- 1Institut national de la recherche scientifique, University of Quebec, Montreal, QC, Canada
- 2Digital Health Research, Centre for Digital Health and Wellbeing, Fondazione Bruno Kessler, Trento, Italy
- 3University Health Network, Toronto, ON, Canada
- 4Michael Garron Hospital, Toronto, ON, Canada
- 5School of Health Policy and Management, York University, Toronto, ON, Canada
- 6Department of Medicine, University of Toronto, Toronto, ON, Canada
Introduction: This study aimed to investigate the psychological and physiological impacts of audio-visual (AV) and audio-visual-olfactory (AVO) stimuli within an immersive virtual nature environment.
Methods: Twenty-two nurses from the mental health in-patient ward of a Canadian hospital participated in the study. Each participant chose one of the three available immersive scenarios (beach, lake, waterfall) to experiment with under the AV and AVO conditions. Psychological assessments were conducted via questionnaires to investigate relaxation levels and other measures of user experience. Although the AVO condition demonstrated the greatest improvement in relaxation relative to baseline, no significant differences were observed either between the conditions or across the scenarios. Physiological metrics were collected using an in-house instrumented Oculus Quest 2 virtual reality (VR) headset that allowed us to investigate brain activity via electroencephalography (EEG).
Results: Results show a significant difference between the two conditions in certain brain regions. Significant differences in neural patterns were also seen for the participants who reported improvements in relaxation, relative to those who did not report any improvements. For these latter-referenced participants, decreased relaxation resulted from the non-congruence of the presented smells with participant expectations. Furthermore, neuromarkers measured from the EEG, such as frontal alpha asymmetry (a measure of approach/withdrawal), engagement score, as well as valence and arousal indices suggested increased relaxation levels in the AVO condition.
Conclusion: Our results suggest that multisensory immersive experiences can impact both physiological and psychological outcomes, resulting in increased relaxation levels and enhanced sense engagement for certain scenes. The instrumented VR headset enabled the monitoring of user neural and behavioural patterns, thus allowing for new insights to be gained beyond those achievable with only questionnaires.
1 Introduction
In the context of our evolving society, stress and anxiety stand out as pronounced global challenges, accentuated remarkably by the repercussions of the COVID-19 pandemic. Reports reveal a rise in mental health disorders, with a 25.6% increase in anxiety and a 27.6% growth in depression since the pandemic’s onset (Pietrabissa and Simpson, 2020; Smith et al., 2020; Millroth and Frey, 2021; Santomauro et al., 2021; Hawes et al., 2021). The World Health Organization reports that, globally, over 280 million individuals suffer from depression, while another 301 million face challenges related to anxiety disorders. Alarmingly, approximately two-thirds of those experiencing these mental health conditions remain without treatment (WHO, 2022).
Mental disorders significantly affect individual wellbeing and the broader community. As a response, relaxation techniques have proven to be effective tools in addressing these challenges (Lolak et al., 2008). During the pandemic, a positive trend emerged where many turned to nature-based activities, such as nature walking and forest bathing, to alleviate anxiety and stress. Studies support the mental health benefits derived from such interactions with nature (Kotera et al., 2021; Giannico et al., 2021; Yao et al., 2021), demonstrating psychophysiological improvements without dependence on pharmacological solutions (Fu et al., 2022; Corazon et al., 2019). Nonetheless, these outdoor engagements are not universally accessible, excluding individuals with restricted mobility, those undergoing hospitalization, or even those living in urban settings without direct access to nature due to several barriers.
For example, physical barriers such as busy streets, railways, and fences impede direct access, while psychological barriers, such as social norms and hostile environments can discourage individuals from visiting and using urban green spaces (Biernacka and Kronenberg, 2018). Moreover, even when these spaces are accessible, their attractiveness may be compromised by issues such as poor maintenance, noise, congestion, and a lack of necessary amenities, further reducing their usability (Biernacka and Kronenberg, 2019). This has highlighted the need for alternative strategies that are more accessible and/or that may be done at the comfort of one’s home. Virtual reality (VR) has, as such, emerged as a promising solution.
Recent studies have shown that VR can be effective in reduce mental health issues by providing immersive experiences in simulated real-world environments (Bouchard et al., 2017; Maples-Keller et al., 2017). In addition, research has demonstrated an impact on psychological and physiological outcomes when interacting with VR-simulated natural environments. Psychological outcomes can include restoration of positive emotions, reduction of negative emotions, such as stress, and changes in cognitive fatigue. Physiological outcomes, in turn, can include changes in brain activity and autonomic nervous system function, as well as variations in heart rate variability (HRV), heart rate, and blood pressure [e.g. (Jo et al., 2019; Lee et al., 2022; Syed Abdullah et al., 2021)].
However, most current VR applications focus on visual and auditory stimulation. While sight and hearing dominate our sensory perceptions, research indicates that our memory, sense of presence, and relaxation responses are significantly influenced by olfactory cues (Herz, 1998; Chu and Downes, 2002; Munyan III et al., 2016; Igarashi et al., 2014a; Igarashi et al., 2014b). Many therapeutic benefits of nature can be attributed to its aromatic elements. Aromatherapy is a technique known for its stress-relieving properties (Redd et al., 1994; Diego et al., 1998), and its principles have been applied to virtual experiences, demonstrating greater relaxation and stress reduction when scents are integrated (Amores et al., 2018; Aiken and Berry, 2015; Pizzoli et al., 2021; Baus and Bouchard, 2010; Lopes et al., 2022).
Typically, researchers assess changes in stress/anxiety states using subjective tools, such as questionnaires, or objective tools, such as neurophysiological monitoring. For objective measurements, wearable devices are typically used offering real-time physiological monitoring in everyday settings. Recent findings, for example, have shown links between relaxation and changes in heart rate as well as breathing patterns while immersed in a virtual forest (Lopes et al., 2022). Similar findings have also been reported recently for multisensory VR experiences involving audio-visual-olfactory stimuli (De Jesus et al., 2022), including changes in heart rate variability (HRV) and cognitive functioning for post-traumatic stress disorder patients (De Jesus Junior et al., 2023). Electrodermal activity (EDA) has also demonstrated great potential in assessing mental health benefits during immersive experiences (Anderson et al., 2017).
One other neurophysiological modality that has emerged as a promising candidate is electroencephalography (EEG) [e.g. (Zhang et al., 2021)]. EEG is a non-invasive technique that records scalp electrical activity produced by neuronal interactions, effectively reflecting central nervous system activity and higher-level cognitive processes, including emotions (Ward, 2003; Li et al., 2018). These recordings allow the identification and quantification of changes in the brain’s activity, serving as valuable biomarkers that can be used to track the outcomes of different interventions.
Collecting neural data while a user wears a head-mounted display (HMD) can be challenging, due to the placement of electrodes, movement artifacts, and limited mobility due to wired connections. Recent innovations in sensor-embedded HMDs, however, offer a promising new avenue for monitoring user behavior and tracking intervention outcomes in highly ecological settings (Cassani et al., 2020; Moinnereau et al., 2022b; Bernal et al., 2022). Features derived from the EEG can provide new insights into the immersive experience (Moinnereau et al., 2023), as well as the impact of smells on human emotional responses (Abbasi et al., 2019; Wu et al., 2023), thus may be useful in quantifying the impact of multisensory VR on mental health. To date, very little is known about the impact of multisensory immersive nature stimuli, its benefits in helping manage stress, and the underlying neural underpinnings involved (Lopes and Falk, 2024). This work aims to take one step to fill these gaps.
In particular, here we conduct a study in which we monitor the changes in brain activity while participants are immersed in a VR nature scene under two conditions: audio-visual only (AV) and audio-visual-olfactory (AVO). An in-house instrumented HMD is developed to track EEG signals in real-time to extract several different biomarkers of valence, arousal, attention, engagement, mental workload, and withdrawal, as well as traditional EEG subband powers. Participants are comprised of nurses from a mental health in-patient ward. Nurses have been notably challenged by high-stress levels and demanding environments during the pandemic (Riedel et al., 2021; Spoorthy et al., 2020); demands which have persisted post-pandemic.
