for retrieving passive soil moisture (de Jeu et al., 2008). Then, the SM products with various spatial resolutions were resampled to the 36 km Equal-Area Scalable Earth Grid version 2.0 (EASE-Grid 2.0) (Brodzik et al., 2012) using the nearest neighbor.
Finally, the TCA results are evaluated by various climate zone (CZ) and land cover (LC) classes. In this study, we use the five primary CZ classes from the updated Köppen–Geiger climate classification presented by Peel et al. (2007): tropical, arid, temperate, cold, and polar regions; the six classes from MODIS MCD12C1 (Friedl et al., 2010): forest, shrublands, woodlands, grasslands, croplands, and unvegetated regions.
Triple Collocation (TC) has been instrumental in estimating error variances and correlation coefficients against the unknown truth through an inter-comparison of three independent products (Stoffelen, 1998; McColl et al., 2014). It is known that TC robustly works for anomaly time series (Miralles et al., 2010). This is because the mean seasonal cycle of geophysical data has a limited degree of freedom, and consequently, climatology errors across different datasets are more likely to be cross-correlated and violate the TC assumption, i.e., zero error-correlation (Draper et al., 2013). Thus, this study aims to compare the soil moisture datasets based on TC analyses (TCA) using their anomaly times series.
To obtain the anomaly time series from the raw data, we first calculated the seasonal cycle at each pixel by applying a 31-day moving average (−15 to 15 days) to the raw data, allowing estimation of the anomaly time series by subtracting the seasonal cycle out. For a triplet of zero-mean anomaly time series xi (i = 1, 2, and 3), each data is linearly related to the truth (t) and consists of two additive terms: xi = αit + εi, where αi is the multiplicative bias term, and εi is random error with zero mean. To remove the differences in the dynamic ranges of the datasets, the original data xi is scaled against a reference xr as
Here, the scaling factor αr/αi is estimated as
where xk is another product which is independent from both xr and xi (Yilmaz and Crow, 2013). Hereafter, the products used in this study represent scaled data as per (1) and (2), and the asterisk * is dropped for clarity and succinctness. The three triplets used for the TCA in this study are composed of the “ASCAT+ GLDAS-CLS+L-band product (i.e., SMAP, SMOS-IC, or SMOS),” and GLDAS-CLS is used as the reference xr in common for the scaling. For scaling ASCAT, a L-band product is denoted as xj, and vice versa.
For the scaled products, variances () and covariances (σij, i ≠ j) can be simplified as Equations (3) and (4) based on the assumption of truth-error orthogonality, meaning covariances between the truth and the errors are zero.
where σt is the standard deviation of t. By further adopting the assumption of zero error cross-correlation, i.e., σεiεj = 0, the simultaneous (Equations 3, 4) for i = 1, 2, and 3 can be solved for error variances e2 and the squared data-truth Pearson correlation coefficients ρ2. Accordingly, the column vectors e2 and ρ2 can be represented as
e2 and ρ2 for each L-band product are separately estimated from each triplet as mentioned above. For comparison, we also estimate those for GLDAS-CLS by averaging the results from the three triplets presented in Figures 3, 4. Note only pixels with 50 paired observations at the minimum are used to ensure the statistical robustness of the estimated e2 and ρ2.
In this section, we first present global maps of TCA estimated error variances e2 and squared data-truth correlation coefficients ρ2 for different products. Then, we further stratify their relative performances results by climate and land cover conditions described in section Data.
It is noticeable from the above results that SMOS-IC (top panel of Figure 1) is considerably improved compared to SMOS (middle). The advances in SMOS-IC result from improvements in parameterization and data filtering in the retrieval algorithm (Li et al., 2020b; Wigneron et al., 2021). As presented in the middle row of Figure 1, SMOS exhibits considerably disparate patterns and substantially degraded performances compared to the other two L-band products. The areas of performance degradation for SMOS broadly appear all over the world, and comparable performances with SMOS-IC (middle) and SMAP (bottom) and are observed over only a few regions such as the western part of North America, the south ends of South America and South Africa, and East of Australia. The superiority of SMAP for both metrics is generally observed over most global land grid-cells (bottom), and SMOS-IC ranks next and presents similar spatial patterns of both statistics (top). While the performance degradation of SMAP and SMOS-IC mainly appears over arid regions including northern Africa, Northeast China, and Central Australia, SMOS-IC shows the aspect of somewhat supplementing SMAP over east of North America, South America, western Africa, northern China, and central Australia. This complementarity of their performances is well-illustrated in Figure 2, of which implication in terms of data improvement will be discussed in section Summary and Conclusions.
Figure 1. TCA results for error variances e2 (Left) and squared Pearson correlation coefficients ρ2 (Right) for SMOS-IC (Top), SMOS (Middle), and SMAP (Bottom). Here the gray background on land indicates regions masked by the data preprocessing described in section Data.
Figure 2. Global rank maps showing soil moisture datasets, SMAP (red), SMOS-IC (green), and SMOS (blue), presenting the lowest error variances e2 (Left) and the highest squared Pearson correlation coefficients ρ2 (Right). Here, the numerical values in parentheses represent proportions in percentages that the three soil moisture datasets show the lowest (highest) e2 (ρ2). Here the gray background on land indicates regions masked by the data preprocessing described in section Data.
