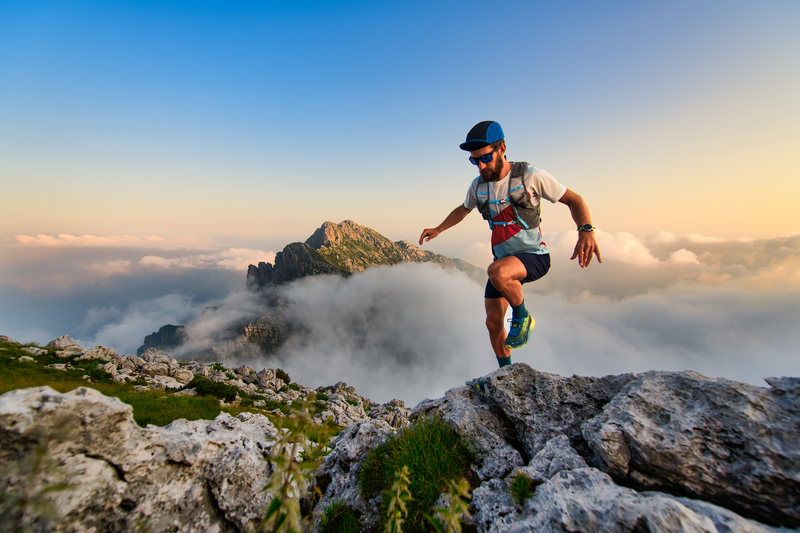
95% of researchers rate our articles as excellent or good
Learn more about the work of our research integrity team to safeguard the quality of each article we publish.
Find out more
ORIGINAL RESEARCH article
Front. Virtual Real. , 19 March 2025
Sec. Virtual Reality in Industry
Volume 6 - 2025 | https://doi.org/10.3389/frvir.2025.1560713
Virtual environments allow training of situations and scenarios that are hazardous and impractical to train for in the physical environment. This paper aims to illustrate the utility of a virtual reality glovebox with haptic feedback as an effective training tool for glovebox operations. Twenty-four participants (16 males, 8 females) volunteered to participate in this study. Each participant completed one trial that consisted of three counter-balanced environments (physical training glovebox, virtual reality with haptic feedback, and virtual reality with handheld controllers), followed by a 10-minute recovery phase after each environment. Each glovebox environment had a near identical glovebox task where participants removed, sorted, and then returned simulated hazardous material to a cannister. Median time to completion (TTC), number of errors, ratings of perceived exertion (RPE), and perceived recovery status scale (PRSS) were calculated to describe the difference in glovebox environment performance. Median TTC was near double for the virtual glovebox environments versus the physical training glovebox environment. Median number of errors were near zero for all environments. Median RPE and PRSS were relatively similar across environments. The results of this study conclude that while the TTC for the physical training glovebox was significantly quicker than the virtual environments, the accuracy of training, RPE, and PRSS were reasonably similar across all environments. Furthermore, given that there is little opportunity and resources for glovebox operators to train this type of task on a regular basis in a physical glovebox, having the opportunity to train this task in a virtual environment provides great value to increase their knowledge, skills, and safety.
Virtual reality (VR) has broad and numerous definitions, so many that peer-reviewed research studies have been conducted to try and unify the term. (Abbas et al., 2023) published one such study and provided a contemporary definition of VR. That definition states: “VR is a three-dimensional computer-generated simulated environment, which attempts to replicate real world imaginary environments and interactions, thereby supporting work, education, recreation, and health.”
The first widespread use of technology resembling contemporary VR was the Link Trainer application used to train pilots during World War II (Jeon, 2015). Today, VR is envisioned as head-mounted display (HMD) systems that occlude environmental information and depict the virtual environment to the user (Rauschnabel et al., 2022). HMDs originated in the gaming and entertainment industries, but are now commonly used for job training and prototyping (Shahab et al., 2021). Additionally, VR has been used in manufacturing (Berg and Vance, 2017) and healthcare (Fertleman et al., 2018), and as a tool to conduct research (Stadler et al., 2019).
In the nuclear industry, near constant communication is required between those working in the field and those in the main control room. VR has four main applications as a training tool for this industry: 1) fault, incident, and accident management, including collaboration with control room operators, 2) maintenance work process planning and training, 3) radiation visualization and hazard detection training, and 4) physical safety training (Pakarinen et al., 2021). These areas of application were identified because VR has provided immersive and collaborative training experiences with great fidelity when compared to some traditional training methods (Pakarinen et al., 2021). An example of this collaborative aspect is using VR to train a skill or process to individuals or groups who are geographically dispersed (Jensen and Konradsen, 2018).
