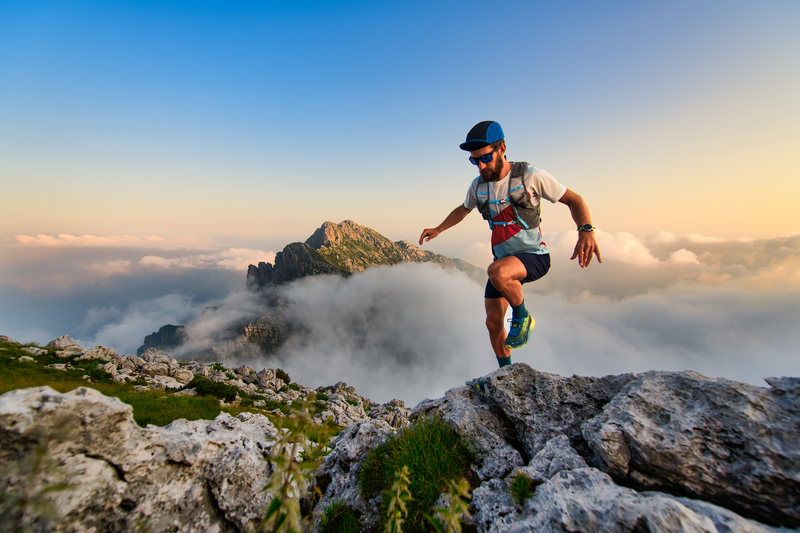
95% of researchers rate our articles as excellent or good
Learn more about the work of our research integrity team to safeguard the quality of each article we publish.
Find out more
ORIGINAL RESEARCH article
Front. Virtual Real. , 19 March 2024
Sec. Virtual Reality and Human Behaviour
Volume 5 - 2024 | https://doi.org/10.3389/frvir.2024.1347102
This article is part of the Research Topic Understanding and Improving the ‘Self’ Using Immersive Virtual Reality View all 8 articles
Public speaking is a communication ability that is expressed in social contexts. Public speaking anxiety consists of the fear of giving a speech or a presentation and the perception of being badly judged by others. Such feelings can impair the performance and physiological activation of the presenter. In this study, eighty participants, most naive in Virtual Reality experience, underwent one of four virtual reality public speaking scenarios. Four different conditions were tested in a between-group design, where the audience could express positive or negative non-verbal behavior (in terms of body gesture and facial expression), together with positive or adverse questions raised during a question-and-answer session (Q&A). The primary outcomes concerned the virtual audience behavior’s effect on perceived anxiety and physiological arousal. In general, perceived anxiety seemed to be unaffected neither by the verbal nor non-verbal behavior of the audience. Nevertheless, experimental manipulation showed a higher susceptibility to public speaking anxiety in those participants who scored higher on the Social Interaction Anxiety Scale (SIAS) than those with lower Social Interaction Anxiety Scale scores. Specifically, in the case where the verbal attitude was negative, high SIAS trait participants reported a higher level of anxiety. Participants’ physiological arousal was also affected by the proposed scenarios. Participants dealing with an approving audience and encouraging Q&A reported increased skin conductance response. The lack of correlation between reported anxiety and skin conductance response might suggest a physiological engagement in an interactive exchange with the virtual audience rather than a form of discomfort during the task.
Public speaking anxiety (PSA) is a specific subtype social anxiety (Hofmann et al., 2004) that considerably impacts on people’s behavior and feelings during public speaking interactions. It consists of a sense of unease and distress experienced when presenting a discussion or speaking in front of an audience. McCroskey (1970), McCroskey (1977) referred to the fear or anxiety felt during real or anticipated communicative scenarios as communicational apprehensions (CA). Actually, it is quite common that individuals may experience different CA levels in both public and interpersonal communicational situations. This apprehension often fluctuates depending on situational variability (state communication apprehension) but may also manifest as a consistent trait within some individuals (trait communication apprehension). People with high CA trait levels, exhibit heightened apprehension across a broad spectrum of oral communication interactions, leading to avoidance behaviors and negative self-evaluation (McCroskey, 1977). This condition can spread beyond mere apprehension and evolve into a pathological state, as PSA is, characterized by elevated stress levels and bodily discomfort that occurs during social interactions (Stein and Chavira, 1998; Hoffman et al., 2004). PSA can be itself an obstacle to professional growth (Harris et al., 2002; Raja, 2017), as it hampers the effective communication of ideas and expertise in public environments (Ferreira Marinho et al., 2017). Moreover, the negative consequences of PSA extend to interpersonal relations, leading to social isolation and hinder the development of meaningful personal relationships due to the fear of negative judgment coupled with the importance attributed to positive evaluations from others (Clark and Wells, 1995; Rapee and Heimberg, 1997; Raja, 2017). According to Clark and Wells (1995), people with social phobia exhibit cognitive distortions regarding their social performance and how others perceive them. Specifically, they tend to overestimate the extent of negative evaluations from others and the probability of committing social errors. This cognitive distortion fuels persistent rumination over perceived flaws or mistakes, perpetuating an inaccurate self-perception and a distorted view of own social performances and interactions. Similarly, Rapee and Heimberg (1997) emphasize that negative self-perception plays a pivotal role in sustaining social anxiety. This negative self-perception is exacerbated by individual’s heightened sensitivity to every negative social cues. Furthermore, those suffering with social phobia often exaggerated the probability of others detecting and negatively evaluating their perceived flaws, even when such judgments are unlikely or non-existent. All of this amplifies anxious symptoms and behaviors, such as avoidance, reinforcing the negative cognitive framework. These models together undelight the critical role of cognitive distortion in perpetuating social phobic conditions, such as PSA. Such distortions may rise to a detrimental cycle of fear, inhibition, and avoidance of anxiety-producing social situations. This contributes to the persistence of the anxiety, increasing both its frequency and intensity, reinforcing dysfunctional beliefs of the individual as inadequate and under judgment.
PSA affects physical and emotional wellbeing, also increasing physiological arousal before, during, and after the task (Goodman et al., 2017). Indeed, according to the three-system model (Lang, 1968), socially triggering situations are linked with a multifaceted range of responses. It is not just the cognitive and overt behavioral reactions that come into play; there’s also an interplay with physiological responses. Grappling with actual or anticipated public speaking situations, individuals experience physiological reactions such as heightened heart rate, facial or skin flushing, and electrodermal activation (Bodie, 2010).
