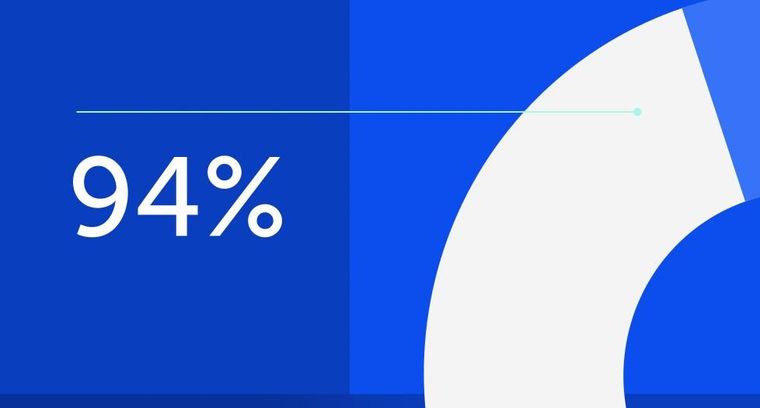
94% of researchers rate our articles as excellent or good
Learn more about the work of our research integrity team to safeguard the quality of each article we publish.
Find out more
ORIGINAL RESEARCH article
Front. Virtual Real., 29 February 2024
Sec. Virtual Reality and Human Behaviour
Volume 4 - 2023 | https://doi.org/10.3389/frvir.2023.1334795
This article is part of the Research TopicVirtual Agents in Virtual Reality: Design and Implications for VR UsersView all 5 articles
Effective navigation and interaction within immersive virtual environments (IVEs) rely on thorough scene exploration. Therefore, wayfinding is essential, assisting users in comprehending their surroundings, planning routes, and making informed decisions. Based on real-life observations, wayfinding is, thereby, not only a cognitive process but also a social activity profoundly influenced by the presence and behaviors of others. In virtual environments, these “others” are virtual agents (VAs), defined as anthropomorphic computer-controlled characters, who enliven the environment and can serve as background characters or direct interaction partners. However, less research has been conducted to explore how to efficiently use VAs as social wayfinding support. In this paper, we aim to assess and contrast user experience, user comfort, and acquisition of scene knowledge through a between-subjects study involving n = 60 participants across three distinct wayfinding conditions in one slightly populated urban environment: (i) unsupported wayfinding, (ii) strong social wayfinding using a virtual supporter who incorporates guiding and accompanying elements while directly impacting the participants’ wayfinding decisions, and (iii) weak social wayfinding using flows of VAs that subtly influence the participants’ wayfinding decisions by their locomotion behavior. Our work is the first to compare the impact of VAs’ behavior in virtual reality on users’ scene exploration, including spatial awareness, scene comprehension, and comfort. The results show the general utility of social wayfinding support, while underscoring the superiority of the strong type. Nevertheless, further exploration of weak social wayfinding as a promising technique is needed. Thus, our work contributes to the enhancement of VAs as advanced user interfaces, increasing user acceptance and usability.
In virtual reality (VR) environments, scene exploration is fundamental, allowing users to understand and navigate immersive virtual environments (IVEs) (Freitag et al., 2018). Effective scene knowledge is thereby vital for decision-making, requiring rapid and accurate exploration techniques (Sokolov et al., 2006). However, to successfully navigate within IVEs, there is a critical need for robust wayfinding support. As stated by Carpman and Grant (2003), “wayfinding ease is part of caring for the user,” while the importance of minimizing cognitive load imposed on users is emphasized by LaViola Jr et al. (2017).
According to Dalton et al. (2019), the concept of wayfinding, however, extends beyond mere psychological higher-order cognitive processes. It takes on the dimension of a sociocultural phenomenon, impacted not only by an individual’s mental navigation but also by the social dynamics at play. Wayfinding becomes a social activity. In our context, wayfinding is shaped by the presence and actions of others surrounding the navigating individual, known as synchronous wayfinding.
Based on real-life observation, Dalton et al. (2019) differentiated between two primary types of social wayfinding: strong and weak. For each type, we will provide a definition, also summarized in Table 1, and how this social setting can be emulated with computer-controlled anthropomorphic virtual agents (VAs).
TABLE 1. According to Dalton et al. (2019, Table 1), strong and weak social wayfinding can be clearly distinguished through six key characteristics. For comprehensibility, we included the following aspects in italic font: category terms per characteristic and a few supplementary aspects for characteristic expressions.
The first type, termed strong social wayfinding, involves deliberate and intentional support to an individual through navigational assistants who actively provide wayfinding information. Communication in these scenarios is typically bidirectional, occurring through verbal or gestural means, often in close proximity for effective exchange. The navigational assistants are consciously known by the individual, with typically only a few or a single assistant. By applying these principles in the realm of VR, strong social wayfinding can be seamlessly emulated using a virtual guide, guiding the VR user through an IVE, while imparting knowledge about visited places or traversed routes.
In contrast, weak social wayfinding refers to the influence of indirect and unintentional support from the social environment, where individuals are guided by non-verbal and distal cues and actions of many others. Those others providing the mainly directional, social cues are referred to as strangers, as they do not engage in direct communication with the individual. This concept, thus, resembles the observation by Munro et al. (1999) that people tend to follow established paths. It is also related to following behavior (Helbing et al., 2000), herding (Baddeley, 2010), the bandwagon effect (Leibenstein, 1950), and the principle of conformity (Chang et al., 2020), all of which illustrate how people tend to adopt behaviors influenced by social cues. By applying these principles in the realm of VR, weak social wayfinding can be seamlessly emulated using orchestrated flows of VAs connecting important locations within a given IVE, subtly guiding the VR user through the scene. Despite being deliberately added from a scene designer’s point of view, these pedestrians-as-cues still provide indirect and unintentional support from a VR user’s perspective. Thus, they are unintentional signifiers (Jerald, 2015), seamlessly blending into the IVE, enhancing realism while mimicking social behavior and directional cues present in the real world.
This research aims to offer a comprehensive evaluation and comparison of the effects of strong and weak social wayfinding techniques in architectural environments, in contrast to unassisted navigation within the same IVE. Our primary focus centers on assessing the impact of these techniques on user comfort, overall experiential quality, and acquisition of spatial knowledge. By contrasting the outcomes, our objective is to provide a holistic understanding of the unique benefits and limitations associated with each approach, ultimately contributing to a deeper comprehension of the role and significance of social wayfinding strategies in IVEs.
Compared to prior work, our research introduces three key contributions to the field: first, we have developed a novel concept of a virtual supporter, representing the strong social wayfinding support, which fulfills a dual role as both a wayfinding guide and a knowledgeable companion. Second, we have devised an intricate methodology for simulating virtual pedestrian flows, serving as a form of weak social wayfinding for enhancing scene exploration. Third and finally, to evaluate the effectiveness of our virtual supporter and virtual pedestrian flows, we conducted a comprehensive between-subjects user study. This study aimed to compare the performance of our new designs against unsupported scene exploration, focusing on key factors such as cognitive load, acquired scene knowledge, and user comfort.
As outlined by Cohen (1985) and Best (2012), VAs embedded as virtual guides fulfill two primary roles: (i) as mentors, VAs enhance users’ comprehension by providing contextual information and insights during scene exploration (Carrozzino et al., 2018); (ii) as pathfinders, they guide users through IVEs, ensuring a structured experience (Chrastil and Warren, 2013), while preventing users from missing vital information or becoming disoriented in intricate scenes (Liszio and Masuch, 2016).
Focusing on a VA’s role as a mentor, research highlights the positive impact of virtual guides on the user experience, including increased engagement and attention (Ibanez et al., 2003b; Chittaro and Ieronutti, 2004). Virtual guides were found to outperform text panels and voice-only conditions in content understanding (Carrozzino et al., 2018), while some participants preferred audio-only conditions for learning due to subjectively perceived reduction of distractions (Rzayev et al., 2019a; Rzayev et al., 2019b). Therefore, we aim for a balance between human-like vitality and composed demeanor through deliberate gestures and tranquil postures. Anthropomorphic characters such as virtual guides, as opposed to audio-only conditions or human-like robots, were, moreover, perceived as more realistic and enhance social presence (Rzayev et al., 2019a; Rzayev et al., 2019b). Hoffmann et al. (2009) state that this sense of social presence frequently leads participants to interact with virtual guides, similarly to how they engage with real humans, which is consistent with the Media Equation theory (Reeves and Nass, 1996; Hoffmann et al., 2009). To further enhance the social presence, it is, moreover, crucial for virtual guides to align their behavior with user expectations (Ibáñez-Martínez et al., 2008), incorporating factors such as gazing (Martinez et al., 2010; Pfeiffer-Lessmann et al., 2012; Pejsa et al., 2015) and gestures (Jerald, 2015). Additionally, natural user–agent interaction can be enhanced by including linguistic style adjustments as found in research by, e.g., de Jong et al. (2008) in terms of politeness and formality, or by Ibanez et al. (2003a) and Ibanez et al. (2003b) in terms of the audience and the VA’s background and character. This aligns with findings that virtual guides with personalities (Bates, 1994; Lim and Aylett, 2007; Lim and Aylett, 2009) and storytelling abilities (Isbister and Doyle, 1999) can enhance engagement. For the virtual supporter presented in this work, we aimed at designing a friendly and humorous VA, offering the flexibility of human-in-the-loop interactions and automatic agent behavior.
Focusing on a VA’s role as a pathfinder, it is evident that VAs can successfully guide users through scenes. Doyle and Hayes-Roth (1997a), e.g., demonstrated that a VA can, thereby, select the next location to be visited based on user feedback, while Cao et al. (2021) proposed a system in which a predefined tour can be adapted based on user preferences, allowing them to skip locations and revisit them later. Nevertheless, using strictly predefined tours (Ibanez et al., 2003b; Chrastil and Warren, 2013; Liszio and Masuch, 2016) is still common. In addition to taking the full responsibility for wayfinding, VAs can also function as companions, as exemplified by Ye et al. (2021), while maintaining a “location-based sense of contextuality” (Ibanez et al., 2003b) when following the user through the IVE. This context awareness enhances user–agent interactions by tailoring shared knowledge and actions accordingly. Research by Bönsch et al. (2021b), comparing strict guides and knowledgeable companions suggests that a hybrid role, leveraging both conditions, may be best suited for enhancing user acceptance in VR, enabling an interactive and adaptable, yet structured learning experience during scene exploration. One possible design of this hybrid role is given in Section 3.2. Here, we also incorporate socially compliant behavior (van der Heiden et al., 2020) for the VA, considering interpersonal distance preferences, ensuring that the VA approaches users at an appropriate distance and adapts its trajectories to user needs, as suggested by Jan et al. (2009) and Ye et al. (2021).
In the realm of our research, two factors are key when considering pedestrian flows: (i) understanding whether pedestrians can influence user behavior and (ii) implementing a natural flow.
Various research studies have explored if and how virtual characters influence a VR user’s behavior. Watanabe et al. (2020) used static virtual crowds to draw attention to specific shops, while Yoshida et al. (2018) as well as Yoshida and Yonezawa (2021) used virtual pedestrians with adjustable walking speed and gaze direction to draw users’ attention toward advertisements. Zhao et al. (2020) highlighted the importance of copresent avatars representing other participants, improving wayfinding accuracy through real-time interaction, while Ibáñez-Martínez et al. (2008) demonstrated the effectiveness of animal flocks as spatial cues to direct users’ attention toward important locations in large-scale IVEs. Ríos et al. (2018) as well as Rios and Pelechano (2020) emphasized the influence of group behavior and the likelihood of conformity, even in small groups. In a video-based study, Bönsch et al. (2021a) observed that participants tend to naturally align with simulated pedestrian flows, suggesting the effectiveness of this approach for subtly guiding users through IVEs.
