- Virtual Experiences Research Group, Department of Computer and Information Science and Engineering, University of Florida, Gainesville, FL, United States
Introduction: Virtual human work regarding gender is widely based on binary gender despite recent understandings of gender extending beyond female and male. Additionally, gender stereotypes and biases may be present in virtual human design.
Methods: This study evaluates how face gender cues are implemented in virtual humans by conducting an exploratory study where an undergraduate computing population (n = 67) designed three virtual human faces—female, male, and nonbinary.
Results: Results showed that face gender cues were implemented in stereotypical ways to represent binary genders (female and male virtual humans). For nonbinary gender virtual humans, stereotypical face gender cues were expressed inconsistently (sometimes feminine, sometimes masculine), and conflicting gender cues (pairs of cues that signal opposing binary gender) occurred frequently. Finally, results revealed that not all face gender cues are leveraged equally to express gender.
Discussion: Implications of these findings and future directions for inclusive and representative gender expression in virtual humans are discussed.
1 Introduction
Virtual humans are computer-generated characters that can act, look, and talk like real humans (Garau et al., 2005). Due to their human-like capabilities, gender is a characteristic commonly applied to virtual humans (Reeves and Nass, 1996). Embodying gender has considerable impacts. For example, it can be leveraged and tailored for health contexts (ter Stal et al., 2020; Alsharbi and Richards, 2017; Zalake et al., 2019) and serve as social models for women in engineering (Rosenberg-Kima et al., 2008).
However, a major drawback to gendering virtual humans is the potential presence and reinforcement of gender biases and stereotypes (Khan and Angeli, 2009; Silvervarg et al., 2012; Brahnam and De Angeli, 2012). There is a growing concern regarding how developers might play into stereotypes, and the ethical concerns behind it (Fossa and Sucameli, 2022). For example, recent studies have shown that female virtual humans not only experience explicit gender bias when behaving counter-stereotypically (Wessler et al., 2022), but implicit bias as well (Koda et al., 2022).
Gendering virtual humans can also contribute to the reinforcement of the binary gender system due to most virtual human gender work being limited to female and male gender (Loveys et al., 2020; Ter Stal et al., 2020). A more recent understanding in psychology informs that human gender is beyond the two categories of female and male (Morgenroth and Ryan, 2021; Hyde et al., 2019). Several terms have been used to describe genders outside this binary, with nonbinary commonly used as an umbrella term (Thorne et al., 2019) (and the term we will use in this paper). This prevalence of binary gender in virtual humans is likely connected to the dominance of men in computer science research (Jaccheri et al., 2020) alongside men’s increased likelihood of reacting negatively to nonbinary gender (Morgenroth and Ryan, 2021). However, with the increasing visibility of nonbinary individuals (Liszewski et al., 2018), it is important for virtual human developers to expand their understanding of gender beyond a binary system for a more inclusive representation of gender in virtual humans.
Hence, following from the concerns of the presence of gender bias in virtual humans, this study aims to explore how future potential developers implement gender–including nonbinary–in virtual humans. We explore face gender cues, or facial features that are known to signal gender–specifically, age, hair, makeup, facial hair, eyebrow thickness, eyelash length (O’Toole et al., 1998; Hess et al., 2004; O’Toole et al., 1998; Kuehlkamp et al., 2017; Pathoulas et al., 2022; O’Toole et al., 1998; Komori et al., 2011). We explore face gender cues for two reasons: (1) the face plays an important role when communicating visual information such as gender (O’Toole et al., 1998), and (2) the face is the focal point of many virtual human interactions (Feijóo-García et al., 2021; Zalake et al., 2019; Forlizzi et al., 2007). Additionally, it is important to note that previous literature has studied these facial features in the context of binary gender, and therefore these features might be constrained by a binary system. Nonetheless, it is a starting point for exploring and expanding gender expression in virtual humans.
Therefore, there is a need to re-evaluate these face gender cues in female, male, and nonbinary virtual humans. We conducted a repeated measures exploratory study in which undergraduate computing students (n = 67) from a North American university were tasked with designing three virtual human faces of different genders: female, male, and nonbinary. We sampled an undergraduate computing population as they represent a significant demographic that will shape the future of technology design. We explore the following research question.
How are face gender cues (age, use of cosmetics, facial hair, hairstyle, eyebrow thickness, and eyelash length) implemented by an undergraduate computing population to express gender in virtual humans?.
2 Related work
In this section, we first provide some background and a modern understanding of gender. Then, we explore literature related to visual gender cues. Finally, we discuss the state of gender in virtual humans in HCI.
2.1 Gender identity and gender expression
The assumption that there are only two genders–female and male–is known as the gender binary (Hyde et al., 2019). The gender binary is often associated with the view that there are only two genders based on the biological sex of a person and is immutable and does not change over time (Bohan, 1993). However, gender is much more diverse, complex, and fluid. For example, there individuals who consider themselves between binary gender or identify as female at one moment, and male the next (Darwin, 2017). In addition to identifying as female or male, people can also identify as neither female nor male, both at the same time, different genders (not limited to female and male) at different times, or no gender at all (Richards et al., 2016). “Nonbinary” is an umbrella term often used to describe these identities, and the term we will use in this paper.
When discussing gender in the context of this paper, it is important to make the distinction between gender identity and gender expression. Gender identity is internal to the individual (Morgenroth and Ryan, 2021), whereas gender expression refers to how one chooses to outwardly express gender (Matsuno and Budge, 2017). Therefore, in this work, we focus on gender expression in regard to virtual humans. Most gender-related work with virtual humans is through a gender binary lens, exploring embodiment of only female and male gender (Silvervarg, 2016; Niculescu et al., 2009). That being said, there have been studies exploring androgynous (or neutral) gender expression in humans (Nag and Yalçın, 2020; Araujo et al., 2022) in attempts to decrease the negative effects of stereotyping with virtual humans.