With this study, we seek to answer the following four research questions (RQs):
1. Does the multisensory experience result in greater relaxation restoration and improvements in user experience relative to traditional audio-visual immersive experiences?
2. Are there differences observed (subjectively) between the different nature scenes and smells?
3. What changes in EEG and its related biomarkers can be detectable within the AV and AVO conditions? And between the different nature scenes and smells?
4. If changes are detected, how do these changes manifest in the observed improvements or declines in relaxation levels?
2 Materials and methods
2.1 Participants
Twenty-seven nurses (16 females, mean age 40.5
2.2 Experimental design
The study took place in a nursing break room, where only two evaluators who conducted the experiment and the participant were present in the room. Figure 1 outlines the flow diagram of the experimental process, which alternated between questionnaires and VR exposure sessions. Participants selected one of three 360-degree natural scenes (i.e., beach, lake, or forest) to experience twice: once with only AV elements, and again with an added olfactory component (AVO condition). Given the limited availability of the nurses during their work shifts, it was necessary to optimize the duration of the experiment. Including setup, administration of questionnaires, and interviews, this constraint allowed for only one scenario per participant. To ensure that most of the fragrance was dispersed, a window was opened before and between conditions and then closed. To reduce biases, the ordering of the AV and AVO conditions was randomized among participants. Each VR session/condition lasted 2 min and 30 s, totaling 5 min of VR interaction. The primary objective was to assess the effect of VR on relaxation, as well as contrast the results between the two conditions. After concluding the VR exposure and removing the head-mounted display (HMD), participants completed a concluding questionnaire. This feedback is invaluable to refine the protocol for future applications targeting patients.
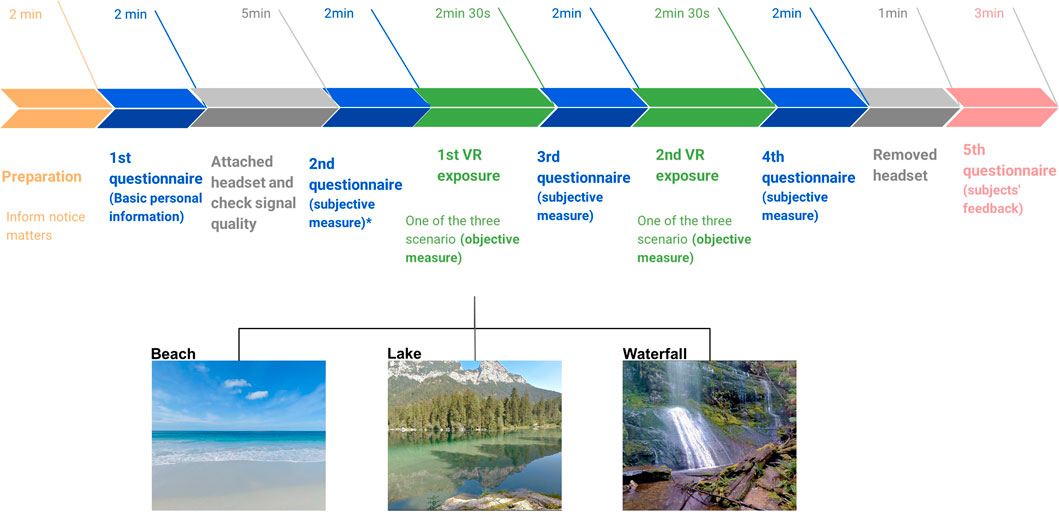
Figure 1. Flow diagram of experiment. The
2.3 Hardware
The experiment used an in-house instrumented Meta Oculus Quest 2 HMD. The headset comprises a single Fast-Switch LCD panel, providing a resolution of
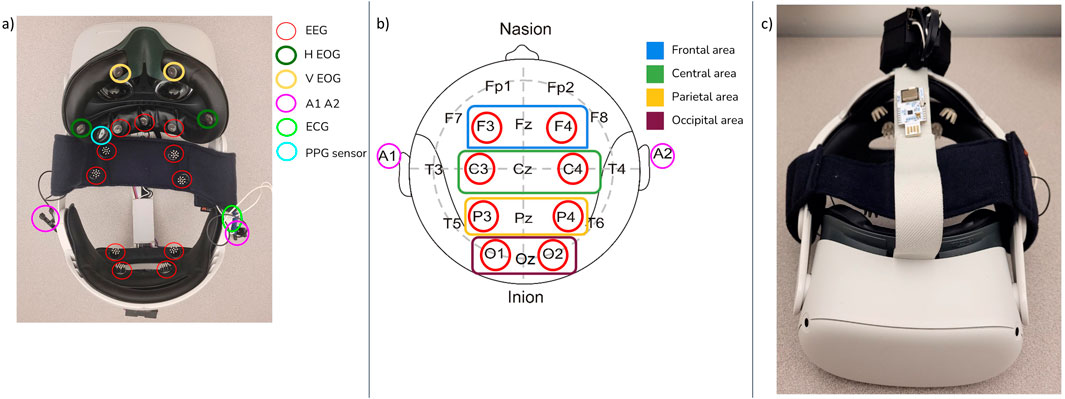
Figure 2. (A) Electrodes from the instrumented HMD and their respective modalities. (B) EEG electrode positions and associated scalp areas. (C) In-house developed instrumented head-mounted display.
OpenBCI Cyton and Daisy bioamplifiers (OpenBCI, United States) were used to acquire 16 ExG signals. The board was placed inside a 3D-printed case in attached to the top of the HMD (see Figure 2C). The instrumented headset allowed for the measurement of EEG data in real-time at a sample rate of 125 Hz. A total of 11 EEG channels were collected from the sensors placed on the foam and straps of the headset at the 10–20 locations Fp1, Fpz, Fp2, F3, F4, C3, C4, P3, P4, O1, and O2, as shown in Figure 2B. These locations have been shown in the literature to be related to stress/anxiety or relaxation (Ganguly and Singla, 2019; Davidson et al., 2003; Field et al., 1998; Kosunen et al., 2016; Parent et al., 2020).
To diffuse smells, an OVR ION2 scent diffusion device (OVR Technologies, United States) was attached to the HMD to provide an olfactory stimulus by dispersing aromas close to the user’s nose (see Figures 3–5). The employed scent kit contains a predefined cartridge with nine different scents. In this study we used the Nature cartridge which has as scents: flowers, earth dirt, forest, ocean breeze, grass, wood, beach, lavender, and citrus.
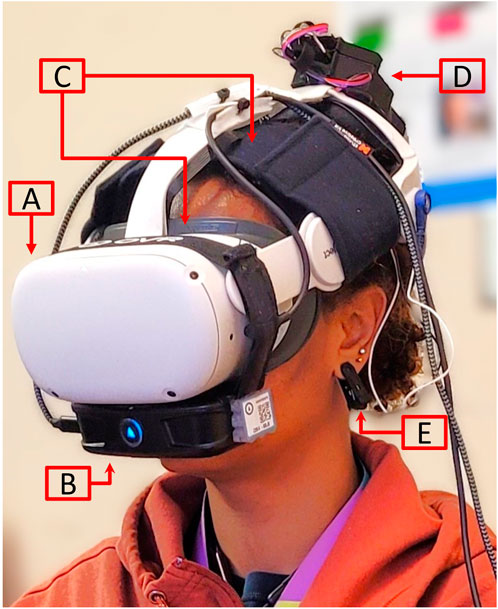
Figure 4. Equipment used including (A) VR headset,(B) ION 2 device, (C) ExG electrodes (not shown), (D) bioamplifier, and (E) reference electrodes.
2.4 Virtual environments
An application was developed, as depicted in Figure 6, where participants were offered a choice among three 360-degree nature videos. The first scene transports the viewer to the Alpine Lake-wide in Bavaria, Germany, where they find themselves in front of a serene lake with distant mountains and cabins. Trees and rocks complete the surrounding landscape. The second scene places the participant on the Arctic Beach in the Lofoten Islands, Norway, with fine white sand underfoot and a view of a turquoise sea, distant mountains, and a blue sky with few clouds. The third video immerses the viewer in a forest setting in Tasmania, Australia, with a prominent waterfall cascading over rocks and logs, surrounded by moss-covered rocks and trees whose leaves sway with the breeze. Accompanying these visuals, for the audio stimuli, original audio from the videos delivered authentic nature sounds such as waves, birdsong, and rustling leaves. All videos were sourced and licensed from the Atmosphaeres Library1.
