- 1Touch Lab, Department of Applied Mechanics and Biomedical Engineering, IIT Madras, Chennai, India
- 2Experiential Technology Innovation Center (XTIC), IIT Madras, Chennai, India
- 3Event Lab, Faculty of Psychology, University of Barcelona, Barcelona, Spain
- 4Institute of Neurosciences of the University of Barcelona, Barcelona, Spain
- 5Arts and Humanities Division, Interactive Media, New York University Abu Dhabi, Abu Dhabi, United Arab Emirates
Research has shown that incorporating haptics into virtual environments can increase sensory fidelity and provide powerful and immersive experiences. However, current studies on haptics in virtual interactions primarily focus on one-on-one scenarios, while kinesthetic haptic interactions in large virtual gatherings are underexplored. This study aims to investigate the impact of kinesthetic haptics on eliciting emotional responses within crowded virtual reality (VR) scenarios. Specifically, we examine the influence of type or quality of the haptic feedback on the perception of positive and negative emotions. We designed and developed different combinations of tactile and torque feedback devices and evaluated their effects on emotional responses. To achieve this, we explored different combinations of haptic feedback devices, including “No Haptic,” “Tactile Stimulus” delivering tactile cues, and “Haptic Stimulus” delivering tactile and torque cues, in combination with two immersive 360-degree video crowd scenarios, namely, “Casual Crowd” and “Aggressive Crowd.” The results suggest that varying the type or quality of haptic feedback can evoke different emotional responses in crowded VR scenarios. Participants reported increased levels of nervousness with Haptic Stimulus in both virtual scenarios, while both Tactile Stimulus and Haptic Stimulus were negatively associated with pleasantness and comfort during the interaction. Additionally, we observed that participants’ sense of touch being real was enhanced in Haptic Stimulus compared to Tactile Stimulus. The “Haptic Stimulus” condition had the most positive influence on participants’ sense of identification with the crowd.
1 Introduction
Crowd behavior is an important research topic from a social, psychological, and political perspective, and the collective emotional dynamics can play a significant role in shaping behaviors within a crowd context (Goldenberg et al., 2021). Crowds can be both overwhelming and exhilarating, and the navigation and response of individuals to them depend on their individual personalities and experiences (Kramer et al., 2014). A person may experience a violation of personal space in a congested environment, whereas another may experience positive intimacy. When our personal space is violated, it triggers an emotional response and activates our defense mechanisms that have evolved to safeguard our physical wellbeing (Todd et al., 2008).
Virtual reality (VR) has been an invaluable tool in the study of human behavior and emotional arousal in crowds (Somarathna et al., 2022), offering precise control and the replication of parameters of the virtual crowds across multiple participants with a detailed assessment of individual data. For example, a study exploring people’s responses to being part of a virtual rock concert audience found that negative sentiments sometimes resulted from interactions with the virtual audience and that the illusion of being at the concert was associated with positive sentiment for men but negative sentiments for women (Beacco et al., 2021; Slater et al., 2022). Dickinson et al. (2019) found a significant increase in negative effects as the density of a virtual crowd increased.
Nevertheless, studies examining virtual crowds are typically limited to remote crowd interactions due to the challenges in visualizing collisions within virtual environments. It is worth noting, however, that when individuals are in real-life crowd situations, human interactions involve both sharing space with others and physical contact. In order to get a deeper understanding of human behavior and interactions in crowded spaces, there has been an increasing interest in incorporating haptics in virtual crowd simulations (Hoppe et al., 2020; Della Longa et al., 2022).
Haptic displays can be classified into two types: kinesthetic or force-feedback displays that stimulate the kinesthetic system of senses and tactile haptic displays that stimulate tactile systems of senses (Srinivasan and Basdogan, 1997). Recent reviews provide comprehensive insights into various haptic technologies and their interactions with virtual objects (Giri et al., 2021; Adilkhanov et al., 2022). Although some studies have explored the use of haptics in virtual crowds, specifically to enhance the interaction with others (Hoppe et al., 2020; Della Longa et al., 2022), the majority of these have focused solely on tactile feedback (Krogmeier et al., 2019; Koilias et al., 2020a; Berton et al., 2022; Monica and Aleotti, 2023), with limited research on force-feedback displays in virtual crowd scenarios.
In this study, we investigated the contribution of various combinations of haptic types or qualities to eliciting emotional responses among participants immersed in affective crowded VR scenarios. Specifically, we examined the influence of collision–torque feedback on the perception of positive and negative emotions. To test this, we designed and developed different haptic feedback devices and evaluated their effect on emotional responses. We initially designed five different haptic devices with varying touch qualities, namely, linear actuator (LA), eccentric rotating mass (ERM) motor, electrical muscle stimulation (EMS), gyro moment device (GMD), and collision haptic device (CHD) that were tested in a pilot study. Although all five devices were evaluated positively, we had to exclude the EMS and gyro moment devices from the pilot experiment due to concerns over electrical safety and size. Based on their performance, the remaining three haptic devices (LA, ERM, and CHD) were selected for the final experiment.
2 Wearable haptic displays in social VR
Kinesthetic haptic devices provide force feedback by being “externally grounded” to another rigid body (e.g., a table, floor, or roof) (Massie and Salisbury, 1994) or “body-grounded” (e.g., exoskeleton, haptic gloves, and finger gloves) (Endo et al., 2011; Nagai et al., 2015). Unlike externally grounded force-feedback devices, body-grounded force-feedback devices are attached to the user’s body as a reference, which makes the workspace larger. Huisman (2017) provided a summary of the substantial psychological and neurological studies on the use of haptic technology in social interactions. These findings suggest that social touch technology has the potential to compensate for the absence of actual social touch and deliver meaningful tactile experiences.
Social touch is a simple yet powerful technique to communicate and convey emotions. The effects of technology-mediated social contact have been found to be similar to those of actual social touch (Huisman, 2017). In an attempt to replicate the sensation of stroking the forearm, Nunez et al. (2020) conducted a study that compared two methods for delivering sequential discrete stimulations. The first involved the application of normal indentation using a vibrotactile actuator and the second employed lateral skin-slip using rotary motors. The results showed that lateral skin-slip using rotary motors created a more continuous sensation, while reducing the number of contact points lessened the continuity. Zhu et al. (2022) used voice coil actuators to deliver touch stimuli to participants’ forearms. These findings showed that slower touch speeds were related to higher assessments of a positive emotional valence, while quicker touch speeds were associated with higher ratings of a negative emotional valence. Cui et al. (2021) studied and compared four different patterns of vibrotactile feedback, along with a condition with no tactile feedback, while hugging a virtual character. Based on subjective self-reported ratings, the study found a strong correlation between the realism of the virtual hug and the presence of vibrotactile feedback.
Wearable haptic vests have been utilized in social interactions, particularly to simulate collisions with virtual people in crowds. According to the findings of the study by Monica and Aleotti (2023), proximity-based vibrotactile haptic feedback supplied by a wearable haptic vest can considerably decrease collisions with barriers in virtual environments even with poor eyesight (e.g., walking backward or in a poorly lit environment). Krogmeier et al. (2019) compared various intensities of tactile feedback with non-haptic feedback in a virtual reality configuration where virtual characters ran against a participant standing at a crosswalk. These findings indicated that haptics result in differences in embodiment and interaction realism. Koilias et al. (2020a) compared the influence of tactile feedback on various parts of the body in virtual crowd scenes using a haptic vest. These findings showed shifts in movement behaviors, such as walking speed and trajectory. Berton et al. (2022) examined the use of vibrotactile haptics to enhance navigation in crowded VR environments. This study revealed that participants avoided collisions with the virtual crowd more actively with haptic feedback than without it.
While there is more effort being made to integrate social touch into virtual interactions, particularly into crowd collision, research continues to primarily focus on dyadic interactions with vibrotactile feedback, leaving kinesthetic haptic or force-feedback interactions in large virtual gatherings largely unexplored. Existing wearable haptic displays lack the ability to simulate heavy- or large-collision force feedback. A prototype with the output force ranging from 0 N to 25 N can be used to simulate different crowd interactions. In our study, we designed and tested wearable kinesthetic haptic devices that are capable of simulating a force feedback of 26.1 N from a torque feedback of 1.3 Nm. We describe our wearable force-feedback (collision haptic device) devices that generate an impulsive force or torques by suddenly changing the inertia of the system to mimic a shoulder collision. This device is attached and actuated at the participant’s shoulder in sync with a virtual collision with a force ranging from 0 N to 26.1 N via a 1.3 Nm torque output and tested in an experimental study to address the effect of haptics on eliciting emotional responses.