Our work aims to expand our understanding of how gender is embodied in virtual humans by acknowledging nonbinary gender. Specifically, we explore how face gender cues can be used to express not only female and male gender in virtual characters, but nonbinary gender as well.
2.2 Face gender cues and conflicting gender cues
When it comes to humans, the face plays an important role in communicating visual information, including gender (O’Toole et al., 1998). Eyebrow thickness is one of these cues, with thicker eyebrows being linked to perceptions dominance and typical in male faces (Hess et al., 2004). Meanwhile, long eyelashes are considered to render a face more attractive, and attractiveness is associated with femininity (O’Toole et al., 1998). Use of cosmetics (versus no cosmetics applied) is also a cue that can signal female gender (Kuehlkamp et al., 2017), whereas facial hair is important to male gender (Pathoulas et al., 2022). Hairstyle (short or long), is another feature, with long hair associated with female gender (O’Toole et al., 1998). Finally, age has been linked to perceived femininity (Komori et al., 2011).
Many of these face gender cues can be found as modifiable features in virtual character creation technologies (Engine, 2021; Reallusion, 2022; Me, 2015). However, these cues have been predominantly studied in the context of female and male gender (both with real humans and virtual humans). As discussed in the previous section, modern understanding of gender has evolved beyond that. There is little work regarding visual face gender cues for nonbinary gender. This might be due to the diverse group of individuals that fall under this umbrella term, making it difficult to capture all the possible facial presentations. More importantly, however, this is likely due to the very nature of nonbinary gender–it exists beyond the gender binary, and these face gender cues are historically binary. Therefore, it is difficult to present nonbinary facial representations in the context of these binary face gender cues.
Despite the possible constraints these binary-based face gender cues might have, it serves as a starting point for the exploration and expansion of gender expression in virtual humans. There has been some work exploring how nonbinary gender might be expressed within the constraints of a binary lens with humans. One study (Darwin, 2017) explored strategies nonbinary people employed in order to visually express their gender identity. One strategy was mixing gender cues (e.g., wearing feminine clothing but in a color scheme more typically masculine), using conflicting gender cues to create a visible gender outside of female and male. It is important to note that this strategy for visually expressing gender is not indicative of a “right way” to express nonbinary gender identity, but is an example of how nonbinary individuals “do” their gender in a binary system.
When it comes to virtual humans, there is a need to evaluate binary face gender cues in order to assess how and to what extent potential developers (undergraduate computing students) employ them to express gender. We will analyze how face gender cues are expressed, in terms of being expressed in a stereotypically masculine or feminine way. In addition, we will explore the presence of any conflicting gender cues–i.e., the co-presence of a stereotypically masculine-expressed face gender cue and a stereotypically feminine-expressed face gender cue–inspired by one of the strategies nonbinary individuals use to express gender in a binary system. This work contributes an updated understanding of how potential virtual human developers might implement facial stereotypical cues to signal gender in virtual humans.
2.3 Gender, bias, and virtual humans
According to the Computers Are Social Actors (CASA) paradigm, humans tend to apply social rules, roles, and expectations to computers. This includes gender. Gender is especially leveraged with virtual humans due to their visual nature. Although some work shows that virtual human gender has no effect on interactions (Shiban et al., 2015; Swidrak and Pochwatko, 2019), other work demonstrates benefit depending on the user’s gender and context of the interaction (Zalake et al., 2019; Forlizzi et al., 2007; Feijóo-García et al., 2021; Rosenberg-Kima et al., 2008). Despite the known benefits, the CASA paradigm extends to bias and stereotypes as well. While implementing gender in virtual humans has its benefits, it can also embody and perpetuate bias. A widely known example is the video game industry, where female video game characters tend to be younger, attractive, and hypersexualized, contributing to the objectification of women in video games (Miller and Summers, 2007; Gestos et al., 2018; Beasley and Collins Standley, 2002). However, gender bias in virtual humans persists beyond the entertainment industry. Studies in HCI have shown female virtual humans can experience social backlash, a type of gender bias that results in a negative evaluation due to stereotype violation (Wessler et al., 2022). Additionally, another study showed that users can also hold implicit biases against female virtual humans (Koda et al., 2022). There is a growing concern regarding how developers might play into stereotypes, and the ethical concerns behind it (Fossa and Sucameli, 2022).
Therefore, the concern of developers playing into and reinforcing gender stereotypes highlights the importance of this work. Our work recruited undergraduates students in computing courses, which represent a significant demographic that will shape the future of technology design. Their perspectives and biases have the potential to influence the future of virtual human design. By targeting this population, our study offers valuable insights that can inform the development of ethical guidelines and design practices for the future of virtual human development. This work provides insights into where the future of gender expression in virtual humans is headed. From there, we can start identifying areas to improve gender representation and inclusion.
3 Materials and methods
To address our research question, we conducted a repeated-measures study with undergraduate computing students from a major university in North America. This study was IRB approved (IRB202200623). Participants were tasked with designing three virtual humans–female, male, and nonbinary–using a web-based virtual human creation platform. Specifically, they designed only the virtual humans’ faces. We limited design to the face for two reasons: 1) the face plays an important role in communicating information about a person, and 2) the face is the focal point of many virtual human interactions (Feijóo-García et al., 2021; Zalake et al., 2019; Forlizzi et al., 2007). Data was collected via a post-questionnaire. The entire study took 30–60 min.
3.1 System description
Participants used Unreal Engine’s MetaHuman Creator (Engine, 2021) to design the virtual human faces. This platform was chosen due to Unreal Engine’s popularity as one of the most widely used game engines (Politowski et al., 2021). Additionally, the MetaHuman Creator is a web-based platform, which makes creating virtual characters even more accessible to developers who might not have 3D modeling experience.