Previous studies in recent years are in line with the above-mentioned findings. Fernandez-Moran et al. (2017) demonstrated that SMOS-IC is considerably improved compared to SMOS against a reanalysis soil moisture data. Chen et al. (2018) presented that SMAP generally outperforms SMOS in terms of data-truth correlation through TCA. Zhang et al. (2021) showed a similar spatial pattern of TC-based correlation coefficients of SMAP and SMOS-IC.
For further evaluation, we also present rank maps in Figure 2, where a dataset is presented at each grid cell if it has the smallest (largest) e2 (ρ2) among the three products.
The three-way comparison in Figure 2 clearly shows that SMAP surpasses the others over considerable portions of the world, 69% for e2 and 65% for ρ2. The remaining parts are mostly filled with SMOS-IC, 29% for e2 and 34% for ρ2, but the domain of SMOS is at ignorable levels for both metrics. Despite the general superiority of SMAP over the globe, the advantage of SMOS-IC is apparent over east of North America, South America, western Africa, northern China, and central Australia. Figure A1 shows another three-way comparison among SMAP, SMOS-IC, and ASCAT to investigate coincidence with existing results. With half of the pixels representing the superiority of SMAP, ASCAT's strengths over Central Asia, northeast America, and southeast America are prominent in both for e2 and ρ2. In case of SMOS-IC, competitive strengths over Australia are noticeable. These patterns are also observable from Kim H. et al. (2020) in which the TCA was implemented using the triplet of SMAP, SMOS-IC, and ASCAT.
In this section, we categorize the results presented in Figures 1, 2 by the five primary CZ classes (tropical, arid, temperate, cold, and polar regions), and the six primary LC classes (forest, shrublands, woodlands, grasslands, croplands, and unvegetated regions), by which possible reasons can be identified with relation to the differences in their retrieval algorithms (Table 1). The categorized results are then presented as boxplots in Figure 3. Here, the metrics of GLDAS-CLS are also included for comparison as averages of the results from the three data triplets. Note that the findings to be presented later do not reflect the performance of the L-band products in dense vegetation because dense vegetation areas were masked through the filtering criteria described in section Data (e.g., VWC <5 kg/m2).
Although its performance tends to vary across different climate and land cover classes for both metrics, SMAP generally outperforms the other products. To be more explicit in the comparison, we sequentially carried out product-wise one-tailed t-tests to assess if the mean of e2 (ρ2) for SMAP is smaller (greater) than that of one of the remaining products (at a significance level α = 0.05). The differences are found significant excepting only for two cases of e2 against GLDAS-CLS over the polar climate and the unvegetated regions. Although SMAP generally outperforms SMOS-IC across the climate and land cover classes, their absolute differences are not as notable as the considerable differences in their occupied ratios presented in Figure 2. While SMOS-IC shows a competitive performance with SMAP, algorithmic improvements and better land surface parameterization can further improve performances. As summarized in Table 1, the most important difference between the SMAP (SCA-V, one polarization, and one incidence angle) and SMOS-IC (V2, multi-angular, and dual-polarization) algorithms is that while SMAP needs external NDVI/LAI derived VWC to estimate vegetation optical depth, SMOS-IC simultaneously retrieve soil moisture and vegetation optical depth based on observations without ancillary data. In addition to this, there are differences between the land surface parameters and the data source for calculating the effective soil temperature. Therefore, sensitivity analyses based on the differences can provide a step to improve the performance.
Figure 3. Box plots representing error variances e2 (Top) and squared Pearson correlation coefficients ρ2 (Bottom) of SMAP (red), SMOS-IC (green), SMOS (blue), and GLDAS-CLS (white), conditioned by five climate (left), and six land cover classes (right).
Both metrics of SMOS-IC are generally better than those for SMOS and GLDAS-CLS, and SMOS shows mostly lower performances than the others across the climate and land cover classes. It is notable the superiority of SMOS-IC compared to SMOS over the overall conditions, which results from the algorithmic improvements in SMOS-IC (Fernandez-Moran et al., 2017; Wigneron et al., 2021). The SMOS-IC algorithm considers pixels as homogeneous without using uncertain ancillary datasets, uses calibrated values of scattering albedo and roughness parameters, and does not rely on MODIS vegetation product for initializing the vegetation optical depth. The new version algorithm (V2) achieves further improvements in vegetation optical depth initialization; data filtering; application of a higher-order radiative transfer modeling; development of a multi-temporal approach considering temporally slowly changing vegetation. GLDAS-CLS outperforms SMOS overall and even shows similar levels of e2 with SMOS-IC over the polar climate and the unvegetated regions. The performance degradation of the satellite-derived products over the unvegetated areas which is partially overlapped with arid and semi-arid regions is notable. Several previous studies (Su et al., 2011; Ma et al., 2019) have reported the shortcomings of satellite-based soil moisture retrievals over sparsely vegetated regions. Su et al. (2011) showed increased uncertainty levels of global coarse resolution soil moisture products through comparisons with soil moisture data from the Tibetan Plateau observatory. Ma et al. (2019) presented poor performances of satellite-derived soil moisture data against in situ measurements collected from arid regions, especially over arid-desert-cold areas. O'Neill et al. (2016) reported that soil temperature profiles can be non-uniformly formed in arid areas based on their preliminary results, which could lead soil moisture retrieval issues.