3 Materials and methods
3.1 Mechanics of a shoulder collision
We modeled the shoulder collision of two humans as a collision between two spherical rigid bodies with three major assumptions:
1. Mass of the human body: The colliding bodies are assumed to be two spherical bodies of lumped mass of m/2 each. Assuming that the average mass of the human body in a crowd is 60 kg, which is equally divided between the two shoulders due to symmetry, with mass M1 = M2 = 30 kg.
2. Colliding angle: Assuming that two shoulders collide or brush with each other at a collision angle
3. Collision state: Collision is assumed to be inelastic.
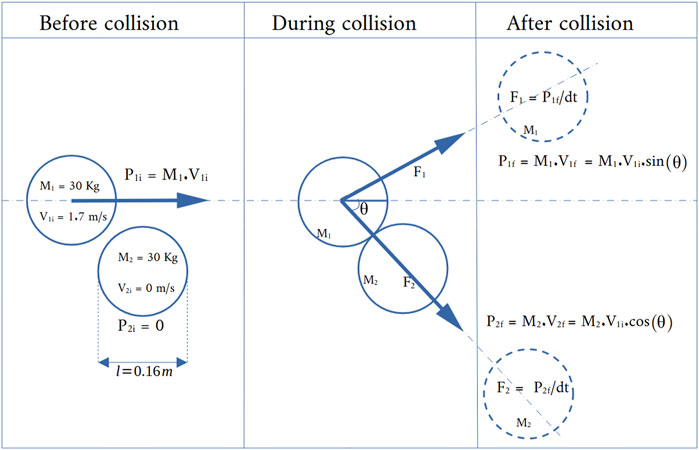
FIGURE 1. Two circles represent the top view of two people in a collision. Before collision: the moving person and the standing person with mass (M1 and M2), initial momentum (P1i and P2i), and initial velocity (V1i and V2i). The cross-sectional width of the shoulder (l) is 0.16 m. During collision: the moving person collides with the standing person at an angle (θ), resulting in two components of forces F1 and F2. After collision: final momentum (P1f and P2f) and final velocity (V1f and V2f).
With this simple model of a shoulder collision, the collision force could be estimated using the principle of the conservation of momentum. The walking speed of a person in an aggressive or busy crowd is estimated to be V1i = 1.7 m/s (Murtagh et al., 2021). With an average shoulder cross section of length l = 16 cm (Wysiadecki et al., 2014), the duration of contact (
Therefore, the minimum impact or impulsive force, as a result of the collision, is estimated to be F2 = 23.618 N. We used this estimated collision force to design a collision haptic device, as described in Section 3.2.3.
3.2 Haptic device design
Our hardware consists of three different haptic modules, namely, “linear actuator (LA),” “eccentric rotating mass (ERM),” and “collision haptic device (CHD),” to generate three varieties of touch stimuli. All the three haptic modules are attached to a common baseplate that could be fitted to the user’s shoulder or arm using a shoulder band (Supplementary Figure S2). The aim of our investigation is to contrast the types of haptic stimuli for different VR contexts.
3.2.1 Linear actuator
A linear actuator is used to push or poke the shoulder, producing a tactile cue in the negative Y-axis toward the shoulder (Figure 2B). LA is internally driven by a 12 V, 20 W geared DC motor with a torque rating of 0.15 Nm. This 0.15 Nm torque is converted to a 15 N linear push/pull force from a toothed linear shaft driven by a gear wheel of radius 1 cm. A tactile cue of 15 N force is delivered to the negative Y-axis perpendicular to and toward the XZ plane of the base of the 3D-printed shoulder rest with 170 ms response time for a stroke length of 22 mm.
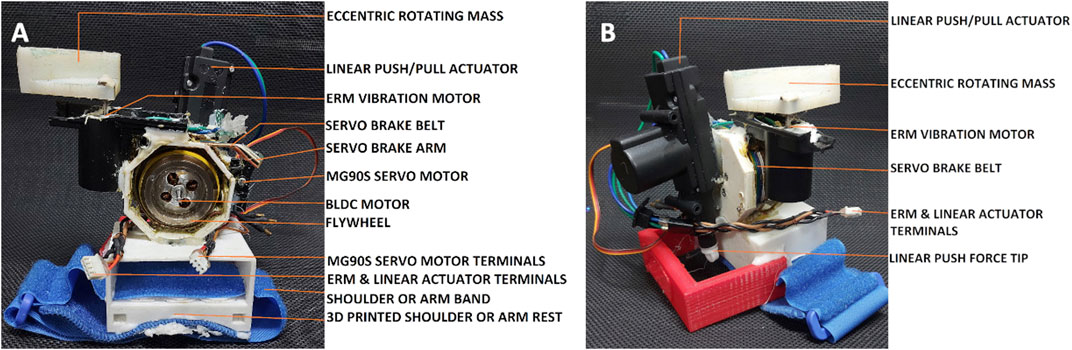
FIGURE 2. Collision haptic device: three different haptic feedback devices are attached to a common baseplate, which is fitted to the arm or shoulder via a tight shoulder or arm band. (A) Frontal view of the prototype. (B) Lateral view of the prototype.
3.2.2 Eccentric rotating mass
The eccentric rotating mass, as shown in Figure 2, is rotated once to produce a strong single eccentric vibration producing a tactile cue. It is powered by another motor in the same way as the LA (12 V, 20 W DC motor and 0.15 Nm torque) and is used to rotate an eccentric mass of 25 g at a radius of 35 mm at an angular velocity of 133.3 rad/s to produce a single eccentric vibration of amplitude equal to 15.6 G to output a centripetal force of 15.6 N in the XZ plane parallel to the base plane of the 3D-printed shoulder rest. With an output vibration frequency of 21.2 Hz and the time of actuation being programmed to be limited to 50 ms, the ERM was designed to spin only one full cycle producing a single eccentric vibration.
3.2.3 Collision haptic device
The collision haptic device was designed to mimic strong collision haptic feedback. A high-impulsive-torque feedback mechanism was developed as same as M-Blocks (Romanishin et al., 2015), which is a simple modular robotic cube capable of delivering ungrounded or mid-air clockwise and counter-clockwise torques of up to 2.6 Nm in approximately three orthogonal axes, resulting in a total of six directional torque cues. Ando et al. (2002) used the concept of this high-impulsive-torque M-Block, adopted as a wearable force display on the wrist for the perception of directional torque cues. We used a simple angular momentum conservation technique to produce impulsive torque cues.
In our prototype, a BLDC motor was used to rotate a steel flywheel with an inner radius of 11 mm, an outer radius of 25 mm, and a flywheel mass of 150 g. The BLDC motor is powered by a 12 V, 1.5 Amp, 40C lithium-ion battery. This mechanism uses the principle of the conservation of angular momentum of the flywheel and an external mechanical brake. This torque feedback is perceived as force feedback at some distance (point of attaching the prototype) from the axis of the flywheel. More details about the parameters involved in the design of the device are given in the Supplementary Material.
The torque output of CHD can be varied by controlling the parameters, such as RPM and brake time of the BLDC motor. Supplementary Figure S1A shows the angular velocity of the flywheel as a function of time, rise time of the flywheel, and brake time of the flywheel (Supplementary Figure S1B). This sharp brake signal is synchronized with visual collision events in the VR crowd scene.
3.2.4 Pilot study
A first pilot experiment was conducted, before the main experiment, which primarily focused on the qualitative perceptual outcome of each combination of haptic devices. The pilot study was conducted with 15 healthy participants (11 men and four women), with ages ranging from 22 to 35 years. The comparisons of three haptic devices (ERM, LA, and CHD) and their combinations were done based on their tactual modes (Loomis and Lederman, 2003). A description of the tactual modes and the axis of actuation for individual and combined haptic cues is presented in Supplementary Table S1.