The MetaHuman Creator interface allows users to modify features of the virtual human through an interactive GUI via UI elements such as sliders and selection (Figure 1). The way a feature is modified depends on what the feature is. Age is modified using a slider scale denoted in the MetaHuman Creator as “Texture”. Hairstyle, eyebrows, eyelashes, and facial hair are modified using selection.
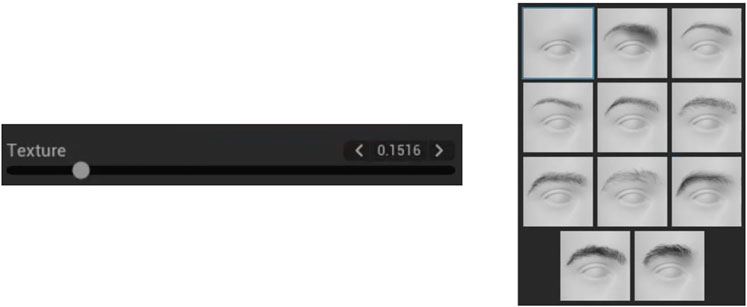
FIGURE 1. Ways in which users can modify virtual human features in MetaHuman Creator (left to right: slider, selection).
In order to mitigate any biases from the system, all participants started with a “neutral” virtual human when designing each face. The “neutral” virtual human’s features were either zero’ed out (in the case of the age slider) or taken off entirely by selecting a “none” option (in the case of hairstyle, eyebrows, eyelashes, and facial hair).
3.2 Participants
A sample population of undergraduate computing students from a major university in North America was recruited for the study. Compensation was provided in the form of course credit for. 95 total participants were recruited regardless of age, gender, and ethnicity. 28 participants’ data was dismissed due to technical difficulties and incomplete post-questionnaire submissions. Hence, we ultimately ended up with a sample population of 67 participants.
The final sample population’s reported age was 19–39 years old, with the mean age being 22.22 years (SD = 3.663). Participants’ gender was reported as: 22.4% female (n = 15), 73.1% male (n = 49), 1.5% nonbinary (n = 1), and 3% female and nonbinary (n = 2). Racial ethnic groups were reported as follows: 37.3% of our participants were Asian (n = 25), 13.4% Hispanic/Latin American (n = 9), and 49.3% White: Non-Hispanic, Non-Latin American (n = 33). We also asked participants to report whether they were a domestic US student (n = 57, or 85.1%) or an international student (n = 10, or 14.9%).
3.3 Procedure
A repeated measures study was conducted in which each participant designed virtual human faces–female, male, and nonbinary–using Unreal Engine’s web-based MetaHuman Creator. In order to mitigate any learning effect that may have occurred from using the web-based software, a Latin Squares design (McNemar, 1951) was used to vary the order in which participants created the virtual humans. All participants started with the same neutral virtual human for each virtual human they created. Participants were asked to only modify the virtual humans’ faces.
Participants completed the study as follows: First, participants signed an informed consent form. Then, they created three folders on their computer: one for each virtual human gender. Next, they proceeded to create each virtual human (starting from the “neutral” virtual human described in Section 3.1). After creating each virtual human, they took screenshots of all the settings they altered and saved the images to the appropriate folder they created at the beginning of the study. Once they finished all three virtual humans, participants zipped each folder. Finally, participants completed the post-questionnaire, where they uploaded the three zipped folders after completing the demographics questionnaire.
3.4 Data analysis
To analyze the virtual humans, the zipped folders containing the screenshots of the settings were first downloaded from the post-questionnaire and then unzipped. The extracted images were analyzed based on how the user modified the setting (slider or selection), and what research question was being addressed.
For the age slider, the value was directly noted down from the MetaHuman Creator. For the features modified via selection, these images were coded in a binary manner in one of two ways depending on the feature. For cosmetics and facial hair, it was coded based on if the user selected an option other than “none” in order to denote the presence of makeup or facial hair. For hairstyle, eyebrows, and eyelashes, the MetaHuman Creator provides short descriptions of each option. These short descriptions were used to code each feature. Ultimately, the data was coded as follows:
• Age: numeric value from slider
• Use of Cosmetics: (0) no cosmetics used, (1) cosmetics used
• Facial Hair: (0) no facial hair, (1) facial hair
• Hairstyle: (0) short, (1) long
• Eyebrows: (0) narrow, (1) thick
• Eyelashes: (0) short, (1) long
We also examined conflicting gender cues by pairing certain features together in order to denote a conflicting cue. The features that were paired together were: use of cosmetics and facial hair, and eyebrow thickness and eyelash length. Use of cosmetics is a typically female gender cue, and presence of facial hair is a typically male gender cue. If both these features appeared together in the virtual human, it was denoted as a conflicting cue. Narrow eyebrows is a typically female gender cue, and short eyelashes is a typically male gender cue. Therefore, if a virtual human was given narrow eyebrows and short eyelashes, this was considered a conflicting cue (and same for thick eyebrows and long eyelashes). Therefore, conflicting cues were coded as follows:
• Conflicting Cues - Cosmetics and Facial Hair: (0) no conflicting cue, (1) conflicting cue
• Conflicting Cues - Eyebrow Thickness and Eyelash Length: (0) no conflicting cue, (1) conflicting cue
4 Results
This section reports the results from the data analysis process presented in Section 3.4. Data analysis was conducted in SPSS Statistics 27. For age, we conducted a nonparametric Friedman test due to repeated measures, continuous outcome variable, multilevel input variable (3 virtual human genders), and non-normality of our data. For the remaining cues, we conducted a Cochran’s Q omnibus test due to the repeated measures, categorical dichotomous outcome variable, and multilevel input variable (3 virtual human genders). All post hoc analyses were conducted using Bonferroni adjusted p-values of .0167.
4.1 Face gender cues
To address our research question, we analyzed how face gender cues (age, use of cosmetics, facial hair, hairstyle, eyebrows, and eyelashes) were applied to virtual humans of different genders.