Consequently, research consistently demonstrated that the use of virtual characters can effectively direct users’ attention in IVEs. Extending the work of Bönsch et al. (2021a), our second contribution is a more comprehensive exploration of pedestrian flows in IVEs. By recognizing the significance of a positive user experience by avoiding any adverse effects resulting from poor flow design, we, thereby, consider the state of the art in flow modulation.
Various methods have been developed to realistically embed virtual pedestrians into IVEs. These techniques focus primarily on simulating crowd behavior, ensuring autonomous and socially compliant movements of virtual pedestrians. A comprehensible overview of agent-based crowd simulations was recently provided by van Toll and Pettré (2021). To enhance the realism, there has been a growing emphasis on introducing diverse and context-specific behaviors within virtual crowds. This includes incorporating role-dependent behavior (Bogdanovych and Trescak, 2017), using influence maps to simulate specific needs (Krontiris et al., 2016), and emulating real-life visiting patterns (Levasseur and Veron, 1983; Chittaro and Ieronutti, 2004).
In the context of social interactions, real-life observations in communal spaces indicated that a significant portion of individuals move in social groups, a phenomenon often mirrored in virtual pedestrian behavior (Rojas and Yang, 2013). Different group formations are, thus, often used and adjusted dynamically based on the current situation, aligning with social preferences (Peters and Ennis, 2009; Moussaïd et al., 2010; Rojas and Yang, 2013; Fu et al., 2014). In this work, we, however, decided to stick to single agents to maintain greater control over the flow.
To further enhance the naturalism of the IVE, visual distinction of the virtual pedestrians is crucial to avoid the perception of clones. To this end, various strategies have been proposed ranging from using different representative body shapes while avoiding extreme variations (Shi et al., 2017) over avoiding appearance clones by incorporating diverse accessories and color variations in clothing, hair, and skin tone, especially for heads and upper torsos (McDonnell et al., 2008; McDonnell et al., 2009), to avoiding motion clones using out-of-sync motion timings to effectively achieving visual distinction (McDonnell et al., 2009). For smaller crowds (8–24 characters), Pražák and O’Sullivan (2011) thereby suggest using three distinct motions, with timing variations for each character. For larger crowds exceeding 250 pedestrians, Adili et al. (2021) found that more than one distinct walking motion per gender does improve perceptual realism, suggesting that a limited variety of motions suffices.
In the context of urban environments, two significant features emerge: points and areas of interest. Although points of interest (POIs) refer to significant items or locations in a given IVE, such as a single statue, areas of interest (AOIs) are defined as relevant and distinct regions within the scene, characterized by well-defined boundaries such as a park, which themselves encompass multiple POIs. In this regard, POIs can either fall within an AOI, rendering them AOI-contained, or exist as isolated and autonomous entities, referred to as individual POIs.
For effective wayfinding in unfamiliar IVEs, virtual guides as direct supporters can help users navigate these scenes. Research by Bönsch et al. (2021b), however, indicated that neither a virtual guide nor a knowledgeable companion in a virtual museum fully met participants’ diverse support requirements. In light of their findings, we propose a hybrid approach to enhance wayfinding support, dynamically combining guiding and accompanying modes based on the specific scenic features. In the guiding mode, the supporter leads users in a structured manner efficiently to individual POI and AOI entrances, reducing search time, especially in complex and large-scale IVEs. In the accompanying mode, users explore the manageable section of an AOI independently, having a sense of autonomy, while the supporter ensures no critical elements are missed and that the tour resumes once all AOI-contained POIs have been visited.
The effectiveness of and seamless transition between both modes relies on the VA’s in-depth scene knowledge, achieved through annotated environments (Doyle and Hayes-Roth, 1997b; Kleinermann et al., 2008). To provide a more flexible and natural user experience, we extended the work of Bönsch et al. (2021b) as follows:
• Avoiding the previous sole usage of shortest paths, we introduced a set of waypoints for controlled and precise user guidance to individual POIs.
• Avoiding the limitation of predefined VA positions next to POIs, our VA dynamically adjusts its location based on the user’s position and focuses randomly on different parts of the POI during explanation.
• We also store the VA’s utterances for each POI. We, however, differentiate between introductory prompts given while traveling to individual POIs, explanatory prompts with the main knowledge a user should gain, and supplementary prompts for interested users with additional information.
• We introduced attention volumes, encompassing the POI’s axis-aligned bounding box and related items, to assess whether the user’s focus is on the POI and thus enhance user–agent interactions.
• For AOIs, we also store the set of waypoints, alignment circle, spherical gaze target zone, and introductory and explanatory prompts. Furthermore, we provide a list of AOI-contained POIs and their visitation status, along with exit markers triggering specific VA prompts on users entering them, such as resuming the tour if all AOI-contained POIs have been visited or notifying users of missed POIs.
Our aim is to create a realistic, human-like, and responsive virtual supporter for scene exploration. Our primary focus is, thereby, on its locomotion behavior, while also controlling the VA’s gaze and speech behavior. To this end, we combined the foundational behavioral units of a guide provided by Bönsch et al. (2021b) with the group navigation framework outlined by Weißker et al. (2020). This results in a custom four-stage interaction process for scene exploration support (Figure 1): (i) The VA awaits and welcomes the user (forming). (ii) The VA clarifies its role and the requested user behavior: follow in the guide mode and explore freely in the companion mode (norming). (iii) The VA and the user switch between walking to the next POI/AOI or standing at a POI or an AOI entrance, with the VA imparting knowledge to the user (performing). (iv) The VA concludes the scene exploration by bidding farewell (adjourning). In our implementation, the VA’s verbal communication is pivotal for clarifying its current role and guiding the user throughout the outlined stages. The VA’s conversational component thereby includes speech with lip sync, while utterances can be played, paused, resumed, and stopped at any time as required for a user-aware behavior, while a set of non-semantic, co-verbal gestures is added. This setting is enhanced by a natural gazing behavior, using a procedural gaze approach by Pejsa et al. (2015), which synchronizes the movements of the VA’s eyes, head, and torso toward a specific target point, such as the user, a POI, or the VA’s walking direction. Finally, footstep sound is added for quick localization, enhancing of spatial awareness, and fostering of natural interaction.
FIGURE 1. Adaptation of the four stages of joint navigation in a shared immersive virtual environment (Adapted from Weißker et al. (2020), Figure 2; with permission from IEEE) to the specific use case of exploring a virtual city with a virtual supporter switching roles between being a virtual guide and being a knowledgeable companion.
The VA’s behavior can be customized using various parameters, detailed as follows. The provided values stem from rigorous internal testing in our target evaluation scenario, supplemented where possible by literature-based ranges.
Upon a user’s scene entry, the virtual supporter initiates the forming stage by approaching the user while maintaining an appropriate interpersonal distance (2 m). Afterward, the VA offers a concise personal introduction to enhance user engagement. Additionally, it provides contextual information about the scene to be explored collaboratively, providing users with a clearer understanding of the upcoming exploration. The VA then transitions to the norming stage by clarifying the navigational responsibilities: if the first encounter occurs at an entrance or inside an AOI, the virtual supporter introduces the respective area before switching to the companion mode, asking the user to explore the area while accompanying the free exploration. Otherwise, it informs the user of its intention to guide them to the first POI or AOI.
Next, the performing stage is entered, where we differentiate between (i) transitioning between the scenic features (discussed here) and (ii) the VA imparting knowledge to the user at a POI or an AOI entrance (Section 3.2.4).
During guided transitions, the virtual supporter guides the user along the specific route determined by predefined waypoints, allowing direct paths while still covering important areas of the scene. We enhance the otherwise boring transitions by conversational prompts from the VA per waypoint. These prompts may be directional (“We are taking a right turn here.”), progress-related (“We are almost there.”), humorous (“My feet hurt from all of this walking.”), or simply small talk (“The weather today is so nice, you can even see the mountains in the distance.”). Moreover, we aim to design a user-aware and responsive VA for a dynamic and interactive experience. To this end, we refined the transition system proposed in Bönsch et al. (2021b) (Figure 2A), with a specific focus on the VA’s gazing and speed component to enhance the joint locomotion between both interactants. Thereby, we expect the user to follow the VA closely or even engage temporarily in a side-by-side formation.
FIGURE 2. (A) Interplay of VA (boxes) and user (arrow labels) in the dynamic transitions during the guide mode with d as interpersonal distance and di as specific thresholds (defined per use case), extending and refining (Reproduced from Bönsch et al., (2021b); with permission from ACM Digital Library). The gaze adjustment, detailed in (B), incorporates the angle between the user (in green) and VA (in blue) and a time component for natural behavior, while the walking speed adjustment, detailed in (C), considers the interpersonal distance between the user and VA.
A walker’s gaze direction can be used to infer the walking trajectory (see, e.g., (Nummenmaa et al., 2009)). Marigold and Patla (2007) emphasized that walkers naturally focus on zones offering maximum information to avoid obstacles and ensure a safe trajectory, applicable to both static environments (Marigold and Patla, 2007) and populated areas where pedestrians concentrate on collision-prone individuals (Meerhoff et al., 2018) and visual cues, as confirmed for real-life and VR by Berton et al. (2020). Consequently, our VA should mimic this behavior by maintaining a forward gaze during movement. However, a sense of connection is required in a dyadic interaction such as ours, as outlined by Goffman (1971). Thus, the VA tries to keep the nearby user engaged by regularly making eye contact (Figure 2B). If the user enters the VA’s visual range, determined by angle β
Typically, the VA maintains a constant walking speed (2 m/s). However, when the user falls behind, surpassing a predefined threshold d1 (3 m), the VA reduces its speed (by 10%), allowing the user to catch up comfortably (Figure 2C). This adaptive slowing keeps the user engaged and prevents them from losing sight of the VA. Once the user catches up, the VA resumes its initial walking speed.
If the user–agent distance remains rather constant for 8 s after the VA turned, it can be inferred that the user has stopped to inspect a scene element. To re-engage the user in the tour, the VA addresses the user directly (e.g., “Hey over here.”), before adopting a patient stance, allowing the user sufficient time to complete their inspection.
If the user approached the VA again up to d < d2 (4 m), indicating a successful user re-engagement, it resumes its guidance. This approach thus allows users to shape their experience with small scene inspections aside the predefined tour.
If the user, however, exceeds a threshold d4 (10 m), the VA catches up walking faster than the user’s maximum steering speed. After approaching the user to a threshold d5 (4 m), the VA addresses the user in a friendly but determined manner, asking for continuing the tour. If the user departs further, this behavior is repeated. Otherwise, the tour resumes by returning on the shortest path to the last waypoint where the dyad was initially heading.
Transitioning into the companion mode inside an AOI, the virtual supporter aims to maintain a side-by-side formation with the user, allowing unhindered freedom of movement while fostering a sense of companionship. To achieve this, the concept of two target positions (TSleft and TSright), called slots, used by Bönsch et al. (2021b) was adapted, with slight positional modifications. A larger interpersonal distance dprox (2.5 m) was chosen to allow safe navigation without colliding with the user or invading their personal space. Moreover, the target slots were positioned closer to the user’s field of view boundaries using a larger angular offset α (50°). This was done to mitigate the sensation of “pushing the VA around,” a concern reported by participants in Bönsch et al. (2021b). The new target positions were determined using Formula 1 with the user’s position Pu, the normalized forward vector Uforward, and the rotation matrix R(α) describing a rotation around the world-space up-vector by α.