In nuclear laboratories/facilities, the glovebox is a sealed enclosed space, allowing hazardous material handling in a safe environment by preventing the spread of contamination (Tugal, et al., 2023). Personal protective equipment and puncture-proof gloves are used by glovebox operators while working with the hazardous materials, which reduces the tactile capability due to the thickness and multiple layers of gloves. While this loss of tactile capability is a negative in the physical glovebox environment, it allows the gap between the virtual glovebox and the physical glovebox to narrow; thereby making what is felt in the virtual glovebox more similar to the physical glovebox. Additionally, due to the controlled and stationary nature of glovebox operations, using VR technologies as a training tool seems optimal without much risk for VR sickness (nausea, disorientation, discomfort, eye strain, and drowsiness) (Davis et al., 2014). An advantage of performing glovebox tasks (e.g., adding and removing simulated materials to a protective cannister) in VR versus a real-world environment is the opportunity to train high-risk/low-occurrence tasks in a safe and immersive environment (Jensen and Konradsen, 2018) compared to simply watching training videos or viewing a PowerPoint presentation.
Instead of using a generic virtual environment (VE), training can be made more realistic and familiar by using a digital twin (DT). The DT concept (a digital representation of a particular location and objects within that location) originated with the National Aeronautics and Space Administration (NASA) (Yarali, 2022) and is used within the industry sector for product lifecycle management. There are several compelling reasons for using a DT in training that are important for end-users. Using a DT in training is beneficial to both the organization and end-users because it provides an immersive “real-world” learning experience with consistency and scalability, and it is sustainable for product life-cycle management (Jones et al., 2020). DTs have, for the most part, remained unchanged in that they are a virtual model that integrates all relevant knowledge about a real environment to enable and address a specific issue (Bucchiarone, 2022). Like most things, DTs exist on a spectrum, but that explanation is outside the scope of this study.
In an optimal VE, interactions feel natural, training hinderances are minimized, and the ability to manipulate objects within the environment are realistic (Stamer et al., 2020). Additionally, the more immersive the VE is, the more realistic it should appear to the user; resulting in the user being more present (Slater et al., 1995). To further elaborate, extended reality XR technologies (such as VR), can be used to increase the realism of immersive environments, tasks, scenarios, and conditions since they can be constructed to more closely resemble the real environment. This advancement in technology, coupled with an increased availability for training opportunities allowed by XR, will increase users’ expertise on a task or skill—leading to a higher level of performance and proficiency. These features will prompt the user to think about the next step in the task or consider how to correct an error (if one occurs). However, it must be noted that tasks and scenarios are limited by what can be done in the VEs, which may be somewhat different from what can be done in the physical environment.
Most published research indicates that for VR to be adopted as a training aid there has to be a strong business case (Radhakrishnan et al., 2021). One common way that business case is made is by people, time, and resources being limited, which poses several challenges to industrial training (Abdullah, 2009). As this lack of quality training continues, critical skills, knowledge, and abilities continue to atrophy within the workforce (Hwang et al., 2024).
Given those challenges with training in the physical environment, VR technologies have become efficient and successful at helping train workers in industries that use hand tools or machines to complete a task. (Achberger et al., 2024; Berg and Vance, 2017; Zimmermann, 2008). Two main training capabilities provided by VR are being able to train, and even assist, with cognitive and motor learning. Juliano and Liew (2020) found that head-mounted VR systems significantly improved (p < 0.001) motor learning, which is a key component to becoming proficient at a task. While assistive systems provided cognitive support for complex work tasks at manual assembly workplaces (Bannat et al., 2008; Tang et al., 2003). This aid to training and the planning processes is due in part to VR increasing realism, immersion, and reliability (Grajewski and Hamrol, 2023; Wolf et al., 2020). Additionally, VR adds value by allowing workers to train skills and scenarios on their own (or with minimal instructor oversight) that would otherwise be too dangerous to initially perform in the real world. This allows for cost savings and increased safety (Elmqaddem, 2019; Pavlov et al., 2020; Piroozfar et al., 2017).
VR’s benefits are not without limitations. Previous research concluded that in certain instances VR may be less useful than training in the real world with physical tools and components (Butavicius et al., 2012; Winther et al., 2020). However, that is not to say that those negative outcomes were not due in part to the training scenario and technologies not being a “good fit” for the desired training outcome. One example that showed this being overcome was a VR-only scenario that was enhanced by combining tactile kinesthetic cues when simulating wiping dusty surfaces to make it more realistic (Hwang et al., 2024).
Furthermore, VR training cannot be seen as an omnipotent learning tool, because the effectiveness depends on the actual implementation. Linking the real-world conditions and learning goal with the VR training environment is crucial to achieving accurate models, for transferring the learned skills to actual work, and for ensuring a pleasant learning experience (Pakarinen et al., 2021). The learning goals should be carefully considered and determined, and the training tasks should be designed to promote the development of desired skills. Also, the level of support and guidance from the instructor, as well as the task content and familiarity, affect training results (Pakarinen et al., 2021). When a maintenance task was unfamiliar to the trainee, increased guidance from an experienced trainer improved performance (Nazir et al., 2014). The optimal levels of expert guidance and participation depend on the task novelty, difficulty, and contents. Therefore, general recommendations are difficult to make.
