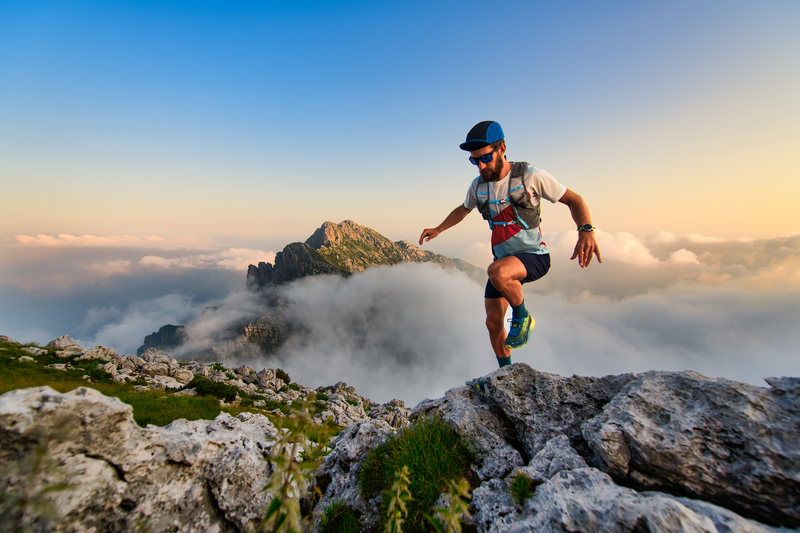
94% of researchers rate our articles as excellent or good
Learn more about the work of our research integrity team to safeguard the quality of each article we publish.
Find out more
ORIGINAL RESEARCH article
Front. Virtual Real.
Sec. Virtual Reality and Human Behaviour
Volume 6 - 2025 | doi: 10.3389/frvir.2025.1523022
The final, formatted version of the article will be published soon.
You have multiple emails registered with Frontiers:
Please enter your email address:
If you already have an account, please login
You don't have a Frontiers account ? You can register here
Dual-task (DT) ability is essential in sports, where athletes must perform motor and cognitive tasks simultaneously. Virtual reality (VR), with its enhanced performance and affordability, offers a valuable tool for training and assessing these abilities. This study aimed to develop VR scenarios to measure DT costs and compare DT ability between athletes from individual (IG) and team (TG) sports using a basketball-specific scenario. 29 participants completed two experiments to examine DT ability: a reaching and a dribbling task (DR). The reaching involved three tasks: walking a 4meter track, standing while reacting to popping balls, and a combination of both. Parameters such as step length, gait time, and reaction were measured. In DR, participants dribbled while reacting to a virtual opponent. Data on conduction time, errors, reaction time, gaze behavior (GB), and decision-making were analyzed. Significant differences were found between single and DT performances, with DT costs reaching up to 20% (p >.05). However, no significant differences were observed between IG and TG for selected parameters (F(1, 28) = 1.104, p = .410, partial η² = .380). Differences in GB and decision-making were noted and discussed. VR proved effective in assessing DT costs and providing insights into decision-making processes.
Keywords: virtual reality, peripheral distractors, head-mounted display, Basketball, dual-task
Received: 05 Nov 2024; Accepted: 12 Mar 2025.
Copyright: © 2025 Pastel, Schwadtke, Krahmer, Altrogge, Bürger, Heilmann and Witte. This is an open-access article distributed under the terms of the Creative Commons Attribution License (CC BY). The use, distribution or reproduction in other forums is permitted, provided the original author(s) or licensor are credited and that the original publication in this journal is cited, in accordance with accepted academic practice. No use, distribution or reproduction is permitted which does not comply with these terms.
* Correspondence:
Stefan Pastel, Otto von Guericke University Magdeburg, Magdeburg, Germany
Disclaimer: All claims expressed in this article are solely those of the authors and do not necessarily represent those of their affiliated organizations, or those of the publisher, the editors and the reviewers. Any product that may be evaluated in this article or claim that may be made by its manufacturer is not guaranteed or endorsed by the publisher.
Research integrity at Frontiers
Learn more about the work of our research integrity team to safeguard the quality of each article we publish.