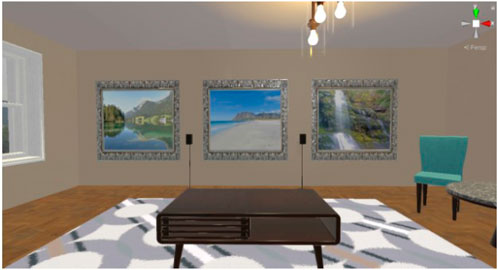
Figure 6. The developed application’s main scene where participants could choose the three nature scenes.
Using the ION2 scent diffuser, different aromas were used for each scene. For the lake scene, smells of flowers, earth dirt, forest, and grass were diffused. This combination was also utilized for the waterfall scene, but complemented by the scent of wood. For the beach scene, scents called beach and ocean breezes were used. Intensities were kept at a level of 1 out of 5, as this was a hospital setting. The timing of the scent dispersion was the same across the three videos to avoid any biases.
2.5 Questionnaires
Questionnaires were used to explore participants’ psychological state and their general experience and observations throughout the procedure. Questionnaires were presented five times, as shown in Figure 1. The first was to collect demographic data, including gender and age. The second asked participants to rate their current relaxation level on an eleven-point Likert scale, where scores ranged from 0 (very low) to 10 (very high). This was filled out once the participant chose their preferred VR environment. The third questionnaire was after the first VR exposure and had users rate not only their relaxation level, but also other so-called human influential factors (HIFs) (Moinnereau et al., 2022a), such as their senses of engagement and sense of immersion, if they had forgotten everyday concerns, lost track of time, and felt any motion sickness. These all relied on the 11-point Likert scale.
Before starting the second VR exposure, participants reassessed their new pre-exposure relaxation levels. After the second exposure, the same battery of questions was then asked about their experience. Upon completion of these questionnaires within the virtual environment, the HMD was removed and a final questionnaire was presented to the participants asking about their overall experience. Here, participants were asked for insights and suggestions to refine the protocol, especially for its potential application in future experiments involving individuals with mental health disorders. The specific questions asked are shown in Table 1.
2.6 Biosignal pre-processing and feature extraction
All neurophysiological data were synchronized with application events using the Lab Stream Layer (LSL). This paper focuses only on the EEG signals. To prepare the EEG signals for feature extraction, a “minimal” pre-processing pipeline was used, inspired by recent findings from Delorme (2023) suggesting that discriminative information may be removed from the EEG data via certain pre-processing algorithms. As this is the first experimentation with EEG and multisensory experiences with the instrumented headset, we chose to modify the signals the least possible. Thus, a notch filter was applied to remove the 60 Hz power line noise. Next, high-pass filtering with a zero-phase Butterworth filter set to a cutoff frequency of 0.25 Hz was used to eliminate low-frequency drifts. Finally, signals were segmented into 2-s epochs without overlap for feature extraction. It is important to emphasize that by using this minimal pre-processing pipeline, data from Fp1, Fp2, and Fpz remained very noisy. In our analyses, these electrodes are hence discarded, resulting in only eight EEG channels for analysis.
For features, normalized power spectral measures were computed for the five conventional EEG subbands, namely: delta (1–4 Hz), theta (4–8 Hz), alpha (8–12 Hz), beta (12–30 Hz), and gamma (30–45 Hz), thus resulting on 40 power measures (8 channels
2.7 Regression
In this study, linear regression analysis was used to assess changes in the above-listed markers throughout the AV and AVO sessions to check if positive, negative, or null trends could be observed. The analysis was done using MATLAB’s (R2021A, MathWorks, Natick, Massachusetts, United States) fitlm function. The goal of this analysis is to explore the impact of the AV and AVO experiences on the measured neuromarkers, compare the differences seen between the two conditions, and potentially find the optimal markers to automatically track relaxation states during VR immersion. To test the significance of the trends, a Wald test was performed.
3 Results
For the analysis of the results, data from five participants had to be removed; three of them due to problems with the signal acquisition, and two of them asked to stop the experiment due to sensitivity to the smells. Consequently, the remaining participants were distributed across the following scenarios: six chose the beach, seven opted for the lake, and nine selected the waterfall. This section shows the obtained results for the subjective and objective outcome measures.
3.1 Subjective measures results
Figure 7 depicts the average ratings reported for the relaxation level. In the Figure 7A shows the rating before (called baseline in the figure) and after the VR interventions for the AV and AVO conditions, in this graph all participants were used, without distinguishing the scenarios. In Figure 7B we separate the participants by scenario, so we can see the influence of different environment and odors. After performing a Shapiro-Wilk test to check the normality of the distributions, the Wilcoxon signed-rank test was used to compare the significance of the differences. As can be seen, using all participants, we have a significant difference between baseline and post-intervention, with
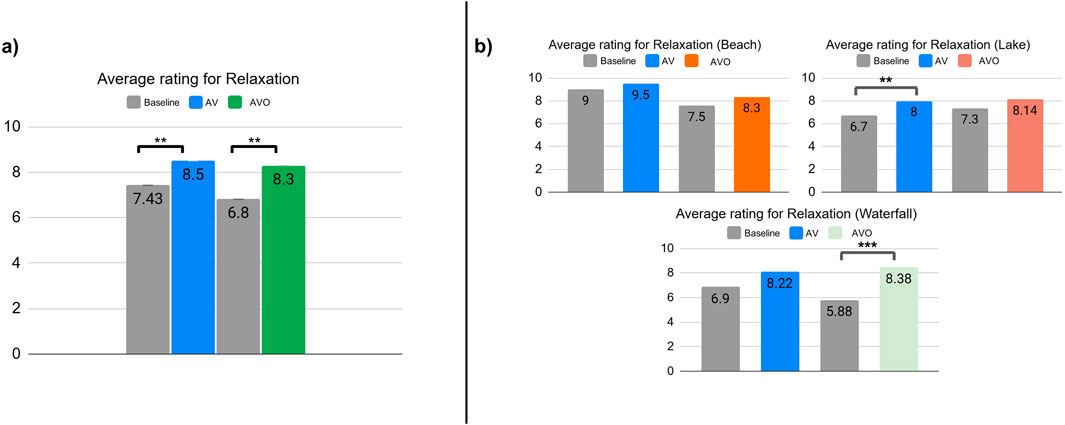
Figure 7. Results average of relaxation ratings. (A) Average rating of all subjects. (B) Average rating per scenario. Beach scenario: 6 participants. Lake scenario: 7 participants. Waterfall scenario: 9. ** - p
For the separate scenarios analysis, the baseline and AV condition shows significant difference (
Furthermore, the Wilcoxon signed-rank test was performed to investigate the significance of the differences reported in the HIF ratings. The following values were found:
• Senses engagement: AV (M = 8.25 SD = 1.66); AVO (M = 8.32 SD = 1.47),
• Sense of immersion: AV (M = 8 SD = 1.85); AVO (M = 8 SD = 1.47),
• Forgot everyday concerns: AV (M = 8.27 SD = 2.33); AVO (M = 7.82 SD = 2.36),
• Lost track of time: AV (M = 7.6 SD = 2.9); AVO (M = 7.4 SD = 2.4), and
• Motion sickness: AV (M = 0 SD = 0); AVO (M = 0.32 SD = 0.95).
None of these differences were found to be significant.