Haptic feedback (often shortened to just haptics) simulates the sense of touch. Humans perceive haptics through cutaneous and kinesthetic systems (Muender et al., 2022). Those systems allow for the perception of a material’s characteristics and the position/movement of their own body (Muender et al., 2022). Additionally, haptic gloves allow users to experience tactile feedback as they perform tasks. Results from (Achberger et al., 2024) indicated that combining weight and collision feedback offers the most significant benefits to haptics. When there were no technical limitations, about half of their participants indicated that being able to grab an object was the most essential feedback type. Furthermore, it was discovered that haptic feedback becomes more effective and natural when it is conveyed via multiple modalities compared to when it is unimodal (Abiri et al., 2019; Wang et al., 2019). For example, simulating the act of wiping dusty surfaces on mechanical components with a towel can be more realistically achieved in VR by combining tactile and kinesthetic cues.
For haptics to be practically useful, the haptic device must be able to interact with other virtual objects. Choi et al., 2019 produced a haptic feedback drilling interface with the same appearance as a conventional hand drill. While passive tools (screwdriver, saw and hammer (Strandholt et al., 2020), wrench, etc.) have also been developed with similar haptic capabilities to the wrench developed for the current study. The purpose of interacting with these objects during training is to help people gain practice (and confidence) working with similar objects before they work with them in the real world—where they could risk damaging the tool or causing an incident.
Combining VR and haptic feedback inside a DT increases the level of immersion because of the increased possibilities for interaction with the environment. The digital renderings allow the user to visualize the task environment as they interact with the simulation. Furthermore, this combination of technologies and components allows the user to experience the VE with most all their senses and interact naturally. Combining haptic feedback with VR inside a DT is what led to a haptic feedback glove (Figure 1), produced by HaptX (headquartered in San Luis Obispo, California), which captures the hand motions needed to perform a task and provides feedback to the user with microfluidic technology. This glove was selected for this study after considering the Manus VR and Sense glove as referenced in (Piviotti, 2021).
Therefore, the purpose of this study was to determine if there is a statistically significant difference for glovebox operations performance among physical training glovebox, haptic plus VR, and handheld controller plus VR environments. We hypothesized that the physical training glovebox environment will have the highest level of performance versus the haptic or handheld environments due to participants being accustomed to working with physical objects. However, the VEs will benefit the participants by allowing them to perform tasks that could not normally be performed outside of a physical glovebox in a controlled environment.
This study was reviewed and approved by the United States Department of Energy’s Central Institutional Review Board (ID number: HRP-510b). A sample size of 24 participants (16 males and 8 females) were recruited and subsequently volunteered to participate in this study. Upon entering the XR laboratory, participants were oriented with each of the glovebox environments (handheld controller with VR HMD, haptic feedback gloves with VR HMD, and the physical training glovebox), and provided an explanation of the purpose, risks, and rewards of the study. Participants were then given a consent form to read and sign if they wanted to volunteer to participate in the study. In the same consent form, a separate field was provided for participants to consent and sign their name to participate in a post-trial questionnaire (described below) and to be audio/video recorded while answering those questions. Each participant consented to the post-trial questionnaire, and most all participants agreed to be recorded while answering the questions. However, since each participant’s qualitative responses about their experience was for internal use only, the detailed description is outside the scope of this study, and their name, image, and likeness are not approved for public release.
Participants then completed the Physical Activity Readiness Questionnaire (Par-Q+) to determine if they were generally healthy enough to participate in the study (healthiness was not a significant concern as the glovebox tasks were less strenuous than their normal work). After the Par-Q+, participants completed the Motion Sickness Susceptibility Questionnaire Short-Form (MSSQ-short) to gauge how susceptible they were to motion sickness in the past. If the Par-Q+ and the MSSQ-short did not return any ‘red flags,’ participants were allowed to participate in the study. If “red flags” were found, they were discussed with the participant and the decision was made whether or not to exclude them from the study. An example of a “red flag” is if the person indicated on the MSSQ-short that they had suffered severe motion sickness in the past that caused them to not be able to complete the task. To tie participant’s motion sickness history to technology use, participants were also asked “if they had used VR or AR to train for a task.” All participants indicated they had never used VR or AR technologies for training purposes.
Prior to participants beginning their first glovebox environment, they were familiarized with visual analog scales (VAS) [ratings of perceived exertion (RPE), and perceived recovery and status scales (PRSS)] (Borg, 1998; Laurent et al., 2011). These VAS provided an acute perception about the participant’s exertion and recovery levels. Careful attention was given to participants understanding the anchoring words associated with each VAS, and that they were to provide a numerical score based on their perception of their entire body.
After each participant completed all glovebox environments, if they had consented to answering the post-trial questionnaire, they answered the following questions: 1) “Do you believe the haptic gloves and the virtual environment are useful tools to help new glovebox workers build muscle memory and/or mental memory for performing unclassified glovebox tasks?” 2) “Do you believe you experienced any discomfort or frustration while participating in any of the three trials?” 3) “If yes, which trial and do you believe that frustration or discomfort negatively impacted your performance?” 4) “What unclassified tasks or scenarios do you believe would be good for new glovebox workers to perform in the haptic gloves virtual environment?” and 5) “Given this interview is anonymous, what testimonial for or against the use of the haptic gloves virtual environment as a training tool for new glovebox workers would you be willing to provide? Keep in mind the tasks or scenarios will be unclassified but geared toward glovebox tasks, skills, or knowledge.” Their anonymous answers to those questions were used to aid decision makers in determining if VR and haptics technologies would be useful tools for training new glovebox workers.