The main objective of the pilot experiment was to rank the qualitative perceptual outcome of the eight combinations of haptic devices. Each participant was provided with three trials of seven haptic combinations (excluding “No Haptic”) of three haptic devices (LA, ERM, and CHD), summing a total of 21 trials per participant (with no audiovisual inputs). After each trial, the participants were asked to subjectively rate the perceived haptic input (“How intense/strong did you perceive the haptic feedback to be?”) using a nine-point rating scale ranging from 1, extremely weak, to 9, extremely strong. Supplementary Figure S3 shows responses reported by 15 participants for all eight combinations of three haptic devices. For our main experiment, we selected three haptic combinations from the responses of the participants and termed them “No Haptic,” in which no haptic or tactile stimulus is provided, “Haptic Stimulus” (ERM + LA + CHD) that includes both kinesthetic and tactile feedback and leads to the maximum perception of the collision, and “Tactile Stimulus” (ERM + LA) that leads to a moderate perception of the collision.
3.3 VR scene design
A crowd was simulated with a 360-degree video rendering of a real crowd in a Meta Quest 2 headset (Aggressive Crowd1 and Casual Crowd2). The 360-degree video was captured using an Insta360 Pro camera3. An open, crowded place was recorded using the camera lens adjusted and positioned at the average height of a person at 155 cm. A circle of radius 50 cm was drawn, and the camera was placed in the center of the circle to avoid artifacts from being very close. The crowd environment was recorded at a 4 K resolution at 30 FPS (Figure 3). The Casual Crowd was differentiated from the Aggressive Crowd by varying two parameters: crowd density (number of participants in the scene) and the rate of crossing the camera (speed) (for further details, see Supplementary Table S2) (Koilias et al., 2020b). The run time of each VR scene is 45 s.
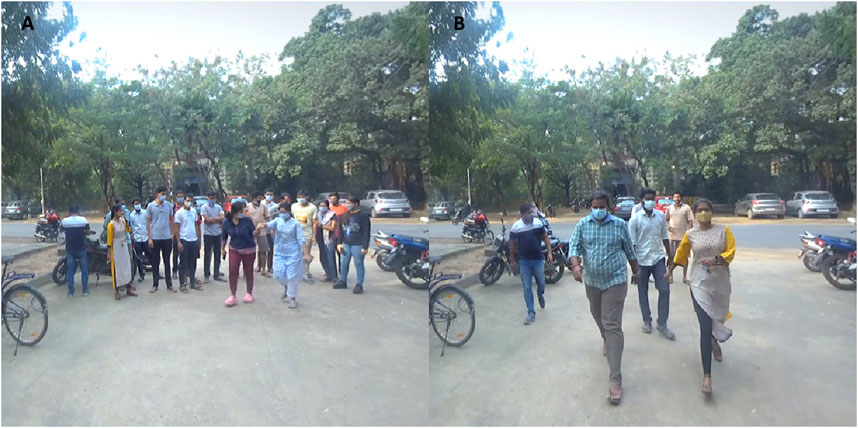
FIGURE 3. Crowd density of the two crowds recorded using the Insta360 Pro camera (Supplementary Table S2). (A) Aggressive Crowd scene showing a densely populated crowd walking at a fast pace (urgent walking). (B) Casual Crowd scene showing a sparsely populated crowd walking at a slow pace (relaxed walking).
3.4 Rendering of a 360-degree video in a VR headset
Each haptic cue was synchronized with the virtual collision in the VR crowd. The VR scene was designed using Unity 3D4 with a pre-recorded 360-degree crowd video, wrapped onto a 3D sphere (game object) using a material created with a normally flipped shader. The main camera was disabled, and the OVR Camera Rig was used as a camera. The ratio of the dimensions of the 3D sphere (where the 360-degree videos are projected) to the field of view of the OVR Camera Rig was set at 10:7 to maintain a realistic scaling of the VR environment compared with the real environment (Škola et al., 2020). The game scene in Unity was streamed wirelessly to the Meta Quest 2 connected to a PC via an air link. The participants experience the virtual crowd with the haptic prototype attached to their shoulder, as shown in Supplementary Figure S2. The total weight of the prototype is 520 g. A tight shoulder band was used to firmly hold and press the prototype over the shoulder of the participant. The Timeline function in Unity 3D was used to synchronize a visual collision event in the VR scene with the actuation of haptic devices via Unity–Arduino serial communication, as shown in Figure 4.
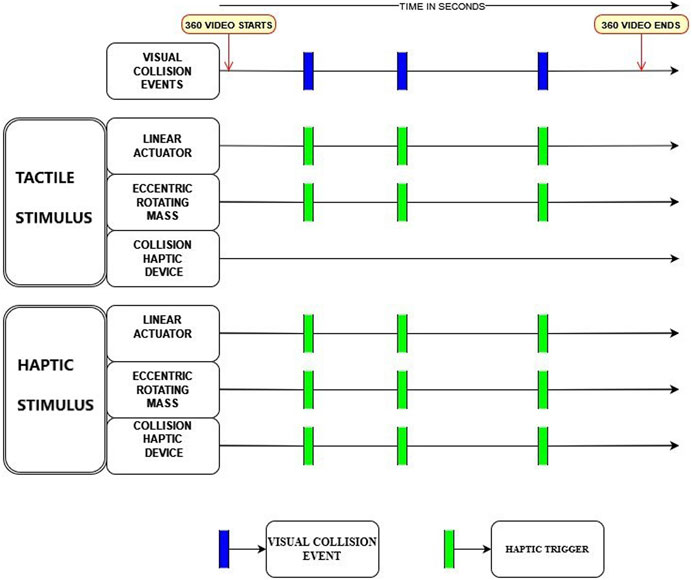
FIGURE 4. Visual collision events from the 360-degree videos are synced to the timeline script of Unity 3D to trigger the corresponding haptic devices via Unity–Arduino serial communication.
3.5 Procedures
All participants were provided with an information sheet about the experiment and were also requested to sign an informed consent form. Each participant visited the laboratory at prearranged times. After completing a demographics questionnaire, they were fitted with the HMD along with the haptic devices on their shoulders before the experiment started. Out of the 20 subjects, 10 experienced the Casual Crowd scene first, followed by the Aggressive Crowd scene, and the remaining 10 experienced the Aggressive Crowd scene first, followed by the Casual Crowd scene after a 10-min break. For each crowd scene, the participants experienced different haptic conditions in a counterbalanced order. Each haptic condition was tested three times. To ensure a counterbalanced order, the sequences of the three haptic conditions were varied in a way such that each condition was experienced after the other two haptic conditions. The sequences were 1-2-3-1-3-2-1-2-3-1 / 3-1-2-3-2-1-3-1-2-3/2-3-1-2-1-3-2-3-1-2, where 1 = No Haptic (NH), 2 = Haptic Stimulus, and 3 = Tactile Stimulus. For example, the sequence 1-2-3-1-3-2-1-2-3-1 means that the participant experiences No Haptic (NH) first, followed by Haptic Stimulus and Tactile Stimulus, followed by No Haptic, Tactile Stimulus, and Haptic Stimulus, then by No Haptic, Haptic Stimulus, and Tactile Stimulus, and, finally, by No Haptic. The experiment lasted for approximately 24 min in total, including a 10-min break between the crowd conditions.
3.6 Experimental design
The objective of this study was to investigate the contribution of quality or type of haptics to eliciting emotional responses in crowded VR scenarios. We carried out a 2 × 3 within-group factorial experiment with the factors Crowd and Haptics. The Crowd factor has two levels, Casual Crowd and Aggressive Crowd, and refers to the type of social interaction between the participant and a depicted surrounding crowd. Under the Casual Crowd condition, the crowd members casually passed by the participant, whereas under the Aggressive Crowd condition, passers-by occasionally brushed/collided with the participant’s shoulder. The Haptics factor has three levels and refers to the type or quality of haptic feedback that the participants received from passers-by based on the haptic design implementation. This consists of “Tactile Stimulus,” “Haptic Stimulus,” and “No Haptic” (See Section 3.4 for details on haptic device design). The haptic devices were actuated in synchronization with the collision with the crowd in the scene visually. Twenty participants experienced each of these six factors three times, in a counterbalanced order, resulting in 18 repetitions for each participant.
