- 1ITIS, Luxembourg Institute of Science and Technology, Esch-Sur-Alzette, Luxembourg
- 2MRT Department, Luxembourg Institute of Science and Technology, Esch-sur-Alzette, Luxembourg
This paper presents a proof-of-concept Augmented Reality (AR) system known as “SMARTLab” for safety training in hazardous material science laboratories. The paper contains an overview of the design rationale, development, methodology, and user study. Participants of the user study were domain experts (i.e., actual lab users in a material science research department, n = 13), and the evaluation used a questionnaire and free-form interview responses. The participants undertook a virtual lab experiment, designed in collaboration with a domain expert. While using the AR environment, they were accompanied by a virtual assistant. The user study provides preliminary findings about the impact of multiple dimensions, such as Performance Expectancy, Emotional Reactivity, and Spatial Presence, on SMARTLab acceptance by analyzing their influence on the Behavioral Intention dimension. The findings indicate that users find the approach useful and that they would consider using such a system. Quantitative and qualitative analysis of the SMARTLab assessment data suggests that a) AR-based training is a potential solution for laboratory safety training without the risk of real-world hazards, b) realism remains an important property for some aspects such as fluid dynamics and experimental procedure, and c) use of a virtual assistant is welcome and provides no sense of discomfort or unease. Furthermore, the study recommends the use of AR assistance tools (a virtual assistant, an attention funnel, and an in-situ arrow) to improve usability and make the training experience more user-friendly.
1 Introduction
Laboratory accidents have detrimental effects on finances, equipment, time, and most importantly, the health and safety of those working there. The motivation of our work focuses on the longer term, with the aim of looking at ways to reduce accidents in chemical laboratories. For example, in the US alone, the U.S. Chemical Safety and Hazard Investigation Board1, identified 224 chemical accidents in US public and industrial laboratories in the period between 05.2020 and 12.2022, causing 126 serious injuries and 31 fatalities. Additionally, a recent report revealed that 67.1% of the chemical accidents in South Korea from 2008 to 2018 were caused by human error Jung et al. (2020). These human failures can occur due to a lack of competence in the usage of chemical substances with hazardous properties (e.g., flammability, explosivity, and toxicity). Therefore, the prevention of chemical risks in laboratories using efficient training methods is crucial. An analysis of 169 events collected from the French database ARIA (Analysis, Research, and Information on Accidents) showed that the causes were mainly related to operator errors Dakkoune et al. (2018). Bai et al. (2022) gathered 110 publicly reported university laboratory accidents in mainland China to investigate the proximate causes of the accidents and identify potential deficiencies existing in the current safety management of laboratories. They found that human factors were the most common cause and that the training element was a critical competency (with respect to deficiencies) in laboratory safety management. Hence, human performance is critical to ensure safety and health in hazardous chemical settings.
Therefore, developing effective training methods to improve safety and reduce accidents and doing this without the risk of real-world hazards, such as exposure to dangerous chemicals, explosive materials, and toxic gases are important challenges. In order to address these challenges, this paper presents a system known as SMARTLab (see Figure 1). SMARTLab is a proof-of-concept Augmented Reality (AR) system that provides a training scenario for material science laboratory users. It builds upon the growing trend toward using novel technologies such as Virtual Reality (VR) or AR as a means to improve human performance, providing realistic simulations, enhanced learning experiences, and personalized learning, see Kaplan et al. (2021).
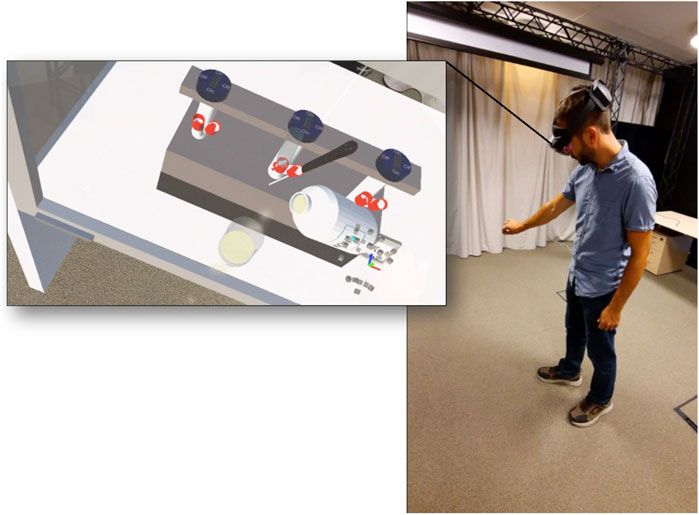
Figure 1. The SMARTLab training tool for material science laboratory safety demonstrating a chemical manipulation through an AR-HMD.
At the time of writing, AR technologies are gaining popularity, and despite significant improvements, for example, between Microsoft HoloLens 1 and HoloLens 2, they remain hindered by limitations such as field-of-view. While improvements will continue, a challenge is to assess whether such technologies can provide an acceptable level of user experience. Furthermore, while it is possible to stick to the idea of simply implementing a scenario that mirrors a laboratory, a key point is to understand how assistance approaches, such as a virtual assistant (sometimes referred to as a virtual agent), an attention funnel, or an in-situ arrow, enhance the user experience. As a result, this paper focuses on assessing these aspects rather than the HoloLens platform itself although it is acknowledged that the technical aspects of HoloLens will have an impact.
SMARTLab was designed and evaluated by domain experts, who work in a materials science laboratory and who are considering using AR training techniques. While in the long-run the emphasis should be on learning effects, the focus of this paper is on exploring the user experience aspects of SMARTLab, namely, user acceptance, sense of spatial presence, attention, and realism. These are assessed through a questionnaire, while richer free-form responses are also collected and analyzed.
This paper starts with a review of the related literature, an overview of the requirements capture process, and the chosen scenario. The implementation and testing of the scenario are then explained and results are presented, followed by a discussion and conclusion.
2 Background
Carmigniani et al. (2011) state that Augmented Reality (AR) provides a “real-time direct or indirect view of a physical real-world environment that has been enhanced/augmented by adding virtual computer-generated information to it”. Other works such as that of Azuma (1997), include the concept of limited interaction as part of the definition of AR, but this is not universal in the literature. While Microsoft HoloLens is often referred to as Mixed Reality, we have chosen to use the term Augmented Reality. This is due to the fact that SMARTLab only overlays sound and graphics into the real world. This definition is drawn from Milgram et al. (1995), who defined Augmented Reality as part of the Mixed Reality (MR) range of technologies. Furthermore, SMARTLab does not connect to any physical objects. To maintain consistency, the participant questionnaire also used the term Augmented Reality.This section provides a background for Augmented Reality Head-Mounted Displays (AR-HMDs) as training tools in different fields, as well as the state of the art related to the paper’s contributions.
2.1 Training with AR-HMDs
The integration of AR into training has become a notable area of interest; along with other immersive technologies, it can be used to enhance learning experiences in various fields. Junaini et al. (2022) confirm that AR applications can potentially be used for Occupational Safety and Health (OSH) training purposes in the context of regular displays, i.e., not HMDs.
With rapid developments in consumer-level head-mounted displays and computer graphics, HMD-based AR such as Meta Quest Pro or Microsoft HoloLens (which is used in our study) has the potential to improve training. Furthermore, they avoid the isolation effect and have negligible symptoms of motion sickness when compared to virtual reality Vovk et al. (2018). The lack of an isolation effect and visibility of the real world (and possibly physical hazards) in AR allows SMARTLab to be used in a wider range of locations, allowing for more flexibility in running training sessions. Kim et al. (2018a) reviewed AR technologies and applications from the last 2 decades and identified maintenance, simulation, and training in industrial, military, and medical fields as the most popular AR application topics. The overarching assumption behind these applications is that the real-time situated visual guidance can potentially augment the users’ capabilities and subsequently improve the users’ skills. For example, Yamaguchi et al. (2020) suggest an AR training system for assembly and disassembly procedures using a complete and detailed AR instructional 3D tutorial. They found that AR instructions caused less mental effort and cognitive load than common video tutorials. Another systematic review by Avila-Garzon et al. (2021) indicates that the current emerging and trending research topics in AR in education include special educational needs, Industry 4.0, storytelling, 3D printing, mobile applications, and higher education.
Training using HMD-based AR has grown in popularity. This is in part due to the rise of Microsoft HoloLens, which provides support for gesture recognition, reasonable accurate tracking, and graphics of an acceptable quality, despite still suffering from field-of-view limitations. González et al. (2019) evaluated participants performance and usability between AR-HMDs and a desktop interface for Scenario-Based Training (SBT). They found no significant difference between the two interfaces in the time taken to accomplish tasks. However, the desktop interface was preferred by the participants. The work by Zhu et al. (2018) proposed an application based on AR to teach biochemistry laboratory safety as an alternative to traditional lecture-based training methods. The application was used for laboratory safety training and the participants were asked to find the locations of items in the laboratory and move to them while listening to a holographic narrator. The results found that AR training had a learning gain effect similar to a lecture. However, the students enjoyed the AR course more than the traditional lecture-based program. Werrlich et al. (2018) conducted an experiment with 30 trainees to demonstrate the difference in terms of speed and mistakes between AR-based and paper-based training. The results showed that after completing an entire scenario using AR-HMDs, people were 62.3% more accurate and 32.14% less frustrated than in the paper-based training group. Recently, a study proposed by De Micheli et al. (2022) compared the performance of 12 students who followed a course using AR-HMDs to that of 12 students using in-person learning. The objective of the course was to have students learn how to build a microfluidic device with an understanding of fluid phenomena. They observed a greater building of intuition and engagement in students with the AR-HMDs compared to that for in-person learning.
Rather than comparing the learning effect of AR versus traditional learning approaches, this paper focused on the suitability of using AR for training in a material science laboratory. It focused on less explored aspects such as the AR assistance tools and realistic fluid simulation.