The VA’s task is to maintain a close proximity to a target slot and thus the user. Formula 2 models the VA’s speed SVA dynamically in response to changes in the user’s walking speed SU and the relative Euclidean distance between both interactants (dbehind). The second factor is scaled by a fixed coefficient f (0.2 s−1), avoiding too strong influences of distance in close proximity but also allowing the virtual supporter to catch up when lagging too far behind.
If a user enters an AOI’s exit marker, the VA infers the wish to leave. If not all AOI-contained POIs have been visited, the VA informs the user about missing spots and allows them to decide whether to stay or continue. If all AOI-contained POIs have been visited, the VA switches back to the guiding mode and guides the user to the next POI or AOI.
To get to know when to start a respective explanation and thus move on to the second part of the performing state, the VA in the companion mode is required to infer the user’s interest in a POI. This is done by our interest inference method, also used later to determine the appropriate amount of information to be shared with the user at an individual POI.
Foulsham et al. (2011) found that humans tend to maintain objects of interest at the center of their visual field. Thus, we trace a sphere-shaped volume along the user’s line of sight (sphere radius: 0.6 m, tracing distance: 20 m), efficiently detecting collisions along a narrow path. If the user fixates a POI, described by the attention volumes of the respective POI and an information sign associated to it, or the VA for a certain duration, interest is likely. To fine-tune the detection, proxemics is considered as well, as a user tends to approach the currently regarded POI. Consequently, when a user maintains uninterrupted focus on a POI for a specified duration tf (5 s) while situated within a proximity smaller than the distance threshold df (5 m), their interest in that POI is inferred, even if a closer POI exists. As a result, the virtual supporter strategically positions itself at the respective POI and initiates the explanatory prompt. Alternatively, if the user focuses on the VA for tf, it is interpreted as seeking for information, triggering the need to determine the currently regarded POI. In such instances, our heuristic identifies all AOI-contained POIs within the distance threshold df around the user and finally selects the closest POI to the user. Subsequently, the virtual supporter positions itself appropriately at the respective POI and initiates the explanatory prompt. To handle all POIs equally, these prompts are intentionally designed to have the approximately same length, while the important aspects are noted as bullet points on information signs located at the respective POI.
After guiding the user to a specific individual POI or inferring the user’s interest within an AOI, a positioning algorithm continuously positions the virtual supporter at a strategic location to share information about the scenic feature.
The virtual assistant should be positioned near the POI or AOI entrance to facilitate a seamless connection between information and visual experiences, ensuring a natural and user-focused experience by providing an unobstructed view of the scenic feature. It should also allow users to circle smaller POI-like statues to maintain engagement while the VA walks along naturally.
To this end, the position-aware virtual agent locomotion technique (PAVAL) (Ye et al., 2021) was used as baseline. After a pre-study by Ye et al. to determine the subjective optimal positioning of a guide in front of a POI based on different user-to-POI distances, PAVAL provides an energy function, optimizing the agents’ position and orientation for arbitrary user-to-POI distances, ensuring a good balance between timely position adaptions based on user movements and the avoidance of unnecessary shifts. We extended this baseline, by redefining the reference point (POI center in approach of Ye et al. (2021)) to be located on the alignment circle associated with the respective POI. This modification allows for an easier account of the size of the scenic feature by considering the radius of the alignment circle: the extended approach involves four steps, summarized in Figure 3: (i) defining the reference point as the intersection of the alignment circle and the vector connecting the circle’s center with the user’s position, (ii) determining the two optimal positions for the VA as done in PAVAL, (iii) moving both optimal positions toward the POI by a distance dback (1 m) accounting for the reference point being farther away from the POI in our approach, and (iv) using the corrected optimal positions to refine the current position of the VA as done by Ye et al. (2021).
FIGURE 3. User circling a POI while getting closer to look at details, sketched in four time steps. During this process, the optimal right and left positions for the virtual supporter are computed, based on our extended version of PAVAL by Ye et al. (2021).
While imparting knowledge, the VA shall maintain a predominant gaze toward the user. Periodic shifts toward the scenic feature, more concretely a random point within the predefined spherical gaze target zone associated with the respective POI or AOI, are used to direct the user’s focus to the POI or AOI. The established gazing pattern involves alternating between maintaining an eye contact with the user for a certain duration tuser (6–9 s) and redirecting its gaze to the scenic feature for a duration of tfeature (2–3 s).
If a user departs while the VA is still in its explanatory prompt, the VA pauses its monologue and catches up with the user and asks them to return to the POI or AOI entrance. Once they both returned, the VA resumes the explanatory prompt, as this information is crucial to be conveyed.
If the user leaves while the VA is providing the supplemental prompt, this action is interpreted as disinterest. The VA terminates its speech, as the additional information on the POI is optional, reentering the norming stage to clarify its mode before transitioning back to the performing stage. In the guiding mode, the VA then directs the user to the next scenic feature, while in the companion mode, the VA encourages the user to explore the AOI further or informs them that all contained POIs have been found.
As the virtual supporter introduces the POI, an attention estimate e is tracked based on the sphere trace detailed earlier, reflecting the user’s focusing time on the POI or the VA. The time during which the user does not gaze at the feature or VA is deduced from e with a factor c > 1 (1.5), subject to e ≥ 0. This scaling improves the representation of diminishing interest, leading to a more accurate representation of user engagement dynamics. The duration’s final value determines the user’s interest or disinterest throughout the conversation. A value exceeding the threshold ϵ (5 s) indicates interest, while a value below it signifies disinterest. This threshold allows fine-tuning without changing the decrease rate and depends on the average length of explanatory prompts (M = 16.54 s, SD = 3.39). If the user is inferred as interested, the VA automatically provides the supplemental prompt. If the user is inferred as uninterested, the VA lets the user choose between being provided the supplemental prompt or continuing the exploration. Continuation has three possible scenarios: (i) if the POI is the last in the scene, the VA will bid farewell; (ii) if there are undiscovered POIs, the VA will (a) provide an introductory prompt for the next destination and initiate the guiding process when being in the guiding mode or (b) signal the user to continue their free exploration when being in the accompanying mode.
The supported scene exploration ends when the final POI, either individual or AOI-contained, is explained. In our implementation, the virtual supporter initiates the adjourning stage by bidding farewell and leaving, allowing the user to navigate the city independently.
Virtual characters effectively direct users’ attention within IVEs. Bönsch et al. (2021a), e.g., introduced a subtle wayfinding technique that uses virtual pedestrians as social cues to steer users indirectly and subtly as they explored a scene. Despite limited interactivity and motion freedom, participants in the video-based evaluation generally followed the pedestrians as intended. However, a more sophisticated VR-based analysis is needed to validate and refine this weak social wayfinding technique. To this end, we extended their approach as detailed below.
Although users freely explore a scene, we aim to subtly direct them toward both important scenic features: POIs and AOIs. Moreover, participants should also retain their autonomy inside AOIs; hence, directional guidance to specific AOI-contained POIs is not required. This way, we mimic the strong wayfinding support by our virtual supporter in a more subtle manner.
To subtly direct users to POI and AOI entrances, metrics are required to automatically determine appropriate locations. As proposed by Freitag et al. (2018), this can be achieved by assigning priority scores to scenic features and focusing on the top three scoring options for guidance. These priorities can be determined through methods like static, object-based metrics (Freitag et al., 2015) or dynamic distance-based scores with closer POIs and AOIs receiving higher scores than distant ones. In our subsequent evaluation, we set initial priority scores manually for simplicity.
To provide effective guidance toward undiscovered places, priority scores for each feature must be continuously updated as users explore the scene. One option is that the priorities gradually decrease to zero as users explore the respective scenic feature, allowing for new flows to unseen POIs and AOIs, and then gradually restore them for later revisits. In our implementation, visiting an individual POI resets the corresponding priority to 0, while visiting an AOI-contained POI reduces the respective AOI’s priority by the POI’s score, until all POIs are visited and the AOI priority is 0. Consequently, this approach also reduces the number of pedestrian flows, resulting in a clear endpoint for scene exploration with weak wayfinding support.
To ensure that the pedestrians of the individual flows visually inspect the scenic features, guiding the user’s focus to it, an inspection circle is manually defined around each individual POI and around the central point of AOIs. For the latter, this strategic approach allows guiding agents to subtly steer users toward an AOI’s center, fostering exploration, while avoiding directional cues to allow users to uncover the area at their own pace.
The virtual pedestrians’ behavior can be customized using various parameters, detailed below. The provided values stem from rigorous internal testing in our target evaluation scenario, supplemented where possible by literature-based ranges.
As defined by Bönsch et al. (2021a), guiding agents form pedestrian flows and serve as social cues to guide users indirectly and subtly through the IVE. Although pedestrian flows in real-life typically consist of social groups with different group formations (Peters and Ennis, 2009; Rojas and Yang, 2013), our approach uses single VAs, moving in a socially compliant manner (van der Heiden et al., 2020), to maintain control, providing a foundation for future studies on social group dynamics during the guiding process.
As proposed by Freitag et al. (2018), our approach initiates three pedestrian flows near the user’s current location (Figure 4A). Each flow is directed toward a distinct POI or AOI with high priority scores. Each pedestrian in a flow thus shares a common destination but has a unique random point within the inspection circle for enhanced realistic behavior. When reaching this location, the guiding agent stops and turns toward the POI’s center to inspect the location for some time, expressing appreciation, via clapping or gesturing. The inspection time varies randomly within a predefined range, resulting in slightly different behaviors for more variation (1–5 s). When the agent’s inspection time expires, it selects a new random point within the inspection circle and starts another inspection cycle. This continues until the scenic feature’s priority is reset to 0, marking the location as visited. After completing its current inspection cycle, the pedestrian then proceeds to guide the user to a new unvisited place.
FIGURE 4. (A) Scheme showing three pedestrian flows to individual POIs and AOIs, while the fourth scenic attraction is ignored for now. (B) Demonstration of how guiding agents are handled in relation to the user’s position and orientation within the IVE: VAs outside the user’s attention region, which is attached to his or her head, are subject to relocation or removal, while VAs inside this region proceed toward their destination. (C) A flow which is formed by integrators is diversified by VAs merging from a random direction or VAs traveling along parallel streets. The cross symbolized the direction change during the relocation process. (D) Two base pedestrians as background noise either crossing the flow or walking in the opposite direction.
To enable pedestrian flows to align with the user’s locomotion behavior, the algorithmic framework must establish a creation–relocation–destruction loop, described next.
On starting the simulation, we aim to quickly establish initial flows toward the three highest-ranked scenic features by creating and spawning a basic set of guiding agents, with the same number of VAs assigned to each flow (20 VAs per flow).
During scene exploration, guiding agents are automatically created and spawned behind the user, extending each pedestrian flow with new VAs. This is especially critical to form continuous pedestrian flows in long, unobstructed streets, ensuring constant access to subtle directional cues. The additional guiding agents are spawned at a predefined rate (3/s). To conserve system resources and prevent overcrowding, the continuous spawning is temporarily deactivated when a user is inside an AOI or close to an individual POI (inspection circle’s radius +30 m).