After becoming familiar with the VAS, an Institutional Review Board-approved data collection sheet was used to gather demographic data about participants. Participants were asked about their job title category; the number of years worked in that job category; what shift they were currently working; the number of hours they slept last night; and their experience performing tasks inside an XR environment.
The HaptX DK2 Gloves increase realism for VR users by allowing them to ‘feel’ the virtual objects at their figure tips. During the planning phase of this study, HaptX DK2 was the only glove we were able to test that provided force feedback (other than simple vibration), the ability to feel ‘weight’ due to the tethers on the back on the fingers. But that is not to say that advancement in this area hasn’t occurred in recent years.
To further describe the HaptX DK2 haptic feedback technology, 130 tactile microfluidic actuators were distributed across the fingers and palm of each glove (Stamer et al., 2020) to create this capability. A force-feedback exoskeleton, located on the back of each glove and powered by the same microfluidic technology, provides static resistance on the fingers to simulate the size, shape, and weight of virtual objects (Stamer et al., 2020). This force feedback technology allows for up to four pounds of force (approximately 18 N) per digit (Stamer et al., 2020). The magnetic finger-tracking provides six-degree-of-freedom tracking for each finger, and the sub-millimeter precision allows for natural hand motions (Stamer et al., 2020). By making use of virtual colliders (as known from rendering engines such as Unity3D and Unreal Engine), it is possible for the virtual hand model to prevent penetration of the interacting 3-D model.
It is important to note that in its standard state, the HaptX DK2 did not function as similar to working with physical objects as the researchers had hoped. For that, HaptX Inc. took extensive action to tune the system and virtual objects to optimize the experience participants would have when working in the virtual glovebox. This tuning and optimization went a long way in allowing participants to interact with the virtual objects without excessive errors (dropped objects) or objects coming apart due to collisions. Without this optimization, completing the virtual glovebox task with the haptic feedback gloves would not have been possible. Our future research aims to publish (in great detail) what went into the tuning of the glove; but that is beyond the scope of this study.
After participants were familiar with each VAS and allowed time to ask questions of the research team, they transitioned to their first glovebox environment. Glovebox environments were counter-balanced to prevent an ordering effect. Due to constraints of the study, only one trial was able to be performed which prevented participants from returning for follow-up testing. Therefore, participants who performed in the haptic glovebox environment last were invited to complete a second (identical) haptic glovebox environment after a 10-min recovery period to determine if there was a change in time to completion (TTC). Only those participants performing the haptic glovebox last were selected to prevent a potential crossover effect from having the participant train in a different glovebox environment between the first and second haptics glovebox environment. In doing this, roughly only 1/3 of the participants were tested; making the sample underpowered. Given this is preliminary data and only descriptive statistics were used (and not trying to demonstrate statistical significance), no major concerns were raised by the research about collecting data in this manner.
The handheld controller environment included HTC VIVE Steam VR brand controllers, HTC VIVE Pro 2 HMD, and the digital twin of the XR laboratory housing the virtual glovebox. The haptic feedback environment utilized the HaptX gloves, HTC VIVE HMD, and the same digital twin of the XR laboratory housing the virtual glovebox that was used in the hand-held environment. The physical training glovebox utilized a custom built MBRAUN (New Hampshire, USA) training glovebox; Honeywell brand gloves; a Kurt J. Lesker Company brand canister; generic busy board; McMaster-Carr brand torque wrench and socket; tackleboxes; HPFIX brand mat for sorting simulated material; McMaster-Carr brand vials; and small wooden rectangle-shaped blocks (colored either yellow or blue), which served as simulated material.
Within each of the glovebox environments (see Figures 2, 3), a single standardized glovebox task was completed. While the comparison is not a perfect correlation across each of the glovebox environments, the handheld controller and haptic feedback environments/tasks were constructed to operate as close to the physical training glovebox/task as possible (while acknowledging the technological limitations of each virtual glovebox environment/task). Additionally, a single-blind paradigm was used to prevent participants from knowing they were being timed during each glovebox environment, and that the primary outcome measures were the number of errors (times vial, vial lid, or simulated material was dropped) that occurred while sorting simulated material. While TTC and the number of errors performed by participants were collected, this was done strictly to inform decision makers on the time commitment differential and how many errors might occur during training if they decide to adopt these technologies versus training in a physical glovebox.
Each glovebox environment began with a researcher recording the glovebox environment name, glovebox environment number (this depended on the counter-balanced order assigned to each participant), and the beginning of the familiarization period. During the familiarization, participants utilized the busy board to perform each of the following tasks two times in chronological order: zipper, buckle, click switch, and latch. This task helped familiarize participants with how to handle items in the glovebox environment and what to expect from haptic feedback.
Immediately following the familiarization period, participant’s pre-environment RPE and PRSS were collected directly before the glovebox task began. To start the glovebox task, participants were instructed to “pick up the torque wrench (see Figures 4, 5).” Upon picking up the torque wrench, the time of day was noted on the data collection sheet to mark the glovebox task start time.