2.2 Acceptability of augmented reality as a training tool
Various technology acceptance models have been developed to explain and predict technology use, with the most commonly used being the Technology Acceptance Model (TAM) Davis (1989). Venkatesh et al. (2003) extended TAM by including other decision-making theories and proposed a new framework called UTAUT. In 2012, UTAUT was expanded to UTAUT2 by Venkatesh et al. (2012), who identified hedonic motivation, price, and habit as additional key constructs to be integrated. Different studies have investigated the influential factors in adopting AR-HMDs, such as Kalantari and Rauschnabel (2018), which found that consumer’s adoption decision for Microsoft AR-HMDs is driven by various expected benefits including usefulness, ease of use, and image quality. However, hedonic benefits were not found to influence the adoption intention, as also confirmed by Stock et al. (2016) who found an indirect negative influence of perceived health risk on the Behavioral Intention to use AR-HMDs. An AR-based dance training system proposed by Iqbal and Sidhu (2022) was evaluated using the Technology Acceptance Model (TAM). The obtained results indicate a general acceptance of the suggested system among users who are interested in exploring new technology in this domain. Wild et al. (2017) collected items from existing technology acceptance models (TAM, UTAUT, etc.) to build a model of what derives acceptance and use of AR technology in the context of training on operating procedures as well as in maintenance and repair operations in aviation and space. The study shows that respondents enjoy and look forward to using AR technologies due to finding them intuitive and easy to learn to use. The authors stated that “The technologies are still seen as forerunner tools, with some fear of problems of integration with existing systems”.
In the work of Papakostas et al. (2022), the integration of AR in welding training was evaluated by 200 trainees using a modified TAM, extending it by two external variables (perceived enjoyment and system quality). The intention to use AR in welding training was positively influenced directly by system quality and perceived ease of use. Although the simplicity of TAM is considered a key strength of the model, it has also been widely criticized for ignoring different aspects of decision-making across different technologies, Bagozzi (2007). Therefore, this paper considers UTAUT2, which is extended from TAM and UTAUT, to cover more dimensions such as Hedonic Motivation that could affect SMARTLab adoption. Moreover, to the best of our knowledge, the acceptance of using AR for safety training in a material science laboratory use case has not yet been explored. Per Zhu et al. (2018) and Liu et al. (2019), further investigation on adopting AR-HMD-based safety training tools, which mitigate the risks associated with real-world hazards, and on their benefits compared to traditional learning methods is required. Therefore, this work suggests an AR acceptance Model adapted from the UTAUT2 model’s ability to predict the acceptance of an AR application. To realize this, we include the following dimensions: Behavioral Intentions, Effort Expectancy, Performance Expectancy, Emotional Reactivity, Hedonic Motivation, Personal Innovativeness, and Spatial Presence. These dimensions are taken from UTAUT2 Venkatesh et al. (2012) and other sources Mousas et al. (2018a, 2021), Agarwal and Karahanna (2000), and Vorderer et al. (2004) as illustrated in Table 1. Additionally, new dimensions (Attention and Realism) are added to our proposed AR acceptance model, as described in Section 4.3.1.
2.3 AR assistance tools
Different tools are proposed within applications of AR-HMDs to enhance the perception of virtual objects and to improve the understanding of tasks. AR assistance tools lead also to a reduction in the time needed for visual search. Therefore, AR increases task attention and simplifies keeping track of errors such as incorrectly selected virtual objects, see Werrlich et al. (2018) and de Melo et al. (2020). Consequently, to improve usability and acceptance, this paper evaluates, via the Attention and Emotional Reactivity dimensions described in section 4.3.1, the following three AR assistance tools that are embedded in SMARTLab, see also Figure 2.
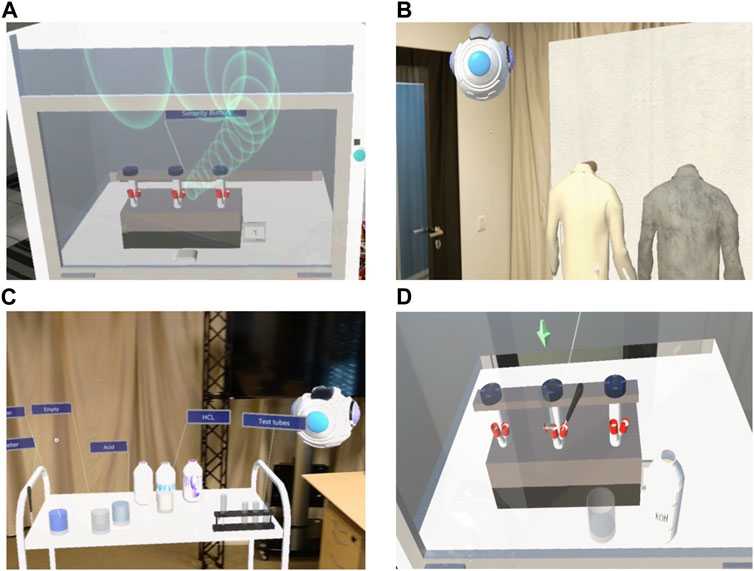
Figure 2. (A): Attention funnel (B) and (C): Virtual assistant: simple flying robot (D): In-situ arrow.
2.3.1 Virtual assistant
In many AR-based applications related to treatment and rehabilitation Bigagli (2022) and training de Melo et al. (2020), a virtual assistant is used to help the user accomplish a variety of goals. Moreover, many of these applications demand that the virtual assistant look, move, behave, or communicate effectively no matter their level of realism. Furthermore, when friendliness is explicitly modeled, the Friendliness of Virtual Agents (FVA) shows a statistically significant improvement in terms of the perceived friendliness and social presence of a user, compared to an agent without friendliness modeling Randhavane et al. (2019). The work by de Melo et al. (2020) compared performance at a desert survival task using HoloLens with embodied assistance, voice-only assistance, and no assistance. They found that both assistant conditions led to higher performance compared to the no assistant condition. Moreover, the embodied assistant achieved this with less cognitive burden on the decision-maker than the voice assistant.
To improve the global understanding of the experience, a virtual assistant has been included in SMARTLab to guide the trainee during the training session. The assistant explains each task one by one and interacts with the trainee. Kim et al. (2018b) investigated how visual embodiment and social behaviors influence the perception of Intelligent Virtual Agents to determine whether or not a digital assistant requires a body. The results indicated that imbuing an agent with a visual body in AR and natural social behaviors can increase the user’s confidence in the agent’s ability to influence the real world. However, creating a complete virtual assistant is a complicated task that includes aspects such as behavior modeling and animation. Indeed, having a humanoid virtual assistant with bad animation or low texture could lead to the uncanny valley effect. Pollick (2009) describes the uncanny valley as something equivocal, as it depends on people’s perception. However, getting close to this valley, by using low texture 3D models or animations that are bad or incomplete, can cause discomfort, which leads to a reduction in the overall level of user acceptance. In SMARTLab, we have chosen to represent the virtual assistant as a simple flying robot providing vocal guidance, thereby avoiding the uncanny valley.
2.3.2 Attention funnel
Identification and localization of virtual/real items or places can be supported using an attention funnel Biocca et al. (2006) which is also referred to as an augmented tunnel Werrlich et al. (2018). This is an AR interface technique that interactively guides the attention of a user to any object, person, or place in space. Biocca et al. (2006) validated the use of an attention funnel in an experiment where 14 participants were asked to undertake scenarios where the main goal was to find objects in the environment.
They found that the attention funnel increased the consistency of the user’s search by 65%, decreased the visual search time by 22%, and decreased the mental workload by 18%. SMARTLab uses an augmented tunnel to attract trainee attention to the positions of virtual objects that are required to complete the tasks.
2.3.3 In-situ arrow
This user interface feature for attention guiding highlights a target object or location using an arrow that hovers above the target object. In qualitative feedback from the work of Blattgerste and Pfeiffer (2020), an in-situ arrow that shows the physical position in a room was positively received. Gruenefeld et al. (2018) propose pointing toward out-of-view objects on AR devices using a flying arrow. This method is integrated into Microsoft HoloLens2. In SMARTLab, the selection of objects in a virtual material science laboratory is guided using an animated arrow moving up and down to focus the trainee’s attention. Evaluation of the advantages of an in-situ arrow is conducted via the Attention dimension (see Section 4.3.1).
2.4 Realism of liquid simulation in AR application
Liquid Simulation (LS) is an important component in most AR-HMD-based safety training tools, and within materials science laboratories, manipulating liquids is a common task. LS is an example of Computational Fluid Dynamics (CFD) which is a very active research field and many efficient LS algorithms have been developed, see Macklin and Müller (2013). However, applying such CFD/LS algorithms on an AR device such as the Microsoft HoloLens is still a major challenge due to the capability of such an AR device to perform these algorithms in real-time, with an acceptable frame rate. The work by Bahnmüller et al. (2021) presented a Microsoft HoloLens framework to visualize a large set of particle distributions based on their position to understand the behavior of aerosol distribution. This method displays nearly 80,000 moving particles at an average rate of 35 frame seconds using the Unity3D particle system. Asgary et al. (2020) used the Microsoft HoloLens to visualize volcanic eruptions via an application called HoloVulcano which uses the Unity particle system to visualize normal degassing.Works by Zhu et al. (2019) and Mourtzis et al. (2022) proposed applying cloud-computing to reduce the amount of computation required by CFD on AR devices. However, as described in Cheng et al. (2020), despite current trends toward cloud computing and cloud storage, support is still lacking on AR devices. This study uses Unity particle systems and a texture shader to simulate liquid. Such a particle system has demonstrated good performance (30 FPS) on HoloLens (v1) Koenig et al. (2021). Rather than doing an evaluation of LS performance on AR-HMDs, this paper examines the visual representation of LS via the Realism dimension, as described in detail in Section 4.3.1. Recent work by Itoh et al. (2021) highlights the challenges of visual realism and underscores that, besides a limited Field of View (FoV), several other factors, such as light control, contribute to a convincing augmentation of the physical environment. In this study, we analyze the influence of the realism dimension on the behavioral intention to use SMARTLab.