Table 3 further lists the reported HIF ratings separated by the scenarios for each condition. Using a Kruskal-Wallis test, we can see in that only the senses engagement for the AV condition shows a difference significative between scenarios (
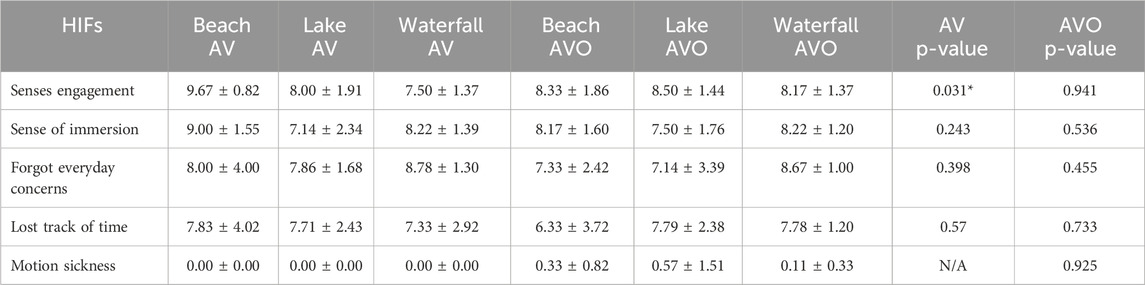
Table 3. Results of comparative analysis for the AV and AVO conditions. The reported p-values are from a Kruskal-Wallis test to measure significance between the three different scenarios for each AV and AVO condition.
3.2 Objective measures results
Table 4 shows the result of a linear regression analysis for the conditions AV and AVO for the five different EEG subbands, per electrode. In this analysis, the slope direction is reported to show if EEG subband powers are increasing or decreasing (significantly) during the exposure. One, two, or three asterisks are used to indicate the significance levels, i.e., 95%, 99%, and 99.9%, respectively. As we used a minimal pre-processing pipeline, we had to discard three electrodes (Fp1, Fpz, and Fp2) due to the large amounts of artifacts. As can be seen from the Table, most electrodes presented statistically significant drops over the exposure for both AV and AVO conditions. This can be interpreted differently for each band and brain area. This will be discussed in further detail in Section 4.
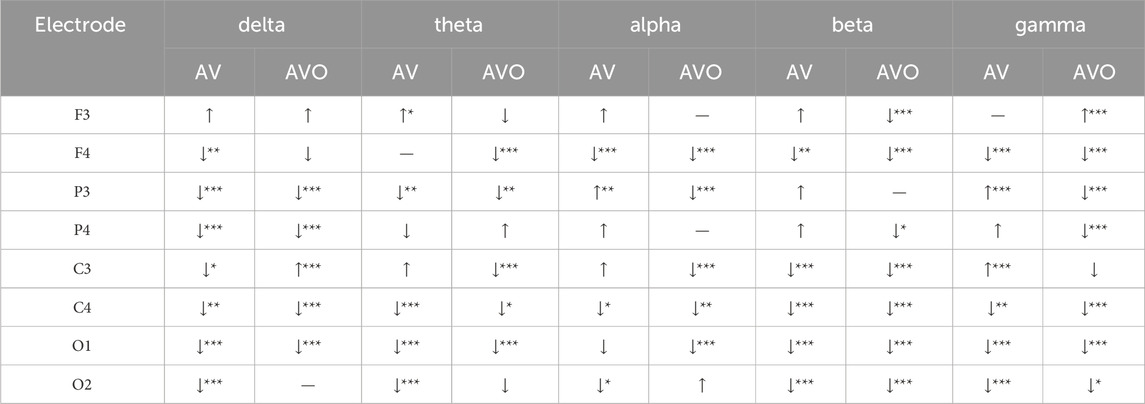
Table 4. Linear regression slope direction and significance. * - p
In particular, the delta band presented significant negative slopes across both conditions, except for the central left region in the AVO condition. Overall, more significant drops were observed in the AVO condition. In turn, an increase in frontal (F3) theta power was only seen in the AV condition, while most other regions showed a significant decrease. The beta band shows decreased activity in many channels across both conditions, while alpha power showed decreasing trends for most electrodes in the AVO condition, but only on half of the channels in the AV condition. Lastly, although most of the slopes presented in the gamma band were negative, for some regions, such as the left parietal and central regions, a strong positive significance was seen for the AV condition, while a negative slope was seen with the AVO condition.
As an additional step to help answer RQ#3, a Mann-Whitney U test with Holm-Bonferroni correction was performed between the AV and AVO conditions across each EEG channel. Significant differences were observed in channels F3, C3, P4, and O1 for delta; F4 and P4 for theta; C4 and F4 for alpha; C4, F4, C3, and O2 for beta; and F3, F4, C3, C4, and O2 for gamma.
To further help investigate RQ#3, a Kruskal-Wallis test with Holm-Bonferroni correction was employed to observe differences across EEG channels under the three scenarios within the AV and AVO conditions. In the AV condition, statistically significant differences were noted across the following channels: C4, F4, O2, P3, and P4 for delta; C4, F3, O2, P3, and P4 for theta; C3, C4, and P4 for alpha; C4, F4, O2, and P4 for beta; and C4, F3, F4, O1, O2, P4 for gamma. In the AVO condition, significant differences were detected in channels C3, C4, F3, F4, and O2 for delta; C4, O2, and P4 for theta; C3, C4, F3, and O2 for alpha; C4, O1, P3, and P4 for beta; and C3, C4, F3, F4, O1, and P4 for gamma.
Next, we explore the changes seen with the six metrics tested as proxies for mental/cognitive/affective states, as listed in Table 2. Mann-Whitney U test analysis between the AV and AVO was performed. The attention feature shows a significant difference (
To better visualize the changes in neural patterns, a topographical plot visualization is used. In this analysis, we group the subjects into two groups: those that reported improvements in relaxation with VR exposure and those that did not. Figure 8 shows the alpha band topoplots for the “negative” group (i.e., did not show improvements in relaxation) on the left and the “positive” group (i.e., those that showed increased relaxation) on the right. Top plots are for the AV condition and the bottom plots are for the AVO. As can be seen, for the AVO conditions, the asymmetry in alpha bands inverts from left to right hemispheric dominance. This is corroborated by the FAA metric, which showed a significant difference with (M = −0.125, SD = 1.62) for the ‘negative’ group and (M = 0.24, SD = 1.77) for the ‘positive’ group. This result will be discussed in further detail in Section 4.
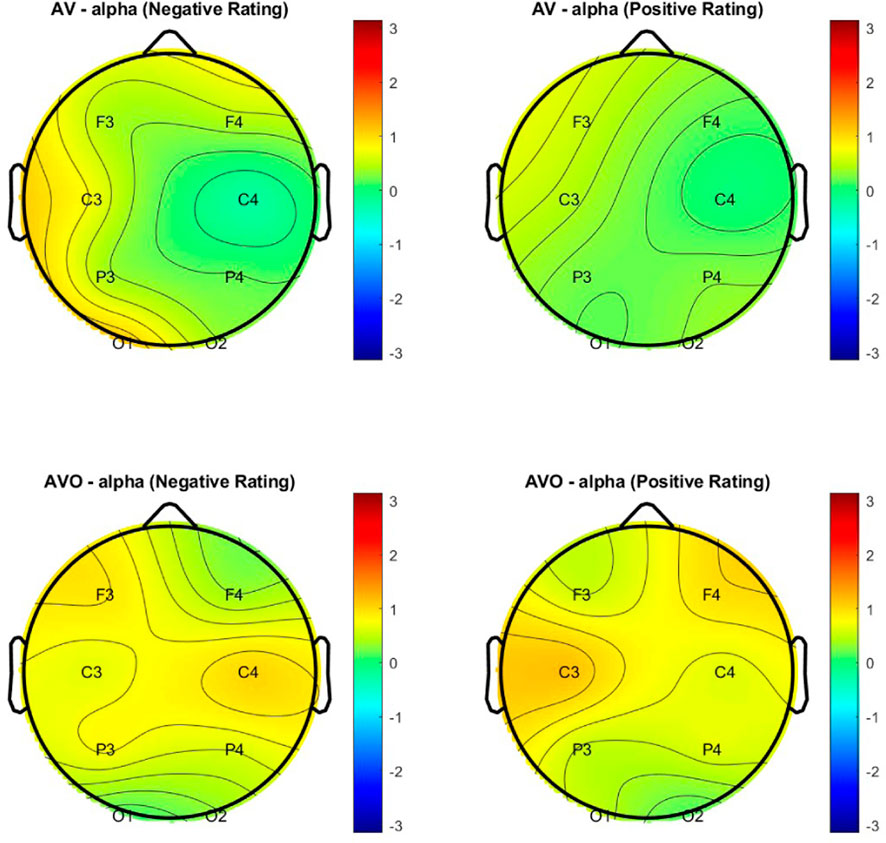
Figure 8. Topoplot of alpha band distribution. Positive and negative relaxation ratings for AV and AVO sessions.