Virtual Reality (VR) is a valuable tool for experiencing public speaking in an immersive environment and studying anxiety in a realistic yet controlled way (North et al., 1998). Indeed, VR has the potential to evoke physiological reactions and comparable levels of presence to natural public talk environments within a PSA situation (Riva, 2009; Gallace et al., 2011; Higuera-Trujillo et al., 2017; Lanier, 2017), even in front of an audience composed by a small group of virtual character (Mostajeran et al., 2020). Furthermore, people tend to treat a virtual human as a social actor rather than a mere image (Reeves and Nass, 1996). Thus, a VR conversation task may elicit levels of subjective distress comparable to a corresponding in vivo task (Powers et al., 2013). Moreover, VR enables manipulation of the virtual audience and the interaction dynamics between participants and the audience, exploring the influence of listeners’ features during a public speech. According to Blascovich (2002), humans induce a greater sense of social presence than agents during interactions, that result in greater social influence. Essentially, real humans consistently wield social influence, while virtual humans influence hinges on their behavioral authenticity, depending on the realism and the agency of the virtual audience. Research in this direction (El-Yamri et al., 2019) pointed out how it is a complex task to create realistic feedback in the audience for the presentation. It is influenced not only by the words but emotions, as a non-verbal behavior transmitted by the presenter and the audience. One of the hardest tasks of researchers is to create agents or avatars in virtual reality environments expressing emotions in a way that is convincing on a subconscious level, too (Norman, 2005). That’s why creating virtual audiences (VAs), made of humanlike virtual spectators, takes inspiration from the seven universal emotional facial expressions (Ekman, 1999) and how the entire body, by postures or gestures, is involved in sending information about our emotional state to others around us (Nummenmaa et al., 2014). Static nonverbal behavior of the virtual audience (VA) can stand for an emotionally neutral audience, friendly and appreciative behavior simulates positive reactions towards the speaker; instead, adverse and bored expressions throughout the presenter’s speech can lead to perceiving the audience negatively (Pertaub et al., 2001). Also, supportive or non supportive VAs’ feedback, comparable to real audiences, can influence the levels of anxiety in speakers (Kelly et al., 2007). Considering that nonverbal behaviors of the audience can be perceived in the dimensions of valence (opinion) and arousal (engagement) (Kang et al., 2016), cognitive models, such as valence-arousal (Chollet et al., 2014; Chollet and Scherer, 2017), can also guide the configuration of nonverbal behaviors in order to express clearly recognizable and replicable information in virtual multimodal public speaking performance (Glemarec et al., 2021). The frequency of gazing away or looking at the presenter is a fundamental indicator of the audience’s engagement, such as a bored or interested attitude. Head movements, like nodding in agreement or disagreement, suggest the speaker the audience’s opinion (Chollet and Scherer, 2017; Etienne et al., 2022).
A recent study (Girondini et al., 2023a) shows that the audience’s feedback during a simulated public speaking task can uplift or relieve Public Speaking Anxiety (PSA). Positive audience feedback can enhance the speaker’s self-esteem, reduce anxiety, and increase overall satisfaction with the speaking experience. Conversely, negative audience feedback can have detrimental effects on the speaker. However, in real-life scenarios, the audience’s feedback might be expressed through verbal and non-verbal communication during a speech. Nonverbal interactions, such as body language, can complete verbal messages in a public speaking context and play a crucial role in connecting the speaker and the audience. Non-verbal behavior cues, such as eye-gazing, facial expression, hand gestures, and voice pinch, impact the perceived persuasiveness and credibility of the speaker (Burgoon et al., 1990), as well as be an indicator of perceived anxiety and the emotional states lived by the speaker during the speech (Laukka et al., 2008). The audience’s body gestures or postures can add further negative or positive feedback to the speakers and thus affect their performance and feelings.
Recognizing the influence of the audience’s non-verbal behavior and more explicit feedback might help understand and define mechanisms contributing to public speaking anxiety. Nevertheless, in virtual as in real scenarios, public speaking is not a one-way but a bidirectional exchange of communication between the speakers and the audience; the orator can overcome the positive or negative feedback of the listeners (Slater et al., 1999). Notably, the specific effects of audience feedback can vary depending on individual differences and situational factors. Some speakers may be more sensitive to feedback, while others may be more resilient. In this context, considering psychological traits related to anxiety may be an essential aspect to be considered when investigating public speaking anxiety. Indeed, previous studies have shown that individual anxiety traits could be a predictive factor influencing the outcomes of experimental manipulations on public speaking performance, with a positive correlation between social anxiety traits and experienced anxiety before and during the speech (Cornwell, et al., 2006; Witt et al., 2006).
The present work aims to explore the interplay among different audience behaviors (verbal and non-verbal) and unique anxiety traits of the speakers in inducing public speaking anxiety. We assigned each participant to one of four experimental groups characterized by various combinations of the audience’s non-verbal behavior (interested vs. uninterested) and explicit audience feedback following the talk (positive questions vs. negative questions). The measurements in this study included self-report anxiety questionnaires, the perceived anxiety after the performance, sense of presence, and audience perception. Moreover, the physiological activation (Heart Rate and Skin Conductance Response) was recorded before and during the task. We expected a difference in perceived anxiety and physiological arousal between the supportive and the hostile audience, with a more pronounced effect for the verbal component than the non-verbal manipulation. The hypothesis is supported by the fact that the nonverbal component may be less clearly inferred than the verbal one; some people could not be able to use facial expressions as evidence of the feedback judgment of audience (Kang et al., 2016). Also, nonverbal neutral static audience scenarios are less effective than positive or negative, more interactive speaking publics (Pertaub et al., 2001). Specifically, based on previous studies, we hypothesized that negative verbal behavior would induce a higher level of perceived anxiety and increased arousal. Instead, positive feedback (in both verbal and non-verbal features) should ease perceived anxiety during and after the performance. However, the impact of hostile non-verbal feedback might amplify the effect of negative verbal behavior compared to hybrid conditions, whereas verbal and non-verbal behaviors do not align. Given the lack of previous studies investigating the relationship between the audience’s verbal and non-verbal components on induced anxiety during public speaking, the nature of this experiment is, in part, explorative in this specific aspect.
The participants were recruited by self-enrollment using the University website. University students were given course credit for participating. The sample size for the study was comparable with previous research with similar design and measurement (Kroczek and Mühlberger, 2023). Eighty healthy participants (mean age = 25,16 years, SD = 6,48, age range = 18–58, 48 female) participated in the experiment. A sensitivity power analysis with eighty participants, beta = 0.80 and alpha = 0.05, allow detecting the effect of small-to-medium effect size (f = 0.3). Thirty-two participants had previous experiences with Virtual Reality, while forty-eight had not. They gave written informed consent to participate in the study, which was approved by the Ethical Committee of the University of Milano-Bicocca and conducted following the standards of the Helsinki Declaration.
The experimental design involved participants performing a virtual reality public speaking task. Participants were randomly distributed into four experimental groups (twenty participants in each group), which differed for the Verbal behavior (positive vs. negative questions) and Nonverbal behavior (positive vs. negative attitudes) expressed by the virtual audience during the performance. This resulted in a 2 × 2 between design, with the main factors of Verbal and Non-verbal behaviors. The nonverbal behavior refers to the first part of the talk, where the audience could express positive (e.g., Nodding in agreement, Looking the presenter in the eyes, Facial Expressions: Enjoyment and Surprise) or negative (e.g., Nodding in disagreement, talking to others in the audience, Looking another way instead) nonverbal behaviors while the participants exposed a cooking recipe (rice or cake recipe). The second part of the performance comprised a Question-and-Answer session (Q&A), where the virtual audience asked participants about the recipe. Again, the virtual audience could ask positive questions (e.g., encouraging questions: It was a very innovative recipe! Will be difficult to find ingredients during summer?) or negative questions (e.g., annoying questions: I’m not convinced about your recipe, it seems so obvious, why did you choose to present this recipe?).
Using a mixed factorial design regarding Verbal and Nonverbal behaviors expressed by the audience, four experimental conditions characterized the investigation:
- Positive Attitude–Positive Questions (PA_PQ): The scenario was composed by a virtual audience who expressed positive Nonverbal behavior and raised positive questions
- Positive Attitude–Negative Questions (PA_NQ): The scenario was composed by a virtual audience with positive Nonverbal behavior, but raised negative questions
- Negative Attitude–Positive Questions (NA_PQ): The scenario was composed by a virtual audience who expressed negative Nonverbal behavior but raised positive questions
- Negative Attitude–Negative Questions (NA_NQ): The scenario was composed by a virtual audience who expressed negative Nonverbal behavior and raised negative questions
Figure 1 shows a graphical representation of the experimental design used in this study. The primary outcome measure concerned the participants’ perceived anxiety after the talk, depending on the verbal and non-verbal behaviors of the audience. Secondary analyses on perceived anxiety were performed considering the anxiety questionnaire scores. Additionally, physiological activity during the performance (skin conductance activity and heart rate) was collected and analyzed. Finally, correlation analyses were conducted between subjective reports, anxiety, and physiological activity. Overall, this design served us to investigate how Verbal and Nonverbal behaviors expressed by the virtual audience during the task impact anxiety and physiological responses during public speaking performances in virtual reality.