3.7 Participants
The main study was approved by the Institutional Ethics Committee of IIT Madras (IEC/2022-01/MM/03/11). Twenty healthy adult participants aged 20–35 years took part in the experiment (the participants were distinct to those in the pilot study). The participants had correct or corrected vision and were recruited by advertising around the Department of Applied Mechanics at the Indian Institute of Technology Madras. Most of them were comparable across different parameters, such as prior experience of VR, knowledge of computers and programming, and the time they spent on playing video games (Table 1 and Supplementary Figure S4). Overall, the participants had a high level of prior computer and programming knowledge but were unlikely to have used VR before and were not computer game players.
3.8 Response variable questionnaire
After each of the 18 trials (2 crowd scenes × 3 haptic conditions × 3 repetitions), the participants were requested to complete the questionnaire shown in Table 2. The responses were provided on a Likert 1–7 scale, where 7 indicated maximum agreement and 1 indicated least agreement. Here, we were interested to examine whether the factors (haptics conditions and crowd scenes) influenced these responses.
4 Results
First, we provide a descriptive presentation of the results, followed by a formal statistical analysis to either substantiate or refute these descriptive findings.
4.1 Descriptive data analysis
The questions in Table 2 are divided into three categories (apart from identification): 1) haptic assessment (touchcomfort and touchreal), 2) negative emotional responses (nervous, judged, and rejected), and 3) positive emotional responses (comfortable, friendly, and pleasant). The box plots for each of these categories are shown in Figure 5.
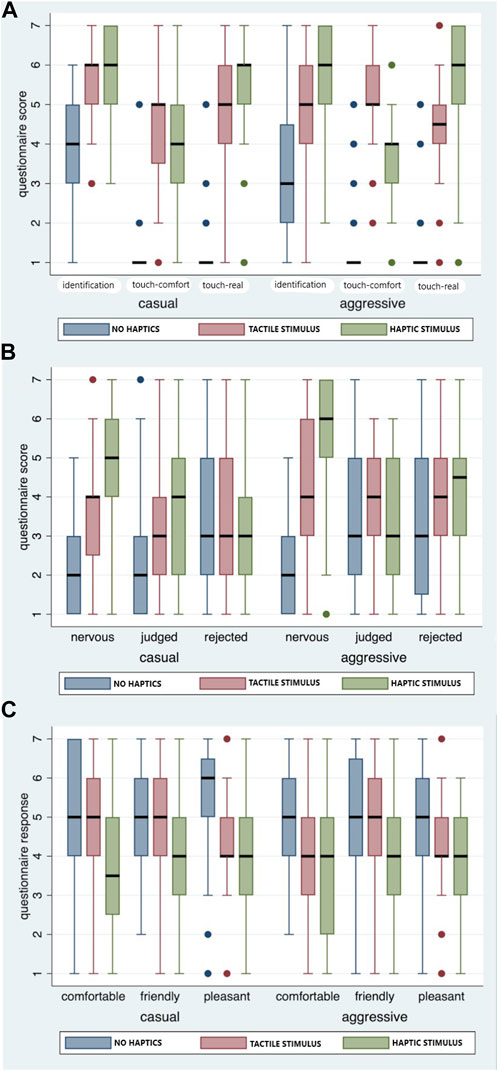
FIGURE 5. Box plots of the response variables by the factors: (A) haptic assessment, (B) negative emotional responses, and (C) positive emotional responses. The thick horizontal lines represent medians, the boxes represent interquartile ranges (IQRs), and the whisker plots run from the maximum (lowest value, lower quartile −1.5 IQR) to the minimum (highest value, upper quartile +1.5 IQR). Points outside this range are displayed separately.
Figure 5A suggests that identification with the crowd increases with Haptic Stimulus, regardless of whether the crowd is Casual or Aggressive. For touchcomfort, Figure 5A shows that Tactile Stimulus is rated to be greater than Haptic Stimulus, implying that participants are more comfortable with Tactile Stimulus than Haptic Stimulus. Under the Casual condition, there is little difference between Tactile Stimulus and Haptic Stimulus. Under the aggressive condition, touchcomfort is rated higher for the Tactile Stimulus (since this concerns comfort, this is not surprising). However, touchreal is greater for the Haptic Stimulus than Tactile Stimulus under both conditions, with the difference being more pronounced in the Aggressive case; the entire interquartile range (IQR) of Haptic Stimulus is greater than the upper quartile of Tactile Stimulus.
Figure 5B shows the negative emotional responses. In the Casual condition, nervous clearly increases with Haptic Stimulus, and this is more pronounced in the Aggressive condition. For judged, there is a little increase with Haptic Stimulus under the Casual condition, although the maximum median score is still only 4, and there is little difference under the Aggressive condition. For rejected, no effect of any type of haptics is observed under the Casual condition, and there is some hint of an increase under the Aggressive condition, but the scores are low.
Figure 5C suggests that the No Haptic condition has the highest positive emotional responses and Haptic Stimulus has the least. Again, this is not surprising because it is presumably more comfortable to have No Haptic on the arm compared to the other conditions.
4.2 Statistical model
The aforementioned descriptive analysis provides some insights into the effects of the touch stimuli, and we discuss the formal statistical inference in this section. Since all the responses are ordinal on a 1–7 scale, we cannot use parametric statistics that assume continuous response variables. Instead, we use ordered logistic regression on each of the nine variables shown in Table 2, which correctly treats the responses as ordinals.
We employ a Bayesian model, which has the advantage of allowing multiple response variables to be examined simultaneously in one overall model, allowing for multiple inferences across various response variables. This also avoids the problem of multiple comparisons, diminishing the validity of significance levels in null hypothesis significance testing. Therefore, a Bayesian logistic regression was performed on all of the nine questionnaire response variables in one overall model that included all nine variables. Moreover, taking into account the fact that each participant had 18 trials, allowing for differences between individuals is essential. The individuals are treated as random effects, and the factors and other variables are treated as fixed effects. For each response, the linear predictor is given in the following form:
Here, Aggressive = 1 for the Aggressive Crowd and 0 for the Casual Crowd.
Tactile = 1 for Tactile Stimulus and 0 otherwise.
Haptic = 1 for Haptic Stimulus and 0 otherwise.
Hence, No Haptic corresponds to Tactile = Haptic = 0.
Sex = 1 for female and 0 for male.
The remaining terms are interaction terms.
The major focus of interest is on the interaction terms, Crowd-Type × Tactile, and Crowd-Type × Haptic, and the other terms are only used to account for variations in the sample.
The formal linear predictor for the model is given as
Here,
The
The prior distribution for each coefficient was taken to be normal (mean = 0, SD = 10), hence, the prior 95% credible intervals were −20 to 20. The logistic model needs cut points, and the prior distributions were also assigned normal values (mean = 0; SD = 10). According to Gelman et al. (2006) and Lemoine and Lemoine (2019), these prior distributions are weakly informative or appropriate probability distributions with high variances that reflect substantial uncertainty.
The analysis was carried out using Stan5 (Stan Development Team, 2011–2019) through the R interface6. A total of 2,000 iterations were used over four cores. All models converged satisfactorily.