4.1.1 Age
We found a significant difference between the genders (p < .001) when it came to the age of the virtual humans. Post-hoc analysis found all three genders’ ages were significantly different, with the female virtual humans expressed as youngest, followed by nonbinary, and then male. Specifically, female virtual humans were significantly younger than the male (p < .001) and nonbinary p = .002) virtual humans. Nonbinary virtual humans were less pronounced in their age difference with male virtual humans, although still significant p = .016). Female virtual humans were 73% younger than the male virtual humans, and 52% younger than the nonbinary virtual humans. Nonbinary virtual humans were 23% younger than male virtual humans.
4.1.2 Use of cosmetics
We found a significant difference in the proportion of virtual humans that were given cosmetics based on the virtual human’s gender (p < .001). Upon further post hoc analysis, we found a statistically significant difference between the proportion of female and male virtual humans that had cosmetics (p < .001), and between the proportion of nonbinary and male virtual humans that had cosmetics (p < .001). As shown in Figure 2, a similar, high proportion of female and nonbinary virtual humans were applied cosmetics (97% and 88% respectively), whereas a much lower proportion of male virtual humans were applied cosmetics (25%).
4.1.3 Facial hair
We found a significant difference in the proportion of virtual humans that were given facial hair based on the virtual human’s gender (p < .001). Post-hoc analysis found that the proportion of virtual humans that were given facial hair differed between all three genders. Specifically, we found a statistically significant difference between the proportion of female and male virtual humans that had facial hair (p < .001), nonbinary and male virtual humans that had facial hair (p < .001), and nonbinary and female virtual humans that had facial hair (p < .001). As shown in the Figure 3, hardly any female virtual humans were given facial hair (1%). A higher proportion, although still fairly low, of nonbinary virtual humans were given facial hair (24%). Meanwhile, a majority of the male virtual humans were given facial hair (88%).
4.1.4 Hairstyle
We analyzed hairstyle using a Cochran’s Q omnibus test. We found a significant difference in the proportion of virtual humans that were given short versus long hair based on the virtual human’s gender (p < .001). Further post hoc analysis found that the proportion of virtual humans that were given short versus long hair differed between all three genders. Specifically, we found a statistically significant difference between the proportion of female and male virtual humans (p < .001), nonbinary and male virtual humans (p = .001), and nonbinary and female virtual humans (p < .001). As shown in the Figure 4, most female virtual humans were given a long hairstyle (96%), whereas few male virtual humans were given a long hairstyle (3%) and given a short hairstyle instead. A little over a third of nonbinary virtual humans were given long hair (36%).
4.1.5 Eyebrow thickness
We found a significant difference in the proportion of virtual humans that were given thick versus narrow eyebrows based on the virtual human’s gender (p < .001). The post hoc analysis found that the proportion of virtual humans that were given thick versus narrow eyebrows differed between all three genders. Specifically, we found a statistically significant difference between the proportion of female and male virtual humans (p < .001), nonbinary and male virtual humans (p < .001), and female and nonbinary virtual humans (p = .004). As shown in Figure 5, few female virtual humans were given thick eyebrows (10%) and instead narrow eyebrows, whereas most male virtual humans were given thick eyebrows (75%). A third of nonbinary virtual humans were given thick eyebrows (33%).
4.1.6 Eyelash length
We found a significant difference in the proportion of virtual humans that were given long versus short eyelashes based on the virtual human’s gender (p < .001). Post-hoc analysis revealed that the proportion of virtual humans that were given long versus short eyelashes differed between all three genders. Specifically, we found a statistically significant difference between the proportion of female and male virtual humans (p < .001), nonbinary and male virtual humans (p < .001), and nonbinary and female virtual humans (p < .001). As shown in Figure 6, most female virtual humans were given long eyelashes (81%), whereas few male virtual humans were given long eyelashes (12%) and short eyelashes instead. Nonbinary virtual humans were given long and short eyelashes generally equally (43%).
4.2 Conflicting face gender cues
To further explore our research question, we analyzed how conflicting, opposing gender cues (use of cosmetics and facial hair, eyebrow thickness and eyelash length) were applied to virtual humans of different genders.
4.2.1 Use of cosmetics and facial hair
We found a significant difference in the proportion of virtual humans that were given both cosmetics and facial hair based on the virtual human’s gender (p < .001). We conducted further post hoc analysis using multiple McNemar’s tests with a Bonferroni adjusted p-value of .0167, and found that the proportion of virtual humans that were given this conflicting cue differed between the proportion of female and male virtual humans (p < .001), and female and nonbinary virtual humans (p < .001). Interestingly, this mixture of cues was present in the same proportion of male and nonbinary virtual humans (22%), whereas it was hardly present in the female virtual humans (1%) (Figure 7).
4.2.2 Eyebrow thickness and eyelash length
We found a significant difference in the proportion of virtual humans that were given either 1) thick eyebrows and long eyelashes or 2) narrow eyebrows and short eyelashes based on the virtual human’s gender (p < .01). We conducted further post hoc analysis using multiple McNemar’s tests with a Bonferroni adjusted p-value of .0167, and found that the proportion of virtual humans that were given this conflicting cue differed between male and nonbinary virtual humans (p = .015) and nonbinary and female virtual humans (p < .001). This conflicting gender cue of mismatched eyebrow thickness and eyelash length was more common in nonbinary virtual humans (57%) than male (34%) or female (18%) virtual humans (Figure 8).
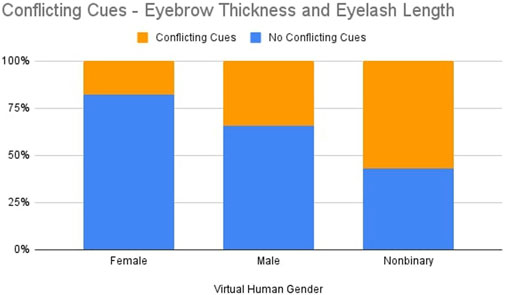
FIGURE 8. Proportion of virtual humans that had the conflicting cue of mismatched eyebrow thickness and eyelash length.