The VR equipment used for the experiment included an Oculus Quest 2 Head-mounted- display (HMD) with a 1280 × 1440-pixel resolution per eye (refresh rate 80 Hz). The HMD was connected to a computer featuring an Intel Core i7-7800X CPU, 16 GB of RAM and a GeForce GTX GPU. The four virtual reality environments (VREs) (Figure 2) were developed with the Unity graphics Engine (https://unity.com/.) for this experiment. Human voices were added to the audience characters. Each scenario showed a room where eleven chairs were arranged. Eight avatars were sitting in some of these chairs, with four males and four females, all dressed informally. A virtual projection board hanging on the wall just behind the audience and in front of the speaker showed the sequence of the different pictures involved in preparing the recipe being presented by the participant. Adobe Fuse was used for the creation of the humanlike avatars. Body movements were selected from the Adobe Mixamo library (https://www.mixamo.com), combined, and blended into Unity using layers and masks. All members of the audience were in a sitting position. Different sitting positions were used again for the purpose of a natural look. For example, sitting with legs crossed was applied on female avatars, while a more relaxed pose was applied on male avatars. In total, 5 sitting poses are used, including 3 females and 2 males. To create a natural setting, avatars in the same pose are not sitting close to each other. The neutral movements are turning the head left or right, scratching a hand, moving on the chair, breathing, and blinking. The positive and negative gestures are represented by nodding respectively in agreement or disagreement. Facial expressions were also part of the movements. The positive ones are represented by enjoyment and surprise, while the negative ones by contempt and disgust. The animations on the faces were based on Paul Ekman’s characteristics of the facial expressions of emotions (Ekman, 1999). Negative non-verbal behavior was conveyed through facial expressions expressing contempt or disgust, whereas positive non-verbal conditions were represented by expressions of enjoyment or surprise. Unity blend shapes were used to create these animations by altering the faces of the avatars. Once created, those animations were reused by applying them on different avatars. The facial expressions were applied to the audience from the first rows to increase the visibility of the speaker. All animated body movements were created with the Unity graphics engine. The timeline and the number of gestures were kept the same between the two visual settings. The frequency of the movements is spread throughout the length of the experiment, with different time windows between each. The same number of facial expressions and gestures with the same timeline are used in both experimental environments with replacing of the negative with positive gestures and facial expressions between the versions. In total, 17 facial expressions and 17 body movements were implemented for all avatars throughout the experiment’s duration (i.e., positive non-verbal behavior: Nodding in agreement, Looking the presenter in the eyes; i.e., negative non-verbal behavior: Nodding in disagreement, Talking to others in the audience, Looking another way instead of at the presenter). The spread of movements, both facial and body, was random. For the question session, mouth movement was implemented in Unity and added to one of the audience avatars. The animation was done using blend shapes in Unity, the same used for the facial expressions animation. The mouth movement was synchronized with the duration of the avatar speech. Two audio recordings, respectively negative and positive versions, were used and embedded into the timeline to be replicated the same way between experiments. All animations (body movements, facial expressions, blinking, breathing, speaking) were blended together with Unity features layers and masks. This allows the avatar to perform a few movements in a smoothed way simultaneously, e.g., nodding in agreement and blinking. Different speed index is applied between the avatars on the animations for breathing and blinking to avoid the movements happening at the same time, therefore, creating a natural looking audience.
STAI (State-Trait Anxiety Inventory): STAI is a well-known anxiety self-assessment scale comprising two subscales measuring transient and enduring anxiety levels (Spielberger et al., 1971). Each scale has 20 items, and both scales include items that describe symptoms of anxiety and items that define the absence of anxiety. We used the Italian version of STAI.
SIAS (Social Interaction Anxiety Scale): SIAS is a self-report questionnaire that measures the presence of fear during general social interactions. The Italian validated version of the questionnaire contains 19 items evaluated on a 5-point Likert scale, rating from 0 (not at all) to 4 (extremely) (Heimberg et al., 1992).
SSQ (Simulator Sickness Questionnaire): SSQ is a motion sickness questionnaire that assesses sickness after virtual reality experiences. The version used in this experiment contains 16 items, divided into three categories: nausea, oculomotor problems and disorientation (Kennedy et al., 1993).
Three self-report statements were used to assess participants’ subjective experiences during the task. Each statement was evaluated using a 10-point Likert scale, ranging from 1 (not at all) to 10 (extremely). The measurements were collected after each experimental session. The focus of Likert assessments concerned:
- Perceived immersion in the virtual environment (I felt like I was inside the environment shown)
- Perceived audience attention (I had the feeling that the audience (e.g., characters) was listening to me)
- Level of anxiety evoked by the task (What level of anxiety did you experience on a scale from 1 to 10)
Furthermore, the participants’ arousal and emotional states following the performance were assessed using the Self-Assessment Manikin (SAM) (Bradley and Lang, 1994). They were instructed to express their arousal and emotional state by choosing one of nine manikins that depicted varying levels of emotional (facial expression) and arousal (body) states.
Electrodermal activity (EDA): The measurement of the phasic level of skin conductance is a highly suitable marker for sympathetic nervous system activation (Turpin et al., 2009). EDA is a well-known marker of anxiety during public speaking (Giesen and McGlynn, 1997; Arsalan and Majid, 2021). For example, Croft and colleagues used EDA values to predict state-dependent speech anxiety in a student sample during a public speaking task (Croft et al., 2004). The focus of EDA measurement during public speaking was related to the phasic (fast) change of electrodermal activity. In particular, for each exposure, we used the Non-specific Skin Conductance Response (NS-SCR) as an index of electrodermal activity: The NS-SCR is the frequency of phasic level of electrodermal activity that occurs spontaneously, not related to external stimuli (in a fixed time-window) (Nikula, 1991; Gertler et al., 2020). This measure has been previously used to measure fear-induced arousal during public speaking situations (Niles et al., 2015). In the present study, a Biopac BioNomadix MP 150 device recorded the electrodermal signal through two AgCl electrodes attached to the participant’s index finger and ring finger for Skin Conductance measurements. As regards the electrodermal activity measurements, data were further elaborated using the Matlab-based script Ledalab (version 3.4.8) by adopting a continuous decomposition approach (Benedek and Kaernbach, 2010). The signal was recorded at 100 Hz and downsampled to 10 Hz for the analysis. We extracted one measure of interest for each exposure, which lasted approximately 6 min: the mean amplitude of non-specific skin conductance responses (NS-SCR) that overcame the 0.03 micro Siemens and used it as the dependent variable in the analyses.
Heart rate (HR). Heart rate is a standard measurement for evaluating physiological stress in public speaking situations (Slater et al., 2006; Owens and Beidel, 2015; Takac et al., 2019). We measured Beats per minute (bpm) using a Procompt Infiniti 5 device through a Blood Volume Pulse (BVP) sensor attached to the middle finger. Since HR indicates participants’ stress-related sympathetic activity, elevated HR values are markers for heightened physiological arousal.