4.3 Results of the model
Tables 3–5 show the resulting posterior distributions of the parameters of the model. If a
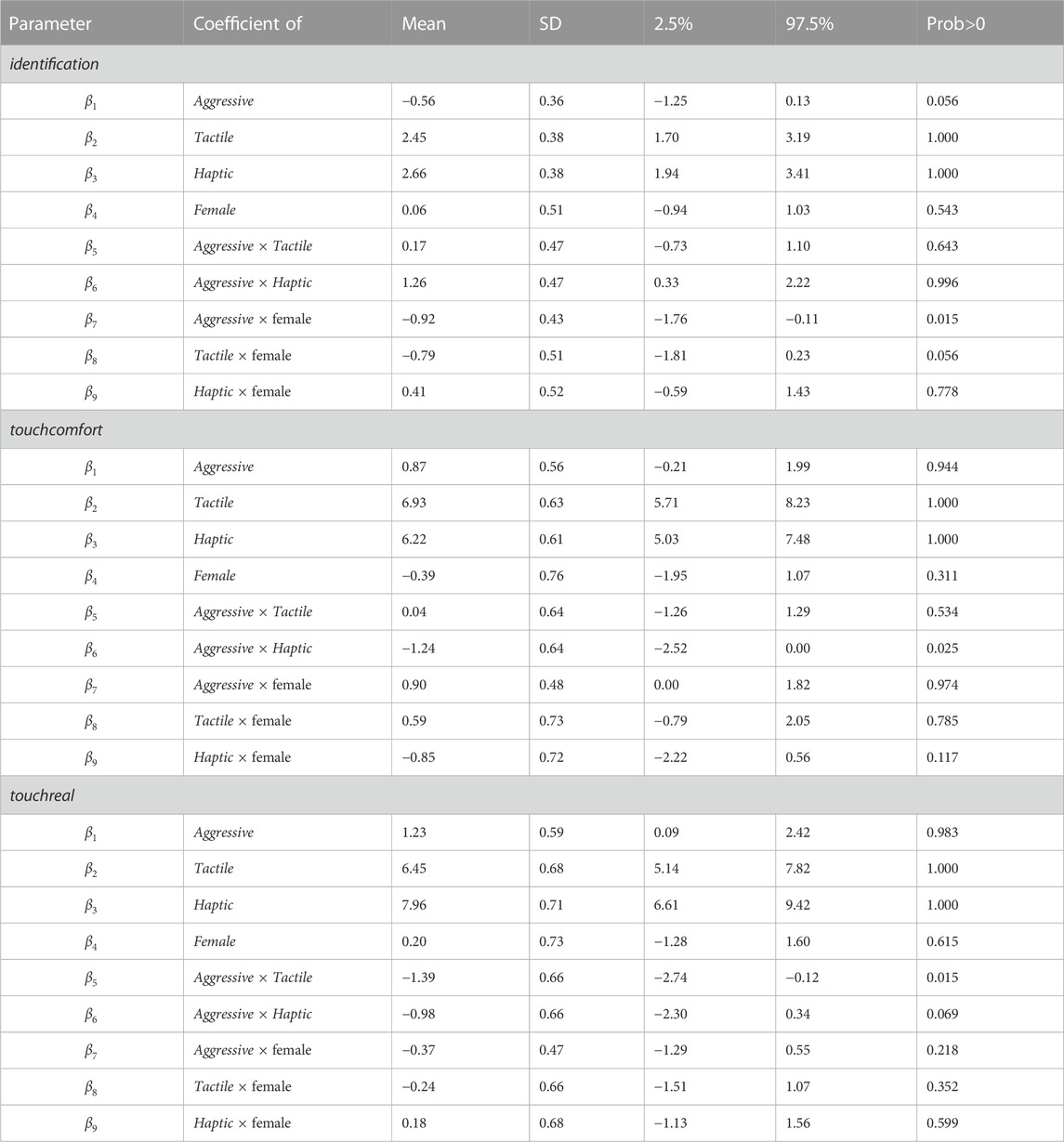
TABLE 3. Posterior distributions of the parameters identification, touchcomfort, and touchreal of the model, showing the mean, standard deviation, and 95% credible interval. Prob>0 is the probability that the corresponding parameter is >0.
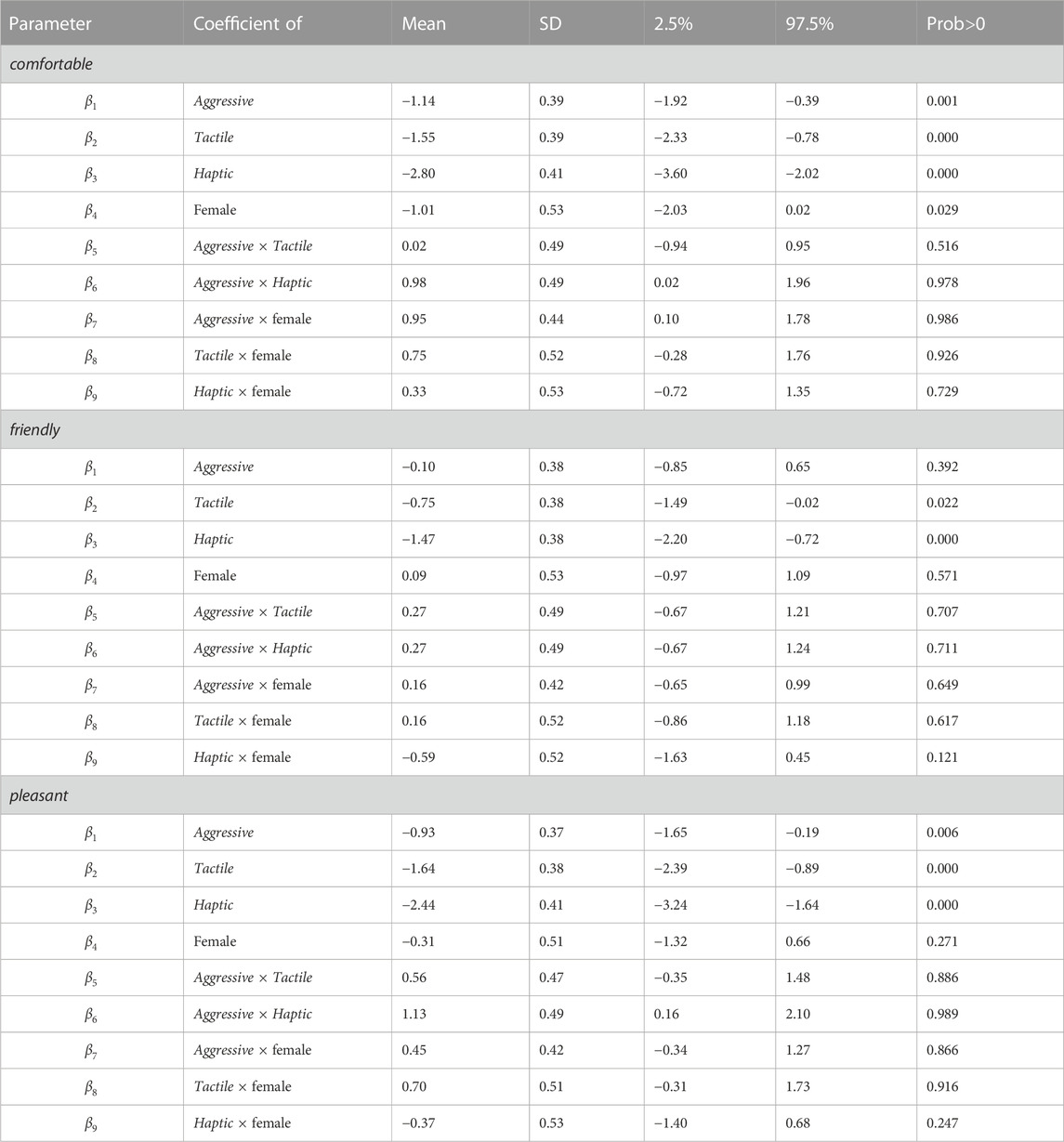
TABLE 4. Posterior distributions of the parameters comfortable, friendly, and pleasant of the model, showing the mean, standard deviation, and 95% credible interval. Prob>0 is the probability that the corresponding parameter is >0.