Guiding agents are, thereby, not tied to a specific scenic feature, but to a top-three priority level set, namely, top-1, top-2, or top-3. Thus, when a new set of top-3 features is defined, the guiding agents are automatically rerouted to their new destinations.
To create the impression of a vivid IVE, it is crucial to populate those areas the user sees and might walk to next. This region can be visualized as a capsule, created by combining a rectangle with semicircles at both ends (Figure 4B), termed user’s attention region. The shape is defined by two parameters: capsule’s half-height h comprising half of the rectangle’s height plus the semicircle’s radius (66 m) and radius r as the semicircle’s radius (48 m).
To handle the pedestrians outside this attention region, we distinguish between two types of guiding agents: (i) integrators shape an established flow, subtly guiding users within it. They are destroyed on leaving the user’s attention region and are replaced by newly spawned VAs. This approach maintains a consistent flow while addressing the challenge of population density. (ii) Diversifiers are strategically used to improve the naturalness of the pedestrians’ paths. They randomly merge from diverse trajectories into the existing flow or take side streets to complement their flow’s primary direction. They comprise the set of initially spawned guiding agents and are continuously relocated to rejoin the visible action and are only destroyed when the number of pedestrian flows decreases. The relocation process thereby encompasses the following sequence:
Step 1. Teleportation
The initial step in repositioning a diversifier is to teleport it to a point inside a pedestrian-accessible region (green area in Figure 5A). Therefore, a spatial grid (200 m × 200 m) is established around the user’s current position, with points evenly sampled at equidistant intervals (5 m). Any point visible by the user or those behind the user is discarded (blue points in Figure 5B). A linear proximity score is computed for each remaining candidate point, assigning higher scores to those closer to the user (color range from green to red). A predefined threshold ϵ
FIGURE 5. (A) Green navigation mesh of a scene excerpt with red areas assigned higher navigation costs to avoid population in these areas. (B) Sampling for finding a suitable teleportation spot for guiding agents. (C) Sampling for finding a suitable interim location before progressing toward the assigned POI or AOI for guiding agents. Created with Unreal Engine.
Step 2. Diversifying the flow
Next, an interim point for its further travel is determined by sampling a spatial grid (140 m × 140 m) around the user, similar to the previous step. The approach uses varying parameter values (spacing of 10 m, ϵ ≥ 75%) and an inverse scoring, with higher scores for more distant points (Figure 5C). After finding an appropriate interim point, the guiding agent is directed toward it. This results in the VAs either going down a street parallel to the direction of their flow, enhancing pedestrian movement patterns in the scene, or integrating with their flow taking different paths to increase the believability of flow generation (Figure 4C).
Step 3. Guiding to scenic feature
When a diversifier reaches the predetermined interim point, it is smoothly guided toward its destination. To this end, a random point within the inspection circle of the respective POI or AOI entrance is determined and set as a new walking destination. As the VA progresses toward its destination, it assists users in two modes: (i) as a member of the main flow, the VA guides through collective movement; (ii) while traversing parallel streets, the VA reinforces the significance of the specific direction when being visible at intersections.
As guiding agents improve the liveliness only in a confined scope, we also added base agents to travel randomly and autonomously across the environment, adding to the IVE’s vibrancy. In addition, variations in their locomotion paths, such as enlivening side streets and countering or intersecting the guiding agents’ flows, ensure users do not perceive all VAs as moving away. To prevent creating another pedestrian flow that may mislead the user, it is crucial to develop a variety of random directions for the base agents, achieved by using a similar approach to the guiding agent’s creation–relocation–destruction loop.
As for the guiding agents, base agents spawn unnoticeably outside the user’s field of view, with their main trajectories steering within the user’s field of view. To achieve this, multiple spawners are distributed within the IVE, creating as many permanent base agents as needed for the background noise population (initially 20 VAs, +5 VAs per deleted pedestrian flow). Additional spawners are activated to enrich the population in large, open urban spaces, creating temporary base agents. The number of these VAs depends on the space’s dimensions and is manually defined per space.
As for the guiding agents, only the user’s attention region needs to be populated. We opted for a circular region with a radius r (60 m, Figure 4D).
When a base agent exits the circular region, its handling depends on its characteristic: a permanent VA is repositioned and assigned a new walking direction, while a temporary VA is destroyed if the user leaves the respective open space; otherwise, it is repositioned as well.
As for guiding agents, the teleportation (grid size: 160 m × 160 m, spacing of 6 m, ϵ ≥ 75%) and walking locations (grid size: 120 m × 120 m, spacing of 10 m, ϵ ≥ 75%) were determined using the same approach. This time, however, the determined location is not only an interim point but also the final location for the base agent’s movement. Consequently, base agents commence their new trajectories in proximity to the user, walking toward a randomly selected point that is situated at a greater distance from the user but remains within the potential user’s line of sight. This ensures their visibility, as they either intersect with the user’s path or populate visible side streets. In case they reach their assigned destination while still being in the user’s attention circle, they are redirected by being sent to another random point within the user’s attention circle.
To compare the performance and user preferences between our strong and weak social wayfinding approaches, we chose a between-subjects design with the provided wayfinding support being our independent variable. This results in three conditions: Cfree in which no wayfinding support is granted, Csupporter with strong support, and Cflow with weak support. As an ecologically valid setting for studying user comfort, spatial memory, and object recognition, allowing insights into active and passive exploration effects (Attree et al., 1996), we used a generic and versatile approach by immersing participants in a virtual city with an intricate layout and a multitude of paths and exploration options and tasked them to freely explore this IVE, adhering to their respective assigned conditions.
We expected the following hypotheses to be fulfilled:
H1. Gaining knowledge about the virtual city is more successful in Csupporter, followed by Cflow and then by Cfree.
H2. The subjective task load is smallest for Csupporter, followed by Cflow and then by Cfree.
H3. Exploring a sparsely populated virtual city with a dedicated virtual scene expert (Csupporter) is more enjoyable and comfortable than navigating it indirectly through pedestrian flows (Cflow). Furthermore, Cflow is preferable to exploring the city without any support (Cfree).
We grounded these hypotheses on the fact that an active, structured supporter (Csupporter) relieves participants from the cognitive load to navigate themselves for most of the time (H2) and ensures that all POIs are visited (H1). Furthermore, guiding flows (Cflow) also contribute to this, while still requiring participants to choose directions and gain knowledge about POIs by themselves. As a result, we anticipate that acquiring information will be more difficult in Cfree, which will also raise the subjective task load.
H3 is based on the expectation that participants value both levels of support (Csupporter, Cflow) as being more convenient and enjoyable. Therefore, the first part of this hypothesis is rooted on the assumption that VR users value the interactive component, e.g., that the supporter dynamically reacts on their behavior, as well as the realized balance between being structurally guided and having the autonomy to freely explore specific, information-dense regions. Additionally, we expect participants to appreciate the indirect guidance of agents simulating real-life human behavior when there is a lack of explicit guidance. However, due to a lack of tailored support, this appraisal is expected to be weaker compared to that from an interactive guide, who offers more structure and keeps participants engaged and curious about the city.
We used an HTC Vive Pro 2 headset at 90 Hz, tracked in a 3.5 m × 3.5 m area with two tripod-mounted SteamVR Base Stations. The build-in headphones were worn at all times, but only used in Csupporter for the VA’s utterances. Participants were equipped with two HTC Vive controllers. In all three conditions, the left controller displayed the remaining exploration time and progress percentage. Moreover, participants used one controller of choice (right or left depending on handedness) for navigation. Walking speed was controlled via the controller’s touch pad, allowing a speed range of 0–2.5 m/s, with minimal adjustments by natural walking.
We designed the fictitious, complex, large-scale, and pedestrian-only city North Bucbrook as a test scene (Figure 6). It included a set of base agents (Section 3.3.2) wandering through the city, adding vibrancy. A total of sixteen locations can be found: six individual POIs and two AOIs, each with five POIs. These locations were strategically chosen to balance walking time while maximizing scene coverage during exploration. We included 14 obvious POIs, representing locations that were easy to find, while the lantern and the car were hidden. Each POI had one or more information signs, essential for scene knowledge in Cfree and Cflow.
FIGURE 6. An overview of the slightly populated North Bucbrook with six POIs (A, C, D, F, G, and H) and two AOIs (B and E) with five contained POIs each. Created with Unreal Engine.
The base agents, guiding agents, and their animations were taken from the Aglobex Character Pack, (2023), allowing a large variety of visual appearances for flow plausibility (Figure 7), with a maximal walking speed of 1.8 m/s. As a supporter, we employed a male MetaHuman, 2023 character (Figure 8) with a casual style to establish an informal atmosphere, in line with the recommendations of Sylaiou et al. (2019). The clothing selection aimed for a friendly and approachable environment, with bright colors enhancing the VA’s visibility, while grooming and overall appearance enhanced the VA’s credibility as a knowledgeable partner during scene exploration. As natural voices are typically preferred over synthetic ones (Ehret et al., 2021), a co-author recorded the required verbal content. Oculus Lipsync, 2023 was used for lip-syncing. Full-body animations for the VA came from Adobe Mixamo, 2023, with a maximal walking speed of 2.0 m/s.
FIGURE 7. User walking from first person’s perspective in a flow of guiding agents. Created with Unreal Engine.
FIGURE 8. Our virtual supporter and a user (displayed as an unreal mannequin) engage in a conversation about a park fountain as a few base agents walk by. Created with Unreal Engine.
Regarding the exploration support, we defined a path through the city, visiting all POIs and AOIs in the alphabetical order of Figure 6. The virtual supporter ensured that this path was taken in the correct order. The guiding agents in Cflow, however, formed pedestrian flows toward the top three unvisited POIs/AOIs. As the list of unvisited locations narrowed down, the number of flows decreased, but more base agents were added to maintain an active city. Thus, the flow support automatically ended once all POIs/AOIs had been visited. We manually set initial priorities based on the virtual supporter’s path (Figure 9A). Once an individual POI is visited, its priority becomes 0, and it is removed as a flow destination. For AOIs, we distribute the initial priority equally among the contained POIs (Figure 9B). By this, we can gradually reduce the priority after a contained POI has been visited, ensuring the AOI remains a potential destination.
FIGURE 9. (A) Top–down view onto the virtual city with initial priorities marked in yellow. (B) Details of view on one AOI with the initial priority marked in yellow and the fair division shown in red. Created with Unreal Engine.
In Csupporter, participants are in a direct interaction with the VA. To ensure a controlled yet natural interaction, we used a hybrid interaction method allowing the experimenter to trigger appropriate VA responses to participants’ spoken words using a Wizard of Oz approach. For maintaining meaningful interactions, we, thereby, limited participants to a specific set of questions and statements, which were displayed as text overlays on the right virtual controller at appropriate times.
Upon arrival, participants were assigned to their respective conditions while ensuring an equal gender distribution. They were informed about the study, gave written consent, and completed a pre-study questionnaire covering demographics, the Santa Barbara Sense of Direction Scale (SBSOD) (Hegarty et al., 2002) for spatial and navigational abilities and the Perspective Taking/Spatial Orientation Test (PTSOT) (Kozhevnikov and Hegarty, 2001; Hegarty, 2004) in the electronic version by Weißker (2015), testing the understanding of spatial relationships. After familiarizing themselves with the headset and the controls in a different city environment populated by some base agents, the main session began.