The participants were asked to sign the informed consent form and complete online self-report questionnaires assessing anxiety, interoception and previous VR experiences. Then, participants were comfortably seated in a silent room, and they were asked to wear the respiration sensor around their chest, and the EDA and BVP sensors were attached to the participant’s left hand. A 3-min preliminary psychophysiological data recording was made (Baseline phase). The experimenter explained to the participants that they would have to present a recipe to the VR audience and recipe images behind the audience, which would have helped them during the task. So, participants read and memorized the steps of a recipe, rice or pie, as best they could in about 5 minutes. When the participants were ready, participants wore the Head-Mounted-Display (HMD). They started their public speaking task (PST), which consisted of explaining the recipe they had read before to the avatars while psychophysiological data were recorded (Figure 3).
In the first part of the task, the audience rested in silence, listening to the participant’s speech and showing a positive or negative non-verbal attitude. Specifically, in the positive scenario, the audience was quiet, nodding, and attentive, while in the negative scenario, the audience was inattentive, shaking their heads, rolling their eyes or snorting. At the end of the 3-min presentation, the avatars applauded the speaker. Then, the Questions & Answers (Q&A) session started, characterized by automatic questions from the audience about the recipe that participants had to answer in 45 s each. The four rice recipe questions were positive (es. “Thanks for your presentation; I like your recipe so much … I do not like mushrooms. Can I substitute them with something else?”; “I always found it very difficult to cut the pumpkin, but your methods seem very efficient. Could you repeat this?”), while the pie recipe ones were negative (es. “Cinnamon is disgusting, why would I ever mess this recipe with it?”, “Honestly, I too have my doubts, are you sure lemon is an ingredient … my grandmother never prepared the recipe with lemon”).
The speaking task lasted 6 minutes; after that, the participants removed the HMD and underwent the phase of 3-min rest psychophysiological data recording (Rest phase).
At the end of the experiment, participants filled in a 10-point Likert scale to report their perceived sense of presence, the audience’s interest, anxiety, and experience of pleasantness; SAM to state their perceived level of arousal and emotional feelings; SSQ to check their sickness after virtual reality experience (Figure 4).
Figure 4. Sketch of the experimental procedure. Participants filled in preliminary questionnaires. In the following baseline phase, psychophysiological data were recorded (3 min). Then, participants read the recipe steps. During the Public Speaking Task, participants explained the recipe and answered the audience’s questions while psychophysiological data were recorded (6 min). At the end of the task, psychophysiological data were recorded in the Rest phase (3 min). Then, SAMs, Likert scales, and SSQ were filled in.
Statistical analyses were performed by R software (www.r-project.org). As the first analysis, we performed a correlation analysis (r-Spearman) to individuate a possible correlation between the anxiety questionnaire scores and perceived anxiety after the public speaking performance. The same approach was used for correlation analysis between physiological measurements with perceived anxiety after the performance and anxiety questionnaire scores.
Then, self-report measurements (Likert scales) were analyzed using a 2 × 2 between model ANOVA with Verbal behavior (positive vs. negative questions) and Non-verbal behavior (positive vs. negative attitude) as main factors. ANOVA analyses exhibited robustness to normality distribution violations arising from using the Likert scale, as indicated by Norman (2010) and also supported by Higgins et al. (2022) and Girondini et al. (2023b) within public speaking investigations. Secondary analysis explored any plausible relationship between experimental manipulation, self-report anxiety scores, and perceived anxiety. We extracted each participant’s average mean value as the dependent variable to analyze the Skin conductance (NS-SCR). Raw data were normalized using log-transformation and analyzed using mixed ANOVA with two main factors: verbal behavior (positive vs. negative questions) and non-verbal behavior (positive vs. negative attitude). For Heart rate (HR), the average values were first corrected with baseline subtraction. A mixed ANOVA with two main factors of verbal behavior (positive vs. negative questions) and non-verbal behavior (positive vs. negative attitude) was used to analyze skin conductance and HR measurements.
The average anxiety score revealed normative values, confirming that our participants belonged to a nonpathological sample: STAI-S (M = 38.04, SD =8.67), STAI-T (M = 45.90, SD = 9.56), SIAS (M = 26.70, SD = 11.10). The internal reliability for anxiety self-report questionnaire was 0.74 (ω). The values of each experimental group are presented in Table 1. SSQ questionnaires were assessed after the VR exposure to detect the possible presence of sickness due to the device used. The SSQ scores revealed a low level of sickness (M= 3.13, SD = 2.68, raw values), suggesting that the participants endured the VR public speaking experiences well.
The scatterplot with Spearman Correlation is presented in Figure 5. The p-value of each correlation is presented in Table 2. A positive correlation among anxiety questionnaires (SIAS STAI-T and STAI-S) was found. Only SIAS score positively correlated with perceived anxiety (r = 038, p < 0.001). Perceived immersion in virtual environment negatively correlated with STAI-T (r = −0.27, p = 0.018) and SIAS (r = 0.029, p = 0.012), but positively correlated with perceived audience attention (r = 0.61, p < 0.005). SAM arousal positively correlated with SIAS (r = 0.23, p = 0.049) and perceived anxiety (r = 0.51, p < 0.001). SAM emotion negatively correlated with STAI-S (r =–0.38, p < 0.001) and SIAS (r = −0.44, p < 0.001) and positively correlated with perceived audience attention (r = 0.26, p = 0.023) and perceived immersion in virtual environment (0.036, p = 0.001). Notably, no correlation was found between self-report measurements and skin conductance activity.
Figure 5. Scatterplot with Spearman Correlation for self-report measurements and skin conductance activity.
Perceived immersion in virtual reality: No main effect of Verbal behavior [F (1,76)=1.28, p = 0.261] or Nonverbal behavior [F (1,76) = 0.49, p = 0.485] was found in respect to the perceived immersion in virtual reality. The interaction effect Verbal behavior * Nonverbal behavior resulted non-significant [F (1,76) = 1.53, p = 0.219].
Perceived audience attention: No main effect of Verbal behavior [F (1,76) = 1.12, p = 0.292] or Nonverbal behavior [F (1,76) < 0.01, p = 0.995] was found for the perceived audience attention. The interaction effect Verbal behavior * Nonverbal behavior showed a non-significant trend [F (1,76) = 2.98, p = 0.087]. The interaction plot (Figure 6) shows the source of the exchange. Specifically, for negative Nonverbal behavior (negative attitude during the speech), the audience’s evaluation depended on the audience’s verbal behavior during Q&A. That is, participants who experienced negative nonverbal behavior but coupled with positive questions judged the audience to be more attentive to the speech (M = 7.15, SD = 2.08) compared to when the negative attitude was followed by negative questions (M = 5.9, SD = 1.71). Regarding positive Nonverbal behaviors, the perceived audience attention was comparable after positive questions (M = 6.65, SD = 2.03) and negative questions (M = 6.35, SD = 2.16).
Perceived anxiety after the performance: No main effect of Verbal behavior [F (1,76) = 0.05, p = 0.817] or Nonverbal behavior [F (1,76) = 0.66, p = 0.419] was found in perceived anxiety after the performance. The interaction effect Verbal behavior * Nonverbal behavior showed a non-significant effect [F (1,76) = 1.34, p = 0.250]. A second analysis, including anxiety questionnaire scores as a covariate, was conducted as no significant effects were observed when considering only the two factors. The secondary analysis considered three models, including the anxiety questionnaire scores (SIAS, STAI-T, and STAI-S) as covariates. We employed the Akaike Information Criterion (AIC) and Bayesian Information Criterion (BIC) parameters to select the most appropriate model. The analysis revealed that the covariate SIAS had a more significant influence on the statistical model than other scales (DV ∼ verbal behavior * nonverbal behavior * SIAS: AIC = 327.744, BIC = 346.182; DV ∼ verbal behavior * nonverbal behavior * STAI-S: AIC = 337.679, BIC = 359.117; DV ∼ verbal behavior * nonverbal behavior * STAI-T: AIC = 335.233, BIC = 356.671).