The bar charts in Figure 4 present global occupied ratios (%) for both metrics of the three L-band products as well as GLDAS-CLS to provide a different perspective on the performance results obtained. Here, the occupation ratio (%) is defined as the proportion of the products presenting the lowest error variances e2 (top) and the highest squared Pearson correlation coefficients ρ2 (bottom) for each climate or land cover class. While SMAP is generally excellent across the climate and land cover classes, SMAP shows considerable performance degradation compared to others over unvegetated areas, partially including arid and semi-arid regions. GLDAS-CLS shows a similar functioning with SMAP, and SMOS rather shows better performances compared to itself for other land cover classes. A possible reason for the degradation of SMAP over arid regions is the way to compute the effective soil temperature (Teff), considering the non-uniform soil temperature profiles especially in arid areas (O'Neill et al., 2016). As summarized in Table 1, the three retrieval algorithms are based on Choudhury scheme (Choudhury et al., 1982) in common, but use different parameters and ancillary datasets (sources and depths) for the calculation of Teff. Particularly, SMAP uses 0.246 of the C parameter suggested by Choudhury et al. (1982), but SMOS-IC/SMOS use C varied by soil moisture and soil type which could be a hint to reduce the performance degradation of SMAP over unvegetated regions.
Figure 4. Bar charts showing global occupied ratios in percentages of SMAP (red), SMOS-IC (green), SMOS (blue), and GLDAS-CLS (white) presenting the lowest error variances e2 (Top) and the highest squared Pearson correlation coefficients ρ2 (Bottom) for each climate or land cover class.
As remote sensing technology advances, the hydrological application of satellite-derived soil moisture data is increasing. In particular, the superiority of passive L-band products stands out compared to products derived from other microwave bands. Nevertheless, there is little effort for intercomparing existing L-band soil moisture products through a large-scale validation technique such as triple collocation. In this regard, this study compared three passive L-band derived soil moisture datasets, SMAP, SMOS-IC, and SMOS at a global scale through triple collocation analyses, aiming to identify strengths, and weaknesses inherent in each product.
The key findings of the study are as follows.
1. Considerable improvements in SMOS-IC are observed compared to SMOS—confirming the positive contribution of retrieval algorithm improvement for the SMOS mission.
2. SMAP generally outperforms the other products over the globe, being followed by SMOS-IC and SMOS in that order.
3. Despite the superiority of SMAP, we note that SMOS-IC supplements the weakness of SMAP over some regions including east of North America, South America, western Africa, northern China, and central Australia.
The findings presented here show that strengths and weaknesses of the three L-band soil moisture products in varied climate and land cover regions across the globe. From the results presented here, one could conclude that a merging of SMAP and SMOS-IC would result in improved soil moisture estimation at the global scale.
Note that we were not able to estimate the error covariances of the studied products due to the inherent limitations of dependence across the three separate triplets to be used in TCA. The improvement in the merged data (i.e., minimization of the mean squared error) results from complementarity in their retrieval algorithms, sensors, and so on. This complementarity is explicitly reflected in the merging process by the error covariance matrix consisting of error variances (diagonal) and error covariances (off-diagonal) (Timmermann, 2006). While only the error variances with or without TC have been popularly used for merging various datasets as the next best thing (Clemen and Winkler, 1986; Gruber et al., 2017), Kim S. et al. (2020) demonstrated that data merging results considering the error covariances are superior than those for cases that do not consider it. Recent advances in TC affiliates could allow us to estimate the error covariances. For example, Gruber et al. (2016a) introduced the quadruple collocation (QC) that used a data quadruple to estimate the error covariances by relaxing the assumption of error independence among the datasets. Dong et al. (2020) proposed an extended double instrumental variable (EIVD) method, which needs only two independent products for replicating QC. Besides, it is notable that Wu et al. (2021) proposed a dynamic TC scheme in which time-varying error variances can be estimated through temporally moving windows. The time-varying error variances can be incorporated into a temporal dynamic setting of data merging for better merging results (Coulson and Robins, 1993; Chowdhury and Sharma, 2011). Aside from the purpose of merging datasets, the knowledge of error covariance is also useful for improving error estimation. Yilmaz and Crow (2014) pointed out that ignoring the error covariances in TC can underestimate errors.
Lastly, it should also be acknowledged that the validation results here can be further classified with more diverse static and dynamic retrieval conditions. For example, Zhang et al. (2019) carried out a multidimensional analysis of the performance of SMAP regulated under static conditions (climate, soil properties, and land cover), and dynamic properties (soil wetting, vegetation density, and surface temperature). This can allow us to view soil moisture products from different angles and provide data users with further insights into improvements and guidance.