For Cfree and Cflow, participants were immersed in North Bucbrook directly. In Csupported, participants were first informed orally that a virtual guide was waiting to support them in exploring the city. On participants’ being immersed, the guide introduced itself and a small input system test was conducted to adjust the volume of the guide’s voice for user comfort.
Subsequently, participants had 40 min to explore the scene. Afterward, participants filled out a post-study questionnaire, including the NASA-TLX workload questionnaire (Hart and Staveland, 1988), the engagement scale of the Temple Presence Inventory (TPI) (Lombard et al., 2009), and specific scene-related questions. Data on the participants’ and VAs’ head orientation and position were logged continuously.
The study session lasted approximately 80 min, with immersion carried out for approximately 50 min, with sweets and drinks as rewards.
The study involved 20 participants per condition with equal gender distribution (Cfree: 13 male and seven female subjects; Csupporter: 13 male, six female, and one non-binary subjects; Cflow: 14 male and six female subjects), recruited from university mailing lists and social media. Their ages varied slightly (Cfree: M = 26.0, SD = 3.97; Csupporter: M = 24.6, SD = 3.52; Cflow: M = 25.4, SD = 2.66). All participants had normal motor skills and (corrected-to) normal vision, were unaware of the other conditions, and were naive to the study’s purpose. Evaluating the data on spatial cognition and orientation abilities, gathered in the beginning of the experiment, indicated participant uniformity among the three conditions, as we found no significant differences between the normally distributed SBSOD scores (one-way ANOVA: F (2, 57) = 0.18, p = .84; Cfree: M = 4.25, SD = 1.25; Csupporter: M = 4.42, SD = 1.0; and Cflow: M = 4.47, SD = 1.39) or the angular error of the PTSOT, which was not normally distributed (Kruskal–Wallis: χ2 = 2.65, df = 2, p = .27; Cfree: M = 19.30, SD = 9.23; Csupporter: M = 14.73, SD = 6.45; and Cflow: M = 23.44, SD = 21.17).
Statistical analysis was performed using R 4.3.0. We used a significance level of .05 for all tests and assessed data normality with the Shapiro–Wilk test. One-way analysis of variance (ANOVA) was performed if the data showed a normal distribution. When necessary, post hoc pairwise comparisons were made using t-tests with Bonferroni correction to account for significance. In contrast, Kruskal–Wallis tests were used to determine statistical significance if the data considerably departed from a normal distribution with post hoc Dunn–Bonferroni tests if necessary. Only significant results are reported.
We tested object knowledge by providing a list of 17 objects and places and asked participants to identify those embedded in the scene. For the analysis, correct answers included identifying items in the scene and item absence accurately. A Kruskal–Wallis test found a significant effect among the three conditions (χ2 = 15.80, df = 2, p < .001). Post hoc tests showed that object knowledge was significantly lower (p < .001) in Cfree (M = 14.10, SD = 2.61) than in Csupporter (M = 16.85, SD = 1.18) and significantly lower (p = .02) in Cflow (M = 15.25, SD = 1.890) than in Csupporter. There was no significant difference between Cfree and Cflow.
For factual knowledge, nine questions were asked. A Kruskal–Wallis test across the conditions revealed a significant effect (χ2 = 20.07, df = 27, p < .001). Post hoc tests showed that participants in Cfree (M = 2.20, SD = 1.61, p < .001) and Cflow (M = 3.20, SD = 1.77, p = .013) performed significantly worse compared to those in Csupporter (M = 4.90, SD = 1.41). There was no significant difference between Cfree and Cflow.
As factual knowledge in Csupporter was presented automatically, participants in Cfree and Cflow had to read the descriptive signs located at the POIs. When asking about whether they read the information signs carefully on a 5-point Likert scale from 1 (strongly disagree) to 5 (strongly agree), a Kruskal–Wallis test revealed no significant difference (χ2 = 1.89, df = 1, p = .17) between the answers in Cfree (M = 2.85, SD = 1.35) and Cflow (M = 3.4, SD = 1.1).
In terms of landmark knowledge, surveyed by indicating seven POI positions on the top–down city map, a Kruskal–Wallis test revealed no significant difference (χ2 = 2.34, df = 2, p = .31) between the three conditions in terms of correct answers (Cfree: M = 3.60, SD = 2.26; Csupporter: M = 4.55, SD = 2.14; Cflow: M = 4.00, SD = 1.86).
We tested participants’ route knowledge in Csupporter by presenting four distinct routes per three route segments and asking them to identify the route they had been guided on. The accuracy of their responses was 12 out of 20 in the first, 13 out of 20 in the second, and 12 out of 20 in the third instance. A chi-squared test revealed a significant difference between the observed and the expected frequencies for all three route choices (12 correct choices: χ2 = 13.07, df = 1, p < .001; 13 correct choices: χ2 = 17.07, df = 1, p < .001). Consequently, participants’ choices were not random, with a strong tendency to select the actual taken route.
Delving into POI coverage by evaluating which POIs were visited leads to the following findings: in Cfree, the average coverage was 80.31% (SD = 21.77 percentage points), with only two participants finding all 16 POIs. The coverage of obviously placed POIs was 87.86% (SD = 22.96 percentage points) and of hidden POIs 27.50% (SD = 34.32 percentage points). In Cflow, the average coverage was 91.86% (SD = 13.77 percentage points), with eight participants finding all 16 POIs. The coverage of obviously placed POIs was 95.36% (SD = 12.96 percentage points) and of hidden POIs 67.50% (SD = 43.75 percentage points). In Csupporter, the average coverage was 100% (SD = 0 percentage points). Comparing the visiting frequency of the obvious POIs pairwise between the three conditions via Welch’s two-sample t-tests only revealed a significant difference between Cfree and Csupporter (t (19) = 2.36, p = .03). Welch’s two-sample t-tests comparing the visiting frequency of the hidden POIs pairwise resulted in three statistically significant difference pairs: Cfree and Cflow (t (35.96) = −3.22, p = .003), Cfree and Csupporter (t (19) = 9.45, p < .001), and Cflow and Csupporter (t (19) = 3.32, p = .004).
To evaluate the mental workload, we used the NASA-TLX with a uniform weighting (2.5) for all six factors. A one-way ANOVA revealed a significant effect for workload among the three conditions (F (2, 57) = 4.362, p = .017). Post hoc tests showed that a significantly lower workload (p = .007) in Csupporter (M = 40.2, SD = 9.60) compared to Cflow (M = 52.3, SD = 13.4). There was no significant difference (both p’s
Participants were asked to rate their experience with the virtual supporter directly after experiencing the virtual tour, as summarized in Table 2. Non-parametric Wilcoxon signed rank tests with continuity correction per item to compare the distribution of responses to the neutral value of 3 were conducted to determine if there is a statistically significant deviation from that value. The results are reported in the table.
TABLE 2. Participants’ ratings with respect to the virtual supporter’s behavior (M denotes the mean, SD denotes the standard deviation, and Mdn denotes the median).
Furthermore, 14 out of the 20 participants found the number of monologues during the dynamic transitions to be just right, while 6 participants wished for more. In the free-text field, those six stated concerns about boredom (1x) and discomfort (2x) arising from extended silences wished for more occasional turn announcements (1x) or expressed their desire for more fun facts instead of small talk (1x) as well as more city-related information (1x).
Evaluating whether participants listened to the supporter’s supplemental prompts resulted in the following findings. Three participants (15%) opted to skip just one prompt, two skipped two prompts (10%), one (5%) skipped three prompts, two missed four prompts (10%), and two participants (10%) chose to skip five prompts each. From these 28 missed prompts, only 2 belonged to the individual POIs (lantern and old bar), while the others were from AOI-contained POIs. For the AOI visited first (park), 20 supplemental prompts were missed in total, while only 6 prompts were skipped for the AOI visited second (forecourt). Still, when relating the total number of available supplemental prompts and the number of given prompts for individual and AOI-contained POIs individually, a binomial test per POI category revealed a significant deviation from the expected equal distribution of outcomes (p < .001). Six individual POIs and 20 participants resulted in the potential for 120 supplementary prompts to be provided, from which 118 were given. The 10 AOI-contained POIs thus resulted in the potential of 200 supplementary prompts, from which 174 were given.
Although being naive to the study’s purpose, 17 participants noticed particular flows of pedestrians moving into the same direction often, while the remaining 3 participants only noticed them occasionally. A preference was noted for following these flows, with 14 participants wanting to do so, while five preferred an alternative path, and one participant showed indifference to the pedestrian flows’ directions. This preference was confirmed by a chi-squared test (χ2 = 13.3, df = 2, p = .001), indicating a departure from the expected uniform distribution.
After being told that the pedestrian flows were supposed to subtly guide participants, five participants stated that the flow’s objective was obvious, while 15 participants stated that they suspected so after a while. Additionally, 12 participants realized they followed the pedestrians, while four avoided them, and four were not affected at all. A chi-squared test (χ2 = 6.4, df = 2, p = .04) indicated a departure from the expected uniform distribution.
Moreover, participants were asked to rate their experience with the pedestrian flows based on various questions, as summarized in Table 3. We, again, used non-parametric Wilcoxon signed rank tests with continuity correction per item and reported the results in the table.
TABLE 3. Participants’ ratings with respect to the behavior of the virtual pedestrians in Cflow (M denotes the mean, SD denotes the standard deviation, and Mdn denotes the median).
We asked our participants to rate their general experience while exploring the virtual scene in terms of fun and exploration time (Table 4). Each question was evaluated with a Kruskal–Wallis test; however, only the exploration time question revealed a significant difference (p < .001). Post hoc tests revealed a significant difference (p < .001) between Cfree and Csupporter as well as between Csupporter and Cflow, reporting that time was perceived as less sufficient for the free and the flow-supported exploration.
TABLE 4. Participants’ ratings with respect to their general experience (M denotes the mean, SD denotes the standard deviation, and Mdn denotes the median).
We also evaluated the dimension engagement of the TPI (Figure 10). A one-way ANOVA (F (2, 57) = 1.09) revealed no significant differences (p = .34) between the three conditions.
FIGURE 10. Evaluation of the engagement dimensions of the TPI on a 1–7 Likert scale, with 1 being the least desired and 7 the most favorable answer with p < .001 (***), p < .01 (**), p < .05 (*), and p < .1 (+) and error bars indicating the standard error.
As proposed by Slater et al. (2022), we performed a sentiment analysis with the R-package sentimentr (v2.9.0) on participants’ open-ended answers, asking about preferences, concerns, and their experience. We first expanded ambiguous comments to include sentiment (from “movement speed” to “I like/dislike the movement speed”) and aggregated the responses per participant to compute sentiment/polarity scores (∈ [−1, 1]). A Kruskal–Wallis test revealed a significant difference (p < .001) in sentiment scores among the conditions. Post hoc tests revealed significant differences between Csupporter (M = 0.17, SD = 0.15) and Cfree (M = −0.06, SD = 0.20, p < .001) as well as Cflow (M = 0.04, SD = 0.20, p = .04).
The two social support techniques functioned well, as shown later in connection with H3, allowing us to discuss the insights gained regarding our comparative hypotheses first.
To assess the acquired scene knowledge (H1), we concentrated on four knowledge categories: object, factual, landmark, and, for Csupporter, route knowledge. Our findings indicate a significant difference in object and factual knowledge among our conditions: Participants in Csupporter showed superior knowledge, while no significant difference was found in knowledge acquisition between the two remaining conditions. Thus, H1 can only be partially accepted for object and factual knowledge acquisition.