Mixed model ANOVA with SIAS as a covariate revealed no main effect of Verbal behavior [F (1,76) = 0.06, p = 0.797] and no main effect of Nonverbal behavior [F (1,76) = 0.81, p = 0.369] in respect to the perceived anxiety. SIAS score was significant on perceived anxiety [F = 12.82, p < 0.001]. The interaction between Verbal behavior * Nonverbal Behavior was not significant [F (1,76) = 2.01, p = .160]. However, a significant interaction effect on Verbal behavior * SIAS was found [F (1,76) = 4.45, p = 0.038].
In order to investigate the interaction effect, we performed a post hoc analysis utilizing an independent t-test with Bonferroni correction, where the adjusted p-value was set to 0.012 (0.05 divided by 4) and participants were divided into two groups (High vs. Low Social Interaction Anxiety Scale (SIAS)) using a median split. Post-hoc t-test resulted in a non-significant effect for perceived anxiety in high vs. low anxiety traits under positive verbal behavior (t = 0.26, df = 37.56, p = 0.791). The contrast positive vs. negative behavior resulted in non-significant in both high SIAS participants (t = 1.95, df = 35.37, p = 0.058) and in the low SIAS participants (t = - 1.16, df = 39.91, p = 0.251). However, post hoc analysis comparing perceived anxiety in high vs. low SIAS anxiety traits resulted significantly under the negative verbal behavior condition (t = −3.28, df = 37.81, p = 0.002). The interaction effect is presented in Figure 7. That is, participants with high SIAS scores exposed to negative questions reported more anxiety (M = 5.83, SD = 1.72) compared to participants exposed to the same scenario but with low SIAS scores (M = 3.91, SD = 1.97). In comparison, the SIAS score did not impact perceived anxiety in the case of positive verbal behavior (High SIAS: M = 4.75, SD = 1.68; low SIAS: M = 4.6, SD = 1.88). No interaction effect in Nonverbal behavior * SIAS was found [F (1,76) < 0.01, p = 0.994].
Notably, the three-way interaction Verbal Behavior * Nonverbal behavior * SIAS was marginally significant [F (1,76) = 4.17, p = 0.044]. Again, the source of three-way interaction concerned the difference in perceived anxiety depending on the SIAS score within the combination of Verbal and Nonverbal behavior (Figure 8). Specifically, participants with higher SIAS scores exposed to both negative verbal and non-verbal behavior reported more anxiety (M = 6.12, SD = 1.96) compared to low SIAS participants (M = 4.58, SD = 2.19) exposed to the same scenario. Similarly, participants higher in SIAS exposed to positive non-verbal behavior but negative questions reported more anxiety (M = 5.6, SD 1.58) compared to low SIAS participants exposed to the same scenario (M = 3.1, SD = 1.37). In case of positive verbal behavior expressed by the audience, the perceived anxiety was comparable between positive vs. negative nonverbal behavior in high vs. low SIAS score (negative nonverbal behavior and high SIAS score, M = 4.5, SD = 1.78; negative nonverbal behavior and low SIAS score, M = 4.7, SD = 2.31; positive nonverbal behavior and high SIAS score, M = 5, SD = 1.63; positive nonverbal behavior and low SIAS score, M = 4.5, SD = 1.43). However, none of the comparisons were significant after post hoc correction (all p n. s).
Figure 8. Three-way interaction Verbal behavior * Nonverbal behavior * SIAS traits on perceived anxiety.
Heart rate (HR): No main effects of Verbal behavior [F (1,76) = 2.24, p = .138] and Nonverbal behavior [F (1,76) = 0.23, p = 0.630] were found concerning the HR (baseline corrected). The interaction effect Verbal behavior * Nonverbal behavior resulted non-significant [F (1,76) = 0.91, p = 0.647 The interaction effect Verbal behavior * Nonverbal behavior resulted non-significant [F (1,76) = 0.21, p = 0.342].
Non-specific skin conductance response (NS-SCR): Six participants (PA-PQ = 3, PA-NQ = 1, NA-PQ = 2) were excluded from the analysis given the lack of skin conductance response during the public speaking task. Then, we performed the analysis on the remaining 74 participants. A main effect of Verbal behavior [F (1,70) = 5.52, p = 0.021] was found (Figure 9). Participants exposed to a positive verbal behavior audience presented higher amplitude (M = 0.541, SD = 0.343) compared to the negative condition (M = 0.377, SD = 0.255) (Figure 9). The main effect of Nonverbal behavior [F (1,70) = 0.29, p = 0.682] and the interaction effect [F (1,70) = 3.51, p = 0.065] Verbal behavior * Nonverbal behavior resulted in a non-significant effect.
The present study investigated the interplay of verbal and non-verbal behavior of virtual audiences in inducing public speaking anxiety using virtual reality. Participants were distributed into four experimental groups in which the virtual audience of the speech exhibited positive or negative non-verbal attitudes when the participants told the topic of the speech. During the second part of the task, the same virtual audience was engaged in a Q&A session with the participants, expressing supportive or annoying questions. The study included self-report measurements of the virtual experience, perceived anxiety and the physiological activation (skin conductance) during the performance.
First, no difference in the perceived immersion inside the virtual environment was found across different conditions. This result is not surprising, given that the graphic and acoustic elements used for the favorable and hostile audiences were similar among the scenarios. Moreover, participants did not report a clear difference in audience perception between positive and negative non-verbal behavior. Participants’ self-report measurement revealed a trend toward the impact of non-verbal behavior in evaluating the audience’s interest during the speech. This might suggest that our participants probably did not entirely capture the features used to manipulate the audience’s attitude, perhaps due to the difficulty of mimicry implicit and subtle audience features in virtual reality (Kroczek and Mühlberger, 2023). This lack of distinction might reflect the difficulty of reproducing a more implicit component of social interaction, as the non-verbal behavior is. Another explanation is that including pictures behind the agents might have distracted the participants from the audience, letting the speaker focus more on the speech. Including further measurements, such as eye-tracking, might be helpful to clarify if participants pay attention to the audience or not during the exposure.
The analysis of the perceived anxiety did not reveal the main effects of verbal and non-verbal behavior, nor the interaction effect. This evidence could come from the fact that our participants may have failed to capture the manipulated public attitudes features completely; this would not have allowed us to detect an effect of the assigned scenario on perceived anxiety. However, the absence of any effect from the experimental manipulation could also be ascribed to variations in susceptibility to public speaking anxiety between individuals with high versus low anxiety traits. Indeed, a supplementary analysis was conducted using anxiety scale scores as covariates. Notably, the Social Interaction Anxiety Scale (SIAS), which concentrates on the anxiety of social interactions, explained the more variance with the data as opposed to more general anxiety scales such as the State-Trait Anxiety Inventory (STAI). This is further substantiated by the observed positive correlation between SIAS scores and reported anxiety post-performance, contrasting with the absence of such correlation for the STAI scales. As one might expect, participants higher on the SIAS scale reported more anxiety compared to the low SIAS score participants. Notably, the SIAS score also influenced the impact of the negative verbal behavior of the audience during the speech on perceived anxiety. Indeed, participants with high SIAS scores reported much more anxiety compared to participants exposed to the same scenario but characterized by low SIAS scores. Similarly, the three-way interaction showed a higher level of anxiety in the case of both negative verbal and nonverbal behavior scenarios for high SIAS scores participants. This suggests that a hostile and adverse audience can significantly impact anxious speakers, particularly those with higher SIAS scores, who may also experience more pronounced anxiety, maybe due to a specific negative belief system. (Beidel et al., 1985). According to Rokeach (1960), a belief system constitutes the array of convictions, values, and perspectives that influence how individuals interpret the world and their experiences. So, during our task, participants with high SIAS levels might have analyzed the experience as more anxious by perceiving more significant threats or risks than they were. Indeed, our findings align with previous research on public speaking and anxiety traits. For instance, Perowne and Mansell (2002) found that, compared to low-trait anxiety scores, participants with higher anxiety scores were more likely to perceive their performance as worse.