3 Proposition
3.1 Requirements capture and design
Our research organization has state-of-the-art materials science laboratories where hazardous chemicals and equipment are used on a daily basis. Despite an excellent safety record, there is a desire to ensure that current and new staff can access safety training regularly. Furthermore, they have an interest in exploring new learning techniques, such as using AR.
In order to identify a suitable scenario, we shortlisted a range of scenarios and tasks. In each of these scenarios, we drew up a list of required interactions, with real and virtual objects that were needed to fulfill the learning objectives. We then conducted an initial technical feasibility assessment looking at the chosen hardware (HoloLens 2) and explored if we should connect to real-world external equipment. In the end, it was decided that we would not do so and instead focus on building 3D virtual models of the required scenario. A further discussion took place to decide on a final scenario and develop more detailed task breakdowns.
A visit to the laboratory facility was undertaken, and during this time, the team was walked through the steps of the chosen scenario, photographs of the equipment used were taken, and careful consideration was given to challenges that may arise in a real lab setting. One identified challenge was that a lot of equipment may be available in the drawers or on the tables, and the trainee must choose the right item. Often, many items may look correct, but a relatively small difference may rule out their use. Based on the visit, a final scenario plan was drafted, including revisions to the tasks and storyboard. This approach allowed us to then agree on which parts would finally be implemented in the system, keeping in mind the relevant learning objectives and the risks people may face. In particular, we ensured there were a range of false options available when selecting equipment.
3.2 Scenario
Mixing hydrochloric acid (chemical formula HCI) with potassium hydroxide (chemical formula KOH) was selected as a scenario because it is a very common task. It is also performed using a Chemical Reactor Station (CRS) which requires a sequence of correct steps to be followed, see Figure 3. As a result, two main stages are involved:
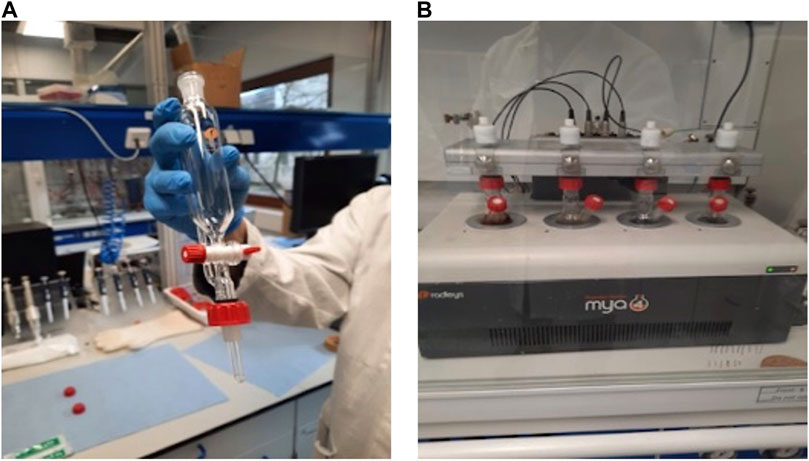
Figure 3. (A, B) are two pictures captured from our chemical laboratory and represent the Solid Addition Funnel and MYA 4 Reaction Station.
1) Firstly, the trainee must select the correct equipment before entering a materials science laboratory. During this stage, the virtual assistant asks the participant to select (using a grabbing action) the correct virtual items. These consist of a lab coat, safety glasses, and gloves chosen from among a range of objects such as a pair of boots, a torn lab coat, and cracked glasses. Once the correct objects are selected, the participant can use a voice command or press a virtual button to move on to the next stage.
2) The laboratory stage begins with the virtual assistant vocally listing all of the objects in the virtual scene: a Fumehood, the CRS which already contains the HCI, temperature probes, solid addition funnels, vessels, bottles with multiple chemical substances (TRIVOREX, KOH, and Reverix), an empty beaker, a beaker of acid, and a beaker of water. It is up to the participant to choose the necessary pieces of equipment to mix HCI with KOH. These are: a) an empty beaker, b) solid addition funnels, c) temperature probes, and d) the KOH bottle. The participant is then vocally prompted by the virtual assistant to perform the following tasks: 1) turning on the Fumehood which contains the CRS, 2) opening the sash, 3) placing the thermometer and solid addition funnels inside the CRS as well as an empty beaker and the KOH bottle inside the Fumehood, 4) verifying the CRS security buttons, 5) pouring KOH from the bottle into the empty beaker and then from the beaker to the solid addition funnels, and 6) turning on the stopper of the solid addition funnels to pour KOH inside the CRS with two modes, fast and slow.
When the experience ends, the Time To Completion (TTC) and Number of Errors (NE) that occurred are displayed. TTC is calculated for the entire task and includes the time taken by the virtual assistant to provide explanations. Selecting incorrect equipment before entering the laboratory, as well as an inappropriate object for mixing KOH with HCI are considered errors, as is performing the tasks in the wrong order (e.g., opening the sash before turning on the Fumehood).
3.3 Technical implementation
The system was implemented using a Microsoft HoloLens 2 using the Unity3D development environment. Due to device limitations, standard fluid dynamic libraries such as Obi Fluid3 could not be used. Instead, we used a particle system implemented in Unity3D to simulate any liquid outside of the containers. Liquid levels inside containers, such as beakers or bottles, are rendered using a custom-developed shader. This shader updates the level of liquid inside the container by adjusting the height size of an input texture. When participants are performing the tasks within SMARTLab, the purpose is that they interact correctly and efficiently with the core scenario, that items are not hidden, and participants should not have to spend unnecessary time searching for them. The previously described AR assistance tools (the virtual assistant, attention funnel, and in-situ arrow) support this.
4 Materials and methodology
4.1 Participants
The participants were recruited from the materials science department of the authors’ research institute. Participants completed informed consent forms prior to taking part. The final experiment cohort contained 13 participants, 10 of whom were men and 3 women, with ages varying from 27 to 51 (M = 37, SD = 7.2).
The participants were asked to indicate their educational background and provide general feedback about the experience at the end of the experiment. All participants declared that they had no prior experience with head-mounted display technology (AR or VR), and two participants had already used AR on a mobile phone. As mentioned previously, the participants are domain experts and actual lab users. Therefore, all the participants have knowledge about safety procedures through a lecture-based training called “Visa entry”, provided by our institute for all employees working in laboratories.
4.2 Experimental conditions
Three evaluators conducted the experimental test; however, only one evaluator was responsible for each participant’s experience. Two rooms were reserved at our institute, and two experiments were conducted simultaneously. The experiences were spread over 2 weeks depending on the availability of participants. The two rooms had the same configuration, each containing only a chair and a table that participants used to complete the survey at the end of the experiment. The study began with an introduction and project description given by the evaluator. Next, the participant was asked to wear the HoloLens and perform some practice gesture interactions. Afterward, they were asked to start the SMARTLab application from the HoloLens menu. While the structure of the experiment had been explained to the participant, the specific scenario tasks that the participant would undertake had not been discussed. The participants were informed that they were allowed to quit the experiment at any point without any repercussions. Once the participant finished using the SMARTLAB application, the evaluator provided a paper-based survey to the participant and left them alone in the room to complete it. The evaluator remained outside the room until the participant finished their responses. The total duration of the experiment was on average 40 min. Ten participants performed the entire SMARTLab scenario once. Three participants had to restart the application due to problems with the gesture recognition which caused the device HoloLens to freeze. However, all participants (n = 13) provided answers for the questionnaire explained in the next section.
4.3 Measurements and ratings
To assess and evaluate SMARTLab, questionnaires, acceptance models, and free form written responses were explored. However, Task Completion Time (TCT) and Error Rate were excluded from the evaluation, see section 4.5 for details.
4.3.1 Questionnaire
We used nine independent dimensions to evaluate the SMARTLab training tool. The questionnaires are illustrated in the Supplementary Material. We have selected these dimensions from multiple reference works and developed SMARTLab-specific ones where there was no suitable pre-existing dimension in the literature (see Table 1). The first four dimensions were related to the acceptability of the system.
The acceptability of the AR environment is measured with 13 items derived from the UTAUT2 questionnaire Venkatesh et al. (2012) with a 5-point Likert scale from 1 “Strongly disagree” to 5 “Strongly agree”. The UTAUT2 model in the context of the SMARTLab training tool provides an understanding of the different drivers that influence the behavioral intention to use SMARTLab. Social Influence, Facilitating Conditions, Habit, and Price value are not evaluated as AR is still considered a new technology, especially in a training context, and the goal of SMARTLab assessment is to achieve TRL 3 which refers to “Proof of concept demonstrated”. We used four dimensions for the assessment of SMARTLab: 1) Performance Expectancy (PE), 2) Effort Expectancy (EE), 3) Hedonic Motivation (HM), and 4) Behavioral Intention (BI) as described by Venkatesh et al. (2012). Performance Expectancy relates to whether an individual feels that the system will help them fulfill their work goals. Effort Expectancy relates to the amount of effort required in order for them to complete their goals and is similar to ease of use. Hedonic Motivation relates to the perceived fun or enjoyment the user obtains while using the system. Behavioral Intentions relate to the tendency to use SMARTLab.
The next dimension is Personal Innovativeness (PI) with four questions and a 5-point Likert scale from 1 “Strongly disagree” to 5 “Strongly agree”. The questionnaire is derived from Agarwal and Karahanna (2000) and adapted for the SMARTLab evaluation. This dimension relates to the interest of the user in new technologies and using AR for training.
Mousas et al. (2018a, 2021) propose eight items as part of an Emotional Reactivity (ER) questionnaire to examine the influence of appearance and motion of virtual object characters on participants’ emotional reaction. This was adapted to explore the appearance and motion of the virtual assistant in the scene. One question (“Would you feel uneasy if this virtual character tried to touch you?”) was eliminated from the evaluation questionnaire as even in future versions we would not implement such behavior. Question responses were given as suggested by Mousas et al. (2018a, 2021) via a 7-point Likert scale ranging from “Not uncomfortable at all” to “Totally Uncomfortable” (Q1,Q2,Q3), from “Strongly disagree” to “Strongly agree” (Q4,Q5,Q7), and from “Totally uneasy” to “Not uneasy at all” (Q6). The data derived from ER dimensions is reversed and normalized such that the nine dimensions have the same sub-scale from one to five.