Publicly available datasets were analyzed in this study. This data can be found here: SMAP, GLDAS-CLS and MODIS: https://earthdata.nasa.gov/; SMOS: https://www.catds.fr/; SMOS-IC: https://ib.remote-sensing.inrae.fr/; Updated Köppen-Geiger climate map of the world: https://people.eng.unimelb.edu.au/mpeel/koppen.html.
All authors listed have made a substantial, direct and intellectual contribution to the work, and approved it for publication.
The authors declare that the research was conducted in the absence of any commercial or financial relationships that could be construed as a potential conflict of interest.
Al Bitar, A., Mialon, A., Kerr, Y. H., Cabot, F., Richaume, P., Jacquette, E., et al. (2017). The global SMOS Level 3 daily soil moisture and brightness temperature maps. Earth Syst. Sci. Data 9:293. doi: 10.5194/essd-9-293-2017
Al-Yaari, A., Wigneron, J. P., Ducharne, A., Kerr, Y., De Rosnay, P., De Jeu, R., et al. (2014). Global-scale evaluation of two satellite-based passive microwave soil moisture datasets (SMOS and AMSR-E) with respect to Land Data Assimilation System estimates. Remote Sens. Environ. 149, 181–195. doi: 10.1016/j.rse.2014.04.006
Bates, J. M., and Granger, C. W. J. (1969). The combination of forecasts. J. Operat. Res. Soc. 20, 451–468. doi: 10.1057/jors.1969.103
Beck, H. E., Pan, M., Miralles, D. G., Reichle, R. H., Dorigo, W. A., Hahn, S., et al. (2021). Evaluation of 18 satellite-and model-based soil moisture products using in situ measurements from 826 sensors. Hydrol. Earth Syst. Sci. Discuss. 25, 17–40. doi: 10.5194/hess-25-17-2021
Bengoa, B., and Zapata, M. (2009). SMOS Level 2 and Auxiliary Data Products Specifications. Paris. SO-TN-IDR-GS-0006, 358.
Brocca, L., Ciabatta, L., Massari, C., Moramarco, T., Hahn, S., Hasenauer, S., et al. (2014). Soil as a natural rain gauge: estimating global rainfall from satellite soil moisture data. J. Geophys. Res. Atmosph. 119:2014JD021489. doi: 10.1002/2014JD021489
Brodzik, M. J., Billingsley, B., Haran, T., Raup, B., and Savoie, M. H. (2012). EASE-grid 2.0: incremental but significant improvements for earth-gridded data sets. ISPRS Int. J. Geo Inform. 1, 32–45. doi: 10.3390/ijgi1010032
CATDS (2016). CATDS-PDC L3SM Filtered - 1 Day Global Map of Soil Moisture Values From SMOS Satellite. CATDS(CNES, IFREMER, CESBIO).
Chen, F., Crow, W. T., Bindlish, R., Colliander, A., Burgin, M. S., Asanuma, J., et al. (2018). Global-scale evaluation of SMAP, SMOS and ASCAT soil moisture products using triple collocation. Remote Sens. Environ. 214, 1–13. doi: 10.1016/j.rse.2018.05.008
Chen, J. L., Wen, J., and Tian, H. (2016). Representativeness of the ground observational sites and up-scaling of the point soil moisture measurements. J. Hydrol. 533, 62–73. doi: 10.1016/j.jhydrol.2015.11.032
Choudhury, B. J., Schmugge, T. J., and Mo, T. (1982). A parameterization of effective soil temperature for microwave emission. J. Geophys. Res. Oceans 87, 1301–1304. doi: 10.1029/JC087iC02p01301
Chowdhury, S., and Sharma, A. (2011). Global sea surface temperature forecasts using a pairwise dynamic combination approach. J. Clim. 24, 1869–1877. doi: 10.1175/2010JCLI3632.1
Clemen, R. T., and Winkler, R. L. (1986). Combining economic forecasts. J. Bus. Econ. Stat. 4, 39–46. doi: 10.1080/07350015.1986.10509492
Coulson, N. E., and Robins, R. P. (1993). Forecast combination in a dynamic setting. J. For. 12, 63–67. doi: 10.1002/for.3980120106
Crow, W. T., Berg, A. A., Cosh, M. H., Loew, A., Mohanty, B. P., Panciera, R., et al. (2012). Upscaling sparse ground-based soil moisture observations for the validation of coarse-resolution satellite soil moisture products. Rev. Geophys. 50:RG2002. doi: 10.1029/2011RG000372
Crow, W. T., Chen, F., Reichle, R. H., Xia, Y., and Liu, Q. (2018). Exploiting soil moisture, precipitation, and streamflow observations to evaluate soil moisture/runoff coupling in land surface models. Geophys. Res. Lett. 45, 4869–4878. doi: 10.1029/2018GL077193
Cui, C., Xu, J., Zeng, J., Chen, K.-S., Bai, X., Lu, H., et al. (2018). Soil moisture mapping from satellites: an intercomparison of SMAP, SMOS, FY3B, AMSR2, and ESA CCI over two dense network regions at different spatial scales. Remote Sens. 10:33. doi: 10.3390/rs10010033