The differences observed in factual knowledge potentially stem from the presentation technique utilized: the superior Csupporter used both oral and written information, while Cfree and Cflow relied solely on written information signs. Our findings thus suggest that oral delivery enhanced learning in our setting compared to using only written signs, indicating a potential limitation in our design. To address this, we propose incorporating an audio tour guide in Cfree and Cflow in future studies. While refraining from providing directional cues, the audio guide would provide the virtual supporter’s explanatory prompts at the respective POIs, ensuring consistent factual knowledge presentation.
With respect to landmark knowledge, H1 is not supported, as participants performed similarly in locating POIs on a top–down city map, with the mean scores ranging between 3.6 and 4.55 out of 7. Interestingly, participants in Csupporter showed notable route awareness, which surprisingly was not reflected in their proficiency in locating specific POIs. The performance difference reveals the complexity of knowledge acquisition and underscores the necessity for further studies to understand the relationship between route and landmark knowledge.
A prominent influencing factor on scene knowledge is POI coverage. Although participants in Csupporter, obviously, visited all POIs, fewer were visited in Cflow, and even fewer in Cfree. This clearly demonstrates the importance of social wayfinding support. For the obvious POIs, Csupporter had a significantly higher mean coverage than Cfree. This suggests that weak social wayfinding has a moderate influence in this context. In the case of hidden POIs, however, significant differences were observed among all three conditions, leading to a decreasing coverage order: Csupporter, Cflow, and Cfree. This again highlights the pivotal role of Csupporter during scene exploration. Moreover, this suggests that pedestrian flows are effective at enhancing exploration in complex or less obvious scenarios. They assist users in uncovering details that might otherwise go unnoticed during free exploration, thereby enhancing their spatial awareness. For more profound insights, future studies should explore the precise mechanisms by which pedestrian flows facilitate exploration and how this effect can be further enhanced.
For participants’ task load (H2), our findings indicate that the workload in Csupporter was significantly lower than that in Cflow, partially supporting H2 (Csupporter < Cflow). However, Cfree did not exhibit a higher workload, as initially expected. Instead, it falls between Csupporter and Cflow without statistically significant differences, leading to a partial rejection of H2 (Cflow < Cfree). This observation may be due to the high pedestrian density in Cflow. Participants reported concerns about crowded narrow streets and navigation difficulties due to pedestrian congestion within the open response sections, which may have increased their mental workload. Thus, the perceived workload seems to be more influenced by navigation complexity and frustration from collisions and blocked pathways, rather than the complexity of wayfinding decisions.
Although we expected to find a higher engagement rate in Csupporter due to the direct user–agent interaction, we did not find significant differences on the TPI’s engagement scale between the conditions. Furthermore, no significant difference was found when asking participants whether their experience was fun. This indicates a partial rejection of H3 in terms of enjoyment. To address the user comfort of H3, we need to discuss the individual responses per condition.
Participants in Cfree randomly traversed the city without a clear pattern (Figure 11A) finding only 80.31% of the POIs in the given time. While one participant stated in the free-text fields that the Sun, shadows, and mountains on the skyline helped with orientation, other participants negatively commented that they “got very lost at the end and didn’t know where to look for the missing POI” and that the “environment is sometimes confusing,” which resulted in “no sense of orientation.” One participant explicitly stated that “I had no time to orientate and locate myself in the city. I couldn’t create a mental map of the city. Therefore, I felt lost and helpless.” As a result, six participants voluntarily expressed their wish for assistance in the open-ended response fields, asking for maps (3×), street names (1×), landmarks (1×), or direction signs (3×). Thus, considering the comparably low performance value for POI coverage and the anecdotal indications, we find support that incorporating a social wayfinding technique may improve the exploration experience.
FIGURE 11. Visualization of the trajectories of each participant, colored individually, in a top–down view of the virtual city for (A) the free exploration, (B) the flow-based exploration, and (C) the supporter-based exploration.
In Cflow, the granted subtle support relies on the participants’ recognition of the pedestrian flows and their conforming behavior toward the directional cue, resulting in an exhibition of a higher level of coherence and efficiency during the scene exploration (Figure 11B). All participants recognized the flows and understood the subtle guiding mechanism either directly or after a while, while the size of the flows was perceived as plausible and, in the case of large open spaces, also appropriate. Seventy percent of participants wanted to follow the pedestrian flows when recognizing them, and 60% acknowledged that they had been influenced by pedestrians’ objectives in retrospect, highlighting the substantial impact of the flows on the participants’ behavior. Participants found high value in being indirectly guided by pedestrian flows to interesting locations. In the free-text fields, they stated the guidance was “helpful in finding POIs” “due to the subtle cues where to go.” They also noted positively that “pedestrians always guiding [them] wherever [they are]” and that they, thus, had a permanent “indication of POIs” by means of the flows. Still, they “were able to spend as much time as [they] want in each place,” allowing participants to maintain their autonomy while still providing a user-friendly and continuous guidance. Aside from the guidance, participants appreciated the presence of pedestrians due to their contribution to a lively atmosphere as stated in the open-comment sections. Participants felt a sense of comfort and realism, preventing the simulation from feeling like a “desolate ghost town.” Thus, embedding this social wayfinding support enhanced the overall experience and the performance in terms of finding POIs. Nevertheless, the flow management in Cflow needs improvement for future studies, evident from reservations in the free-text fields. Comments such as the presence of “too many strangers,” “large crowds forming quickly,” resulting in “lining up,” and “too many pedestrians in way sometimes blocking movements” suggest that the density of the flows was suboptimal in certain regions and situations, leading some participants to prefer other routes to avoid the pedestrians. Some participants even wished for more social interactions between the pedestrians such as taking photos at POIs or talking to each other while traversing the scene, enhancing the realism of the scene.
In Csupporter, the granted support is given by a direct interaction with the virtual supporter, resulting in completely structured trajectories (Figure 11C). Participants preferred the virtual support provided to explore the city over self-guided exploration, indicating that the virtual supporter’s assistance was noticeable and contributed to their positive experience. The balanced nature of the design was also highlighted, as participants’ preference for self-guided exploration was not overshadowed by the support offered. The findings of the post-study questionnaire are supported by responses in the free text fields: “Having the guide by my side helped me to explore the whole city,” as stated by one participant, clearly supports that participants value being guided while also having navigation autonomy. In general, participants had a sense of control throughout the experience, indicated by statements such as “Even though being guided, I haven’t felt being out of control over which points are being visited. “The guidance, they noted, alleviated the need for them to make own navigation decisions, enabling them to fully engage with the surroundings as indicated by statements such as “I could just observe the environment without the need of deciding where to go next,” “I liked the concept of following a guide, since the city was big and it would have been difficult to find all attractions” and that “not having to worry about the navigation in the city” was enjoyed. Furthermore, participants positively noted the structured manner of the guidance as indicated by “the comments of the guide structured the experience.” Participants also expressed the significance of the flexibility to independently explore specific sections of the city, indicated by statements for what they liked about the offered experience such as “possibility of exploring areas on my own with the guide for additional information”, “multiple sub sights for exploring on your own,” or “the ability to free-roam in certain areas, like the south-western park.” Importantly, the interactive attributes of the virtual supporter were also highlighted positively, with participants appreciating instances where the guide waited for a participant fallen behind (“the guide waits when he isn’t followed”) or uttered conversational prompts at the waypoints (“the guide […] makes small talk”). The quantity of these interim prompts was in general rated well by 14 participants. The remaining six even wished for more utterances, i. e., to get to know more city-related information and fun facts, or some more turn announcements. Participants, moreover, expressed satisfaction with the virtual supporter’s interest interference, which was used to start explanatory prompts for AOI-contained POIs and supplemental prompts for all POIs. A significant number of supplemental prompts were given, but they were more likely to be skipped for AOI-contained POIs, especially for the first AOI. This disparity may be attributed to the nature of AOI-based exploration. Guided participants at individual POIs likely engaged more with the POI due to the explicit introduction via the virtual supporter. During free exploration, however, participants may have bypassed supplementary prompts due to either a lower perceived relevance or a preference to discover more POIs instead of engaging with a specific POI in detail. The latter may also explain the difference in skipping behavior between the first and second AOIs, as the former required more active searching, while the POIs in the latter were immediately visible. More research is needed to understand participants’ skipping behavior and the potential impact of the supporter’s behavior.
Although each condition itself is rated well, a sentiment analysis revealed that participants in Csupporter reported significantly higher sentiment scores, indicating a greater sense of comfort and satisfaction with the direct support provided by the VA. In contrast, Cflow was perceived as less comfortable and engaging, while Cfree was the least preferred option, even rated negatively. These results support H3 in terms of user comfort.
However, it is essential to acknowledge the limitations of the evaluated implementations. Despite generally positive ratings, an implementation error in Csupporter led to the VA waiting for participants during guiding when they were ahead. This unintended delay in exploration frustrated participants, indicating the need for a continuous, smooth support. Furthermore, a more natural trajectory design would enhance user comfort. For Cflow, the aforementioned issue of congested pedestrian flows in narrow spaces posed challenges for user navigation, especially as the pedestrians became less socially compliant, reducing user comfort due to collisions. Still, Cflow was enjoyed and seems to be a promising support option to be considered next to Csupporter. However, the choice depends on the specific use case. Csupporter requires direct interaction with a VA, resulting in a dependence on the VA and limited autonomy for the user, while providing a suitable structure in a well-definable time. On the other hand, Cflow allows for greater user autonomy with support being always readily available; however, the exploration duration is more challenging to estimate.
The time aspect nicely aligns with two interesting findings: first, Csupporter received a positive indication of exploration time sufficiency (exploration time: 40 min), while Cflow and Cfree received significantly lower ratings. Second, some participants desired a faster movement speed in Cfree (6×) and Cflow (5×), while none did in Csupporter. This difference in perception of their own movement speed could be attributed to the experience of walking collaboratively with a VA versus walking alone. In Csupporter, participants may not have desired faster movements since they relied on their supporter. In Cfree and Cflow, however, participants may have felt the need for increased speed due to a sense of independence and full control over their movement. We initially chose continuous locomotion, matching human walking speed, for realism and to foster collaborative navigation. However, future research might also explore alternative navigation techniques, such as redirected walking (Nguyen et al., 2020; Sayyad et al., 2020) and teleportation (Weißker et al., 2019).
Thus, our results indicate the benefits of social support techniques during scene exploration and highlight that strong social wayfinding (Csupporter) is often superior to weak social wayfinding (Cflow). Still, it is evident that pedestrian flows are a promising technique and that overcoming current limitations may optimize the effectiveness of this approach.
Our research highlights the benefits of using strong and weak social wayfinding strategies for users exploring unknown IVEs. Strong social wayfinding was realized by a virtual supporter (Csupporter), who guides users through the IVE, while switching into a knowledgeable companion in information-dense AOIs. Alternatively, pedestrian flows toward interesting locations (Cflow) were used as weak social wayfinding support. The results showed that Csupporter enhances knowledge acquisition and uses fewer mental resources. However, for users prioritizing autonomy and ongoing support, weak social wayfinding may be better suited. Cflow provides visual cues that improve spatial awareness and knowledge acquisition in comparison to no support, allowing users to access essential areas, even in less obvious locations. However, further research is needed to understand the precise mechanisms by which pedestrian flows influence scene exploration and to optimize them for a more effective social wayfinding experience.
The raw data supporting the conclusion of this article will be made available by the authors, without undue reservation.