Each step of the glovebox task sequence was prerecorded and divided into single sub-task audio files to mimic the two-person, step-by-step process used at our location. Each subsequent audio file was played once the participant had completed the current task. Participants were offered a written copy of the glovebox task sequence in case they felt they were unable to complete the task using only the audio instructions.
After picking up the torque wrench, the participant began to loosen each of the eight bolts around the circumference of the cannister lid using a star/triangle pattern. Once all the bolts were removed, the lid was removed and placed on the floor of the glovebox. Then each of the three vials (see Figure 6) inside the canister were removed from the cannister and placed in the material sorting area. Vials containing a blue block were opened one-by-one and the blue block was removed and placed in the tacklebox to the right of the cannister. A single yellow block was retrieved from the left tacklebox and placed in the vial, the vial lid was replaced on the vial, and all three of the vials were returned one-by-one to the cannister. Then the cannister lid was replaced on top of the cannister and all bolts tightened to 7.4 N m (Nm) of torque in a triangle/start pattern.
Participants knew when the bolts were torqued to the proper level by the torque wrench “breaking.” Once the final bolt was sufficiently tightened, the torque wrench was laid down on the floor of the glovebox and the time of day was noted. At the end of each glovebox task, the difference between the end and start time was entered into the data sheet as the task TTC. When exiting the glovebox environment, participants were asked to provide their RPE and PRSS score.
After completing each glovebox environment, participants performed seated rest for 10 min without the use of their cellphones or caffeine. During recovery, participants were allowed to drink water, go to the restroom, and have casual conversation. This recovery is less than half of the time recommended for cognitive recovery as researched by Mark et al. (2015). However, given the short duration of the glovebox task and the low-intensity of the work, it was determined that a shorter recovery time would be less cognitively stressful and cause less frustration than having them sit for up to 80 min without a cellphone.
After the required blanket request for research participants went out to persons in the glovebox operator job category, 24 glovebox operators volunteered to participate in the study. With that, an a priori power analysis was conducted using GPower software that resulted in an alpha of 0.05, beta of 0.80, and an effect size of 0.27 for this study to be sufficiently powered. The authors felt confident in this power analysis given the effect size in GPower is automatically set at 0.25 and an effect size of 0.27 still accounts for a “small effect.” TTC, RPE, PRSS, and material handling errors (vial, vial lid, and simulated material combined) were reported as medians due to data not being normally distributed per the Shapiro-Wilk Test of Normality when testing the assumptions of the repeated-measures analysis of variance (ANOVA). Therefore, nonparametric statistics were used; where the Friedman’s test measured for main-effects and the Wilcoxon test was used for the post hoc analysis. The p-values for each comparison can be found in the boxplot figures. Statistical significance was assumed with a p < 0.05. When the p-value was smaller than 0.001, “p < 0.001” was used. All statistical calculations and figures were performed/created using Python 3.12.4.
Figure 7 depicts TTC (in minutes) by glovebox environment. Both virtual glovebox environments had double the median TTC compared to the physical glovebox environment, and provided confidence that no further statistical analysis was needed to indicate there was a difference in TTC between the handheld controller/haptic glovebox environments and the physical training glovebox. Also, in Figure 7, the handheld and haptic glovebox environment median TTC was virtually identical.
Given TTC of the first versus the second haptic glovebox environment trial is preliminary (n = 5), that data is not depicted. However, participants completed the haptic glovebox environment approximately 15% faster during the second trial versus the first trial.
Total errors during each of the glovebox environments are depicted in Figure 8. Given the median errors were two or less and each environment was only one unit apart, it can be reasonably determined that errors during virtual or physical glovebox environments were not much of an issue when it comes to successfully performing the glovebox task.
Figure 8. Total errors (vial, vial lid, and simulated material) for each of the glovebox environments.
RPEs across each of the three environments increased (pre-versus post-environment). But as depicted in Figure 9, each of the three glovebox environments had a similar median RPE rating—suggesting exertion was somewhat consistent across the glovebox environments. As seen in Figure 10, the PRSS ratings agreed with the RPEs median value in Figure 9 that as participants perceived their exertion level increased, they also perceived their recovery level to decrease (indicating more fatigue). Along with RPE, PRSS had similar median values across each of the glovebox environments, indicating each environment caused a somewhat similar amount of median fatigue.
The purpose of this study was to determine if haptic feedback gloves and a VR HMD would be a usable training tool for glovebox tasks compared to a physical glovebox. Participants performed significantly slower in both VEs compared to the physical glovebox environment. This is likely due to not being familiarized with the virtual environment and the haptic gloves. This condition will be similar in a real training scenario, as most glovebox operators do not have experience working in this type of virtual environment. Material handling errors across all three environments were almost nonexistent and practically identical for all three environments. RPE and PRSS variables showed good agreement across all environments. This indicated that when participants perceived that they were working harder that their level of perceived recovery was less suggesting increased fatigue. There is little published research on the use of VR and haptics for training glovebox operators in the nuclear industry. However, there has been some research on persons required to handle virtual objects, and the results of this study are similar in that there is a learning curve and a need to optimize the technology to human nature. A limitation of this work was discovered, as loosening and tightening the bolts on the cannister in the VE did not follow the same mechanics as required in the physical glovebox, which led to frustration and longer TTC. Future research will seek to further enhance the participant’s ability to work in the virtual glovebox with their normal mechanics.