Das, N. N., Entekhabi, D., Njoku, E. G., Shi, J. J., Johnson, J. T., and Colliander, A. (2013). Tests of the SMAP combined radar and radiometer algorithm using airborne field campaign observations and simulated data. IEEE Trans. Geosci. Remote Sens. 52, 2018–2028. doi: 10.1109/TGRS.2013.2257605
de Jeu, R. A. M., Wagner, W., Holmes, T. R. H., Dolman, A. J., Van De Giesen, N. C., and Friesen, J. (2008). Global soil moisture patterns observed by space borne microwave radiometers and scatterometers. Surv. Geophys. 29, 399–420. doi: 10.1007/s10712-008-9044-0
Dong, J., Wei, L. N., Chen, X., Duan, Z., and Lu, Y. (2020). An instrument variable based algorithm for estimating cross-correlated hydrological remote sensing errors. J. Hydrol. 581:124413. doi: 10.1016/j.jhydrol.2019.124413
Draper, C., Reichle, R., De Jeu, R., Naeimi, V., Parinussa, R., and Wagner, W. (2013). Estimating root mean square errors in remotely sensed soil moisture over continental scale domains. Remote Sens. Environ. 137, 288–298. doi: 10.1016/j.rse.2013.06.013
Entekhabi, D., Njoku, E. G., O'neill, P. E., Kellogg, K. H., Crow, W. T., Edelstein, W. N., et al. (2010). The soil moisture active passive (SMAP) mission. Proc. IEEE 98, 704–716. doi: 10.1109/JPROC.2010.2043918
Fernandez-Moran, R., Al-Yaari, A., Mialon, A., Mahmoodi, A., Al Bitar, A., De Lannoy, G., et al. (2017). SMOS-IC: an alternative SMOS soil moisture and vegetation optical depth product. Remote Sens. 9:457. doi: 10.3390/rs9050457
Ford, T. W., Wulff, C. O., and Quiring, S. M. (2014). Assessment of observed and model-derived soil moisture-evaporative fraction relationships over the United States Southern Great Plains. J. Geophys. Res. Atmosph. 119:2014JD021490. doi: 10.1002/2014JD021490
Friedl, M. A., Sulla-Menashe, D., Tan, B., Schneider, A., Ramankutty, N., Sibley, A., et al. (2010). MODIS Collection 5 global land cover: algorithm refinements and characterization of new datasets. Remote Sens. Environ. 114, 168–182. doi: 10.1016/j.rse.2009.08.016
Fujii, H., Koike, T., and Imoka, K. (2009). Improvement of the AMSR-E algorithm for soil moisture estimation by introducing a fractional vegetation coverage dataset derived from MODIS data. J. Remote Sens. Soc. Jpn. 29, 282–292. doi: 10.11440/rssj.29.282
Gruber, A., Dorigo, W. A., Crow, W., and Wagner, W. (2017). Triple collocation-based merging of satellite soil moisture retrievals. IEEE Trans. Geosci. Remote Sens. 55, 6780–6792. doi: 10.1109/TGRS.2017.2734070
Gruber, A., Su, C. H., Crow, W. T., Zwieback, S., Dorigo, W. A., and Wagner, A. (2016a). Estimating error cross-correlations in soil moisture data sets using extended collocation analysis. J. Geophys. Res. Atmosph. 121, 1208–1219. doi: 10.1002/2015JD024027
Gruber, A., Su, C. H., Zwieback, S., Crowd, W., Dorigo, W., and Wagner, W. (2016b). Recent advances in (soil moisture) triple collocation analysis. Int. J. Appl. Earth Observ. Geoinform. 45, 200–211. doi: 10.1016/j.jag.2015.09.002
Jackson, T. J., Schmugge, T. J., and Wang, J. R. (1982). Passive microwave sensing of soil-moisture under vegetation canopies. Water Resour. Res. 18, 1137–1142. doi: 10.1029/WR018i004p01137
Jackson, T. J. III. (1993). Measuring surface soil moisture using passive microwave remote sensing. Hydrol. Proc. 7, 139–152. doi: 10.1002/hyp.3360070205
Kang, C. S., Zhao, T., Shi, J., Cosh, M. H., Chen, Y., Starks, P. J., et al. (2020). Global soil moisture retrievals from the chinese FY-3D microwave radiation imager. IEEE Trans. Geosci. Remote Sens. 59, 4018–4032. doi: 10.1109/TGRS.2020.3019408
Karthikeyan, L., Pan, M., Wanders, N., Kumar, D. N., and Wood, E. F. (2017). Four decades of microwave satellite soil moisture observations: Part 2. Product validation and inter-satellite comparisons. Adv. Water Resour. 109, 236–252. doi: 10.1016/j.advwatres.2017.09.010
Kerr, Y. H., Waldteufel, P., Richaume, P., Wigneron, J. P., Ferrazzoli, P., Mahmoodi, A., et al. (2012). The SMOS soil moisture retrieval algorithm. IEEE Trans. Geosci. Remote Sens. 50, 1384–1403. doi: 10.1109/TGRS.2012.2184548