5 Discussion
This paper explored trends in how a population of undergraduate computing students implement face gender cues when designing virtual humans of different genders. Our main findings are as follows.
1. We found that participants follow stereotypical expression of face gender cues in female and male virtual humans, suggesting that gender stereotypes may influence their design choices.
2. When tasked with representing nonbinary gender within a predominantly binary gendered population, participants applied face gender cues in an inconsistent and conflicting manner. This indicates a potential lack of understanding nonbinary gender or lack of guidance regarding how to express nonbinary genders in the virtual world.
3. Finally, we found that not all face gender cues are leveraged equally when expressing gender. This highlights an area for further study–understanding when and why certain cues are favored over others.
The findings from our research offer valuable insights into the biases and challenges users face when translating real-world gender constructs into the virtual world. This section will expand on the implications of these findings.
5.1 For female and male virtual humans, face gender cues are expressed in stereotypical ways
We found that participants follow stereotypical expression of face gender cues in female and male virtual humans, suggesting that gender stereotypes may influence their design choices. This is supported by our observation that on average female virtual humans appeared 73% younger than their male counterparts, and were more frequently portrayed with cosmetics than their male and nonbinary counterparts.
Literature shows that perceived femininity is associated with youthfulness in real humans (Komori et al., 2011), and that cosmetics use is a way to make a face look younger (Porcheron et al., 2013). In females, youthfulness is a factor in determining attractiveness (Komori et al., 2011). This association of youth and femininity goes beyond reflecting a design choice, and has wider implications for how gender is perceived and represented. In the video game industry, where the use of virtual humans is prevalent, female characters tend to be younger, attractive, and hypersexualized, contributing to the objectification of women in video games (Miller and Summers, 2007; Gestos et al., 2018; Beasley and Collins Standley, 2002).
This demonstrates the consequences of a subconscious design choice that could ultimately manifest as a harmful representation (i.e., the objectification of women). Furthermore, this finding mirrors a cycle of gender expectation reinforcement (Morgenroth and Ryan, 2021; Fossa and Sucameli, 2022). Society’s gender expectations are reflected in the design of virtual humans, and these design choices, in turn, reinforce and propagate the same expectations back into society. Hence, the design of virtual humans not only reflects societal norms but also plays a significant role in shaping them. Future research is needed to more directly explore these biases. This is expanded on in more detail in Section 6.
5.2 Face gender cues are applied inconsistently and often in a conflicting manner in order to express nonbinary gender in a predominantly binary gendered population
We found that the proportion of nonbinary virtual humans with stereotypically feminine or masculine expression of gender cues was inconsistent among all gender cues. Stereotypical expression of gender cues fell somewhere between the proportions for female and male gender: for a single face gender cue, nonbinary virtual humans were expressed with feminine face gender cues at times, but masculine face gender cues at other times. For example, the frequency of facial hair in nonbinary characters was in between females and males (Figure 3). However, this trend did not persist when it came to the expression of conflicting gender cues (i.e., pairs of cues that signal opposing binary gender). Nonbinary virtual humans were most often expressed with conflicting gender cues, rather than in between how often female and male virtual humans expressed conflicting cues (for example, 8).
Given the inconsistent expression of binary face gender cues and frequent occurrence of conflicting cues for nonbinary gender, these findings suggest an undergraduate computing population’s unfamiliarity with nonbinary gender (as only three participants identified as nonbinary). Participants may have combined binary gender cues to represent a gender that does not align strictly with female or male, or a gender that is relatively unfamiliar to them (Kirsch, 2022). This practice of inconsistent and conflicting cues could also reflect participants’ observations of real-world nonbinary individuals navigating a binary gender system (Darwin, 2017). Further research is needed to fully understand these potential phenomena, and is discussed in more detail in Section 6.
5.3 Not all face gender cues are leveraged equally when expressing gender
Thus far, we have discussed the trend of females and males frequently expressed in opposing stereotypical ways with nonbinary somewhere in between. Although this trend was consistently present, it was not equally pronounced across all face gender cues. Specifically, there were two face gender cues where differences in expression were not significant between all three virtual human genders: cosmetics and eyebrow thickness. Interestingly, only male virtual humans’ expression for these cues were distinctly different (from female and nonbinary virtual humans). For example, female and nonbinary virtual humans were commonly and similarly applied cosmetics. However, male virtual humans were given cosmetics much less frequently.
The reasons behind why these face gender cues specifically were not as pronounced remains unclear. These findings suggest that not all face gender cues are leveraged equally when expressing gender; some face gender cues appear to matter more than others. Another interpretation could be that these face gender cues are more important for expressing male gender. Future research is needed to better address these interpretations. We expand on this future work in the next section.
6 Limitations and future work
This work provides a foundation to the understanding of gender representation and biases and design trends in virtual humans within an undergraduate computing population. In this section, we address limitations of our study and highlight potential avenues for future research directions as well as practical guidelines for developers.
This paper is not without its limitations. The majority of our population is male (about 75%). To address this, the authors did analyze the male and non-male (female, nonbinary, and nonbinary and female) participants’ data independently of each other, and found the same trends (although not all necessarily as pronounced) present in both populations. Therefore, the authors analyzed all the data together in the final analysis presented in this work. Also, this work aimed to gain an understanding of how prospective virtual human developers might implement gender in virtual humans, and therefore sampled a representative undergraduate computing population. The landscape of a predominantly male population speaks to wider issues with gender representation and potential bias present not only in virtual human design, but technology overall.