Considering the physiological arousal during the speech, participants engaged in a positive and encouraging Q&A session showed increased skin conductance activity. Our finding might contradict the classical idea of physiological arousal as a marker of stress and anxiety (Jacobs et al., 1994). However, it is essential to note that skin conductance and physiological arousal, in general, reflect the activity of the sympathetic nervous system without any emotional valence (i.e., excitement or stress or fear). Indeed, physiological arousal indicates how exciting an emotional experience is (Kensinger, 2004) but does not explain the valence of the experience: the aroused state feels no different from one type of emotion to another (i.e., joy, anger, passion, anxiety). Moreover, skin conductance might also reflect a measurement of attention and engagement (Frith and Allen, 1983). The physiological activity should also be interpreted considering the self-report experience and the context of experimental manipulation. In a previous study, our research group (Frigione et al., 2022) demonstrated different meanings of skin conductance activity depending on the context in which the participant was exposed. This is particularly true for VR experiments involving participants in immersive and realistic experiences. Indeed, in the present study, it is more plausible that the increased skin conductance activity reflected the “engagement” of the participants in a pleasant and interactive exchange with the virtual audience. This interpretation seems supported by the lack of correlation between the skin conductance activity and the perceived anxiety reported by the participants. Future investigations are needed to clarify the meaning of physiological measurement in a public speaking context and integrate it with what the participants report from the experience (implicit explicit measurement comparison). In this case, the arousal experienced during the PS task may increase goal engagement in the speaker, progress toward goals (i.e., completing the speech), and self-efficacy (Carver and Scheier, 1998; Pavett, 2016).
One limitation of this study is related to the graphic similarity among the scenarios that could have weakened the impact of the nonverbal gestures of avatars that needed to be more clearly distinguishable in positive and negative. Moreover, the interaction between audience and speakers was based on pre-recorded questions. In the future, the use of artificial intelligence may overcome these limits and allow online interaction. A second main limit is the lack of deeper investigation regarding the sense of presence: we limited the investigation of perceived immersion in the virtual environment using a Likert scale. Indeed, the lack of Italian-validated questionnaires prevents us from using standardized presence scores. However, it is important to note that sense of presence was not a primary outcome in our study since graphical features of the environment were comparable across experimental conditions. In general, using HMD allowed participants to live a virtual experience. Nevertheless, the absence of any sensory feedback limited the sense of immersion and emotional engagement (Montana et al., 2020). This circumstance could have affected the participants’ responses. Another important consideration concerns the sample, which is mainly composed of university students. Future investigations could confirm these findings in a large and heterogeneous population.
To summarize, this study explored the impact of the audience’s verbal and non-verbal behavior in inducing public speaking anxiety in immersive exposure (VR). As the main findings, the negative attitudes expressed by a virtual audience impact differently on perceived anxiety in high vs. low anxiety traits. Moreover, participants involved in a pleasant Q&A session (verbal behavior) showed increased physiological activity, which might reflect engagement during the performance.
The datasets presented in this study can be found in online repositories. The names of the repository/repositories and accession number(s) can be found below: https://osf.io/hnwbf/.
The studies involving humans were approved by the University of Milano Bicocca, Department of Psychology. The studies were conducted in accordance with the local legislation and institutional requirements. The participants provided their written informed consent to participate in this study. Written informed consent was obtained from the individual(s) for the publication of any identifiable images or data included in this article.
MG: Conceptualization, Data curation, Formal Analysis, Methodology, Supervision, Writing–original draft, Investigation. IF: Conceptualization, Data curation, Investigation, Writing–original draft. MM: Data curation, Investigation, Writing–original draft. MS: Methodology, Software, Writing–review and editing. MP: Project administration, Writing–review and editing. AM: Resources, Writing–review and editing. AG: Conceptualization, Project administration, Resources, Writing–review and editing.
The author(s) declare that no financial support was received for the research, authorship, and/or publication of this article.
The authors declare that the research was conducted in the absence of any commercial or financial relationships that could be construed as a potential conflict of interest.
All claims expressed in this article are solely those of the authors and do not necessarily represent those of their affiliated organizations, or those of the publisher, the editors and the reviewers. Any product that may be evaluated in this article, or claim that may be made by its manufacturer, is not guaranteed or endorsed by the publisher.
Arsalan, A., and Majid, M. (2021). Human stress classification during public speaking using physiological signals. Comput. Biol. Med. 133, 104377. doi:10.1016/j.compbiomed.2021.104377
Beidel, D. C., Turner, S. M., and Dancu, C. V. (1985). Physiological, cognitive, and behavioral aspects of social anxiety. Behav. Res. Ther. 23, 109–117. doi:10.1016/0005-7967(85)90019-1
Benedek, M., and Kaernbach, C. (2010). A continuous measure of phasic electrodermal activity. J. Neurosci. Methods 190 (1), 80–91.
Blascovich, J. (2002). “A theoretical model of social influence for increasing the utility of collaborative virtual environments,” in Proceedings of the 4th international conference on collaborative virtual environments (CVE '02) (New York, NY, USA: Association for Computing Machinery), 25–30. doi:10.1145/571878.571883
Bodie, G. D. (2010). A racing heart, rattling knees, and ruminative thoughts: defining, explaining, and treating public speaking anxiety. Commun. Educ. 59 (1), 70–105. doi:10.1080/03634520903443849
Bradley, M. M., and Lang, P. J. (1994). Measuring emotion: the self-assessment manikin and the semantic differential. J. Behav. Ther. Exp. psychiatry 25 (1), 49–59. doi:10.1016/0005-7916(94)90063-9
Burgoon, J. K., Birk, T., and Pfau, M. (1990). Nonverbal behaviors, persuasion, and credibility. Hum. Commun. Res. 17 (1), 140–169. doi:10.1111/j.1468-2958.1990.tb00229.x
Carver, C. S., and Scheier, M. F. (1998). On the self-regulation of behavior. New York: Cambridge University Press.
Chollet, M., and Scherer, S. (2017). Perception of virtual audiences. IEEE Comput. Grap. Appl. 37, 50–59. doi:10.1109/mcg.2017.3271465
Chollet, M., Sratou, G., Shapiro, A., Morency, L.-P., and Scherer, S. (2014). “An interactive virtual audience platform for public speaking training,” in Proceedings of the 2014 international conference on autonomous agents and multi-agent systems (Richland, SC: International Foundation for Autonomous Agents and Multiagent Systems), 1657–1658.
Clark, D. M., and Wells, A. (1995). “A cognitive model of social phobia,” in Social phobia: diagnosis, assessment, and treatment. Editors R. G. Heimberg, M. R. Liebowitz, D. A. Hope, and F. R. Schneier (New York: Guilford), 69–93.