The Spatial Presence (SP) dimension is an important factor in AR applications to evaluate the feeling of “being there” when the participant works with SMARTLab. This is due to the fact that a key part of working in a laboratory is the feeling of risk and danger when working with chemicals. Therefore, we would assume that if there is a higher level of feeling of “being there” in a lab setting, they would behave in a similar manner to how they would in a real laboratory. The dimension contains four questions derived from Vorderer et al. (2004) and adapted for the SMARTLab assessment. We proposed four different items through Attention (At) to evaluate the AR assistance tools: the attention funnel and in-situ arrow, which aimed to guide the user during the training session. The virtual assistant was evaluated through the Emotional Reactivity dimension. We evaluated the Realism (Re) of a) the experimental procedure in general and b) the liquid simulation of the SMARTLab training tool by proposing three different items. Moreover, SP, At, and Re questionnaires are provided with a 5-point Likert scale from 1 (Strongly disagree) to 5 (Totally agree).
Finally, we assessed SMARTLab for simulator sickness, determining cybersickness levels. The seven assessment items were adapted from the Simulator Sickness Questionnaire (SSQ), Bouchard et al. (2007) and Kennedy et al. (1993), with a 4-point scale, from 1 (no sign) to 4 (severe). SSQ was analyzed separately from the nine dimensions mentioned previously; therefore, the sub-scale (1–4) was kept without normalization. SSQ was comprised of two factors: oculomotor and nausea. The oculomotor factors were fatigue, headache, eyestrain, difficulty focusing, difficulty concentrating, and blurred vision. The nausea factor was general discomfort. Nine items considered inappropriate for the training scenario were eliminated: increased salivation, stomach awareness, burping, sweating, nausea, fullness of the head, dizziness with eyes open, dizziness with eyes closed, and vertigo.
4.3.2 SMARTLab acceptance model and hypotheses
We extended the UTAUT2 model to provide an understanding of different dimensions that influence Behavioral Intentions to use SMARTLab. We suggested an AR acceptance model that uses four dimensions derived from UTAUT2 to assess the Behavioral Intentions toward SMARTLab: 1) Performance Expectancy, 2) Effort Expectancy, 3) Hedonic Motivation, and 4) Behavioral Intentions Venkatesh et al. (2012). Spatial presence, Personal Innovativeness, Attention, Emotional Reactivity, and Realism are exploited via the proposed model. Personal Innovativeness and Spatial presence refer to participants’ motivation to use novel technology, such as AR, and the participants’ impression of being in an AR environment, respectively; therefore, PI and SP could have a direct influence on BI. The questionnaires of Attention and Emotional Reactivity are proposed to evaluate AR assistance tools (the attention funnel, in-situ arrow, and virtual assistant) as described in Section 4.3.1. Whereas the Realism dimension relates to the realism of liquid inside the beaker, the action of pouring, and the whole experience. Therefore, the two dimensions could also have an influence on Behavioral Intentions. Consequently, we hypothesized:
• H1, H2, H3, H4, and H5: Performance Expectancy, Effort Expectancy, Hedonic Motivation, Personal Innovativeness, and Spatial Presence will have a positive influence on the Behavioral Intentions to use SMARTLab.
• H6: The attention funnel and in-situ arrow presented via the Attention dimension will have a positive influence on the Behavioral Intentions to use SMARTLab.
• H7: The virtual assistant described via the Attention dimension will have a positive influence on the Behavioral Intentions to use SMARTLab.
• H8: Realism will have a positive influence on the Behavioral Intentions to use SMARTLab.
Figure 4 illustrates the AR acceptance model and the relationships between the dimensions considering the hypotheses mentioned above.
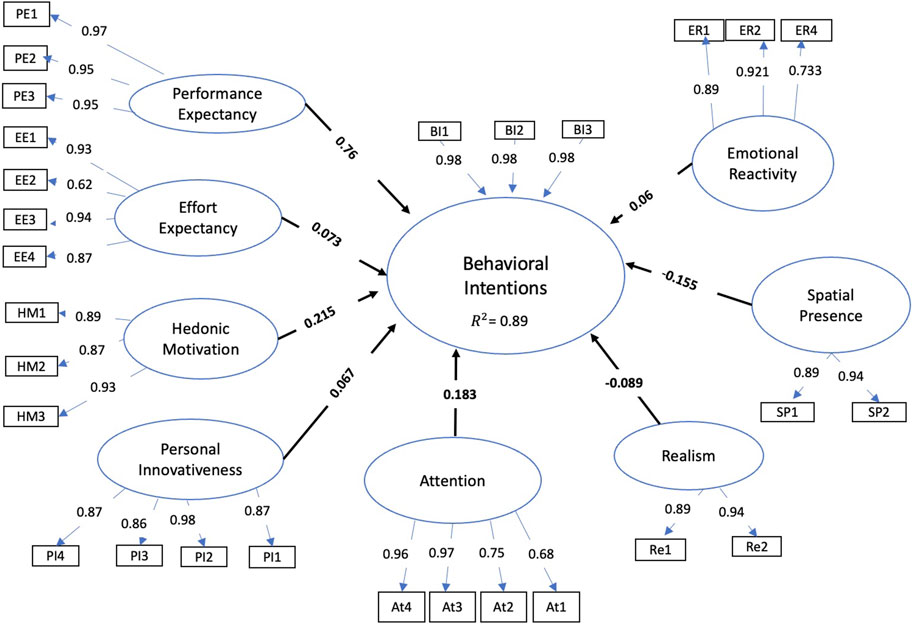
Figure 4. AR acceptance model to evaluate Behavior Intention toward SMARTLab. Values between dimensions and Behavioral Intentions refer to path coefficients β values. Outer loading values are described between questions and related dimensions. R2 indicates the coefficient determination for Behavioral Intentions.
4.4 Free form written responses
At the end of each session, participants were asked to provide qualitative feedback on SMARTLab. The responses were analyzed by a researcher who was not involved in the design or implementation of SMARTLab, using the four-step process of Erlingsson and Brysiewicz (2017). The steps consisted of identifying meaningful units from the text provided by the participants, coding the meaning units, creating categories for codes, and finally creating themes for sets of categories. The analysis was done across all participants.
4.5 Task completion time and error rate
We chose not to evaluate the participant’s performance using TTC and NE in this study for two main reasons: 1) the paper does not focus on comparing SMARTLab with other training tools and 2) the NE metric requires further examination as certain problems may arise due to graphical fidelity (e.g., I do not understand that this is a dirty coat) or technical aspects such as the HoloLens grabbing action (e.g., I cannot select the right coat) or lack of expert domain knowledge (e.g., I do not know that I should not be selecting the dirty coat).
5 Results
In this section, first, we present the results derived from questionnaire data, including the individual sub-scales and the AR acceptance model. Second, the results of written responses are described.
5.1 Questionnaire data
Before any other processing of the data was done, Reliability analysis, Convergent Validity, and Discriminant Validity were performed on all dimensions. These metrics allow us to examine the relationship between items within the same dimension, as well as the relationship between dimensions.
5.1.1 Reliability analysis
We ascertained the reliability analysis by examining the outer loading, Cronbach’s alpha, and Composite Reliability. Outer loading describes how well a question represents the underlying dimension. Outer loading greater than 0.70 is believed to provide statistical significance and indicates that the measurement model fits Vinzi et al. (2010). The outer loading value, which is between 0.40 and 0.70, should be considered for deletion if the removal of the related question contributes to an increase in Composite Reliability and Average Variance Extracted (AVE) described in the following paragraph. Furthermore, questions with outer loading below 0.40 should always be removed Leguina (2015). Hence, in the final decision stage as mentioned in Table 2, a total of seven items (questions) from SP (two items (SP3 and SP4)), Re (one item (Re3)), and ER (four items (ER3, ER5, ER6, and ER7)) were removed from the measurement model.
Moreover, if the answers to the questions within a dimension are highly correlated, this is called high internal consistency, which is measured via Cronbach’s alpha Gliem and Gliem (2003) and Composite Reliability (CR) Nunnally and Bernstein (1978). These are measures of the extent to which the questions within a group are related to each other and provide an estimate of the measurement accuracy, which is called the reliability of a group of items. Cronbach’s alpha and CR values above 0.7 are acceptable; values substantially lower than 0.7 indicate unreliability. The Cronbach’s alpha values ranged from 0.74 for Realism to 0.98 for Behavioral Intentions, and CR values ranged from 0.85 for Realism to 0.97 for Performance Expectancy. For both measures, all dimensions exceeded the recommended cutoff of 0.7 as illustrated by Table 3, thereby suggesting high internal reliability.
5.1.2 Convergent Validity
Convergent Validity (CV) Fornell and Larcker (1981) was assessed by measuring the Average Variance Extracted (AVE). AVE is a measure of the amount of variance that is captured by a dimension in relation to the amount of variance due to measurement error. The AVE ranged from 0.71 to 0.96 as illustrated in Table 3 and is greater than 0.5 for each dimension, thereby indicating Convergent Validity (CV).
5.1.3 Discriminant validity
The purpose of the Discriminant Validity (DV) assessment is to confirm that a dimension (e.g., Attention, Realism, etc.) is genuinely distinct from other dimensions in the model. It ensures that a dimension demonstrates a stronger relationship with its own items than with the items of any other dimension in the model. To evaluate DV, the square root of the AVE of each dimension Ab Hamid et al. (2017) is compared with the others. The square root of the AVE of a dimension should be greater than its correlation with other dimensions to achieve satisfactory DV. Additionally, the diagonal values should be higher than the off-diagonal values in the corresponding columns and rows. For each dimension shown in Table 4 highlighted in bold, the square root of the AVE exceeded the inter-dimension correlations, thereby indicating an appropriate level of DV.