Following from the finding of inconsistent expression of binary face gender cues and frequent occurrence of conflicting cues for nonbinary gender, it is possible that our undergraduate computing population was largely unfamiliar with nonbinary gender (as only three participants identified as nonbinary). A limitation of this work is that we did not measure if participants had any education or familiarity with gender diversity related topics. This opens an avenue for future work exploring if gender diversity exposure and/or education could affect how users express gender in virtual humans.
Additional future work should more directly investigate the link between bias–both explicit and implicit–and the design choices of a computing population. Additional future work stemming from this study involves having a separate set of representative users evaluate the genders of the virtual humans–that is, having female participants evaluate female virtual humans, and so on–to see if perception differed. Such work can inform and improve on proper representation and inclusiveness in virtual human design when it comes to gender. For developers, we suggest that they have their virtual human designs choices (especially those related to gender) vetted by the populations they are intended to represent. This could mitigate and prevent the propagation of biases that the population faces.
The authors are aware that nonbinary gender expression may not be adequately captured by the binary face gender cues explored in this study. Analyzing nonbinary gender through a binary lens is limiting and potentially reductive. That being said, the results should be interpreted a starting point for assessing how nonbinary gender might be expressed with our current technologies, albeit a binary lens, in order to consider the limitations and constraints. Exploring this with an undergraduate computing population also enhances our current understanding of how nonbinary virtual humans might be currently represented, as they are representative of the virtual human development field.
As a result, our study provides an initial perspective on how nonbinary gender is likely to be represented in virtual humans based on a representative undergraduate computing population and a popular virtual human creation platform. As mentioned earlier, the historical binary perspective of gender in virtual human development might limit the implementation of nonbinary genders in current creation platforms. This work highlights the importance of future work exploring nonbinary gender expression with a nonbinary population: their perceived limitations and benefits of existing virtual character creation platforms could guide inclusive and representative virtual human design. Future developers of virtual human creation platforms should work with nonbinary populations in order to create platforms that embrace gender diversity.
Another limitation was imposed by the virtual human creation platform. For example, the age slider did not measure age in years. However, this enabled the authors to interpret the findings as overarching trends rather than specific numbers. This presentation allows for a more digestible interpretation of results, and that underlying trends are more insightful in the context of our research question. Based on our findings, future research should delve into these trends more quantitatively, offering a richer numerical analysis.
Finally, our findings uncover an area for future work regarding gender expression with virtual humans. Future research should aim to identify the most impactful face gender cues in expressing gender and examine if their importance varies depending on the gender being expressed. Additionally, the impact of the face gender cue should be explored in the context of the designer’s own gender–for example, male designers might leverage cues differently than nonbinary designers. This will provide further clarity for guidelines on face gender cues and their role in gender expression within virtual human design.
7 Conclusion
This work explored how gender is expressed in virtual humans by having an undergraduate computing population design virtual human faces of 3 genders: female, male, and nonbinary. Our findings suggest that for an undergraduate computing population, binary face gender cues are used in a stereotypical manner for binary gender expression (female and male). This demonstrates societal gender expectations making their way into virtual human design and continuing to reinforce these expectations via seemingly subtle and subconscious design choices. Additionally, binary face gender cues are applied to nonbinary virtual humans in inconsistent (sometimes stereotypically female, sometimes stereotypically male) and conflicting ways (i.e., pairs of cues that signal opposing binary gender) that highlight the need to re-evaluate gender cues beyond a binary system and with a nonbinary population in order to better represent diverse genders. Finally, not all face gender cues are leveraged equally to express gender in virtual humans; some cues might matter more, and it may depend on which gender is being expressed.
Ultimately, this work serves as a starting point to provide insights on the current state of how gender is expressed in virtual humans, specifically with a potential population of developers. From this work, we conclude that developers should be more aware and intentional with their design choices when implementing gender in order to prevent accidental reinforcement of bias. This can be achieved by having designs vetted by the population the virtual human is intended to represent. Furthermore, to expand beyond a binary system, virtual human gender needs to be studied with a nonbinary population in order to better understand the constraints of current virtual human development and areas to improve. Developers of virtual human creation platforms should actively involve nonbinary populations in the development of their systems in order to create diversity embracing, inclusive platforms. Finally, our work uncovered a novel area warranting further research in understanding the role face gender cues play in signalling gender in virtual humans. Future research is needed to explore what face gender cues are most important in expressing gender, and what underlying factors influence the importance of those cues.
Ultimately, our current findings contribute important insights for the diversity and inclusion in the virtual human community. We hope that these exploratory findings inspire and encourage more exploration to update and broaden our understanding of gender expression in virtual humans in order to promote proper representation and improve accessibility for diverse genders.
Data availability statement
The raw data supporting the conclusion of this article will be made available by the authors, without undue reservation.
Ethics statement
The studies involving humans were approved by University of Florida Institutional Review Board. The studies were conducted in accordance with the local legislation and institutional requirements. The participants provided their written informed consent to participate in this study.
Author contributions
RG conducted the user study, carried out the data analysis, and wrote the manuscript in consultation with all others. PF-G was the instructor for the course in which the user study was conducted. All authors contributed to the article and approved the submitted version.
Acknowledgments
We would like to thank the members of the Virtual Experiences Research Group in their help in providing feedback.
Conflict of interest
The authors declare that the research was conducted in the absence of any commercial or financial relationships that could be construed as a potential conflict of interest.
Publisher’s note
All claims expressed in this article are solely those of the authors and do not necessarily represent those of their affiliated organizations, or those of the publisher, the editors and the reviewers. Any product that may be evaluated in this article, or claim that may be made by its manufacturer, is not guaranteed or endorsed by the publisher.