4 Discussion
4.1 Subjective measures analysis
4.1.1 Answering RQ1: impact of multisensory experiences on relaxation and user experience
As showed in Section 3.1, in both conditions, all participants felt significantly more relaxed after the AV and AVO exposures, despite the differences between the AV and AVO conditions not being significant. Similar results were observed with the HIF ratings. Notwithstanding, when analyzing the results per scenario, some interesting differences could be observed. For example, most participants who experienced the beach scenario reported lower relaxation ratings in the AVO condition, while those who experienced the other two scenarios reported higher relaxation ratings in the AVO condition. These results show the power of multisensory experiences in influencing our levels of relaxation and other experiential factors, as further discussed next.
4.1.2 Answering RQ2: impact of scenes and smells
As mentioned above, the beach scene was the only one in which the AVO condition resulted in poorer relaxation levels compared to the AV setting. Similarly, for the HIF ratings in the lake and waterfall scenarios, the AVO condition resulted in greater sense of engagement, sense of immersion, and of participants losing track of time, as compared to the AV condition.
Further investigation into the unexpected behavior seen in the beach scene suggests this could be related to the idiosyncratic interpretation of smells and non-congruence with expectations. For example, participants mentioned they were expecting a tropical beach and related smells (one mentioned the smell of sunblock lotion), while the actual presented video and smells represented that of a more Arctic beach. Candid feedback from the participants included: “The smell did not agree with the reality of their beach scene. It should be more salty and sandy not very sweet as this one was.”
In turn, for the waterfall and lake scenarios, the smells more closely matched what was expected. Candid feedback from some of the users in these two scenarios included: (1) “In the first section (without the smell) it was pretty. I could only hear the waterfall. However, in the second one, the smell made the experience more real. It took me back to when I was there (in a waterfall). All my senses were engaged.”, (2) “I know it is virtual but I did feel I was sitting in front of the waterfall”, and (3) “smells were a good addition to the virtual experience.”
4.2 Objective measures analysis
4.2.1 Answering RQ3: changes in EEG signals
To help answer RQ#3, we first start with the EEG subband power comparisons. As reported in Table 4, the delta band presented a negative slope in most electrodes. Typically, the delta band is associated with meditation or sleep in resting states. In fact, Teplan et al. (2014) demonstrated that a reduction in delta power correlated with successful relaxation. Other studies have also shown that a decrease in delta could be related to increased motivation (Cowley and Ravaja, 2014).
Moreover, changes in theta band powers have been associated with attention or cognitive load, with a reduction in theta power indicating a reduced level of attention (Sowndhararajan and Kim, 2016). The work by Cahn et al. (2010) showed an increase in frontal theta power during meditation, as well as a significant increase in parieto-occipital gamma power. In the present study, only frontal (F3) theta power showed an increase, and for most other regions results showed a significant decrease. Decreases in theta powers have also been linked with positive valence or pleasantness (Reuderink et al., 2013); this could explain the changes observed herein.
The fact that we are relying on VR in our study could also have an impact on EEG beta band activity. In Tarrant et al. (2018), for example, a VR natural environment was used for mindfulness practice and decreased beta activity was observed. Beta activity is linked to active thinking and alertness. In our obtained results, the decreases in beta activity across many channels in both sessions suggest a general decrease in active cognitive processing or a shift towards a more relaxed state. On the other hand, increases in alpha powers have been linked to increasing emotional arousal (Reuderink et al., 2013). Comparing the results from the alpha and theta changes, one can observe a decrease in arousal and an increase in pleasantness, thus moving towards a more calm and relaxed emotional state.
Gamma activity has been associated with high-level cognitive (Fitzgibbon et al., 2004) processing and sensory integration, thus corroborating the fact that the majority of the channels showed significant changes. In fact, the work in Zhang et al. (2019) showed the importance of gamma activity for odor recognition, indicating a strong relationship between olfactory processing and gamma band activity in the brain. This may explain the more significant changes seen in gamma band activity with the AVO condition relative to the AV one.
Moreover, as reported in Section 3.2, the attention feature showed a significant difference between the AV and AVO conditions, suggesting changes in visual attention once additional sensory cues were provided. The FAA metric also had a significant difference between both conditions. The negative mean in the AV session and positive mean in the AVO session indicate a shift from a withdrawal tendency to a greater approach tendency (Goodman et al., 2013) with the AVO experience.
The engagement index also showed a significant difference between the two conditions, with a negative mean for AV and positive for AVO, thus corroborating the increased sense of immersion and senses engagement reported by the participants. This was also corroborated by candid feedback from the participants, which included: (1) “More senses were activated making the experience more engaging and increasing the sense of presence,” (2) “I preferred the multisensory experience because I could engage with the environment better. I think the smell is a smooth smell and it makes me relax better.” and (3) “It is great. I have not tried VR before, with smells being more engaging and more relaxing. I wanted to stay there longer.”
4.2.2 Answering RQ4: neural pattern changes associated with variations in relaxation levels
When analyzing the results shown in Figure 8, it can be observed for the AVO condition that the shift in the alpha band asymmetry occurred between the negative and positive groups, corroborating the withdrawal/approach behaviors that have been widely reported in the literature (more on this below). This interhemispheric asymmetry is not as pronounced in the AV-only condition, suggesting some differences in neural patterns between AV and AVO conditions.
As mentioned previously, the withdrawal behavior is likely due to the non-congruence of the expected smells, especially with the beach scenario. This helps answer RQ#4 and highlights the importance of customizing experiences on a per-user basis to minimize such issues. In addition, our results corroborate those by Park et al. (2011), where positive emotional information was reflected in a decrease in alpha power of the left frontal hemisphere, while the processing of negative emotional information was reflected by the decrease of alpha power over the right frontal hemisphere (Reuderink et al., 2013).
Overall, these findings suggest that indeed there are neural differences between the AV and AVO conditions and several traditional metrics are sensitive enough to detect these changes. It is hoped that these insights can lead to improved interventions, such as neurofeedback nature immersion tools, that can help patients and nurses better manage their stress levels.
4.3 Limitations and future research directions
This study is not exempt from limitations. First, the limited number of participants decreases the statistical power of the analyses, thus the findings reported herein are to be considered preliminary. As the study was conducted in a hospital setting with nurses, their busy schedules limited the number of participants that could be recruited during the short period of time we were given access to the premises. The busy schedules of the participants also limited the amount of time in which we could collect data, thus limiting us to a total of two conditions (AV and AVO) of 2.5 min each.
The limited amount of time available also limited us to choose questionnaires based on single items and using the Likert scale, as opposed to standardized tests, which commonly rely on multiple questions that are then aggregated into a final rating, thus providing a more comprehensive assessment [e.g., see (Moinnereau et al., 2022a)]. This may have been the reason that many of the subjective responses did not show a significant difference between the two conditions. Future work should explore the use of standardized questionnaires for comparisons. This time limitation also required us to pre-select smell intensity and types. This lack of customization meant that some subjects dropped out of the study because of sensitivity to the smells, while others did not appreciate the non-congruence of the presented beach smell with what was expected. This caused biases in both the subjective and objective ratings. Future work should explore per-user scenarios and scent-enabling customization to reduce these unwanted effects.
Lastly, in our analysis, we decided to pursue a minimal pre-processing pipeline for the EEG signals, motivated by recent findings from Delorme (2023). This resulted in data from three channels, namely Fp1, Fp2, and Fz being very noisy, and thus being discarded from our analyses. Future work can explore the impact of different EEG enhancement algorithms and the potential inclusion of these channels in the overall analyses.