Therefore, the decision was made to not reject any dimension for further analyses. We focus on 1) reporting the individual sub-scales and 2) drawing overall conclusions on the relationships between them.
5.1.4 Individual sub-scales
Table 5 presents descriptive statistics, including the mean and standard deviation, for the nine dimensions. Users perceived SMARTLab to be fun (M = 4.21 SD = 0.95), and they also noted that they intended to use it (M = 4.08 SD = 1.27). They also perceived that it required moderate effort to use (M = 3.67 SD = 1.31), while also indicating that it was likely to help them fulfill their goals (M = 3.79 SD = 1.31). Hence, the dimensions derived from UTAUT2 (PE, EE, HM, and BI) provide a preliminary positive indicator of the acceptability of the AR environment. This alignment is consistent with various studies, e.g., Sunardi et al. (2022) and Faqih and Jaradat (2021), related to AR acceptance in the training domain and indicates the applicability of the UTAUT2 model to assess an AR system.
Related work has demonstrated the impact of the appearance of virtual characters on the participants’ emotional reactions, Mousas et al. (2018b) and Schrammel et al. (2009), and perceptions, Ruhland et al. (2015). In SMARTLab, the appearance and motion of the virtual assistant providing vocal instruction had no negative effect on the participant’s emotional reaction (M = 4.22, SD = 0.94). This was also confirmed via the Structural model as illustrated in the next subsection.
To the best of our knowledge, the two AR assistance tools (the attention funnel and in-situ arrow) have not been addressed in any state-of-the-art acceptance model. The attention dimension that encompasses these tools was ranked fourth in Table 5, confirming the benefits of using the attention funnel and in-situ arrow in the context of AR safety training. This ranking was also reflected in the acceptance of the AR model, as mentioned in the next paragraph.
However, the results of the Realism dimension (M = 2.67, SD = 0.93) show that the experience is not very close to a real situation. Visual realism, as emphasized in Zhao et al. (2022) and Itoh et al. (2021), is still regarded as a key challenge in constructing an AR application. This challenge may stem from the difficulty of fully controlling the light within an AR-HMD, as noted in Itoh et al. (2021), amongst other factors.
Besides the evaluation of nine dimensions, participants were asked to fill out a Simulator Sickness Questionnaire (SSQ). Despite the advantages of using AR over VR headsets, which can cause motion sickness and isolation effects, Vovk et al. (2018), some participants had reported difficulty focusing (M = 1.54, SD = 0.66) and general discomfort (M = 1.5, SD = 0.37) after using SMARTLab, as described in Table 6.
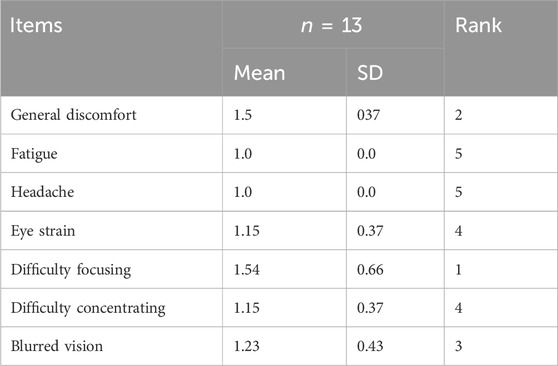
Table 6. Descriptive statistics for seven items from the adapted version of the Simulator Sickness Questionnaire (SSQ) Bouchard et al. (2007); Kennedy et al. (1993) with a 4-point scale, from 1 (no sign) to 4 (severe).
5.1.5 Structural model
The AR acceptance model was analyzed using a Structural Equation Model (SEM) based on Partial Least Squares (PLS) Chin (1998). PLS allows for evaluation of the influences of Performance Expectancy, Effort Expectancy, Hedonic Motivation, Personal Innovativeness, Emotional Reactivity, Spatial Presence, Attention, and Realism on Behavioral Intentions. Figure 4 shows the result of the structural model with interaction effects. The path coefficients β and coefficient of determination R2 are analyzed using PLS. The path coefficient refers to the direct influence of a variable assumed to be a cause on another variable assumed to be an influence. Hence, the path coefficients indicate the changes in a dependent variable’s value that are associated with standard deviation unit changes in an independent variable. A path coefficient between Attention and Behavioral Intentions of 0.183, as illustrated in Figure 4, indicates that when the Attention dimension (an independent variable) increases by one standard deviation unit, Behavioral Intentions (the dependent variable) will increase by 0.183 standard deviation units. The path coefficient values Lehner and Haas (2010) that are in the range of −0.1 to 0.1 are considered insignificant, values greater than 0.1 are significant and directly proportional, and smaller values of −0.1 are significant values and inversely proportional.
Therefore, Emotional Reaction β = 0.06, Realism β = 0.089, Effort Expectancy β = 0.073, and Personal Innovativeness β = 0.0.067 do not have a significant influence on Behavioral Intentions.Performance Expectancy, Hedonic motivation, and Attention (β = 0.76, 0.215, and 0.183, respectively) have a significant positive influence, whereas Spatial Presence (β = −0.155) has a significant negative influence.
As far as we know, there are no studies on AR acceptance models that specifically address Emotional Reaction, Realism, and Attention. However, Performance Expectancy and Hedonic Motivation, in line with the previous research of Marto et al. (2023) and Alqahtani and Kavakli (2017), have been shown to influence Behavioral Intention (BI). This confirms that a stronger Performance Expectancy and participants’ hedonic motivation, emphasizing pleasure and fun in using the system, lead to a stronger behavioral intention to use the system. In the following section, the influence of each dimension on Behavioral Intention (BI) will be discussed in detail.
The coefficient of determination R2 refers to the proportion of the variation in the dependent variable such as Behavioral Intentions that is predictable from the independent variable(s). R2 measures how well a statistical model predicts an outcome. The outcome is represented by the model’s dependent variable which is Behavioral Intentions in this case. The lowest possible value of R2 is 0 and the highest possible value is 1. The better a model is at making predictions, the closer its R2 will be to 1. Therefore, the R2 value for Behavioral Intentions of 0.89 indicates that the independent variables (Performance Expectancy, Hedonic Motivation, Effort Expectancy, Personal Innovativeness, Spatial Presence, Attention, Emotional Reaction, and Realism) explain a high proportion of the variation in Behavioral Intentions (the dependent variable).
5.2 Written responses
Due to the nature of qualitative data, evaluations that adopt a qualitative approach usually involve a limited number of participants, see Merino et al. (2020). In SMARTLab, as mentioned previously in Section 4.4, the four steps outlined by Erlingsson and Brysiewicz (2017) have been implemented on the feedback provided by the participants at the end of the experience:
• Themes: the analysis resulted in two broad themes being identified; these were 1) “positive aspects of the experience” and 2) “user suggestions and issues related to user experience”, as outlined in Tables 7, 8. In the following section, the number of items per code and category is indicated in brackets.
• Meaning Units: a total of 15 items are identified for each theme.
• Codes: within “Positive aspects of the experience” and “users’ suggestions and issues related to user experience”, eight and four codes were identified, respectively (see Tables 7, 8).
• Category: the codes of the first theme fell into two categories, the first focusing on positive aspects of the UX (15 items in total): fun and enjoyment (3), learning curve (1), interaction (3), and immersion (1). The second category related to SMARTLab being a potential solution for training and included aspects such as identification of right and wrong actions (1), simulation (1), general and future training (3), and safety procedures (1). The codes of the second theme were also grouped into two categories. The first category was related to interface enhancements (9) and contains two codes: guidance (6) and intuitiveness (3). The second category focuses on potential software and hardware advances (6) and contains two codes: realism (3) and awareness (3).
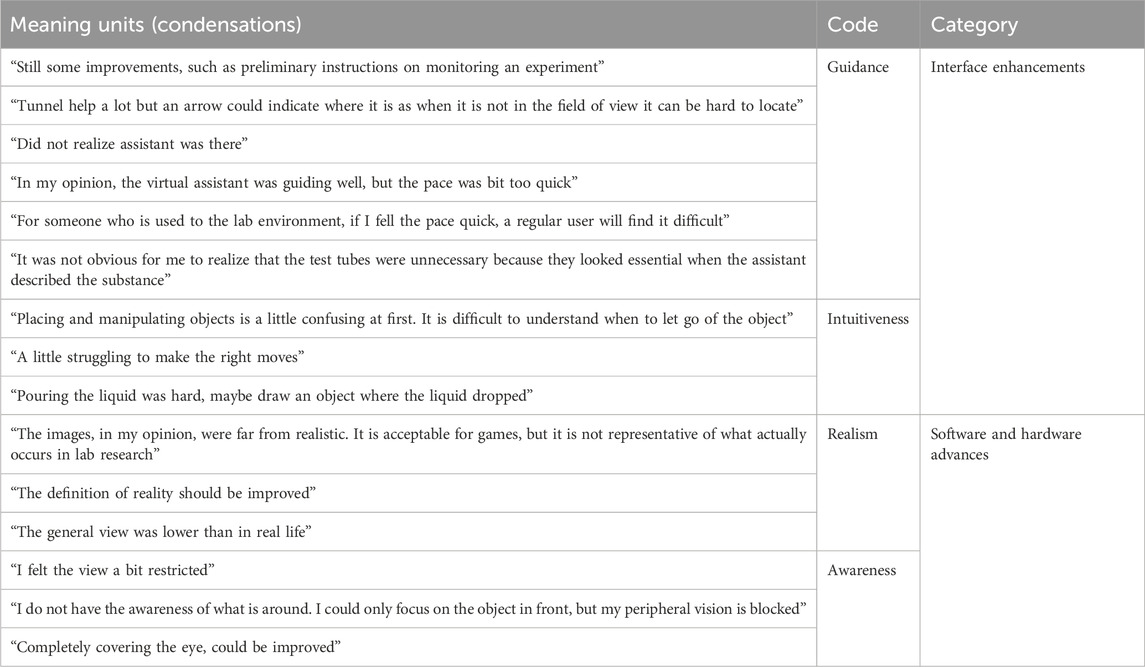
Table 8. Content analysis results: Theme 2 - Users’ suggestions and issues related to the experience.