Cornwell, B. R., Johnson, L., Berardi, L., and Grillon, C. (2006). Anticipation of public speaking in virtual reality reveals a relationship between trait social anxiety and startle reactivity. Biol. Psych. 59 (7), 664–666. doi:10.1016/j.biopsych.2005.09.015
Croft, R. J., Gonsalvez, C. J., Gander, J., Lechem, L., and Barry, R. J. (2004). Differential relations between heart rate and skin conductance, and public speaking anxiety. J. Behav. Ther. Exp. Psychiatry 35 (3), 259–271. doi:10.1016/J.JBTEP.2004.04.012
El-Yamri, M., Umer, H. M., Alazab, A. M., and Manero, B. (2019). Designing a VR game for public speaking based on speakers features: a case study. Smart Learn. Environ. 6 (1), 12. doi:10.1186/s40561-019-0094-1
Etienne, E., Leclercq, A. L., Remacle, A., and Schyns, M. (2022). “Perception of avatar attitudes in virtual reality,” in Proceedings of the international conference on artificial intelligence and robotics in society. AIRSI2022).
Ferreira Marinho, A. C., Mesquita de Medeiros, A., Cortes Gama, A. C., and Teixeira, L. C. (2017). Fear of public speaking: perception of college students and correlates. J. Voice 31 (1), 127.e7–127.e11. doi:10.1016/J.JVOICE.2015.12.012
Frigione, I., Massetti, G., Girondini, M., Etzi, R., Scurati, G. W., Ferrise, F., et al. (2022). An exploratory study on the effect of virtual environments on cognitive performances and psychophysiological responses. Cyberpsychology, Behav. Soc. Netw. 25 (10), 666–671. doi:10.1089/cyber.2021.0162
Frith, C. D., and Allen, H. A. (1983). The skin conductance orienting response as an index of attention. Biol. Psychol. 17 (1), 27–39. doi:10.1016/0301-0511(83)90064-9
Gallace, A., Ngo, M. K., Sulaitis, J., and Spence, C. (2011). “Exploring the potential and constraints of multisensory presence in virtual reality,” in Multiple sensorial media advances and applications: New developments in MulSeMedia (Pennsylvania, United States: IGI Global), 1–38. doi:10.4018/978-1-60960-821-7.ch001
Gertler, J., Novotny, S., Poppe, A., Chung, Y. S., Gross, J. J., Pearlson, G., et al. (2020). Neural correlates of non-specific skin conductance responses during resting state fMRI. NeuroImage 214, 116721. doi:10.1016/j.neuroimage.2020.116721
Giesen, J. M., and McGlynn, F. D. (1997). Skin conductance and heart-rate responsivity to public speaking imagery among students with high and low self-reported fear: a comparative analysis of “response” definitions. J. Clin. Psychol. 33 (S1), 68–76. doi:10.1002/1097-4679(197701)33:1+<68::aid-jclp2270330114>3.0.co;2-p
Girondini, M., Stefanova, M., Pillan, M., and Gallace, A. (2023a). The effect of previous exposure on virtual reality induced public speaking anxiety: a physiological and behavioral study. Cyberpsychology, Behav. Soc. Netw. 26 (2), 127–133. doi:10.1089/cyber.2022.0121
Girondini, M., Stefanova, M., Pillan, M., and Gallace, A. (2023b). Speaking in front of cartoon avatars: a behavioral and psychophysiological study on how audience design impacts on public speaking anxiety in virtual environments. Int. J. Human-Computer Stud. 179, 103106. doi:10.1016/j.ijhcs.2023.103106
Glemarec, Y., Lugrin, J., Bosser, A., Jackson, A. C., Buche, C., and Latoschik, M. E. (2021). Indifferent or enthusiastic? Virtual audiences animation and perception in virtual reality. Front. Virtual Real. 2. doi:10.3389/frvir.2021.666232
Goodman, W. K., Janson, J., and Wolf, J. M. (2017). Meta-analytical assessment of the effects of protocol variations on cortisol responses to the Trier Social Stress Test. Psychoneuroendocrinology 80, 26–35. doi:10.1016/J.PSYNEUEN.2017.02.030
Harris, S. R., Kemmerling, R. L., and North, M. M. (2002). Brief virtual reality therapy for public speaking anxiety. Cyberpsychology Behav. 5 (6), 543–550. doi:10.1089/109493102321018187
Heimberg, R. G., Mueller, G. P., Holt, C. S., Hope, D. A., and Liebowitz, M. R. (1992). Assessment of anxiety in social interaction and being observed by others: the social interaction anxiety scale and the social phobia scale. Behav. Ther. 23 (1), 53–73. doi:10.1016/s0005-7894(05)80308-9
Higgins, D., Zibrek, K., Cabral, J., Egan, D., and McDonnell, R. (2022). Sympathy for the digital: influence of synthetic voice on affinity, social presence and empathy for photorealistic virtual humans. Comput. Graph. 104, 116–128. doi:10.1016/j.cag.2022.03.009
Higuera-Trujillo, J. L., López-Tarruella Maldonado, J., and Llinares Millán, C. (2017). Psychological and physiological human responses to simulated and real environments: a comparison between Photographs, 360° Panoramas, and Virtual Reality. Appl. Ergon. 65, 398–409. doi:10.1016/J.APERGO.2017.05.006
Hoffman, H. G., Richards, T. L., Coda, B., Bills, A. R., Blough, D., Richards, A. L., et al. (2004). Modulation of thermal pain-related brain activity with virtual reality: evidence from fMRI. Neuroreport 15 (8), 1245–1248. doi:10.1097/01.wnr.0000127826.73576.91
Hofmann, S. G., Heinrichs, N., and Moscovitch, D. A. (2004). The nature and expression of social phobia: toward a new classification. Clin. Psychol. Rev. 24 (7), 769–797. doi:10.1016/j.cpr.2004.07.004
Jacobs, S. C., Friedman, R., Parker, J. D., Tofler, G. H., Jimenez, A. H., Muller, J. E., et al. (1994). Use of skin conductance changes during mental stress testing as an index of autonomic arousal in cardiovascular research. Am. Heart J. 128 (6), 1170–1177. doi:10.1016/0002-8703(94)90748-x
Kang, N., Brinkman, W.-P., Birna van Riemsdijk, M., and Neerincx, M. (2016). The design of virtual audiences: noticeable and recognizable behavioral styles. Comput. Hum. Behav. 55, 680–694. doi:10.1016/j.chb.2015.10.008
Kelly, O., Matheson, K., Martinez, A., Merali, Z., and Anisman, H. (2007). Psychosocial stress evoked by a virtual audience: relation to neuroendocrine activity. CyberPsychology Behav. 10 (5), 655–662. doi:10.1089/cpb.2007.9973
Kennedy, R. S., Lane, N. E., Berbaum, K. S., and Lilienthal, M. G. (1993). Simulator sickness questionnaire: an enhanced method for quantifying simulator sickness. Int. J. Aviat. Psychol. 3 (3), 203–220. doi:10.1207/s15327108ijap0303_3
Kensinger, E. A. (2004). Remembering emotional experiences: the contribution of valence and arousal. Rev. Neurosci. 15 (4), 241–251. doi:10.1515/revneuro.2004.15.4.241
Kroczek, L. O., and Mühlberger, A. (2023). Public speaking training in front of a supportive audience in Virtual Reality improves performance in real-life. Sci. Rep. 13 (1), 13968. doi:10.1038/s41598-023-41155-9
Lanier, J. (2017). Dawn of the new everything: encounters with reality and virtual reality. Henry Holt and Company.