5 Conclusion
This pilot study has explored the impact of multisensory nature immersion as a tool to improve the relaxation of nurses in an in-patient hospital in Canada. Via an instrumented VR headset, we tracked the EEG signals of 22 nurses while they were immersed in one of the natural scenes (beach, waterfall and lake), chosen by them, under two conditions, namely audio-visual (AV) and audio-visual-olfactory (AVO). Comparing the two conditions, without distinguishing between scenes, the AV condition resulted in higher levels of relaxation. In turn, when considering specific scenarios (i.e., lake and waterfall), the AVO condition showed greater improvements in relaxation levels, as well as improvements across several other user experience ratings, such as sense of immersion and engagement levels. These findings suggest that sensory congruence may play a role in enhancing the user experience when the olfactory sense is stimulated.
Moreover, using the instrumented headset, significant changes were seen in the neural patterns between the two conditions, with the AVO condition showing increased behavioral approach (via a frontal alpha asymmetry measure), increased engagement, as well as several changes in EEG subband powers, suggesting greater relaxation levels, increased valence, and decreased arousal, just to name a few. The importance of smell congruence became very clear from both the subjective and objective analyses, suggesting that customization may be crucial for multisensory immersive experiences.
Overall, despite the limitations of the study (i.e., limited number of subjects, limited experiment duration, limited customization of smells) the in-house instrumented headset provided invaluable insights that were not available with just the questionnaires. The potential to monitor, in real-time, participant mental, affective, and cognitive states, as well as relaxation and user experience levels may allow for next-generation multisensory, adaptive immersive biofeedback applications that are optimized to each user’s experience. Given that olfaction is a very subjective sensory input, such monitoring may allow for real-time detection of scent incongruence, which we have seen here can substantially (negatively) effect the overall experience.
Data availability statement
The datasets presented in this article are not readily available because the access to records and data is limited to authorized persons, including the Principal Investigator, co-Principal Investigators, and members of the research team. Requests to access the datasets should be directed to Christopher Smith, Y2hyaXN0b3BoZXIuc21pdGhAdGVobi5jYQ==.
Ethics statement
The studies involving humans were approved by Michael Garron Hospital Ethics Committees and, Institut national de la recherche scientifique Ethics Committees. The studies were conducted in accordance with the local legislation and institutional requirements. The participants provided their written informed consent to participate in this study. Written informed consent was obtained from the individual(s) for the publication of any potentially identifiable images or data included in this article.
Author contributions
ML: Data curation, Formal Analysis, Investigation, Methodology, Project administration, Resources, Software, Supervision, Validation, Visualization, Writing–original draft, Writing–review and editing, Conceptualization. BD: Data curation, Methodology, Writing–review and editing. OR: Resources, Validation, Visualization, Writing–review and editing. SP: Methodology, Writing–review and editing. LA: Funding acquisition, Methodology, Supervision, Writing–review and editing. CS: Funding acquisition, Methodology, Supervision, Writing–review and editing. TF: Conceptualization, Funding acquisition, Methodology, Supervision, Validation, Visualization, Writing–review and editing.
Funding
The author(s) declare that financial support was received for the research, authorship, and/or publication of this article. The authors acknowledge funding from the Natural Sciences and Engineering Council (NSERC) of Canada (RGPIN-2021-03246) and TD Community Health Solutions (2022-06-RIC003). The funder was not involved in the study design, collection, analysis, interpretation of data, the writing of this article, or the decision to submit it for publication.
Acknowledgments
We would like to thank the partnership with Michael Garron Hospital and the nurses who took time out of their super-busy schedules to participate in the experiment. We would also like to thank Reza A. Gougeh for his help with the Unity application used in this study.
Conflict of interest
The authors declare that the research was conducted in the absence of any commercial or financial relationships that could be construed as a potential conflict of interest.
The author(s) declared that they were an editorial board member of Frontiers, at the time of submission. This had no impact on the peer review process and the final decision.
Publisher’s note
All claims expressed in this article are solely those of the authors and do not necessarily represent those of their affiliated organizations, or those of the publisher, the editors and the reviewers. Any product that may be evaluated in this article, or claim that may be made by its manufacturer, is not guaranteed or endorsed by the publisher.
Footnotes
1https://www.atmosphaeres.com/
References
Abbasi, N. I., Bose, R., Bezerianos, A., Thakor, N. V., and Dragomir, A. (2019). “Eeg-based classification of olfactory response to pleasant stimuli,” in 2019 41st annual international conference of the IEEE engineering in medicine and biology society (EMBC) (IEEE), 5160–5163.
Aiken, M. P., and Berry, M. J. (2015). Posttraumatic stress disorder: possibilities for olfaction and virtual reality exposure therapy. Virtual Real. 19, 95–109. doi:10.1007/s10055-015-0260-x
AlShorman, O., Masadeh, M., Heyat, M. B. B., Akhtar, F., Almahasneh, H., Ashraf, G. M., et al. (2022). Frontal lobe real-time eeg analysis using machine learning techniques for mental stress detection. J. Integr. Neurosci. 21, 20. doi:10.31083/j.jin2101020
Amores, J., Richer, R., Zhao, N., Maes, P., and Eskofier, B. M. (2018). “Promoting relaxation using virtual reality, olfactory interfaces and wearable eeg,” in 2018 IEEE 15th international conference on wearable and implantable body sensor networks (BSN) (IEEE), 98–101.
Anderson, A. P., Mayer, M. D., Fellows, A. M., Cowan, D. R., Hegel, M. T., and Buckey, J. C. (2017). Relaxation with immersive natural scenes presented using virtual reality. Aerosp. Med. Hum. Perform. 88, 520–526. doi:10.3357/amhp.4747.2017
Baus, O., and Bouchard, S. (2010). The sense of olfaction: its characteristics and its possible applications in virtual environments. J. CyberTherapy Rehab 3, 31–50.
Bernal, G., Hidalgo, N., Russomanno, C., and Maes, P. (2022). “Galea: a physiological sensing system for behavioral research in virtual environments,” in 2022 IEEE conference on virtual reality and 3D user interfaces (VR) (IEEE), 66–76.
Biernacka, M., and Kronenberg, J. (2018). Classification of institutional barriers affecting the availability, accessibility and attractiveness of urban green spaces. Urban For. and urban Green. 36, 22–33. doi:10.1016/j.ufug.2018.09.007
Biernacka, M., and Kronenberg, J. (2019). Urban green space availability, accessibility and attractiveness, and the delivery of ecosystem services. Cities Environ. (CATE) 12, 5.
Bouchard, S., Dumoulin, S., Robillard, G., Guitard, T., Klinger, E., Forget, H., et al. (2017). Virtual reality compared with in vivo exposure in the treatment of social anxiety disorder: a three-arm randomised controlled trial. Br. J. Psychiatry 210, 276–283. doi:10.1192/bjp.bp.116.184234
Cahn, B. R., Delorme, A., and Polich, J. (2010). Occipital gamma activation during vipassana meditation. Cogn. Process. 11, 39–56. doi:10.1007/s10339-009-0352-1
Cassani, R., Moinnereau, M.-A., Ivanescu, L., Rosanne, O., and Falk, T. H. (2020). Neural interface instrumented virtual reality headsets: toward next-generation immersive applications. IEEE Syst. Man, Cybern. Mag. 6, 20–28. doi:10.1109/msmc.2019.2953627
Chu, S., and Downes, J. J. (2002). Proust nose best: odors are better cues of autobiographical memory. Mem. and cognition 30, 511–518. doi:10.3758/bf03194952
Corazon, S. S., Sidenius, U., Poulsen, D. V., Gramkow, M. C., and Stigsdotter, U. K. (2019). Psycho-physiological stress recovery in outdoor nature-based interventions: a systematic review of the past eight years of research. Int. J. Environ. Res. Public Health 16, 1711. doi:10.3390/ijerph16101711
Cowley, B., and Ravaja, N. (2014). Learning in balance: using oscillatory eeg biomarkers of attention, motivation and vigilance to interpret game-based learning. Cogent Educ. 1, 962236. doi:10.1080/2331186x.2014.962236
Davidson, R. J., Kabat-Zinn, J., Schumacher, J., Rosenkranz, M., Muller, D., Santorelli, S. F., et al. (2003). Alterations in brain and immune function produced by mindfulness meditation. Psychosom. Med. 65, 564–570. doi:10.1097/01.psy.0000077505.67574.e3
De Jesus, B., Lopes, M., Moinnereau, M.-A., Gougeh, R. A., Rosanne, O. M., Schubert, W., et al. (2022). “Quantifying multisensory immersive experiences using wearables: is (stimulating) more (senses) always merrier?,” in Proceedings of the 2nd Workshop on multisensory experiences-SensoryX’22 (SBC).