As a result of this content identification process, the suggestions for improvement rest in some cases with a need to improve the design of SMARTLab, e.g., the pacing of the guidance and visibility of the virtual assistant, interaction and graphical design, through to issues relating to the use of Microsoft HoloLens, e.g., the small field of view. The concept of immersion in AR is often contested (unlike in VR, users are not immersed in a new 3D world). This may explain why it was only mentioned positively once in Table 8. It could also be argued that this also relates to the more negative comments such as a small field of view reducing awareness, and this is further amplified by other issues relating to lower realism.
6 Discussion
Our primary focus in this study was to explore the acceptance of AR as a training tool for hazardous situations. Junaini et al. (2022) indicate that AR applications have the potential to be utilized for occupational safety and health (OSH) training. Moreover, prior work by Wild et al. (2017) has already pointed to users, particularly early adopters, finding such technologies useful in a range of domains. However, to date, application domains similar to laboratories, such as the one used here, have not been explored. In addition to exploring a subset of traditional acceptance models, such as UTAUT2, this work was extended to explore the presence aspect, as this is particularly relevant for exploring if people are likely to behave in a similar way toward real and virtual experiences of the same situation or virtual agents (social presence).
Furthermore, SMARTLab was assessed for simulator sickness, determining cybersickness level. Examining Table 6, it is noted that wider ergonomic issues such as fatigue and headaches were not reported as an issue.
In this section, we also discuss the dimensions’ impact on Behavioral Intentions to accept SMARTLab, exploring the results derived from: 1) individual sub-scales described in Table 5. 2) SMARTLab acceptance model illustrated in Table 9 which describes the hypotheses and outcomes. The “Conclusion” column indicates whether the hypothesis is supported or not supported. 3) Free form written response mentioned in Tables 7, 8.
6.1 Hedonic motivation
The Hedonic Motivation (or fun and pleasure) dimension was highlighted as a positive aspect of SMARTLab (M = 3.79 SD = 1.31), and the feelings of fun were also conveyed within the interviews, with various comments (see Table 7) relating to fun being identified. Additionally, it is noted (see Table 9) that Hedonic Motivation has a significant influence (β = 0.215) on Behavioral Intentions. These results point to SMARTLab providing an enjoyable experience for trainees.
6.2 Performance expectancy
Based on Table 9, Performance Expectancy has a significant influence (β = 0.76) on Behavioral Intentions. Hence, participants believe that using SMARTLab will enhance their job performance. This was reflected in the qualitative data through the category “Potential of the solution for training”. As indicated in the results section and consistent with prior research Marto et al. (2023) Alqahtani and Kavakli (2017), earlier studies have shown that both Performance Expectancy and Hedonic Motivation play a significant role in influencing Behavioral Intention (BI) to accept an AR system.
6.3 Effort expectancy
Previous works Saprikis et al. (2020) Ronaghi and Ronaghi (2022) Alqahtani and Kavakli (2017) show a significant influence of Effort Expectancy on adopting AR systems. However, in our proposed AR acceptance model, Effort Expectancy (β = 0.073) did not have a significant influence. Therefore, the perceived ease of use for SMARTLab in the domain of AR for training purposes was not considered an important point for participants to increase Behavioral Intentions. However, this was not reflected in the written response. In the Code “Intuitiveness”, illustrated in Table 8, participants mentioned multiple phrases related to the interface enhancements such as “A little struggling to make the right moves”. Accordingly, effort expectancy could have an effect on the adoption of SMARTLab. This disagreement between the results of the AR acceptance model and written form is explained by the fact that AR is a new technology and participants had expected a considerable effort to manipulate the AR environment. However, at the end of the experience, it was handled in quite a straightforward manner, as described by the Code “learning curve” with the following sentence “I quickly got used to this tool”. Therefore, we suggest that in rating the questionnaires related to the Effort Expectancy dimension, participants overlooked the improvements and advances suggested in Table 8, as they found SMARTLab easier to deal with than expected.
6.4 Personal innovativeness
Like Effort Expectancy, Personal Innovativeness (β = 0.067) was also not considered essential to increase Behavioral Intentions. It could be due to the background of the participants who are domain experts and who are familiar with advanced equipment in laboratories.
6.5 Realism
Visual realism is considered a key challenge in constructing an AR application, as indicated by Zhao et al. (2022) and Itoh et al. (2021). In SMARTLab, the Realism dimension was noted as an issue across the different types of evaluation data, with questionnaire responses providing the lowest score (M = 2.67, SD = 0.93). The Realism dimension also has no significant influence on Behavioral Intentions with β = −0.089. On exploring the comments made by end users, this would appear to be related to aspects such as the intuitiveness and the quality of the 3D models used. The behavior of the fluids also requires some improvement, which may have lowered the feeling of realism.
6.6 Spatial presence
Smink et al. (2020) found that an AR application induced more spatial presence than the comparative non-AR application. They also mentioned that the higher level of spatial presence induced by the AR application consequently enhanced both application attitude and behavioral intention. However, measuring the Spatial Presence dimension is somewhat debatable in the context of AR-HMD, as the intention is not to make people feel present somewhere else. Nevertheless, as the objective of SMARTLab is to take people to a virtual lab where they largely ignore the environment around them (within safety limits), it was considered worth exploring. Despite the fact that it is not an immersive experience, people still reported feeling moderately spatially present (M = 3.87, SD = 0.85); there was also one comment specifically on feeling spatially present. The relatively small field of view may also have had some impact on this, as peripheral vision plays an important role in being aware of risks and hazards, which may also have lowered feelings of Realism and Spatial Presence. However, the AR acceptance model illustrates that Spatial Presence has a significant negative influence on Behavioral Intentions (β = −1.55). Hence, increasing the impression of being inside the AR environment will decrease the user’s intention to use SMARTLab due to providing a feeling of discomfort in the environment. However, the underlying reason for the discomfort was not clear and needs to be explored further before any firm conclusions can be drawn. Hence, we encourage the exploration of the Spatial Presence dimension in an acceptance model for AR applications to provide further insight into our finding that the more users feel present in the virtual environment of SMARTLab, the less they intend to use the application.
6.7 Emotional reactivity
The Emotional Reactivity dimension provided a participant evaluation of the virtual assistant via three items (see Table 2) and is highly prominent, with the first rank among the nine dimensions. Therefore, we surmise that the simple hovering robot is welcome and provides no sense of discomfort or unease. Despite the quick movement of the virtual assistant, as commented on in Table 8, it was also mentioned that it provided guidance well. Furthermore, the AR acceptance model shows that the appearance of a virtual assistant represented via Emotional Reactivity does not discourage using SMARTLab with a positive value of β = 0.06. However, Emotional Reactivity does not have any positive or negative influence on Behavioral Intentions. To the best of our knowledge, exploring the influence of Emotional Reactivity on Behavioral Intention to adopt an AR application has not yet been tackled in the state of the art. Therefore, we suggest comparing multiple types of virtual assistant and their effects on Behavioral Intentions in any further study. Most AR applications, see Kim et al. (2018b) and Randhavane et al. (2019), in different domains contain a virtual assistant, virtual avatar, or intelligent virtual agent to guide or assist the user; therefore, using the Emotional Reactivity dimension, as suggested in this work, could help to analyze its influence on the acceptance of AR application.
6.8 Attention
Concerning the Attention dimension which evaluates the in-situ arrow and attention funnel, users’ self-reports indicated that they found it good for future training and that it improved their ability to understand right and wrong actions along with the benefits of simulating dangerous substances. It was noted that while people made mistakes, they often tried to complete the tasks as they would in the laboratory. This was reflected in the AR model acceptance where the Attention dimension had a significant positive influence on Behavioral Intentions with β = 0.183. Accordingly, it has confirmed the benefits of the attention funnel and in-situ arrow in the AR training domain to increase Behavioral Intentions. Despite some suggestions mentioned in qualitative data to improve the virtual assistant and attention funnel, the AR assistance tools, including the three user interface features are highly noted by the participants via Emotional Reactivity and Attention dimensions. Additionally, Attention has a significant influence on Behavioral Intentions. Therefore, this study recommends the use of such tools to make the training experience more acceptable to users.
7 Limitations and future work
SMARTLab has some limitations but was generally well received by end-users. It is our view that subject to some changes, the SMARTLab approach to training has the potential to become more widely used. Key areas of improvement include improved 3D models and more intuitive interaction approaches. One solution may be to use Azure Cloud rendering to improve the graphical aspects. There is also a need to explore improvements to the pacing of assistance and guidance, as well as the prominence given to the virtual assistant. Moreover, the fluid simulation using a particle system requires improvement to convince the participant that the liquid is manipulated in a realistic manner. From a validation perspective, a wider study is needed to validate any new version of SMARTLab against other training methods. From a study perspective, it is important to not only examine the aspects discussed here but also the relevant learning effects of such techniques. Future work is required to assess their impact.
Limitations remain with AR devices, not the least field of view, interaction techniques, and the fact that some users still have problems with focusing on content. With time, many of these limitations will be removed or reduced. However, they remain a significant issue for many tasks and users.