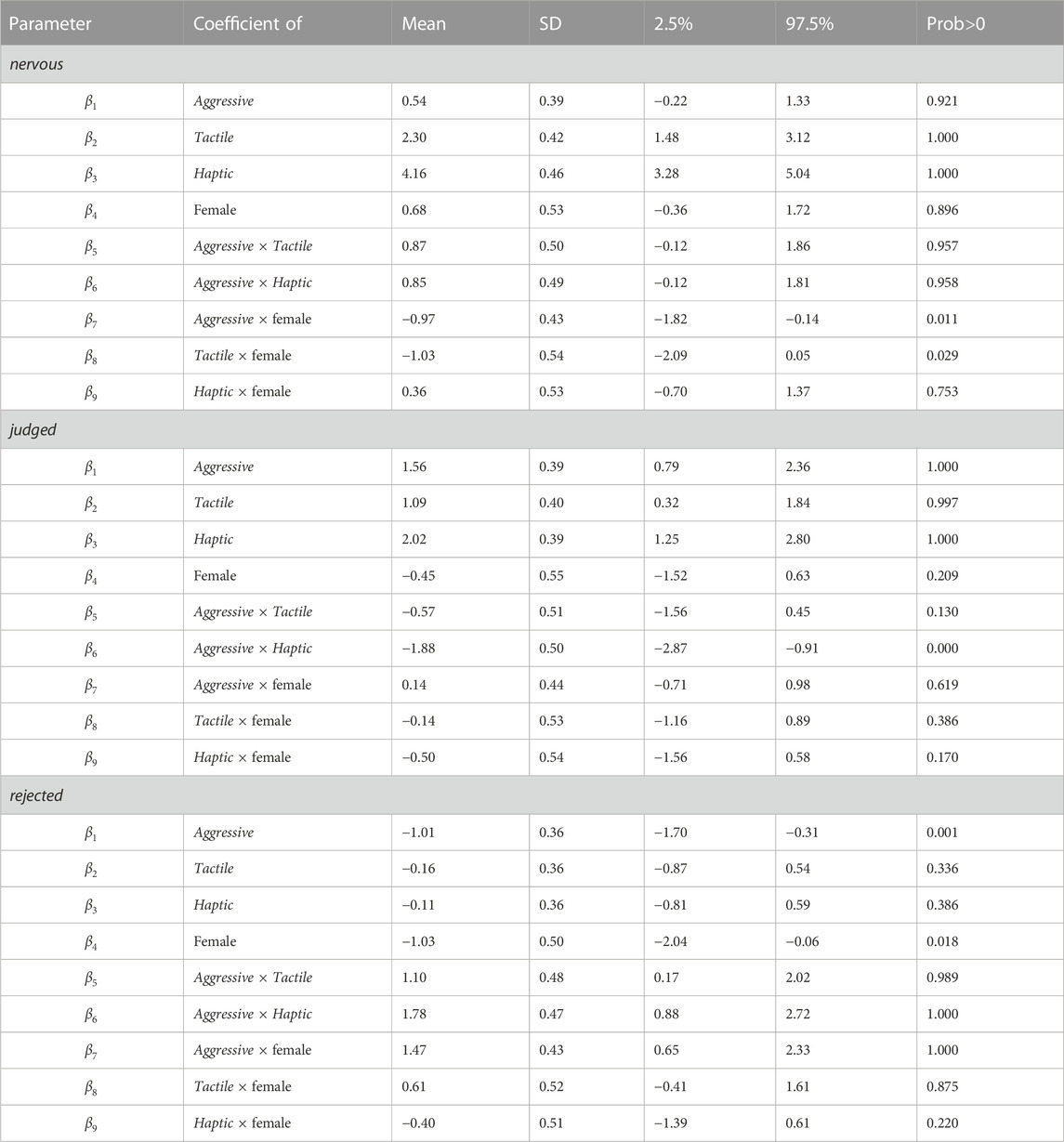
TABLE 5. Posterior distributions of the parameters nervous, judged, and rejected of the model, showing the mean, standard deviation, and 95% credible interval. Prob>0 is the probability that the corresponding parameter is >0.
identification: The interaction term between Aggressive Crowd and Haptic Stimulus has a high probability of being positive (0.996); the mean of the distribution is positive (1.26), and the 95% credible interval does not include 0 (Table 3). Therefore, the evidence supports the finding that identification is positively influenced for Aggressive Crowd with Haptic Stimulus, as shown in Figure 5A.
touchcomfort: Only the main effects of Aggressive Crowd with Tactile Stimulus indicate a positive effect. It is same for the Haptic Stimulus (Table 3).
touchreal: Both Tactile Stimulus and Haptic Stimulus have a positive effect (Table 3).
comfortable: The interaction term for Aggressive Crowd with Tactile Stimulus does not contribute to the effect (the probability of being positive is 0.516) (Table 4). Then, we focus on the main effects. The main effect for Aggressive Crowd is negative (probability = 1–0.001 = 0.999), and the main effect for Tactile Stimulus is negative (probability = 1.000). The Aggressive × Haptic interaction term is positive, but the effect size (mean = 0.98) is outweighed by the main effects of Aggressive (−1.14) and Haptic (−2.80). Hence, according to Figure 5C, comfortable reduces with any type of stimulus.
friendly: Any stimulus is negatively associated with this response; there are no other effects observed (Table 4).
pleasant: Although the interaction terms are positive, these are outweighed by the negative main effects (Table 4). Thus, any type of stimulus reduces the feeling of pleasantness as participants feel unpleasantness with the crowd touch or collision.
nervous: This response (Table 5) increases with Aggressive Crowd for both Tactile Stimulus and Haptic Stimulus, with almost no difference between these two (the credible intervals are almost the same), as shown in Figure 5B.
judged: Aggressive Crowd with Tactile Stimulus shows a negative interaction term, but this is outweighed by the positive main effects. Similarly, for Aggressive Crowd with Haptic Stimulus, this is weaker than the Tactile Stimulus case (Table 5).
rejected: The interaction terms and main effects almost cancel each other out, indicating no particular effect (Table 5).
The main results from the analysis can be summarized as follows:
1. Haptic Stimulus with the Aggressive Crowd has the greatest positive influence on identification.
2. nervous increases with Haptic Stimulus, with no difference between the types of crowds.
3. touchreal increases with Haptic Stimulus.
Overall, the results indicate that there are clear effects of the type of haptics (No Haptic, Tactile Stimulus, and Haptic Stimulus) and sensible interactions with the type of crowd (visual stimuli—Aggressive Crowd and Casual Crowd).
5 Discussion
The main objective of this study was to examine the relationship between the quality or type of haptics and the elicitation of emotions in a VR crowd scenario. We tested three different haptic combinations (No Haptic, Tactile Stimulus, and Haptic Stimulus) reflecting varying types or qualities (perceptual) of haptic feedback, simulating crowd collision within a 360-degree VR setup. We measured the impact of these haptic conditions on emotional responses in two different social contexts (Casual Crowd and Aggressive Crowd) within a simulated virtual environment (positive versus negative) and compared them with a condition without any haptic feedback. Haptic Stimulus was designed to incorporate a combination of tactile cues of the linear actuator and eccentric rotating motor and collision–torque feedback. Tactile Stimulus was based on a combination of tactile cues of the linear actuator and eccentric rotating motor. In the positive social context scenario (Casual Crowd), the number of passers-by was limited, and the participants observed them walking by in a relaxed and casual manner, occasionally colliding with them from the participant’s perspective. In the negative context (Aggressive Crowd), a larger number of passers-by were present, roughly walking past the participant. When examining the emotional responses resulting from crowd collisions facilitated by haptic devices, our findings indicate that both Tactile Stimulus and Haptic Stimulus evoked feelings of nervousness compared to the No Haptics conditions, regardless of the context. However, no other negative emotions, such as being judged or rejected by the crowd, were reported.
Regarding the assessment of haptic devices, our first result shows that both Tactile Stimulus and Haptic Stimulus conditions had a positive effect on enhancing the perceived realism of the crowd interaction experience compared to the No Haptic setup, irrespective of the context of the scenario. This is in line with the previous findings reported in Krogmeier et al. (2019), although it is worth noting that they employed tactile feedback (vest) rather than employing kinesthetic feedback, which was utilized in our study.
Optimal combinations of the type or quality of the haptic input and contextual factors can contribute to an improved sense of presence and social touch. Previous research on the sense of presence suggests that engaging with other agents within a crowd, through physical interactions, such as being pushed or avoiding collisions, or otherwise communicating with them, can enhance the individual’s sense of presence (Stocker et al., 2008). Our results indicate a positive impact on improving the perceived aggression using Haptic Stimulus under the Aggressive Crowd condition, particularly in terms of participants’ identification with the individuals within the scene and their sense of belonging. Ahmed et al. (2016) demonstrated that force-feedback actuators, which exerted pressure on a participant’s palm, were perceived as being more natural and fostered a greater emotional interdependence and a stronger sensation of co-presence compared to vibrotactile touch, regardless of the agent’s emotional expression. However, they did not specifically investigate crowd scenarios. In line with these findings, our study also observed that the Haptic Stimulus condition was consistently rated as being more realistic when experiencing virtual collisions in a VR crowd compared to the No Haptic or Tactile Stimulus condition. This further supports the notion that appropriate haptic stimulation can enhance the overall perception and immersion within virtual crowd interactions.