De Jesus Junior, B. J., Perreault, L., Lopes, M., Roberge, M.-C., Oliveira, A. A. D., and Falk, T. H. (2023). Using multisensory virtual reality nature immersion as a therapeutic modality for improving hrv and cognitive functions in post-traumatic stress disorder: a pilot-study. Front. Virtual Real. 4, 1261093. doi:10.3389/frvir.2023.1261093
Diego, M. A., Jones, N. A., Field, T., Hernandez-Reif, M., Schanberg, S., Kuhn, C., et al. (1998). Aromatherapy positively affects mood, eeg patterns of alertness and math computations. Int. J. Neurosci. 96, 217–224. doi:10.3109/00207459808986469
Field, T., Martinez, A., Nawrocki, T., Pickens, J., Fox, N. A., and Schanberg, S. (1998). Music shifts frontal eeg in depressed adolescents. Adolescence 33, 109–116.
Fitzgibbon, S., Pope, K., Mackenzie, L., Clark, C., and Willoughby, J. (2004). Cognitive tasks augment gamma eeg power. Clin. Neurophysiol. 115, 1802–1809. doi:10.1016/j.clinph.2004.03.009
Fu, D., Serra, N. I., Mansion, H., Mansion, E. T., and Blain-Moraes, S. (2022). Assessing the effects of nature on physiological states using wearable technologies. Int. J. Environ. Res. Public Health 19, 1231. doi:10.3390/ijerph19031231
Ganguly, S., and Singla, R. (2019). “Electrode channel selection for emotion recognition based on eeg signal,” in 2019 IEEE 5th international conference for convergence in Technology (I2CT) (IEEE), 1–4.
Giannico, V., Spano, G., Elia, M., D’Este, M., Sanesi, G., and Lafortezza, R. (2021). Green spaces, quality of life, and citizen perception in european cities. Environ. Res. 196, 110922. doi:10.1016/j.envres.2021.110922
Goodman, R. N., Rietschel, J. C., Lo, L.-C., Costanzo, M. E., and Hatfield, B. D. (2013). Stress, emotion regulation and cognitive performance: the predictive contributions of trait and state relative frontal eeg alpha asymmetry. Int. J. Psychophysiol. 87, 115–123. doi:10.1016/j.ijpsycho.2012.09.008
Hawes, M. T., Szenczy, A. K., Klein, D. N., Hajcak, G., and Nelson, B. D. (2021). Increases in depression and anxiety symptoms in adolescents and young adults during the covid-19 pandemic. Psychol. Med. 52, 3222–3230. doi:10.1017/s0033291720005358
Herz, R. S. (1998). Are odors the best cues to memory? a cross-modal comparison of associative memory stimuli a. Ann. N. Y. Acad. Sci. 855, 670–674. doi:10.1111/j.1749-6632.1998.tb10643.x
Holm, A., Lukander, K., Korpela, J., Sallinen, M., and Müller, K. M. (2009). Estimating brain load from the eeg. TheScientificWorldJOURNAL 9, 639–651. doi:10.1100/tsw.2009.83
Igarashi, M., Ikei, H., Song, C., and Miyazaki, Y. (2014a). Effects of olfactory stimulation with rose and orange oil on prefrontal cortex activity. Complementary Ther. Med. 22, 1027–1031. doi:10.1016/j.ctim.2014.09.003
Igarashi, M., Song, C., Ikei, H., Ohira, T., and Miyazaki, Y. (2014b). Effect of olfactory stimulation by fresh rose flowers on autonomic nervous activity. J. Altern. complementary Med. 20, 727–731. doi:10.1089/acm.2014.0029
Jo, H., Song, C., and Miyazaki, Y. (2019). Physiological benefits of viewing nature: a systematic review of indoor experiments. Int. J. Environ. Res. Public Health 16, 4739. doi:10.3390/ijerph16234739
Ko, K.-E., Yang, H.-C., and Sim, K.-B. (2009). Emotion recognition using eeg signals with relative power values and bayesian network. Int. J. Control, Automation Syst. 7, 865–870. doi:10.1007/s12555-009-0521-0
Kosunen, I., Salminen, M., Järvelä, S., Ruonala, A., Ravaja, N., and Jacucci, G. (2016). “Relaworld: neuroadaptive and immersive virtual reality meditation system,” in Proceedings of the 21st international conference on intelligent user interfaces, 208–217.
Kotera, Y., Lyons, M., Vione, K. C., and Norton, B. (2021). Effect of nature walks on depression and anxiety: a systematic review. Sustainability 13, 4015. doi:10.3390/su13074015
Lee, M., Kim, E., Choe, J., Choi, S., Ha, S., and Kim, G. (2022). Psychological effects of green experiences in a virtual environment: a systematic review. Forests 13, 1625. doi:10.3390/f13101625
Li, X., Song, D., Zhang, P., Zhang, Y., Hou, Y., and Hu, B. (2018). Exploring eeg features in cross-subject emotion recognition. Front. Neurosci. 12, 162. doi:10.3389/fnins.2018.00162
Lolak, S., Connors, G. L., Sheridan, M. J., and Wise, T. N. (2008). Effects of progressive muscle relaxation training on anxiety and depression in patients enrolled in an outpatient pulmonary rehabilitation program. Psychotherapy psychosomatics 77, 119–125. doi:10.1159/000112889
Lopes, M. K., de Jesus, B. J., Moinnereau, M.-A., Gougeh, R. A., Rosanne, O. M., Schubert, W., et al. (2022). “Nat (ur) e: Quantifying the relaxation potential of ultra-reality multisensory nature walk experiences,” in 2022 IEEE international conference on metrology for extended reality, artificial intelligence and neural engineering (MetroXRAINE) (IEEE), 459–464.
Lopes, M. K., and Falk, T. H. (2024). Audio-visual-olfactory immersive digital nature exposure for stress and anxiety reduction: a systematic review on systems, outcomes, and challenges. Front. Virtual Real. 5, 1252539. doi:10.3389/frvir.2024.1252539
Mahajan, R. (2018). Emotion recognition via eeg using neural network classifier. Soft Comput. Theor. Appl. Proc. SoCTA 2016 1, 429–438. doi:10.1007/978-981-10-5687-1_38
Maples-Keller, J. L., Bunnell, B. E., Kim, S.-J., and Rothbaum, B. O. (2017). The use of virtual reality technology in the treatment of anxiety and other psychiatric disorders. Harv. Rev. psychiatry 25, 103–113. doi:10.1097/hrp.0000000000000138
McMahan, T., Parberry, I., and Parsons, T. D. (2015). Evaluating player task engagement and arousal using electroencephalography. Procedia Manuf. 3, 2303–2310. doi:10.1016/j.promfg.2015.07.376
Millroth, P., and Frey, R. (2021). Fear and anxiety in the face of covid-19: negative dispositions towards risk and uncertainty as vulnerability factors. J. Anxiety Disord. 83, 102454. doi:10.1016/j.janxdis.2021.102454
Moinnereau, M.-A., de Oliveira Jr, A. A., and Falk, T. H. (2022a). Immersive media experience: a survey of existing methods and tools for human influential factors assessment. Qual. User Exp. 7, 5. doi:10.1007/s41233-022-00052-1
Moinnereau, M.-A., Oliveira, A. A., and Falk, T. H. (2022b). Instrumenting a virtual reality headset for at-home gamer experience monitoring and behavioural assessment. Front. Virtual Real. 3, 971054. doi:10.3389/frvir.2022.971054
Moinnereau, M.-A., Oliveira, A. A., and Falk, T. H. (2023). Quantifying time perception during virtual reality gameplay using a multimodal biosensor-instrumented headset: a feasibility study. Front. Neuroergonomics 4, 1189179. doi:10.3389/fnrgo.2023.1189179
Munyan III, B. G., Neer, S. M., Beidel, D. C., and Jentsch, F. (2016). Olfactory stimuli increase presence in virtual environments. PloS one 11, e0157568. doi:10.1371/journal.pone.0157568
Myrden, A., and Chau, T. (2017). A passive eeg-bci for single-trial detection of changes in mental state. IEEE Trans. neural Syst. rehabilitation Eng. 25, 345–356. doi:10.1109/tnsre.2016.2641956
Parent, M., Albuquerque, I., Tiwari, A., Cassani, R., Gagnon, J.-F., Lafond, D., et al. (2020). Pass: a multimodal database of physical activity and stress for mobile passive body/brain-computer interface research. Front. Neurosci. 14, 542934. doi:10.3389/fnins.2020.542934
Park, K. S., Choi, H., Lee, K. J., Lee, J. Y., An, K. O., and Kim, E. J. (2011). Emotion recognition based on the asymmetric left and right activation. Int. J. Med. Med. Sci. 3, 201–209.