8 Conclusion
In this paper, we have explored the SMARTLab system, which is designed to provide lab safety training for those working in a hazardous material science laboratory. We have presented the underlying scenario, AR system assessment methodology, technical implementation, and provided a detailed user study. A relevant use case was chosen based on extensive discussions with domain experts. An AR training environment was built which included additional assistance tools, namely, the attention funnel, a virtual assistant, and an in-situ arrow. An evaluation involving 13 people was undertaken using a combination of questionnaires and interviews. Using AR for lab training was positively received by the participants and the assistance tools were deemed beneficial. We suggest an extended UTAUT2 by including additional dimensions, specifically Personal Innovativeness, Attention, Realism, Spatial Presence, and Emotional Reactivity. The study pointed to positive influences of Performance Expectancy, Hedonic Motivation, and Attention on Behavioral Intentions, whereas Spatial Presence has a significant negative influence on Behavioral Intentions. The study also pointed to the positive benefits of using assistance techniques such as the in-situ arrow and attention funnel.
In summary, this paper has shown a methodology to assess the safety of AR training applications using the suggested AR acceptance model. The results describe the benefits of laboratory-based AR training and have provided some design considerations and features that merit further exploration which could be incorporated into future systems.
Data availability statement
The datasets presented in this article are not readily available because We have based our research activity on participants’ consent, as defined by Article 6(1) of the GDPR. It has been mentioned in the consent that the data acquired during the experimental tests for this study will not be shared with anyone outside of our research institute. Requests to access the datasets should be directed to bXVoYW5uYWQuaXNtYWVsQGxpc3QubHU=.
Ethics statement
The studies involve humans and we do not have a formal ethics approval process, the ethical committee provides recommendations which we can choose to accept. The studies were conducted in accordance with the local legislation and institutional requirements. The participants provided their written informed consent to participate in this study.
Author contributions
MI: Writing–original draft, Writing–review and editing. RM: Writing–original draft, Writing–review and editing. FM: Writing–original draft, Writing–review and editing. IB: Writing–review and editing. MS: Writing–review and editing. JB: Writing–review and editing. DA: Writing–review and editing.
Funding
The author(s) declare financial support was received for the research, authorship, and/or publication of this article. This work was supported by internal funding from the Luxembourg Institute of Science and Technology.
Conflict of interest
The authors declare that the research was conducted in the absence of any commercial or financial relationships that could be construed as a potential conflict of interest.
Publisher’s note
All claims expressed in this article are solely those of the authors and do not necessarily represent those of their affiliated organizations, or those of the publisher, the editors and the reviewers. Any product that may be evaluated in this article, or claim that may be made by its manufacturer, is not guaranteed or endorsed by the publisher.
Supplementary material
The Supplementary Material for this article can be found online at: https://www.frontiersin.org/articles/10.3389/frvir.2024.1322543/full#supplementary-material
Footnotes
1https://cen.acs.org/safety/industrial-safety/US-Chemical-Safety-Board-issues/101/web/2023/01
2https://github.com/microsoft/MixedRealityToolkit-Unity
3https://assetstore.unity.com/packages/tools/physics/obi-fluid-63067
References
Ab Hamid, M., Sami, W., and Sidek, M. M. (2017). “Discriminant validity assessment: use of fornell and larcker criterion versus htmt criterion,” in Journal of physics: conference series. (Bristol, England: IOP Publishing), 890, 012163.
Agarwal, R., and Karahanna, E. (2000). Time flies when you’re having fun: cognitive absorption and beliefs about information technology usage. MIS Q. 24, 665–694. doi:10.2307/3250951
Alqahtani, H., and Kavakli, M. (2017). “A theoretical model to measure user’s behavioural intention to use imap-campus app,” in 2017 12th IEEE conference on industrial electronics and applications (ICIEA) (IEEE), 681–686.
Asgary, A., Bonadonna, C., and Frischknecht, C. (2020). Simulation and visualization of volcanic phenomena using microsoft hololens: case of vulcano island (Italy). IEEE Trans. Eng. Manag. 67, 545–553. doi:10.1109/TEM.2019.2932291
Avila-Garzon, C., Bacca-Acosta, J., Duarte, J., and Betancourt, J. (2021). Augmented reality in education: an overview of twenty-five years of research. Contemp. Educ. Technol. 13, ep302. doi:10.30935/cedtech/10865
Azuma, R. T. (1997). A survey of augmented reality. Presence teleoperators virtual Environ. 6, 355–385. doi:10.1162/pres.1997.6.4.355
Bagozzi, R. (2007). The legacy of the technology acceptance model and a proposal for a paradigm shift. J. AIS 8, 244–254. doi:10.17705/1jais.00122
Bahnmüller, A., Wulkop, J., Gilg, J., Schanz, D., Schröder, A., Albuquerque, G., et al. (2021). Augmented reality for massive particle distribution.
Bai, M., Liu, Y., Qi, M., Roy, N., Shu, C.-M., Khan, F., et al. (2022). Current status, challenges, and future directions of university laboratory safety in China. J. Loss Prev. Process Industries 74, 104671. doi:10.1016/j.jlp.2021.104671
Bigagli, D. (2022). Realistic path development in a virtual environment for detection and rehabilitation treatments of Alzheimer’s disease patients. Politecnico di Torino: Ph.D. thesis.
Biocca, F., Tang, A., Owen, C., and Xiao, F. (2006). “Attention funnel: omnidirectional 3d cursor for mobile augmented reality platforms,” in Proceedings of the SIGCHI conference on human factors in computing systems (New York, NY, USA: Association for Computing Machinery), 1115–1122. doi:10.1145/1124772.1124939
Blattgerste, J., and Pfeiffer, T. (2020). Promptly authored augmented reality instructions can be sufficient to enable cognitively impaired workers. doi:10.18420/vrar2020_25
Bouchard, S., Robillard, G., and Renaud, P. (2007). Revising the factor structure of the simulator sickness questionnaire. Annu. Rev. CyberTherapy Telemedicine 5, 128–137.
Carmigniani, J., Furht, B., Anisetti, M., Ceravolo, P., Damiani, E., and Ivkovic, M. (2011). Augmented reality technologies, systems and applications. Multimedia tools Appl. 51, 341–377. doi:10.1007/s11042-010-0660-6
Cheng, J., Chen, K., and Chen, W. (2020). State-of-the-art review on mixed reality applications in the aeco industry. J. Constr. Eng. Manag. 146, 03119009. doi:10.1061/(ASCE)CO.1943-7862.0001749
Chin, W. W. (1998). The partial least squares approach to structural equation modeling. Mod. methods Bus. Res. 295, 295–336.
Dakkoune, A., Vernières-Hassimi, L., Leveneur, S., Lefebvre, D., and Estel, L. (2018). Risk analysis of French chemical industry. Saf. Sci. 105, 77–85. doi:10.1016/j.ssci.2018.02.003
Davis, F. (1989). Perceived usefulness, perceived ease of use, and user acceptance of information technology. MIS Q. 13, 319. doi:10.2307/249008
de Melo, C. M., Kim, K., Norouzi, N., Bruder, G., and Welch, G. (2020). Reducing cognitive load and improving warfighter problem solving with intelligent virtual assistants. Front. Psychol. 11, 554706. doi:10.3389/fpsyg.2020.554706
De Micheli, A. J., Valentin, T., Grillo, F., Kapur, M., and Schuerle, S. (2022). Mixed reality for an enhanced laboratory course on microfluidics. J. Chem. Educ. 99, 1272–1279. doi:10.1021/acs.jchemed.1c00979
Erlingsson, C., and Brysiewicz, P. (2017). A hands-on guide to doing content analysis. Afr. J. Emerg. Med. 7, 93–99. doi:10.1016/j.afjem.2017.08.001
Faqih, K. M., and Jaradat, M.-I. R. M. (2021). Integrating ttf and utaut2 theories to investigate the adoption of augmented reality technology in education: perspective from a developing country. Technol. Soc. 67, 101787. doi:10.1016/j.techsoc.2021.101787
Fornell, C., and Larcker, D. F. (1981). Evaluating structural equation models with unobservable variables and measurement error. J. Mark. Res. 18, 39–50. doi:10.2307/3151312
Gliem, J. A., and Gliem, R. R. (2003). “Calculating, interpreting, and reporting cronbach’s alpha reliability coefficient for likert-type scales,” in Midwest research-to-practice conference in adult, continuing, and community.
González, A. N. V., Kapalo, K., Koh, S., Sottilare, R., Garrity, P., and Laviola, J. J. (2019). “A comparison of desktop and augmented reality scenario based training authoring tools,” in 2019 IEEE conference on virtual reality and 3D user interfaces (VR), 1199–1200. doi:10.1109/VR.2019.8797973
Gruenefeld, U., Lange, D., Hammer, L., Boll, S., and Heuten, W. (2018). “Flyingarrow: pointing towards out-of-view objects on augmented reality devices,” in Proceedings of the 7th ACM international symposium on pervasive displays, 1–6.
Iqbal, J., and Sidhu, M. (2022). Acceptance of dance training system based on augmented reality and technology acceptance model (tam). Virtual Real. 26, 33–54. doi:10.1007/s10055-021-00529-y
Itoh, Y., Langlotz, T., Sutton, J., and Plopski, A. (2021). Towards indistinguishable augmented reality: a survey on optical see-through head-mounted displays. ACM Comput. Surv. (CSUR) 54, 1–36. doi:10.1145/3453157
Junaini, S., Kamal, A. A., Hashim, A. H., Mohd Shaipullah, N., and Truna, L. (2022). Augmented and virtual reality games for occupational safety and health training: a systematic review and prospects for the post-pandemic era. Int. J. Online Biomed. Eng. (iJOE) 18, 43–63. doi:10.3991/ijoe.v18i10.30879
Jung, S., Woo, J., and Kang, C. (2020). Analysis of severe industrial accidents caused by hazardous chemicals in South Korea from january 2008 to june 2018. Saf. Sci. 124, 104580. doi:10.1016/j.ssci.2019.104580
Kalantari, M., and Rauschnabel, P. (2018). “Exploring the early adopters of augmented reality smart glasses: the case of microsoft hololens,” in Augmented reality and virtual reality (Springer), 229–245.