References
Alsharbi, B., and Richards, D. (2017). “Using virtual reality technology to improve reality for young people with chronic health conditions,” in Proceedings of the 9th international conference on computer and automation engineering. (ICCAE '17) (New York, NY, USA: Association for Computing Machinery), 11–15. doi:10.1145/3057039.305708
Araujo, V., Schaffer, D., Costa, A. B., and Musse, S. R. (2022). “Towards virtual humans without gender stereotyped visual features,” in SIGGRAPH asia 2022 technical communications. (SA '22) (New York, NY, USA: Association for Computing Machinery), 1–4. doi:10.1145/3550340.3564232
Beasley, B., and Collins Standley, T. (2002). Shirts vs. skins: clothing as an indicator of gender role stereotyping in video games. Mass Commun. Soc. 5, 279–293. doi:10.1207/s15327825mcs0503_3
Bohan, J. S. (1993). Regarding gender: essentialism, constructionism, and feminist psychology. Psychol. women Q. 17, 5–21. doi:10.1111/j.1471-6402.1993.tb00673.x
Brahnam, S., and De Angeli, A. (2012). Gender affordances of conversational agents. Interact. Comput. 24, 139–153. doi:10.1016/j.intcom.2012.05.001
Darwin, H. (2017). Doing gender beyond the binary: A virtual ethnography. Symb. Interact. 40, 317–334. doi:10.1002/symb.316
Feijóo-García, P. G., Zalake, M., de Siqueira, A. G., Lok, B., and Hamza-Lup, F. (2021). “Effects of virtual humans’ gender and spoken accent on users’ perceptions of expertise in mental wellness conversations,” in Proceedings of the 21st ACM international conference on intelligent virtual agents (New York, NY, USA: Association for Computing Machinery), 68–75. doi:10.1145/3472306.3478367
Forlizzi, J., Zimmerman, J., Mancuso, V., and Kwak, S. (2007). “How interface agents affect interaction between humans and computers,” in Proceedings of the 2007 conference on Designing pleasurable products and interfaces (DPPI '07) (New York, NY, USA: Association for Computing Machinery), 209–221.
Fossa, F., and Sucameli, I. (2022). Gender bias and conversational agents: an ethical perspective on social robotics. Sci. Eng. Ethics 28, 23. doi:10.1007/s11948-022-00376-3
Garau, M., Slater, M., Pertaub, D.-P., and Razzaque, S. (2005). The responses of people to virtual humans in an immersive virtual environment. Presence Teleoperators Virtual Environ. 14, 104–116. doi:10.1162/1054746053890242
Gestos, M., Smith-Merry, J., and Campbell, A. (2018). Representation of women in video games: A systematic review of literature in consideration of adult female wellbeing. Cyberpsychology, Behav. Soc. Netw. 21, 535–541. doi:10.1089/cyber.2017.0376
Hess, U., Adams, R. B., and Kleck, R. E. (2004). Facial appearance, gender, and emotion expression. Emotion 4, 378–388. doi:10.1037/1528-3542.4.4.378
Hyde, J. S., Bigler, R. S., Joel, D., Tate, C. C., and van Anders, S. M. (2019). The future of sex and gender in psychology: five challenges to the gender binary. Am. Psychol. 74, 171–193. doi:10.1037/amp0000307
Jaccheri, L., Pereira, C., and Fast, S. (2020). “Gender issues in computer science: lessons learnt and reflections for the future,” in 2020 22nd international symposium on symbolic and numeric algorithms for scientific computing (SYNASC) (IEEE), 9–16. doi:10.48550/arXiv.2102.00188
Khan, R., and Angeli, A. D. (2009). “The attractiveness stereotype in the evaluation of embodied conversational agents,” in IFIP conference on human-computer interaction (Springer), 85–97.
Kirsch, H. (2022). Effect of conflicting gender cues on the cognitive availability of nonbinary they. University of Pittsburgh. Ph.D. thesis.
Koda, T., Tsuji, S., and Takase, M. (2022). “Measuring subconscious gender biases against male and female virtual agents in Japan,” in Proceedings of the 10th international conference on human-agent interaction. (HAI '22) (New York, NY, USA: Association for Computing Machinery), 275–277. doi:10.1145/3527188.3563909
Komori, M., Kawamura, S., and Ishihara, S. (2011). Multiple mechanisms in the perception of face gender: effect of sex-irrelevant features. J. Exp. Psychol. Hum. Percept. Perform. 37, 626–633. doi:10.1037/a0020369
Kuehlkamp, A., Becker, B., and Bowyer, K. (2017). “Gender-from-iris or gender-from-mascara?,” in 2017 IEEE winter conference on applications of computer vision (WACV) (IEEE), 1151–1159. doi:10.1109/WACV.2017.133
Liszewski, W., Peebles, J. K., Yeung, H., and Arron, S. (2018). Persons of nonbinary gender—Awareness, visibility, and health disparities. N. Engl. J. Med. 379, 2391–2393. doi:10.1056/nejmp1812005
Loveys, K., Sebaratnam, G., Sagar, M., and Broadbent, E. (2020). The effect of design features on relationship quality with embodied conversational agents: A systematic review. Int. J. Soc. Robotics 12, 1293–1312. doi:10.1007/s12369-020-00680-7
Matsuno, E., and Budge, S. L. (2017). Non-binary/genderqueer identities: A critical review of the literature. Curr. Sex. Health Rep. 9, 116–120. doi:10.1007/s11930-017-0111-8
McNemar, Q. (1951). On the use of Latin squares in psychology. Psychol. Bull. 48, 398–401. doi:10.1037/h0060348
Miller, M. K., and Summers, A. (2007). Gender differences in video game characters’ roles, appearances, and attire as portrayed in video game magazines. Sex. roles 57, 733–742. doi:10.1007/s11199-007-9307-0
Morgenroth, T., and Ryan, M. K. (2021). The effects of gender trouble: an integrative theoretical framework of the perpetuation and disruption of the gender/sex binary. Perspect. Psychol. Sci. 16, 1113–1142. doi:10.1177/1745691620902442
Nag, P., and Yalçın, Ö. N. (2020). “Gender stereotypes in virtual agents,” in Proceedings of the 20th ACM International conference on intelligent virtual agents (New York, NY, USA: Association for Computing Machinery), 1–8. doi:10.1145/3383652.3423876
Niculescu, A., Van Der Sluis, F., and Nijholt, A. (2009). “Feminity, masculinity and androgyny: how humans perceive the gender of anthropomorphic agents,” in Proceedings of 13th international conference on human-computer interaction (Heidelberg: Springer Verlag), 628–632.