Pietrabissa, G., and Simpson, S. G. (2020). Psychological consequences of social isolation during covid-19 outbreak. Front. Psychol. 11, 2201. doi:10.3389/fpsyg.2020.02201
Pizzoli, S. F. M., Monzani, D., Mazzocco, K., Maggioni, E., and Pravettoni, G. (2021). The power of odor persuasion: the incorporation of olfactory cues in virtual environments for personalized relaxation. Perspect. Psychol. Sci. 17, 652–661. doi:10.1177/17456916211014196
Pope, A. T., Bogart, E. H., and Bartolome, D. S. (1995). Biocybernetic system evaluates indices of operator engagement in automated task. Biol. Psychol. 40, 187–195. doi:10.1016/0301-0511(95)05116-3
Redd, W. H., Manne, S. L., Peters, B., Jacobsen, P. B., and Schmidt, H. (1994). Fragrance administration to reduce anxiety during mr imaging. J. Magnetic Reson. Imaging 4, 623–626. doi:10.1002/jmri.1880040419
Reuderink, B., Mühl, C., and Poel, M. (2013). Valence, arousal and dominance in the eeg during game play. Int. J. Aut. Adapt. Commun. Syst. 6, 45–62. doi:10.1504/ijaacs.2013.050691
Riedel, B., Horen, S. R., Reynolds, A., and Hamidian Jahromi, A. (2021). Mental health disorders in nurses during the covid-19 pandemic: implications and coping strategies. Front. public health 9, 707358. doi:10.3389/fpubh.2021.707358
Saidatul, A., Paulraj, M. P., Yaacob, S., and Yusnita, M. A. (2011). “Analysis of eeg signals during relaxation and mental stress condition using ar modeling techniques,” in 2011 IEEE international conference on control system, computing and engineering (IEEE), 477–481.
Santomauro, D. F., Mantilla Herrera, A. M., Shadid, J., Zheng, P., Ashbaugh, C., Pigott, D. M., et al. (2021). Global prevalence and burden of depressive and anxiety disorders in 204 countries and territories in 2020 due to the covid-19 pandemic. Lancet 398, 1700–1712. doi:10.1016/s0140-6736(21)02143-7
Smith, B. M., Twohy, A. J., and Smith, G. S. (2020). Psychological inflexibility and intolerance of uncertainty moderate the relationship between social isolation and mental health outcomes during covid-19. J. Contextual Behav. Sci. 18, 162–174. doi:10.1016/j.jcbs.2020.09.005
Sowndhararajan, K., and Kim, S. (2016). Influence of fragrances on human psychophysiological activity: with special reference to human electroencephalographic response. Sci. Pharm. 84, 724–751. doi:10.3390/scipharm84040724
Spoorthy, M. S., Pratapa, S. K., and Mahant, S. (2020). Mental health problems faced by healthcare workers due to the covid-19 pandemic–a review. Asian J. psychiatry 51, 102119. doi:10.1016/j.ajp.2020.102119
Sulaiman, N., Taib, M. N., Lias, S., Murat, Z. H., Aris, S. A., and Hamid, N. H. A. (2011). Novel methods for stress features identification using eeg signals. Int. J. Simul. Syst. Sci. Technol. 12, 27–33.
Syed Abdullah, S. S., Awang Rambli, D. R., Sulaiman, S., Alyan, E., Merienne, F., and Diyana, N. (2021). The impact of virtual nature therapy on stress responses: a systematic qualitative review. Forests 12, 1776. doi:10.3390/f12121776
Tarrant, J., Viczko, J., and Cope, H. (2018). Virtual reality for anxiety reduction demonstrated by quantitative eeg: a pilot study. Front. Psychol. 9, 1280. doi:10.3389/fpsyg.2018.01280
Teplan, M., Krakovská, A., and Špajdel, M. (2014). Spectral eeg features of a short psycho-physiological relaxation. Meas. Sci. Rev. 14, 237–242. doi:10.2478/msr-2014-0032
Ward, L. M. (2003). Synchronous neural oscillations and cognitive processes. Trends cognitive Sci. 7, 553–559. doi:10.1016/j.tics.2003.10.012
Wen, T. Y., and Aris, S. M. (2020). Electroencephalogram (eeg) stress analysis on alpha/beta ratio and theta/beta ratio. Indones. J. Electr. Eng. Comput. Sci. 17, 175–182. doi:10.11591/ijeecs.v17.i1.pp175-182
Wu, M., Teng, W., Fan, C., Pei, S., Li, P., and Lv, Z. (2023). An investigation of olfactory-enhanced video on eeg-based emotion recognition. IEEE Trans. Neural Syst. Rehabilitation Eng. 31, 1602–1613. doi:10.1109/tnsre.2023.3253866
Yao, W., Zhang, X., and Gong, Q. (2021). The effect of exposure to the natural environment on stress reduction: a meta-analysis. Urban For. and urban Green. 57, 126932. doi:10.1016/j.ufug.2020.126932
Zhang, X., Bachmann, P., Schilling, T. M., Naumann, E., Schächinger, H., and Larra, M. F. (2018). Emotional stress regulation: the role of relative frontal alpha asymmetry in shaping the stress response. Biol. Psychol. 138, 231–239. doi:10.1016/j.biopsycho.2018.08.007
Zhang, X., Hou, H., and Meng, Q. (2019). “Eeg-based odor recognition using channel-frequency convolutional neural network,” in 2019 Chinese control conference (CCC) (IEEE), 7763–7767.
Keywords: virtual reality, nature scenery, olfaction, multisensory virtual reality, EEG, relaxation
Citation: Lopes MKS, De Jesus BJ Jr., Rosanne OM, Pardini S, Appel L, Smith C and Falk TH (2024) Stop to smell the virtual roses: a mixed-methods pilot study on the impact of multisensory virtual reality nature experiences on feelings of relaxation. Front. Virtual Real. 5:1451704. doi: 10.3389/frvir.2024.1451704
Received: 19 June 2024; Accepted: 06 December 2024;
Published: 23 December 2024.
Edited by:
Diego Vilela Monteiro, ESIEA University, FranceReviewed by:
Justin Maximilian Mittelstädt, German Aerospace Center (DLR), GermanyMonica Bordegoni, Polytechnic University of Milan, Italy
Copyright © 2024 Lopes, De Jesus, Rosanne, Pardini, Appel, Smith and Falk. This is an open-access article distributed under the terms of the Creative Commons Attribution License (CC BY). The use, distribution or reproduction in other forums is permitted, provided the original author(s) and the copyright owner(s) are credited and that the original publication in this journal is cited, in accordance with accepted academic practice. No use, distribution or reproduction is permitted which does not comply with these terms.
*Correspondence: Marilia Karla Soares Lopes, bWFyaWxpYS5zb2FyZXNAaW5ycy5jYQ==
†ORCID: Christopher Smith, orcid.org/0000-0002-3277-3388