With that, it was concluded that combining haptic feedback gloves with a VR HMD results in a useful tool for training glovebox tasks. During the glovebox environments, participants demonstrated and felt that their understanding and proficiently to perform the glovebox task increased. Additionally, participants felt that they experienced similar cognitive and physical stress during the virtual glovebox environments despite only feeling resistance in the fingers and hands versus resistance also being felt in the elbows, shoulders, and back with the physical glovebox. Therefore, haptic feedback gloves combined with a VR HMD are recommended for training high-hazard/low occurrence glovebox tasks that might be impractical or unsafe to train in a physical glovebox. With that said, given that VR haptics is a training and learning aid tool, it would have been nice to be able to retest the participants to see if a change in learning had occurred and the estimate learning retention. However due to constraints of this study that was not possible and is a limitation of this work. Future work aims to incorporate a test-retest paradigm and incorporate more technical equipment, such as a robotic arm, in conjunction with haptic feedback.
The raw data supporting the conclusions of this article will be made available by the authors, without undue reservation.
This study involved humans and was approved by United States Department of Energy’s Central Institutional Review Board. This study was conducted in accordance with the local legislation and institutional requirements. The participants provided their written informed consent to participate in this study.
JH: Conceptualization, Data curation, Formal Analysis, Funding acquisition, Investigation, Methodology, Project administration, Resources, Software, Supervision, Validation, Visualization, Writing–original draft, Writing–review and editing. AA: Conceptualization, Funding acquisition, Investigation, Project administration, Resources, Software, Supervision, Writing–original draft, Writing–review and editing. AS: Conceptualization, Funding acquisition, Project administration, Resources, Software, Supervision, Writing–original draft, Writing–review and editing. ML: Conceptualization, Project administration, Supervision, Writing–original draft, Writing–review and editing. CM: Software, Writing–original draft, Writing–review and editing. ME: Conceptualization, Software, Writing–original draft, Writing–review and editing. DV: Conceptualization, Software, Writing–original draft, Writing–review and editing. CM: Conceptualization, Software, Writing–original draft, Writing–review and editing. DM: Conceptualization, Software, Writing–original draft, Writing–review and editing. VO: Conceptualization, Resources, Software, Writing–original draft, Writing–review and editing. AD: Conceptualization, Resources, Software, Writing–original draft, Writing–review and editing. BC: Software, Writing–original draft, Writing–review and editing.
The author(s) declare that financial support was received for the research, authorship, and/or publication of this article. Funding for this project was provided by the Y-12 National Security Complex’s Plant Directed Research and Development program.
Authors VO, AD and BC were employed by HaptX Inc.
The remaining authors declare that the research was conducted in the absence of any commercial or financial relationships that could be construed as a potential conflict of interest.
The author(s) declare that no Generative AI was used in the creation of this manuscript.
All claims expressed in this article are solely those of the authors and do not necessarily represent those of their affiliated organizations, or those of the publisher, the editors and the reviewers. Any product that may be evaluated in this article, or claim that may be made by its manufacturer, is not guaranteed or endorsed by the publisher.
Abbas, J. R., O’Connor, A., Ganapathy, E., Isba, R., Payton, A., McGrath, B., et al. (2023). What is Virtual Reality? A healthcare-focused systematic review of definitions. J. health policy Technol. 12, 100741. doi:10.1016/j.hlpt.2023.100741
Abdullah, H. (2009). Major challenges to the effective management of human resource training and development activities. J. Int. Soc. Res. 2 (8).
Abiri, A., Pensa, J., Tao, A., Ma, J., Juo, Y. Y., Askari, S. J., et al. (2019). Multi-modal haptic feedback for grip force reduction in robotic surgery. Sci. Rep. 9 (1), 5016. doi:10.1038/s41598-019-40821-1
Achberger, A., Gebhardt, P., and Sedlmair, M. (2024). An exploratory expert-study for multi-type haptic feedback for automotive virtual reality tasks. IEEE Trans. Vis. Comput. Graph.
Bannat, A., Wallhoff, F., Rigoll, G., Friesdorf, F., Bubb, H., Stork, S., et al. (2008). “Towards optimal worker assistance: a framework for adaptive selection and presentation of assembly instructions,” in In Proceedings of the 1st international workshop on cognition for technical systems (Cotesys. Springer).