O’Toole, A. J., Deffenbacher, K. A., Valentin, D., McKee, K., Huff, D., and Abdi, H. (1998). The perception of face gender: the role of stimulus structure in recognition and classification. Mem. cognition 26, 146–160. doi:10.3758/bf03211378
Pathoulas, J. T., Flanagan, K. E., Walker, C. J., Wiss, I. M. P., Marks, D., and Senna, M. M. (2022). Characterizing the role of facial hair in gender identity and expression among transgender men. J. Am. Acad. Dermatology 87, 228–230. doi:10.1016/j.jaad.2021.07.060
Politowski, C., Petrillo, F., Montandon, J. E., Valente, M. T., and Guéhéneuc, Y.-G. (2021). Are game engines software frameworks? A three-perspective study. J. Syst. Softw. 171, 110846. doi:10.1016/j.jss.2020.110846
Porcheron, A., Mauger, E., and Russell, R. (2013). Aspects of facial contrast decrease with age and are cues for age perception. PloS one 8, e57985. doi:10.1371/journal.pone.0057985
Reeves, B., and Nass, C. (1996). The media equation: How people treat computers, television, and new media like real people, 10. Cambridge, UK. 236605.
Richards, C., Bouman, W. P., Seal, L., Barker, M. J., Nieder, T. O., and T’Sjoen, G. (2016). Non-binary or genderqueer genders. Int. Rev. Psychiatry 28, 95–102. doi:10.3109/09540261.2015.1106446
Rosenberg-Kima, R. B., Baylor, A. L., Plant, E. A., and Doerr, C. E. (2008). Interface agents as social models for female students: the effects of agent visual presence and appearance on female students’ attitudes and beliefs. Comput. Hum. Behav. 24, 2741–2756. doi:10.1016/j.chb.2008.03.017
Shiban, Y., Schelhorn, I., Jobst, V., Hörnlein, A., Puppe, F., Pauli, P., et al. (2015). The appearance effect: influences of virtual agent features on performance and motivation. Comput. Hum. Behav. 49, 5–11. doi:10.1016/j.chb.2015.01.077
Silvervarg, A. (2016). “How students perceive the gender and personality of a visually androgynous agent,” in International conference on intelligent virtual agents (Springer), 420–423.
Silvervarg, A., Raukola, K., Haake, M., and Gulz, A. (2012). “The effect of visual gender on abuse in conversation with ecas,” in International conference on intelligent virtual agents (Springer), 153–160.
Swidrak, J., and Pochwatko, G. (2019). “Being touched by a virtual human. relationships between heart rate, gender, social status, and compliance,” in Proceedings of the 19th ACM international conference on intelligent virtual agents (New York, NY, USA: Association for Computing Machinery), 49–55. doi:10.1145/3308532.3329467
Ter Stal, S., Kramer, L. L., Tabak, M., op den Akker, H., and Hermens, H. (2020). Design features of embodied conversational agents in ehealth: A literature review. Int. J. Human-Computer Stud. 138, 102409. doi:10.1016/j.ijhcs.2020.102409
ter Stal, S., Tabak, M., op den Akker, H., Beinema, T., and Hermens, H. (2020). Who do you prefer? The effect of age, gender and role on users’ first impressions of embodied conversational agents in ehealth. Int. J. Human–Computer Interact. 36, 881–892. doi:10.1080/10447318.2019.1699744
Thorne, N., Yip, A. K.-T., Bouman, W. P., Marshall, E., and Arcelus, J. (2019). The terminology of identities between, outside and beyond the gender binary–a systematic review. Int. J. Transgenderism 20, 138–154. doi:10.1080/15532739.2019.1640654
Wessler, J., Schneeberger, T., Christidis, L., and Gebhard, P. (2022). “Virtual backlash: nonverbal expression of dominance leads to less liking of dominant female versus male agents,” in Proceedings of the 22nd ACM international conference on intelligent virtual agents (New York, NY, USA: Association for Computing Machinery), 1–8. doi:10.1145/3514197.3549682
Zalake, M., Tavassoli, F., Griffin, L., Krieger, J., and Lok, B. (2019). “Internet-based tailored virtual human health intervention to promote colorectal cancer screening: design guidelines from two user studies,” in Proceedings of the 19th ACM international conference on intelligent virtual agents (IVA '19) (New York, NY, USA: Association for Computing Machinery), 73–80. doi:10.1145/3308532.3329471
Keywords: virtual humans, virtual agent, gender, user studies, design study
Citation: Ghosh R, Feijóo-García PG, Stuart J, Wrenn C and Lok B (2023) Evaluating face gender cues in virtual humans within and beyond the gender binary. Front. Virtual Real. 4:1251420. doi: 10.3389/frvir.2023.1251420
Received: 01 July 2023; Accepted: 14 August 2023;
Published: 24 August 2023.
Edited by:
Ali Arya, Carleton University, CanadaCopyright © 2023 Ghosh, Feijóo-García, Stuart, Wrenn and Lok. This is an open-access article distributed under the terms of the Creative Commons Attribution License (CC BY). The use, distribution or reproduction in other forums is permitted, provided the original author(s) and the copyright owner(s) are credited and that the original publication in this journal is cited, in accordance with accepted academic practice. No use, distribution or reproduction is permitted which does not comply with these terms.
*Correspondence: Rashi Ghosh, cmFzaGlnaG9zaEB1ZmwuZWR1