Our next finding concerns how comfortable the participants found the touch, and Haptic Stimulus was rated second to Tactile Stimulus for this variable. This is in line with the previous findings (Kyriakou et al., 2017). This finding is not surprising as comfort is inversely related to crowd collision. Haptic Stimulus improves touch sensation or aggression in crowds, so Tactile Stimulus is more preferred or regarded as more comfortable than Haptic Stimulus.
Considering emotional responses elicited in participants by the haptic devices, our findings show that the participants felt nervous under both Tactile Stimulus and Haptic Stimulus conditions compared to the No Haptic condition, regardless of the social context; however, no other negative feelings, such as being judged or rejected by the crowd, were elicited.
5.1 Limitations
A major limitation of the developed collision haptic device is its inability to produce successive actuation within a 7-s timeframe. This is due to the minimum time required to reach the maximum angular momentum, which is bottle-necked by the rise time of the flywheel (that is, 7 s). However, this limitation does not affect our study, as the objective was to generate momentary collision, specifically during shoulder collisions. Despite variations in the crowd density and rates of passing-by, we were able to deliver and validate four to five actuations per crowd at an approximate interval of 7 s. This was necessary to achieve our desired impulsive force output of 25 N within a 200-ms window, accurately simulating crowd collisions for the participants. Furthermore, being a wearable haptic device, one of its main drawbacks is that both undesired action forces and desired reaction forces are delivered to the participant’s body sites, resulting in sensations felt by the participants themselves.
It is worth noting that in our study, we only considered collisions occurring at the shoulder, while real-life crowd navigation scenarios may involve collisions with other body parts. However, our haptic devices are designed to be attached to various other possible parts of the body to accommodate different scenarios, although further studies are needed to test participants’ reactions.
In our analysis, we relied on subjective questionnaires, and it is important to acknowledge the limitations of subjective analyses, which may include human errors, biased decisions, and variations in haptic perception capabilities among participants and other factors. In the future, more objective measures based on vital sign assessment, such as heart rate variability, breath rate variability (Soni and Muniyandi, 2019), electro-dermal activity (Amba and Muniyandi, 2017), and eye tracking, are needed to be included to offer better insights into emotional responses in virtual environments through haptics.
Additionally, gender differences should be explored further, as there were only six women out of 20 participants in the study. While both men and women may experience similar levels of sensory pleasantness of touch, their perceptions and attitudes toward touch differ at a higher social level. For example, research has shown that women tend to place more value on touch and express higher levels of comfort, even in interactions with less familiar (or unknown) individuals. Additionally, women tend to feel more at ease with touch directed specifically toward the forearm (Schirmer et al., 2022).
In our study, we employed a 360-degree video-based VR environment instead of 3D model-based simulated virtual environments. However, for future iterations, more immersive model-based virtual environments that incorporate realistic gait motion and facial animation of the virtual crowd would be worth exploring. Additionally, investigating the implementation of multiple haptic cues simultaneously across different parts of the body could provide valuable insights into the sense of presence and participants’ emotional responses. Presence and co-presence are not considered in this study. Tactile and haptic feedback and their impact on the sense of co-presence have been studied previously in interpersonal communication and collaboration in VR settings (Biocca and Harms, 2002; Della Longa et al., 2022). For example, Ahmed et al. (2016) showed that force-feedback actuators are perceived as being more natural and lead to better emotional interdependence and a stronger sensation of co-presence than vibrotactile touch. This study can be extended in the future to address the relationship between the intensity of the haptic devices and co-presence.
6 Conclusion
The main aim of this study was to investigate the relationship between the type or quality of the haptic input or feedback and emotion in VR crowd scenarios using two different affective crowded VR scenarios (Casual Crowd and Aggressive Crowd) and, more specifically, the influence of the intensity of haptics on the perception of positive and negative emotions. We designed and conducted trials involving 20 healthy subjects and analyzed the experimental data using descriptive data analysis and statistical data analysis. The results from both analyses show that the type of haptics input has a clear effect on perceiving realism, comfort, and negative and positive emotions. Overall, the results indicate the effects of the quality or type of haptics and sensible interactions with the type of crowd. Therefore, from our study, we conclude that Haptic Stimulus, comprising both tactile and torque feedback, has been found to influence the emotional responses of people in the virtual crowd. The outcome of this study could be used in designing better virtual reality systems for future emotion-rendering engines.
Data availability statement
The raw data supporting the conclusion of this article will be made available by the authors, without undue reservation.
Ethics statement
The studies involving humans were approved by the Institutional Ethics Committee of IIT Madras (IEC/2022-01/MM/03/11). The studies were conducted in accordance with the local legislation and institutional requirements. The participants provided their written informed consent to participate in this study. Written informed consent was obtained from the individual(s) for the publication of any potentially identifiable images or data included in this article.
Author contributions
MM, MS, DB, and RKV contributed to the study design. RKV implemented the scenario, carried out the experiment, and collected the data. MS carried out the statistical analysis. MM, MS, DB, and RKV wrote the paper. DB, MS, and MV supervised the study. MS and DB funded the study. All authors contributed to the article and approved the submitted version.
Funding
This project was supported by the European Research Council (ERC) Advanced Grant MoTIVE (#742989) and the Tamkeen NYU Abu Dhabi Funding Agency (#76 71220 ADHPG VP211). MM and RKV were supported by Institutes of Eminence funded by the Government of India.
Conflict of interest
The authors declare that the research was conducted in the absence of any commercial or financial relationships that could be construed as a potential conflict of interest.
Publisher’s note
All claims expressed in this article are solely those of the authors and do not necessarily represent those of their affiliated organizations, or those of the publisher, the editors, and the reviewers. Any product that may be evaluated in this article, or claim that may be made by its manufacturer, is not guaranteed or endorsed by the publisher.
Supplementary material
The Supplementary Material for this article can be found online at: https://www.frontiersin.org/articles/10.3389/frvir.2023.1242587/full#supplementary-material
Footnotes
1Aggressive walking crowd: https://youtu.be/knP2WRXVOH0
2Casual walking crowd: https://youtu.be/Stpl2ufQi_s
3https://www.insta360.com/es/product/insta360-pro
6https://mc-stan.org/users/interfaces/rstan
References
Adilkhanov, A., Rubagotti, M., and Kappassov, Z. (2022). Haptic devices: wearability-based taxonomy and literature review. IEEE Access 10, 91923–91947. doi:10.1109/ACCESS.2022.3202986
Ahmed, I., Harjunen, V., Jacucci, G., Hoggan, E., Ravaja, N., and Spapé, M. M. (2016). “Reach out and touch me: effects of four distinct haptic technologies on affective touch in virtual reality,” in ICMI 2016 - Proc. 18th ACM Int. Conf. Multimodal Interact., Tokyo Japan, November, 2016, 341–348. doi:10.1145/2993148.2993171
Amba, R. R., and Muniyandi, M. (2017). Quantification of electrodermal activity variation across human fingers: toward a scientific basis of mudras. Int. J. Yoga - Philos. Psychol. Parapsychol. 5, 48. doi:10.4103/IJNY.IJOYPPP_4_17
Ando, H., Obana, K., Sugimoto, M., and Maeda, T. (2002). A wearable force display based on brake change in angular momentum. Available at: http://www.ic-at.org/papers/02016.pdf (Accessed June 14, 2023).
Beacco, A., Oliva, R., Cabreira, C., Gallego, J., and Slater, M. (2021). “Disturbance and plausibility in a virtual rock concert: a pilot study,” in Proc. - 2021 IEEE Conf. Virtual Real. 3D User Interfaces, VR 2021, Lisboa, Portugal, March, 2021, 538–545. doi:10.1109/VR50410.2021.00078
Berton, F., Grzeskowiak, F., Bonneau, A., Jovane, A., Aggravi, M., Hoyet, L., et al. (2022). Crowd navigation in VR: exploring haptic rendering of collisions. IEEE Trans. Vis. Comput. Graph. 28, 2589–2601. doi:10.1109/TVCG.2020.3041341
Biocca, F., and Harms, C. (2002). Defining and measuring social presence - contribution to the networked minds theory and measure. Int. Work. Presence, 1–36.