We meticulously followed the principles outlined in the Declaration of Helsinki to ensure that the experimental protocol was conducted with the highest ethical standards and the wellbeing of participants was upheld throughout the study. The studies were conducted in accordance with the local legislation and institutional requirements. The participants provided their written informed consent to participate in this study.
AB: conceptualization, data curation, formal analysis, investigation, methodology, software, supervision, validation, visualization, writing–original draft, and writing–review and editing. JE: methodology, writing–review and editing, formal analysis, and software. DR: software, writing–review and editing, data curation, and methodology. TK: conceptualization, supervision, writing–review and editing, and resources.
The author(s) declare that no financial support was received for the research, authorship, and/or publication of this article.
The authors thank Azur Ponjavic for his support in modeling the virtual city and Xinyu Xia for her assistance in developing the unreal application for Cflow and data collecting during the user study.
The authors declare that the research was conducted in the absence of any commercial or financial relationships that could be construed as a potential conflict of interest.
All claims expressed in this article are solely those of the authors and do not necessarily represent those of their affiliated organizations, or those of the publisher, the editors, and the reviewers. Any product that may be evaluated in this article, or claim that may be made by its manufacturer, is not guaranteed or endorsed by the publisher.
Adili, R., Niay, B., Zibrek, K., Olivier, A.-H., Pettré, J., and Hoyet, L. (2021). Perception of motion variations in large-scale virtual human crowds. Motion, Interact. Games (ACM). doi:10.1145/3487983.3488288
Adobe Mixamo (2023). Avialable at: https://www.mixamo.com/ (Accessed February 20, 2023).
Aglobex Character Pack (2023). Avialable at: https://www.unrealengine.com/marketplace/en-US/product/population-system (Accessed September 06, 2023).
Attree, E., Brooks, B., Rose, F., Andrews, T., Leadbetter, A., and Clifford, B. (1996). “Memory processes and virtual environments: I can’t remember what was there, but I can remember how I got there. Implications for people with disabilities,” in Europ. Conf. Disability, Virtual Real (& Associated Techn).
Baddeley, M. (2010). Herding, social influence and economic decision-making: socio-psychological and neuroscientific analyses. Philosophical Trans. R. Soc. B Biol. Sci. 365, 281–290. doi:10.1098/rstb.2009.0169
Bates, J. (1994). The role of emotion in believable agents. Commun. ACM 37, 122–125. doi:10.1145/176789.176803
Berton, F., Hoyet, L., Olivier, A.-H., Bruneau, J., Meur, O. L., and Pettre, J. (2020). “Eye-gaze activity in crowds: impact of virtual reality and density,” in 2020 IEEE Conference on Virtual Reality and 3D User Interfaces (VR) (IEEE). doi:10.1109/vr46266.2020.00052
Best, K. (2012). Making museum tours better: understanding what a guided tour really is and what a tour guide really does. Mus. Manag. Curatorsh. 27, 35–52. doi:10.1080/09647775.2012.644695
Bogdanovych, A., and Trescak, T. (2017). “To plan or not to plan: lessons learned from building large scale social simulations,” in Intelligent virtual agents (Springer International Publishing), 53–62. doi:10.1007/978-3-319-67401-8_6
Bönsch, A., Güths, K., Ehret, J., and Kuhlen, T. W. (2021a). “Indirect user guidance by pedestrians in virtual environments,” in ICAT-EGVE 2021 - International Conference on Artificial Reality and Telexistence and Eurographics Symposium on Virtual Environments - Posters and Demos. doi:10.2312/egve.20211336
Bönsch, A., Hashem, D., Ehret, J., and Kuhlen, T. W. (2021b). “Being guided or having exploratory freedom: user preferences of a virtual agent’s behavior in a museum,” in Proceedings of the 21th ACM International Conference on Intelligent Virtual Agents (IVA’21), 33–40. IVA 2021 GALA Audience Award for the video shown under the URL link. doi:10.1145/3472306.3478339
Cao, T., Cao, C., Guo, Y., Wu, G., and Shen, X. (2021). “Interactive embodied agent for navigation in virtual environments,” in 2021 IEEE International Symposium on Mixed and Augmented Reality Adjunct (ISMAR-Adjunct) (IEEE). doi:10.1109/ismar-adjunct54149.2021.00053
Carpman, J. R., and Grant, M. A. (2003). “Wayfinding: a broad overview,” in Handbook of environmental psychology. Editors R. B. Bechtel, and A. Churchman (John Wiley & Sons, Inc.). chap. 28. 427–442.
Carrozzino, M., Colombo, M., Tecchia, F., Evangelista, C., and Bergamasco, M. (2018). “Comparing different storytelling approaches for virtual guides in digital immersive museums,” in 5th International Conference on Augmented Reality, Virtual Reality, and Computer Graphics (AVR’18), 292–302. doi:10.1007/978-3-319-95282-6_22
Chang, D., Cui, L., and Huang, Z. (2020). A cellular-automaton agent-hybrid model for emergency evacuation of people in public places. IEEE Access 8, 79541–79551. doi:10.1109/access.2020.2986012
Chittaro, L., and Ieronutti, L. (2004). “A visual tool for tracing users’ behavior in virtual environments,” in Proceedings of the working conference on Advanced visual interfaces (ACM). doi:10.1145/989863.989868
Chrastil, E. R., and Warren, W. H. (2013). Active and passive spatial learning in human navigation: acquisition of survey knowledge. J. Exp. Psychol. Learn. Mem. Cognition 39, 1520–1537. doi:10.1037/a0032382
Cohen, E. (1985). The tourist guide: the origins, structure and dynamics of a role. Ann. Tour. Res. 12, 5–29. doi:10.1016/0160-7383(85)90037-4
Dalton, R. C., Hölscher, C., and Montello, D. R. (2019). Wayfinding as a social activity. Front. Psychol. 10, 142–214. doi:10.3389/fpsyg.2019.00142
de Jong, M., Theune, M., and Hofs, D. (2008). “Politeness and alignment in dialogues with a virtual guide,” in Proceedings of the 7th International Joint Conference on Autonomous Agents and Multiagent Systems, AAMAS ’08 (Taipei, Taiwan: International Foundation for Autonomous Agents and Multiagent Systems), 207–214. Available at: https://www.ifaamas.org/Proceedings/aamas08/proceedings/pdf/paper/AAMAS08_0548.pdf.
Doyle, P., and Hayes-Roth, B. (1997a). “An intelligent guide for virtual environments,” in Proceedings of the first international conference on Autonomous agents - AGENTS ’97 (New York, NY: ACM Press). doi:10.1145/267658.267797
Doyle, P., and Hayes-Roth, B. (1997b). “Guided exploration of virtual worlds,” in Network and netplay: virtual groups on the internet, 243–264.
Ehret, J., Bönsch, A., Aspöck, L., Röhr, C. T., Baumann, S., Grice, M., et al. (2021). Do prosody and embodiment influence the perceived naturalness of conversational agents’ speech? ACM Trans. Appl. Percept. 18, 1–15. doi:10.1145/3486580
Foulsham, T., Walker, E., and Kingstone, A. (2011). The where, what and when of gaze allocation in the lab and the natural environment. Vis. Res. 51, 1920–1931. doi:10.1016/j.visres.2011.07.002
Freitag, S., Weyers, B., Bönsch, A., and Kuhlen, T. W. (2015). “Comparison and evaluation of viewpoint quality estimation algorithms for immersive virtual environments,” in International Conference on Artificial Reality and Telexistence and Eurographics Symposium on Virtual Environments (ICAT-EGVE’15), 53–60. doi:10.2312/egve.20151310
Freitag, S., Weyers, B., and Kuhlen, T. W. (2018). “Interactive exploration assistance for immersive virtual environments based on object visibility and viewpoint quality,” in IEEE Conference on Virtual Reality and 3D User Interfaces (VR’18), 355–362doi. doi:10.1109/VR.2018.8447553
Fu, Y.-W., Li, M., Liang, J.-H., and Hu, X.-Q. (2014). “Modeling and simulating the walking behavior of small pedestrian groups,” in Communications in computer and information science (Springer Berlin Heidelberg), 1–14. doi:10.1007/978-3-662-45289-9_1
Hart, S. G., and Staveland, L. E. (1988). “Development of NASA-TLX (task load index): results of empirical and theoretical research,” in Advances in psychology (Elsevier), 139–183. doi:10.1016/s0166-4115(08)62386-9
Hegarty, M. (2004). A dissociation between mental rotation and perspective-taking spatial abilities. Intelligence 32, 175–191. doi:10.1016/j.intell.2003.12.001
Hegarty, M., Richardson, A. E., Montello, D. R., Lovelace, K., and Subbiah, I. (2002). Development of a self-report measure of environmental spatial ability. Intelligence 30, 425–447. doi:10.1016/s0160-2896(02)00116-2
Helbing, D., Farkas, I., and Vicsek, T. (2000). Simulating dynamical features of escape panic. Nature 407, 487–490. doi:10.1038/35035023
Hoffmann, L., Krämer, N. C., Lam-chi, A., and Kopp, S. (2009). “Media equation revisited: do users show polite reactions towards an embodied agent?,” in ACM International Conference on Intelligent Virtual Agents (Springer Berlin Heidelberg), 159–165. doi:10.1007/978-3-642-04380-2_19
Ibanez, J., Aylett, R., and Ruiz-Rodarte, R. (2003a). Storytelling in virtual environments from a virtual guide perspective. Virtual Real. 7, 30–42. doi:10.1007/s10055-003-0112-y
Ibanez, J., Aylett, R., and Ruiz-Rodarte, R. (2003b). “That’s my point! Telling stories from a virtual guide perspective,” in Intelligent virtual agents (Springer Berlin Heidelberg), 249–253. doi:10.1007/978-3-540-39396-2_42
Ibáñez-Martínez, J., Delgado-Mata, C., Serrano, O., and Narbona, D. G. (2008). Animal flocks as natural and dynamic spatial clues in adventure video-games. Int. J. Virtual Real. 7, 73–80.
Isbister, K., and Doyle, P. (1999). “Touring machines: guide agents for sharing stories about digital places,” in Proc. Workshop on narrative and artificial intell.
Jan, D., Roque, A., Leuski, A., Morie, J., and Traum, D. (2009). “A virtual tour guide for virtual worlds,” in Proceedings of the 9th International Conference on Intelligent Virtual Agents (IVA’09), 372–378. doi:10.1007/978-3-642-04380-2_40
Jerald, J. (2015). The VR book: human-centered design for virtual reality. New York, NY: Association for Computing Machinery and Morgan & Claypool. doi:10.1145/2792790
Kleinermann, F., Troyer, O. D., Creelle, C., and Pellens, B. (2008). “Adding semantic annotations, navigation paths and tour guides to existing virtual environments,” in Virtual systems and multimedia (Springer Berlin Heidelberg), 100–111. doi:10.1007/978-3-540-78566-8_9
Kozhevnikov, M., and Hegarty, M. (2001). A dissociation between object manipulation spatial ability and spatial orientation ability. Mem. Cognition 29, 745–756. doi:10.3758/bf03200477
Krontiris, A., Bekris, K. E., and Kapadia, M. (2016). “ACUMEN: activity-centric crowd authoring using influence maps,” in Proceedings of the 29th International Conference on Computer Animation and Social Agents (ACM). doi:10.1145/2915926.2915935
LaViola, J. J., Kruijff, E., McMahan, R. P., Bowman, D., and Poupyrev, I. P. (2017). 3D user interfaces: theory and practice. Addison-Wesley Professional.