Berg, L. P., and Vance, J. M. (2017). Industry use of virtual reality in product design and manufacturing: a survey. Virtual Real. 21 (1), 1–17. doi:10.1007/s10055-016-0293-9
Bucchiarone, A. (2022). Gamification and virtual reality for digital twin learning and training: architecture and challenges. Virtual Real. and Intelligent Hardw. 4 (6), 471–486. doi:10.1016/j.vrih.2022.08.001
Butavicius, M. A., Vozzo, A., Braithwaite, H., and Galanis, G. (2012). Evaluation of a virtual reality parachute training simulator: assessing learning in an off-course augmented feedback training schedule. Int. J. Aviat. Psychol. 22 (3), 282–298. doi:10.1080/10508414.2012.691058
Choi, D. S., Ryu, S., Do, Y., Kyung, K. U., Jin, K., and Kim, S. Y. (2019). “Affordable drilling interface for haptic interaction in virtual environment,” in 2019 IEEE international conference on consumer electronics (ICCE) China, 11-13 Jan. 2019, (IEEE), 1–2.
Davis, S., Nesbitt, K., and Nalivaiko, E. (2014). “A systematic review of cybersickness,” in Proceedings of the 2014 conference on interactive entertainment, USA, December 2 - 3, 2014, 1–9.
Elmqaddem, N. (2019). Augmented reality and virtual reality in education. Myth or reality? Int. J. Emerg. Technol. Learn. 14 (3), 234. doi:10.3991/ijet.v14i03.9289
Fertleman, C., Aubugeau-Williams, P., Sher, C., Lim, A. N., Lumley, S., Delacroix, S., et al. (2018). A discussion of virtual reality as a new tool for training healthcare professionals. Front. Public Health 6 (44), 44–45. doi:10.3389/fpubh.2018.00044
Grajewski, D., and Hamrol, A. (2023). Low-cost VR system for interactive education of manual assembly procedure. Interact. Learn. Environ. 31 (1), 68–86. doi:10.1080/10494820.2020.1761836
Hwang, C., Feuchtner, T., Oakley, I., and Grønbæk, K. (2024). “Enriching industrial training experience in virtual reality with pseudo-haptics and vibrotactile stimulation,” in In proceedings of the 30th ACM symposium on virtual reality software and technology, Germany, October 9 - 11, 2024, 1–11.
Jensen, L., and Konradsen, F. (2018). A review of the use of virtual reality head-mounted displays in education and training. Educ. Inf. Technol. 23 (4), 1515–1529. doi:10.1007/s10639-017-9676-0
Jeon, C. (2015). The virtual flier: the link trainer, flight simulation, and pilot identity. Technol. Cult. 56 (1), 28–53. doi:10.1353/tech.2015.0017
Jones, D., Snider, C., Nassehi, A., Yon, J., and Hicks, B. (2020). Characterising the Digital Twin: a systematic literature review. CIRP J. Manuf. Sci. Technol. 29, 36–52. doi:10.1016/j.cirpj.2020.02.002
Juliano, J. M., and Liew, S. L. (2020). Transfer of motor skill between virtual reality viewed using a head-mounted display and conventional screen environments. J. neuroengineering rehabilitation 17, 48–13. doi:10.1186/s12984-020-00678-2
Laurent, C. M., Green, J. M., Bishop, P. A., Sjökvist, J., Schumacker, R. E., Richardson, M. T., et al. (2011). A practical approach to monitoring recovery: development of a perceived recovery status scale. J. Strength and Cond. Res. 25 (3), 620–628. doi:10.1519/jsc.0b013e3181c69ec6
Mark, G., Iqbal, S., Czerwinski, M., and Johns, P. (2015). “Focused, aroused, but so distractible: temporal perspectives on multitasking and communications,” in Proceedings of the 18th ACM conference on computer supported cooperative work and social computing, China, Oct 22, 2025, 903–916.
Muender, T., Bonfert, M., Reinschluessel, A. V., Malaka, R., and Döring, T. (2022). Haptic fidelity framework: defning the factors of realistic haptic feedback for virtual reality. In CHI conference on human factors in computing systems (CHI ’22), april 29-may 5, 2022, New Orleans, LA, USA. May 5, 2022, ACM, 17. doi:10.1145/3491102.3501953
Nazir, S., Kluge, A., and Manca, D. (2014). Can immersive virtual environments make the difference in training industrial operators. Proc. Hum. factors ergonomics Soc., 251–265. Europe.
Pakarinen, S., Laarni, J., Koskinen, H., Passi, T., Liinasuo, M., and Salonen, T. T. (2021). Virtual-reality based nuclear power plant field operator training.
Pavlov, D., Sosnovsky, I., Dimitrov, V., Melentyev, V., and Korzun, D. (2020). “Case study of using virtual and augmented reality in industrial system monitoring,” in 2020 26th conference of open innovations association (FRUCT) USA, 20-24 April 2020, (IEEE), 367–375.
Piroozfar, P., Essa, A., and Farr, E. R. (2017). “The application of Augmented Reality and Virtual Reality in the construction industry using wearable devices,” in In the 9th international conference on construction in the 21st century (CITC-9). China, May-23-2016 0-0).
Piviotti, M. (2021). Providing force and vibrotactile feedback with haptic devices for simulating industrial tools in immersive Virtual Reality. Dr. Diss. Politecnico Torino.
Radhakrishnan, U., Chinello, F., and Koumaditis, K. (2021). “Immersive virtual reality training: three cases from the Danish industry,” in 2021 IEEE conference on virtual reality and 3D user interfaces abstracts and workshops (VRW) USA, April 1 2021, (IEEE), 1–5.