Kerr, Y. H., Waldteufel, P., Wigneron, J.-P., Martinuzzi, J., Font, J., and Berger, M. (2001). Soil moisture retrieval from space: the Soil Moisture and Ocean Salinity (SMOS) mission. IEEE Trans. Geosci. Remote Sens. 39, 1729–1735. doi: 10.1109/36.942551
Kim, H., Wigneron, J.-P., Kumar, S., Dong, J., Wagner, W., Cosh, M. H., et al. (2020). Global scale error assessments of soil moisture estimates from microwave-based active and passive satellites and land surface models over forest and mixed irrigated/dryland agriculture regions. Remote Sens. Environ. 251:112052. doi: 10.1016/j.rse.2020.112052
Kim, S., Pham, H., Liu, Y. Y., Marshall, L., and Sharma, A. (2020). Improving the combination of satellite soil moisture datasets by considering error cross-correlation: a comparison between triple collocation (TC) and extended double instrumental variable (EIVD) alternatives. IEEE Trans. Geosci. Remote Sens. Early Access. 1–11. doi: 10.1109/TGRS.2020.3032418
Kim, S., Zhang, R., Pham, H., and Sharma, A. (2019). A review of satellite-derived soil moisture and its usage for flood estimation. Remote Sens. Earth Syst. Sci. 2, 225–246. doi: 10.1007/s41976-019-00025-7
Koster, R. D., Dirmeyer, P. A., Guo, Z., Bonan, G., Chan, E., Cox, P., et al. (2004). Regions of strong coupling between soil moisture and precipitation. Science 305, 1138–1140. doi: 10.1126/science.1100217
Koster, R. D., Reichle, R. H., Schubert, S. D., and Mahanama, S. P. (2019). Length scales of hydrological variability as inferred from SMAP soil moisture retrievals. J. Hydrometeorol. 20, 2129–2146. doi: 10.1175/JHM-D-19-0070.1
Li, B., Beaudoing, H., Rodell, M., and Nasa/Gsfc/Hsl (2020). GLDAS Catchment Land Surface Model L4 Daily 0.25 x 0.25 Degree GRACE-DA1 V2.2. G.E.S.D.a.I.S.C.G. Disc. 2.2 ed. Greenbelt, MA.
Li, X., Al-Yaari, A., Schwank, M., Fan, L., Frappart, F., Swenson, J., et al. (2020a). Compared performances of SMOS-IC soil moisture and vegetation optical depth retrievals based on Tau-Omega and Two-Stream microwave emission models. Remote Sens. Environ. 236:111502. doi: 10.1016/j.rse.2019.111502
Li, X., Wigneron, J.-P., Frappart, F., Fan, L., Ciais, P., Fensholt, R., et al. (2021). Global-scale assessment and inter-comparison of recently developed/reprocessed microwave satellite vegetation optical depth products. Remote Sens. Environ. 253:112208. doi: 10.1016/j.rse.2020.112208
Li, X., Wigneron, J.-P., Frappart, F., Fan, L., Wang, M., Liu, X., et al. (2020b). “Development and validation of the SMOS-IC version 2 (V2) soil moisture product,” in IGARSS 2020-2020 IEEE International Geoscience and Remote Sensing Symposium (Waikoloa, HI: IEEE), 4434–4437. doi: 10.1109/IGARSS39084.2020.9323324
Ma, H., Zeng, J., Chen, N., Zhang, X., Cosh, M. H., and Wang, W. (2019). Satellite surface soil moisture from SMAP, SMOS, AMSR2 and ESA CCI: a comprehensive assessment using global ground-based observations. Remote Sens. Environ. 231:111215. doi: 10.1016/j.rse.2019.111215
Massari, C., Brocca, L., Ciabatta, L., Moramarco, T., Gabellani, S., Albergel, C., et al. (2015). The use of H-saf soil moisture products for operational hydrology: flood modelling over Italy. Hydrology 2, 2–22. doi: 10.3390/hydrology2010002
McColl, K. A., Alemohammad, S. H., Akbar, R., Konings, A. G., Yueh, S., and Entekhabi, D. (2017). The global distribution and dynamics of surface soil moisture. Nat. Geosci. 10, 100–104. doi: 10.1038/ngeo2868
McColl, K. A., Vogelzang, J., Konings, A. G., Entekhabi, D., Piles, M., and Stoffelen, A. (2014). Extended triple collocation: estimating errors and correlation coefficients with respect to an unknown target. Geophys. Res. Lett. 41, 6229–6236. doi: 10.1002/2014GL061322
Miralles, D. G., Crow, W. T., and Cosh, M. H. (2010). Estimating spatial sampling errors in coarse-scale soil moisture estimates derived from point-scale observations. J. Hydrometeorol. 11, 1423–1429. doi: 10.1175/2010JHM1285.1
Mironov, V. L., Kosolapova, L. G., and Fomin, S. V. (2009). Physically and mineralogically based spectroscopic dielectric model for moist soils. IEEE Trans. Geosci. Remote Sens. 47, 2059–2070. doi: 10.1109/TGRS.2008.2011631