Kaplan, A. D., Cruit, J., Endsley, M., Beers, S. M., Sawyer, B. D., and Hancock, P. A. (2021). The effects of virtual reality, augmented reality, and mixed reality as training enhancement methods: a meta-analysis. Hum. factors 63, 706–726. doi:10.1177/0018720820904229
Kennedy, R. S., Lane, N. E., Berbaum, K. S., and Lilienthal, M. G. (1993). Simulator sickness questionnaire: an enhanced method for quantifying simulator sickness. Int. J. Aviat. Psychol. 3, 203–220. doi:10.1207/s15327108ijap0303_3
Kim, K., Billinghurst, M., Bruder, G., Duh, H. B.-L., and Welch, G. F. (2018a). Revisiting trends in augmented reality research: a review of the 2nd decade of ismar (2008–2017). IEEE Trans. Vis. Comput. Graph. 24, 2947–2962. doi:10.1109/tvcg.2018.2868591
Kim, K., Boelling, L., Haesler, S., Bailenson, J., Bruder, G., and Welch, G. F. (2018b). “Does a digital assistant need a body? the influence of visual embodiment and social behavior on the perception of intelligent virtual agents in ar,” in 2018 IEEE international symposium on mixed and augmented reality (ISMAR), 105–114. doi:10.1109/ISMAR.2018.00039
Koenig, C., Ismael, M., and McCall, R. (2021). OST-HMD for safety training. Ost-hmd Saf. Train., 342–352. doi:10.1007/978-3-030-68449-5_34
Lehner, F., and Haas, N. (2010). Knowledge management success factors – proposal of an empirical research. Electron. J. Knowl. Manag. 8, pp79–90.
Liu, C., Chen, X., Liu, S., Zhang, X., Ding, S., Long, Y., et al. (2019). “The exploration on interacting teaching mode of augmented reality based on hololens,” in International conference on technology in education (Springer), 91–102.
Macklin, M., and Müller, M. (2013). Position based fluids. ACM Trans. Graph. (TOG) 32, 1–12. doi:10.1145/2461912.2461984
Marto, A., Gonçalves, A., Melo, M., Bessa, M., and Silva, R. (2023). Aram: a technology acceptance model to ascertain the behavioural intention to use augmented reality. J. Imaging 9, 73. doi:10.3390/jimaging9030073
Merino, L., Schwarzl, M., Kraus, M., Sedlmair, M., Schmalstieg, D., and Weiskopf, D. (2020). “Evaluating mixed and augmented reality: a systematic literature review,” in 2020 IEEE international symposium on mixed and augmented reality (ISMAR) (IEEE), 438–451.
Milgram, P., Takemura, H., Utsumi, A., and Kishino, F. (1995). Augmented reality: a class of displays on the reality-virtuality continuum. Telemanipulator telepresence Technol. 2351, 282–292. https://doi.org/10.1117/12.197321
Mourtzis, D., Angelopoulos, J., and Panopoulos, N. (2022). Challenges and opportunities for integrating augmented reality and computational fluid dynamics modeling under the framework of industry 4.0. Procedia CIRP 106, 215–220. doi:10.1016/j.procir.2022.02.181
Mousas, C., Anastasiou, D., and Spantidi, O. (2018a). The effects of appearance and motion of virtual characters on emotional reactivity. Comput. Hum. Behav. 86, 99–108. doi:10.1016/j.chb.2018.04.036
Mousas, C., Anastasiou, D., and Spantidi, O. (2018b). The effects of appearance and motion of virtual characters on emotional reactivity. Comput. Hum. Behav. 86, 99–108. doi:10.1016/j.chb.2018.04.036
Mousas, C., Koilias, A., Rekabdar, B., Kao, D., and Anastasiou, D. (2021). “Toward understanding the effects of virtual character appearance on avoidance movement behavior,” in 2021 IEEE virtual reality and 3D user interfaces (VR), 40–49.
Nunnally, J. C., and Bernstein, I. (1978). “Psychometric theory mcgraw-hill New York,” in The role of university in the development of entrepreneurial vocations: a Spanish study, 387–405.
Papakostas, C., Troussas, C., Krouska, A., and Sgouropoulou, C. (2022). User acceptance of augmented reality welding simulator in engineering training. Educ. Inf. Technol. 27, 791–817. doi:10.1007/s10639-020-10418-7
Pollick, F. (2009). In search of the uncanny valley. User Centric Media 40, 69–78. doi:10.1007/978-3-642-12630-7_8
Randhavane, T., Bera, A., Kapsaskis, K., Gray, K., and Manocha, D. (2019). Fva: modeling perceived friendliness of virtual agents using movement characteristics
Ronaghi, M. H., and Ronaghi, M. (2022). A contextualized study of the usage of the augmented reality technology in the tourism industry. Decis. Anal. J. 5, 100136. doi:10.1016/j.dajour.2022.100136
Ruhland, K., Peters, C. E., Andrist, S., Badler, J. B., Badler, N. I., Gleicher, M., et al. (2015). A review of eye gaze in virtual agents, social robotics and hci: behaviour generation, user interaction and perception. Comput. Graph. forum 34, 299–326. doi:10.1111/cgf.12603
Saprikis, V., Avlogiaris, G., and Katarachia, A. (2020). Determinants of the intention to adopt mobile augmented reality apps in shopping malls among university students. J. Theor. Appl. Electron. Commer. Res. 16, 491–512. doi:10.3390/jtaer16030030
Schrammel, F., Pannasch, S., Graupner, S.-T., Mojzisch, A., and Velichkovsky, B. M. (2009). Virtual friend or threat? the effects of facial expression and gaze interaction on psychophysiological responses and emotional experience. Psychophysiology 46, 922–931. doi:10.1111/j.1469-8986.2009.00831.x
Smink, A. R., Van Reijmersdal, E. A., Van Noort, G., and Neijens, P. C. (2020). Shopping in augmented reality: the effects of spatial presence, personalization and intrusiveness on app and brand responses. J. Bus. Res. 118, 474–485. doi:10.1016/j.jbusres.2020.07.018
Stock, B., Ferreira, T., and Ernst, C.-P. (2016). Does perceived health risk influence smartglasses usage? 13–23. doi:10.1007/978-3-319-30376-5_2
Sunardi, S., Ramadhan, A., Abdurachman, E., Trisetyarso, A., and Zarlis, M. (2022). Acceptance of augmented reality in video conference based learning during covid-19 pandemic in higher education. Bull. Electr. Eng. Inf. 11, 3598–3608. doi:10.11591/eei.v11i6.4035
Venkatesh, V., Morris, M. G., Davis, G. B., and Davis, F. D. (2003). User acceptance of information technology: toward a unified view. MIS Q. 27, 425–478. doi:10.2307/30036540
Venkatesh, V., Thong, J., and Xu, X. (2012). Consumer acceptance and use of information technology: extending the unified theory of acceptance and use of technology. MIS Q. 36, 157–178. doi:10.2307/41410412
Vinzi, V. E., Chin, W. W., Henseler, J., Wang, H., et al. (2010). Handbook of partial least squares. Springer.
Vorderer, P., Wirth, W., Gouveia, F., Biocca, F., Saari, T., Jäncke, L., et al. (2004). “Mec spatial presence questionnaire (mec-spq): short documentation and instructions for application,” in Report to the European community, project presence. MEC (IST-2001-37661).
Vovk, A., Wild, F., Guest, W., and Kuula, T. (2018). Simulator sickness in augmented reality training using the microsoft hololens. 1–9. doi:10.1145/3173574.3173783
Werrlich, S., Daniel, A., Ginger, A., Nguyen, P.-A., and Notni, G. (2018). “Comparing hmd-based and paper-based training,” in 2018 IEEE international symposium on mixed and augmented reality (ISMAR), 134–142. doi:10.1109/ISMAR.2018.00046
Wild, F., Klemke, R., Lefrere, P., Fominykh, M., and Kuula, T. (2017). “Technology acceptance of augmented reality and wearable technologies,” in iLRN.
Yamaguchi, M., Mori, S., Mohr, P., Tatzgern, M., Stanescu, A., Saito, H., et al. (2020). “Video-annotated augmented reality assembly tutorials,” in Proceedings of the 33rd annual ACM symposium on user interface software and technology, 1010–1022.
Zhao, Y., Ma, C., Huang, H., and Guo, T. (2022). Litar: visually coherent lighting for mobile augmented reality. Proc. ACM Interact. Mob. Wearable Ubiquitous Technol. 6, 1–29. doi:10.1145/3550291
Zhu, B., Feng, M., Lowe, H., Kesselman, J., Harrison, L., and Dempski, R. (2018). Increasing enthusiasm and enhancing learning for biochemistry-laboratory safety with an augmented-reality program. J. Chem. Educ. 95, 1747–1754. doi:10.1021/acs.jchemed.8b00116
Keywords: human-centered computing (HCC), augmented reality (AR), human computer interaction (HCI), users’ behavioral intentions, acceptance model, safety training
Citation: Ismael M, McCall R, McGee F, Belkacem I, Stefas M, Baixauli J and Arl D (2024) Acceptance of augmented reality for laboratory safety training: methodology and an evaluation study. Front. Virtual Real. 5:1322543. doi: 10.3389/frvir.2024.1322543
Received: 16 October 2023; Accepted: 05 February 2024;
Published: 26 April 2024.
Edited by:
Florian Soyka, Institute for Occupational Safety and Health of the German Social Accident Insurance (IFA), GermanyReviewed by:
Chutisant Kerdvibulvech, National Institute of Development Administration, ThailandValeria Orso, University of Padua, Italy
Copyright © 2024 Ismael, McCall, McGee, Belkacem, Stefas, Baixauli and Arl. This is an open-access article distributed under the terms of the Creative Commons Attribution License (CC BY). The use, distribution or reproduction in other forums is permitted, provided the original author(s) and the copyright owner(s) are credited and that the original publication in this journal is cited, in accordance with accepted academic practice. No use, distribution or reproduction is permitted which does not comply with these terms.
*Correspondence: Muhannad Ismael, bXVoYW5uYWQuaXNtYWVsQGxpc3QubHU=