Rauschnabel, P. A., Felix, R., Hinsch, C., Shahab, H., and Florian, A. (2022). What is XR? Towards a framework for augmented and virtual reality. Comput. Hum. Behav. 133, 107289. doi:10.1016/j.chb.2022.107289
Shahab, M. H., Ghazali, E., and Mohtar, M. (2021). The role of elaboration likelihood model in consumer behaviour research and its extension to new technologies: a review and future research agenda. Int. J. Consumer Stud. 45 (4), 664–689. doi:10.1111/ijcs.12658
Slater, M., Usoh, M., and Chrysanthou, Y. (1995). “The influence of dynamic shadows on presence in immersive virtual environments,” in Virtual environments’ 95: selected papers of the eurographics workshops in barcelona, Spain, 1993, and Monte Carlo, Monaco, 1995 Vienna, December 1-3, 2024, Springer, 8–21.
Stadler, S., Cornet, H., Theoto, T. N., and Frenkler, F. (2019). “A tool, not a toy: using virtual reality to evaluate the communication between autonomous vehicles and pedestrians,” in Augmented reality and virtual reality (Cham,Switzerland: Springer), 203–216.
Stamer, M., Michaels, J., and Tümler, J. (2020). “Investigating the benefits of haptic feedback during in-car interactions in virtual reality,” in HCI in mobility, transport, and automotive systems. Automated driving and in-vehicle experience design: second international conference, MobiTAS 2020, held as part of the 22nd HCI international conference, HCII 2020, Copenhagen, Denmark, july 19–24, 2020 (Springer International Publishing), 404–416.
Strandholt, P. L., Dogaru, O. A., Nilsson, N. C., Nordahl, R., and Serafin, S. (2020). “Knock on wood: combining redirected touching and physical props for tool-based interaction in virtual reality,” in In proceedings of the 2020 CHI conference on human factors in computing systems, USA, 25 Apr 2020, 1–13.
Tang, A., Owen, C., Biocca, F., and Mou, W. (2003). “Comparative effectiveness of augmented reality in object assembly,” in In Proceedings of the SIGCHI conference on Human factors in computing systems, USA, 11 May 2024, 73–80.
Tugal, H., Abe, F., Caliskanelli, I., Cryer, A., Hope, C., Kelly, R., et al. (2023). “The impact of a haptic digital twin in the nuclear industry and potential applications,” in 2023 IEEE international conference on advanced robotics and its social impacts (ARSO) Germany, 5-7 June 2023, (IEEE), 134–139.
Wang, D., Ohnishi, K., and Xu, W. (2019). Multimodal haptic display for virtual reality: a survey. IEEE Trans. Industrial Electron. 67 (1), 610–623. doi:10.1109/tie.2019.2920602
Winther, F., Ravindran, L., Svendsen, K. P., and Feuchtner, T. (2020). “Design and evaluation of a vr training simulation for pump maintenance based on a use case at grundfos,” in 2020 IEEE conference on virtual reality and 3D user interfaces (VR) China, 22-26 March 2020, (IEEE), 738–746.
Wolf, B., Kind, S., and Stark, R. (2020). Smart Hybrid Prototyping in manual automotive assembly validation. Procedia CIRP 88, 82–87. doi:10.1016/j.procir.2020.05.015
Keywords: haptic feedback, virtual reality, glovebox operations, digital twin, training evaluation
Citation: Hornsby JH, Arnwine A, Stowe A, Lin M, Mason C, Eakins M, Veller D, Mendoza C, Metcalf D, Oriaifo V, Dunar A and Crockett B (2025) VR haptics for glovebox operations training. Front. Virtual Real. 6:1560713. doi: 10.3389/frvir.2025.1560713
Received: 14 January 2025; Accepted: 20 February 2025;
Published: 19 March 2025.
Edited by:
Dioselin Gonzalez, Independent Researcher, Greater Seattle Area, WA, United StatesReviewed by:
Sam Van Damme, Ghent University, BelgiumCopyright © 2025 Hornsby, Arnwine, Stowe, Lin, Mason, Eakins, Veller, Mendoza, Metcalf, Oriaifo, Dunar and Crockett. This is an open-access article distributed under the terms of the Creative Commons Attribution License (CC BY). The use, distribution or reproduction in other forums is permitted, provided the original author(s) and the copyright owner(s) are credited and that the original publication in this journal is cited, in accordance with accepted academic practice. No use, distribution or reproduction is permitted which does not comply with these terms.
*Correspondence: Jared H. Hornsby, SmFyZWQuaG9ybnNieUB5MTJuc2MuZG9lLmdvdg==
Disclaimer: All claims expressed in this article are solely those of the authors and do not necessarily represent those of their affiliated organizations, or those of the publisher, the editors and the reviewers. Any product that may be evaluated in this article or claim that may be made by its manufacturer is not guaranteed or endorsed by the publisher.
Research integrity at Frontiers
Learn more about the work of our research integrity team to safeguard the quality of each article we publish.