Miyaoka, K., Gruber, A., Ticconi, F., Hahn, S., Wagner, W., Figa-Saldana, J., et al. (2017). Triple collocation analysis of soil moisture from Metop-A ASCAT and SMOS against JRA-55 and ERA-Interim. IEEE J. Select. Top. Appl. Earth Observ. Remote Sens. 10, 2274–2284. doi: 10.1109/JSTARS.2016.2632306
Mo, T., Choudhury, B. J., Schmugge, T. J., Wang, J. R., and Jackson, T. J. (1982). A model for microwave emission from vegetation-covered fields. J. Geophys. Res. Oceans 87, 11229–11237. doi: 10.1029/JC087iC13p11229
Mo, T., Schmugge, T. J., and Jackson, T. J. (1984). Calculations of radar backscattering coefficient of vegetation-covered soils. Remote Sens. Environ. 15, 119–133. doi: 10.1016/0034-4257(84)90041-5
Naeimi, V., Scipal, K., Bartalis, Z., Hasenauer, S., and Wagner, W. (2009). An improved soil moisture retrieval algorithm for ERS and METOP scatterometer observations. IEEE Trans. Geosci. Remote Sens. 47, 1999–2013. doi: 10.1109/TGRS.2008.2011617
Njoku, E. G., and Entekhabi, D. (1996). Passive microwave remote sensing of soil moisture. J. Hydrol. 184, 101–129. doi: 10.1016/0022-1694(95)02970-2
Njoku, E. G., and Kong, J.-A. (1977). Theory for passive microwave remote sensing of near-surface soil moisture. J. Geophys. Res. 82, 3108–3118. doi: 10.1029/JB082i020p03108
O'Neill, P., Njoku, E., Jackson, T., Chan, S., and Bindlish, R. (2016). Soil Moisture Active Passive (SMAP) Algorithm Theoretical Basis Document Level 2 and 3 Soil Moisture (Passive) Data Products. Pasadena, CA: Jet Propulsion Laboratory, California Institute of Technology, JPL D-66480. Revision B ed.
O'Neill, P. E., Chan, S., Njoku, E. G., Jackson, T., and Bindlish, R. (2018). SMAP L3 Radiometer Global Daily 36 km EASE-Grid Soil Moisture, Version 5 (SPL3SMP). Boulder, CO: NASA National Snow and Ice Data Center Distributed Active Archive Center.
Parinussa, R. M., Holmes, T. R. H., Wanders, N., Dorigo, W. A., and De Jeu, R. A. M. (2015). A preliminary study toward consistent soil moisture from AMSR2. J. Hydrometeorol. 16, 932–947. doi: 10.1175/JHM-D-13-0200.1
Parinussa, R. M., Wang, G., Holmes, T. R. H., Liu, Y. Y., Dolman, A. J., De Jeu, R., et al. (2014). Global surface soil moisture from the Microwave Radiation Imager onboard the Fengyun-3B satellite. Int. J. Remote Sens. 35, 7007–7029. doi: 10.1080/01431161.2014.960622
Peel, M. C., Finlayson, B. L., and Mcmahon, T. A. (2007). Updated world map of the Köppen-Geiger climate classification. Hydrol. Earth Syst. Sci. 11, 1633–1644. doi: 10.5194/hess-11-1633-2007
Scipal, K., Dorigo, W., and De Jeu, R. (2010). “Triple collocation - a new tool to determine the error structure of global soil moisture products,” in 2010 IEEE International Geoscience and Remote Sensing Symposium (IGARSS) (Honolulu, HI), 4426–4429. doi: 10.1109/IGARSS.2010.5652128
Stoffelen, A. (1998). Toward the true near-surface wind speed: error modeling and calibration using triple collocation. J. Geophys. Res. Oceans 103, 7755–7766. doi: 10.1029/97JC03180
Su, Z., Wen, J., Dente, L., Velde, R., Wang, L., Ma, Y., et al. (2011). The Tibetan Plateau observatory of plateau scale soil moisture and soil temperature (Tibet-Obs) for quantifying uncertainties in coarse resolution satellite and model products. Hydrol. Earth Syst. Sci. 15, 2303–2316. doi: 10.5194/hess-15-2303-2011
Timmermann, A. (2006). “Forecast combinations,” in Handbook of Economic Forecasting, eds. G. Elliott, C.W.J. Granger, and A. Timmermann, 135–196, Amsterdam: Elsevier. doi: 10.1016/S1574-0706(05)01004-9
Wagner, W., Lemoine, G., and Rott, H. (1999). A method for estimating soil moisture from ERS scatterometer and soil data. Remote Sens. Environ. 70, 191–207. doi: 10.1016/S0034-4257(99)00036-X
Wang, J., and Choudhury, B. (1981). Remote sensing of soil moisture content, over bare field at 1.4 GHz frequency. J. Geophys. Res. Oceans 86, 5277–5282. doi: 10.1029/JC086iC06p05277
Wigneron, J.-P., Chanzy, A., Calvet, J.-C., and Bruguier, N. (1995). A simple algorithm to retrieve soil moisture and vegetation biomass using passive microwave measurements over crop fields. Remote Sens. Environ. 51, 331–341. doi: 10.1016/0034-4257(94)00081-W
Wigneron, J.-P., Kerr, Y., Waldteufel, P., Saleh, K., Escorihuela, M.-J., Richaume, P., et al. (2007). L-band Microwave Emission of the Biosphere (L-MEB) Model: description and calibration against experimental data sets over crop fields. Remote Sens. Environ. 107, 639–655. doi: 10.1016/j.rse.2006.10.014