Laukka, P., Linnman, C., Åhs, F., Pissiota, A., Frans, Ö., Faria, V., et al. (2008). In a nervous voice: acoustic analysis and perception of anxiety in social phobics’ speech. J. Nonverbal Behav. 32, 195–214. doi:10.1007/s10919-008-0055-9
McCroskey, J. C. (1970). Measures of communication-bound anxiety. Speech Monogr. 37 (4), 269–277. doi:10.1080/03637757009375677
McCroskey, J. C. (1977). Oral communication apprehension. A Summ. recent theory Res. 4 (1), 78–96. doi:10.1111/j.1468-2958.1977.tb00599.x
Montana, J. I., Matamala-Gomez, M., Maisto, M., Mavrodiev, P. A., Cavalera, C. M., Diana, B., et al. (2020). The benefits of emotion regulation interventions in virtual reality for the improvement of wellbeing in adults and older adults: a systematic review. J. Clin. Med. 9 (2), 500. doi:10.3390/jcm9020500
Mostajeran, F., Balci, M. B., Steinicke, F., Kühn, S., and Gallinat, J. (2020). “The effects of virtual audience size on social anxiety during public speaking,” in The proceeding of the 2020 IEEE Conference on Virtual Reality and 3D User Interfaces (VR), Atlanta, GA, USA, March 2020 (IEEE), 303–312.
Nikula, R. (1991). Psychological correlates of nonspecific skin conductance responses. Psychophysiology 28 (1), 86–90. doi:10.1111/j.1469-8986.1991.tb03392.x
Niles, A. N., Craske, M. G., Lieberman, M. D., and Hur, C. (2015). Affect labeling enhances exposure effectiveness for public speaking anxiety. Behav. Res. Ther. 68, 27–36. doi:10.1016/j.brat.2015.03.004
Norman, D. (2005). Emotional design: why we love (or hate) everyday things. New York, NY: Basic Books.
Norman, G. (2010). Likert scales, levels of measurement and the "laws" of statistics. Adv. Health Sci. Educ. 15, 625–632. doi:10.1007/s10459-010-9222-y
North, M. M., North, S. M., and Coble, J. R. (1998). Virtual reality therapy: an effective treatment for the fear of public speaking. Int. J. Virtual Real. 3, 1–6. doi:10.20870/IJVR.1998.3.3.2625
Nummenmaa, L., Glerean, E., Hari, R., and Hietanen, J. K. (2014). Bodily maps of emotions. Proc. Natl. Acad. Sci. U. S. A. 111 (2), 646–651. doi:10.1073/pnas.1321664111
Owens, M. E., and Beidel, D. C. (2015). Can virtual reality effectively elicit distress associated with Social Anxiety Disorder? J. Psychopathol. Behav. Assess. 37 (2), 296–305. doi:10.1007/s10862-014-9454-x
Pavett, C. M. (2016). Evaluation of the impact of feedback on performance and motivation. Hum. Relat. 36 (7), 641–654. doi:10.1177/001872678303600704
Perowne, S., and Mansell, W. (2002). Social anxiety, self-focused attention, and the differentiation of negative, neutral, and positive audience members based on their non-verbal behaviors. Behav. Cognitive Psychotherapy 30 (1), 11–23. doi:10.1017/s1352465802001030
Pertaub, D. P., Slater, M., and Barker, C. (2001). An experiment on fear of public speaking in virtual reality. Stud. Health Technol. Inf. 81, 372–378. doi:10.1037/e705412011-025
Powers, K. L., Brooks, P. J., Aldrich, N. J., Palladino, M. A., and Alfieri, L. (2013). Effects of video-game play on information processing: a meta-analytic investigation. Psychonomic Bull. Rev. 20, 1055–1079. doi:10.3758/s13423-013-0418-z
Raja, F. (2017). Anxiety level in students of public speaking: causes and remedies. J. Educ. Educ. Dev. 4 (1), 94–110. doi:10.22555/joeed.v4i1.1001
Rapee, R. M., and Heimberg, R. G. (1997). A cognitive–behavioral model of anxiety in social phobia. Behav. Res. Ther. 35, 741–756. doi:10.1016/s0005-7967(97)00022-3
Reeves, B., and Nass, C. (1996). The media equation: how people treat computers, television, and new media like real people and places. Cambridge, United Kingdom
Riva, G. (2009). Virtual reality: an experiential tool for clinical psychology. Br. J. Guid. Couns. 37, 337–345. doi:10.1080/03069880902957056
Slater, M., Pertaub, D. P., Barker, C., and Clark, D. M. (2006). An experimental study on fear of public speaking using a virtual environment. Cyberpsychol Behav. 9 (5), 627–633. doi:10.1089/cpb.2006.9.627
Slater, M., Pertaub, D. P., and Steed, A. (1999). Public speaking in virtual reality: facing an audience of avatars. IEEE Comput. Graph Appl. 19 (2), 6–9. doi:10.1109/38.749116
Spielberger, C. D., Gonzalez-Reigosa, F., Martinez-Urrutia, A., Natalicio, L. F., and Natalicio, D. S. (1971). The state-trait anxiety inventory. Revista Interamericana de Psicologia/Interamerican J. Psychol. 5 (3 & 4).
Stein, M., and Chavira, D. (1998). Subtypes of social phobia and comorbidity with depression and other anxiety disorders. J. Affect. Disord. 50, 11–16. doi:10.1016/s0165-0327(98)00092-5
Takac, M., Collett, J., Blom, K. J., Conduit, R., Rehm, I., and De Foe, A. (2019). Public speaking anxiety decreases within repeated virtual reality training sessions. PLoS One 14 (5), e0216288. doi:10.1371/journal.pone.0216288
Turpin, G., Grandfield, T., and Fink, G. (2009). “Electrodermal activity,” in Stress science: neuroendocrinology, 313–316.
Keywords: virtual reality, public speaking anxiety, audience behavior, verbal and non-verbal behavior, physiological arousal
Citation: Girondini M, Frigione I, Marra M, Stefanova M, Pillan M, Maravita A and Gallace A (2024) Decoupling the role of verbal and non-verbal audience behavior on public speaking anxiety in virtual reality using behavioral and psychological measures. Front. Virtual Real. 5:1347102. doi: 10.3389/frvir.2024.1347102
Received: 30 November 2023; Accepted: 05 February 2024;
Published: 19 March 2024.
Edited by:
Domna Banakou, New York University Abu Dhabi, United Arab EmiratesReviewed by:
Marta Mondellini, National Research Council (CNR), ItalyCopyright © 2024 Girondini, Frigione, Marra, Stefanova, Pillan, Maravita and Gallace. This is an open-access article distributed under the terms of the Creative Commons Attribution License (CC BY). The use, distribution or reproduction in other forums is permitted, provided the original author(s) and the copyright owner(s) are credited and that the original publication in this journal is cited, in accordance with accepted academic practice. No use, distribution or reproduction is permitted which does not comply with these terms.
*Correspondence: Matteo Girondini, bS5naXJvbmRpbmlAY2FtcHVzLnVuaW1pYi5pdA==
†These authors have contributed equally to this work and share first authorship
Disclaimer: All claims expressed in this article are solely those of the authors and do not necessarily represent those of their affiliated organizations, or those of the publisher, the editors and the reviewers. Any product that may be evaluated in this article or claim that may be made by its manufacturer is not guaranteed or endorsed by the publisher.
Research integrity at Frontiers
Learn more about the work of our research integrity team to safeguard the quality of each article we publish.