Leibenstein, H. (1950). Bandwagon, snob, and veblen effects in the theory of consumers’ demand. Q. J. Econ. 64, 183. doi:10.2307/1882692
Levasseur, M., and Veron, E. (1983). Ethnographie d’une Exposition. Histoires d’expo, Peuple Cult., 29–32.
Lim, M. Y., and Aylett, R. (2007). “Feel the difference: a guide with attitude,” in Intelligent virtual agents (Springer Berlin Heidelberg), 317–330. doi:10.1007/978-3-540-74997-4_29
Lim, M. Y., and Aylett, R. (2009). An emergent emotion model for an affective mobile guide with attitude. Appl. Artif. Intell. 23, 835–854. doi:10.1080/08839510903246518
Liszio, S., and Masuch, M. (2016). “Lost in open worlds,” in Proceedings of the 13th International Conference on Advances in Computer Entertainment Technology (ACM). doi:10.1145/3001773.3001794
Lombard, M., Ditton, T. B., and Weinstein, L. (2009). “Measuring presence: the temple presence inventory,” in Proceedings of the 12th Annual International Workshop on Presence, 1–15.
Marigold, D., and Patla, A. (2007). Gaze fixation patterns for negotiating complex ground terrain. Neuroscience 144, 302–313. doi:10.1016/j.neuroscience.2006.09.006
Martinez, S., Sloan, R., Szymkowiak, A., and Scott-Brown, K. (2010). “Using virtual agents to cue observer attention: assessment of the impact of agent animation,” in 2nd International Conference on Creative Content Technologies, CONTENT 2010 (Wilmington, DE: International Academy, Research, and Industry Association (IARIA)), 7–12. Available at: https://rke.abertay.ac.uk/en/publications/using-virtual-agents-to-cue-observer-attention-assessment-of-the-.
McDonnell, R., Jörg, S., McHugh, J., Newell, F., and O’Sullivan, C. (2008). “Evaluating the emotional content of human motions on real and virtual characters,” in Proceedings of the 5th Symposium on Applied Perception in Graphics and Visualization (ACM). doi:10.1145/1394281.1394294
McDonnell, R., Larkin, M., Hernández, B., Rudomin, I., and O’Sullivan, C. (2009). Eye-catching crowds: saliency based selective variation. ACM Trans. Graph. 28, 1–10. doi:10.1145/1531326.1531361
Meerhoff, L., Bruneau, J., Vu, A., Olivier, A.-H., and Pettré, J. (2018). Guided by gaze: prioritization strategy when navigating through a virtual crowd can be assessed through gaze activity. Acta Psychol. 190, 248–257. doi:10.1016/j.actpsy.2018.07.009
MetaHuman (2023). l Avialable at: https://www.unrealengine.com/metahuman (Last-visited August 05, 2023).
Moussaïd, M., Perozo, N., Garnier, S., Helbing, D., and Theraulaz, G. (2010). The walking Behaviour of pedestrian social groups and its impact on crowd dynamics. PLoS ONE 5, e10047. doi:10.1371/journal.pone.0010047
Munro, A., Höök, K., and Benyon, D. (1999). “Footprints in the snow,” in Social navigation of information space (Springer London), 1–14. doi:10.1007/978-1-4471-0837-5_1
Nguyen, A., Wüest, P., and Kunz, A. (2020). “Human following behavior in virtual reality,” in 26th ACM Symposium on Virtual Reality Software and Technology (ACM). doi:10.1145/3385956.3422099
Nummenmaa, L., Hyönä, J., and Hietanen, J. K. (2009). I’ll walk this way: eyes reveal the direction of locomotion and make passersby look and go the other way. Psychol. Sci. 20, 1454–1458. doi:10.1111/j.1467-9280.2009.02464.x
Oculus Lipsync (2023). Avialable at: https://developer.oculus.com/downloads/package/oculus-lipsync-unreal/ (Accessed April 11, 2023).
Pejsa, T., Andrist, S., Gleicher, M., and Mutlu, B. (2015). Gaze and attention management for embodied conversational agents. ACM Trans. Interact. Intelligent Syst. 5, 1–34. doi:10.1145/2724731
Peters, C., and Ennis, C. (2009). Modeling groups of plausible virtual pedestrians. IEEE Comput. Graph. Appl. 29, 54–63. doi:10.1109/MCG.2009.69
Pfeiffer-Lessmann, N., Pfeiffer, T., and Wachsmuth, I. (2012). “An operational model of joint attention - timing of gaze patterns in interactions between humans and a virtual human,” in Proceedings of the Annual Meeting of the Cognitive Science Society.
Pražák, M., and O’Sullivan, C. (2011). “Perceiving human motion variety,” in Proceedings of the ACM SIGGRAPH Symposium on Applied Perception in Graphics and Visualization (ACM). doi:10.1145/2077451.2077468
Reeves, B., and Nass, C. (1996). The Media equation: how people treat computers, television, and new Media like real people and places. Cambridge, UK.
Ríos, A., Mateu, D., and Pelechano, N. (2018). “Follower behavior in a virtual environment,” in IEEE Virtual Humans and Crowds in Immersive Environments.
Ríos, A., and Pelechano, N. (2020). Follower behavior under stress in immersive VR. Virtual Real. 24, 683–694. doi:10.1007/s10055-020-00428-8
Rojas, F. A., and Yang, H. S. (2013). “Immersive human-in-the-loop HMD evaluation of dynamic group behaviorin a pedestrian crowd simulation that uses group agent-based steering,” in Proceedings of the 12th ACM SIGGRAPH International Conference on Virtual-Reality Continuum and Its Applications in Industry - VRCAI ’13, 31–40. doi:10.1145/2534329.2534336
Rzayev, R., Karaman, G., Henze, N., and Schwind, V. (2019a). “Fostering virtual guide in exhibitions,” in Proceedings of the 21st International Conference on Human-Computer Interaction with Mobile Devices and Services (ACM). doi:10.1145/3338286.3344395
Rzayev, R., Karaman, G., Wolf, K., Henze, N., and Schwind, V. (2019b). “The effect of presence and appearance of guides in virtual reality exhibitions,” in Proceedings of Mensch und Computer 2019 (ACM). doi:10.1145/3340764.3340802
Sayyad, E., Sra, M., and Hollerer, T. (2020). “Walking and teleportation in wide-area virtual reality experiences,” in 2020 IEEE International Symposium on Mixed and Augmented Reality (ISMAR) (IEEE). doi:10.1109/ismar50242.2020.00088
Shi, Y., Ondrej, J., Wang, H., and O’Sullivan, C. (2017). “Shape up! Perception based body shape variation for data-driven crowds,” in 2017 IEEE Virtual Humans and Crowds for Immersive Environments (VHCIE) (IEEE). doi:10.1109/vhcie.2017.7935623
Slater, M., Banakou, D., Beacco, A., Gallego, J., Macia-Varela, F., and Oliva, R. (2022). A separate reality: an update on place illusion and plausibility in virtual reality. Front. Virtual Real. 3. doi:10.3389/frvir.2022.914392
Sokolov, D., Plemenos, D., and Tamine, K. (2006). “Viewpoint quality and global scene exploration strategies,” in Proceedings of the First International Conference on Computer Graphics Theory and Applications (Setúbal, PortugalSciTePress - Science and and Technology Publications). doi:10.5220/0001350101840191
Sylaiou, S., Kasapakis, V., Dzardanova, E., and Gavalas, D. (2019). “Assessment of virtual guides’ credibility in virtual museum environments,” in Lecture notes in computer science (Springer International Publishing), 230–238. doi:10.1007/978-3-030-25999-0_20
van der Heiden, T., Mirus, F., and van Hoof, H. (2020). Social navigation with human empowerment driven deep reinforcement learning. doi:10.48550/ARXIV.2003.08158
van Toll, W., and Pettré, J. (2021). Algorithms for microscopic crowd simulation: advancements in the 2010s. Comput. Graph. Forum 40, 731–754. doi:10.1111/cgf.142664
Watanabe, T., Tsukamoto, K., Matsushima, Y., and Nakajima, T. (2020). “A new advertisement method of displaying a crowd,” in Proceedings of the Twelfth International Conference on Advances in Multimedia.
Weißker, T. (2015). Electronic version of PTSOT. Avialable at: https://github.com/TimDomino/ptsot (Accessed April 19, 2023).
Weißker, T., Bimberg, P., and Froehlich, B. (2020). Getting there together: group navigation in distributed virtual environments. IEEE Trans. Vis. Comput. Graph. 26, 1860–1870. doi:10.1109/tvcg.2020.2973474
Weißker, T., Kulik, A., and Froehlich, B. (2019). “Multi-ray jumping: comprehensible group navigation for collocated users in immersive virtual reality,” in 2019 IEEE Conference on Virtual Reality and 3D User Interfaces (VR) (IEEE). doi:10.1109/vr.2019.8797807
Ye, Z.-M., Chen, J.-L., Wang, M., and Yang, Y.-L. (2021). “PAVAL: position-aware virtual agent locomotion for assisted virtual reality navigation,” in 2021 IEEE International Symposium on Mixed and Augmented Reality (ISMAR) (IEEE). doi:10.1109/ismar52148.2021.00039
Yoshida, N., Hanasaki, S., and Yonezawa, T. (2018). “Attracting attention and changing behavior toward wall advertisements with a walking virtual agent,” in Proceedings of the 6th International Conference on Human-Agent Interaction (HAI’18), 61–66. doi:10.1145/3284432.3284450
Yoshida, N., and Yonezawa, T. (2021). “Attention-guidance method based on conforming behavior of multiple virtual agents for pedestrians,” in Proceedings of the 21th ACM International Conference on Intelligent Virtual Agents (IVA’21), 209–215. doi:10.1145/3472306.3478346
Keywords: virtual agents, pedestrian flows, virtual reality, wayfinding, social activity, user experience evaluation, spatial knowledge
Citation: Bönsch A, Ehret J, Rupp D and Kuhlen TW (2024) Wayfinding in immersive virtual environments as social activity supported by virtual agents. Front. Virtual Real. 4:1334795. doi: 10.3389/frvir.2023.1334795
Received: 07 November 2023; Accepted: 26 December 2023;
Published: 29 February 2024.
Edited by:
Pierre Raimbaud, École Nationale d’Ingénieurs de Saint-Etienne, FranceReviewed by:
Carlos Andújar, Universitat Politecnica de Catalunya, SpainCopyright © 2024 Bönsch, Ehret, Rupp and Kuhlen. This is an open-access article distributed under the terms of the Creative Commons Attribution License (CC BY). The use, distribution or reproduction in other forums is permitted, provided the original author(s) and the copyright owner(s) are credited and that the original publication in this journal is cited, in accordance with accepted academic practice. No use, distribution or reproduction is permitted which does not comply with these terms.
*Correspondence: Andrea Bönsch, Ym9lbnNjaEB2ci5yd3RoLWFhY2hlbi5kZQ==
Disclaimer: All claims expressed in this article are solely those of the authors and do not necessarily represent those of their affiliated organizations, or those of the publisher, the editors and the reviewers. Any product that may be evaluated in this article or claim that may be made by its manufacturer is not guaranteed or endorsed by the publisher.
Research integrity at Frontiers
Learn more about the work of our research integrity team to safeguard the quality of each article we publish.