Wigneron, J.-P., Li, X., Frappart, F., Fan, L., Al-Yaari, A., De Lannoy, G., et al. (2021). SMOS-IC data record of soil moisture and L-VOD: Historical development, applications and perspectives. Remote Sens. Environ. 254:112238. doi: 10.1016/j.rse.2020.112238
Wigneron, J. P., Jackson, T. J., O'neill, P., De Lannoy, G., De Rosnay, P., Walker, J. P., et al. (2017). Modelling the passive microwave signature from land surfaces: a review of recent results and application to the L-band SMOS and SMAP soil moisture retrieval algorithms. Remote Sens. Environ. 192, 238–262. doi: 10.1016/j.rse.2017.01.024
Wu, K., Ryu, D., Nie, L., and Shu, H. (2021). Time-variant error characterization of SMAP and ASCAT soil moisture using triple collocation analysis. Remote Sens. Environ. 256:112324. doi: 10.1016/j.rse.2021.112324
Yilmaz, M. T., and Crow, W. T. (2013). The optimality of potential rescaling approaches in land data assimilation. J. Hydrometeorol. 14, 650–660. doi: 10.1175/JHM-D-12-052.1
Yilmaz, M. T., and Crow, W. T. (2014). Evaluation of assumptions in soil moisture triple collocation analysis. J. Hydrometeorol. 15, 1293–1302. doi: 10.1175/JHM-D-13-0158.1
Zhang, R., Kim, S., and Sharma, A. (2019). A comprehensive validation of the SMAP Enhanced Level-3 Soil Moisture product using ground measurements over varied climates and landscapes. Remote Sens. Environ. 223, 82–94. doi: 10.1016/j.rse.2019.01.015
Zhang, R., Kim, S., Sharma, A., and Lakshmi, V. (2021). Identifying relative strengths of SMAP, SMOS-IC, and ASCAT to capture temporal variability. Remote Sens. Environ. 252:112126. doi: 10.1016/j.rse.2020.112126
Zhou, W.-C., Han, Z., Han, S.-Y., Wang, Y.-Q., Zhang, X.-W., and Wu, Y.-S. (2019). Characteristics of L-band radio frequency interference detected via the soil moisture active passive radiometer in China and its offshore areas. Results Phys. 12, 1859–1865. doi: 10.1016/j.rinp.2019.01.062
Figure A1. Global rank maps showing soil moisture datasets, SMAP (red), SMOS-IC (green), and ASCAT (cyan) presenting the lowest error variances e2 (Left) and the highest squared Pearson correlation coefficients ρ2 (Right). Here, the results of ASCAT are represented as averages of the results from the three data triplets, and the numerical values in parentheses represent proportions in percentages that the three soil moisture datasets show the lowest (highest) e2 (ρ2). Here the gray background on land indicates regions masked by the data preprocessing described in section Data.
Keywords: soil moisture, L-band, triple collocation, SMAP, SMOS, SMOS-IC
Citation: Kim S, Dong J and Sharma A (2021) A Triple Collocation-Based Comparison of Three L-Band Soil Moisture Datasets, SMAP, SMOS-IC, and SMOS, Over Varied Climates and Land Covers. Front. Water 3:693172. doi: 10.3389/frwa.2021.693172
Received: 10 April 2021; Accepted: 02 June 2021;
Published: 28 June 2021.
Edited by:
David Pulido Velázquez, Instituto Geológico y Minero de España (IGME), SpainReviewed by:
Amen Al-Yaari, Université Paris-Sorbonne, FranceCopyright © 2021 Kim, Dong and Sharma. This is an open-access article distributed under the terms of the Creative Commons Attribution License (CC BY). The use, distribution or reproduction in other forums is permitted, provided the original author(s) and the copyright owner(s) are credited and that the original publication in this journal is cited, in accordance with accepted academic practice. No use, distribution or reproduction is permitted which does not comply with these terms.
*Correspondence: Seokhyeon Kim, c2Vva2h5ZW9uLmtpbUB1bnN3LmVkdS5hdQ==
Disclaimer: All claims expressed in this article are solely those of the authors and do not necessarily represent those of their affiliated organizations, or those of the publisher, the editors and the reviewers. Any product that may be evaluated in this article or claim that may be made by its manufacturer is not guaranteed or endorsed by the publisher.
Research integrity at Frontiers
Learn more about the work of our research integrity team to safeguard the quality of each article we publish.