Cui, D., Kao, D., and Mousas, C. (2021). Toward understanding embodied human-virtual character interaction through virtual and tactile hugging. Comput. Animat. Virtual Worlds 32, e2009. doi:10.1002/CAV.2009
Della Longa, L., Valori, I., and Farroni, T. (2022). Interpersonal affective touch in a virtual world: feeling the social presence of others to overcome loneliness. Front. Psychol. 12, 795283. doi:10.3389/fpsyg.2021.795283
Dickinson, P., Gerling, K., Hicks, K., Murray, J., Shearer, J., and Greenwood, J. (2019). Virtual reality crowd simulation: effects of agent density on user experience and behaviour. Virtual Real 23, 19–32. doi:10.1007/s10055-018-0365-0
Endo, T., Kawasaki, H., Mouri, T., Ishigure, Y., Shimomura, H., Matsumura, M., et al. (2011). Five-fingered haptic interface robot: HIRO III. IEEE Trans. Haptics 4, 14–27. doi:10.1109/TOH.2010.62
Gelman, A., Jakulin, A., Grazia Pittau, M., and Su, Y.-S. (2006). A default prior distribution for logistic and other regression models. Available at: https://stat.columbia.edu/(Accessed June 15, 2023).
Giri, G. S., Maddahi, Y., and Zareinia, K. (2021). An application-based review of haptics technology. Robot 10, 29. doi:10.3390/ROBOTICS10010029
Goldenberg, A., Weisz, E., Sweeny, T. D., Cikara, M., and Gross, J. J. (2021). The crowd-emotion-amplification effect. Psychol. Sci. 32, 437–450. doi:10.1177/0956797620970561
Hoppe, M., Rossmy, B., Neumann, D. P., Streuber, S., Schmidt, A., and MacHulla, T. K. (2020). A human touch: social touch increases the perceived human-likeness of agents in virtual reality. Conf. Hum. Factors Comput. Syst. - Proc. 20. doi:10.1145/3313831.3376719
Huisman, G. (2017). Social touch technology: a survey of haptic technology for social touch. IEEE Trans. Haptics 10, 391–408. doi:10.1109/TOH.2017.2650221
Koilias, A., Mousas, C., and Anagnostopoulos, C. N. (2020a). I feel a moving crowd surrounds me: exploring tactile feedback during immersive walking in a virtual crowd. Comput. Animat. Virtual Worlds 31, e1963. doi:10.1002/CAV.1963
Koilias, A., Nelson, M. G., Anagnostopoulos, C. N., and Mousas, C. (2020b). Immersive walking in a virtual crowd: the effects of the density, speed, and direction of a virtual crowd on human movement behavior. Comput. Animat. Virtual Worlds 31, e1928. doi:10.1002/CAV.1928
Kramer, A. D. I., Guillory, J. E., and Hancock, J. T. (2014). Experimental evidence of massive-scale emotional contagion through social networks. Proc. Natl. Acad. Sci. 111, 8788–8790. doi:10.1073/PNAS.1320040111
Krogmeier, C., Mousas, C., and Whittinghill, D. (2019). Human–virtual character interaction: toward understanding the influence of haptic feedback. Comput. Animat. Virtual Worlds 30, e1883. doi:10.1002/CAV.1883
Kyriakou, M., Pan, X., and Chrysanthou, Y. (2017). Interaction with virtual crowd in Immersive and semi-Immersive Virtual Reality systems. Comput. Animat. Virtual Worlds 28. doi:10.1002/CAV.1729
Lemoine, N. P., and Lemoine, N. P. (2019). Moving beyond noninformative priors: why and how to choose weakly informative priors in Bayesian analyses. Oikos 128, 912–928. doi:10.1111/OIK.05985
Loomis, J., and Lederman, S. (2003). Tactual perception. Cambridge, United Kingdom: Cambridge University Press.
Massie, T. H., and Salisbury, J. K. (1994). “The Phantom Haptic Interface: A Device for Probing Virtual Objects,” in Proceedings of the ASME Winter Annual Meeting, Symposium on Haptic Interfaces for Virtual Environment and Teleoperator Systems, Chicago, November 1994, 295–300.
Monica, R., and Aleotti, J. (2023). Improving virtual reality navigation tasks using a haptic vest and upper body tracking. Displays 78, 102417. doi:10.1016/J.DISPLA.2023.102417
Murtagh, E. M., Mair, J. L., Aguiar, E., Tudor-Locke, C., and Murphy, M. H. (2021). Outdoor walking speeds of apparently healthy adults: a systematic review and meta-analysis. Sport. Med. 51, 125–141. doi:10.1007/s40279-020-01351-3
Nagai, K., Akahane, K., Tanoue, S., and Sato, M. (2015). “Wearable 6-DoF wrist haptic device “sPIDAR-W.”,” in SIGGRAPH Asia 2015 Haptic Media Contents Des. SA 2015, Kobe, Japan, November, 2015. doi:10.1145/2818384.2818403
Nunez, C. M., Huerta, B. N., Okamura, A. M., and Culbertson, H. (2020). “Investigating social haptic illusions for tactile stroking (SHIFTS),” in IEEE Haptics Symp. HAPTICS 2020, Crystal City, VA, USA, March, 2020, 629–636. doi:10.1109/HAPTICS45997.2020.RAS.HAP20.35.F631355D
Romanishin, J. W., Gilpin, K., Claici, S., and Rus, D. (2015). “3D M-Blocks: self-reconfiguring robots capable of locomotion via pivoting in three dimensions,” in Proc. - IEEE Int. Conf. Robot. Autom, Seattle, WA, USA, June, 2015, 1925–1932. doi:10.1109/ICRA.2015.7139450
Schirmer, A., Cham, C., Zhao, Z., Lai, O., Lo, C., and Croy, I. (2022). Understanding sex differences in affective touch: sensory pleasantness, social comfort, and precursive experiences. Physiol. Behav. 250, 113797. doi:10.1016/J.PHYSBEH.2022.113797
Škola, F., Rizvić, S., Cozza, M., Barbieri, L., Bruno, F., Skarlatos, D., et al. (2020). Virtual reality with 360-video storytelling in cultural heritage: study of presence, engagement, and immersion. Sensors 20, 5851. doi:10.3390/S20205851
Slater, M., Cabriera, C., Senel, G., Banakou, D., Beacco, A., Oliva, R., et al. (2022). The sentiment of a virtual rock concert. Virtual Real 1, 651–675. doi:10.1007/s10055-022-00685-9
Somarathna, R., Bednarz, T., and Mohammadi, G. (2022). Virtual reality for emotion elicitation – a review. IEEE Trans. Affect. Comput., 1–21. doi:10.1109/TAFFC.2022.3181053
Soni, R., and Muniyandi, M. (2019). Breath rate variability: a novel measure to study the meditation effects. Int. J. Yoga 12, 45. doi:10.4103/IJOY.IJOY_27_17
Srinivasan, M. A., and Basdogan, C. (1997). Haptics in virtual environments: taxonomy, research status, and challenges. Comput. Graph. 21, 393–404. doi:10.1016/S0097-8493(97)00030-7
Stocker, C., Allbeck, J. M., Badler, N. I., Pelechano, N., Allbeck, J., and Badler, N. (2008). “Being a part of the crowd: towards validating VR crowds using presence,” in 7th International Joint Conference on Autonomous Agents and Multiagent Systems (AAMAS 2008), Estoril, Portugal, May, 2008, 136.1
Todd, S. L., Young, A. B., Anderson, L. S., Connell, T. S. O., and Breunig, M. (2008). Sense of place in outdoor-pursuits trip groups. Peoceedings 2008 Northeast. Recreat. Res. Symp., 72–80.
Wysiadecki, G., Polguj, M., Krasucki, K., Zytkowski, A., Śmigielski, J., Topol, M., et al. (2014). Morphology and a proposed model of innervation of the human deltoid muscle: a pilot study. Folia Morphol. 73, 216–223. doi:10.5603/FM.2014.0032
Keywords: virtual reality, haptic feedback, haptic display, 360-degree video, virtual collisions, virtual crowd, simulating emotions, multimodal virtual environment
Citation: Venkatesan RK, Banakou D, Slater M and M. M (2023) Haptic feedback in a virtual crowd scenario improves the emotional response. Front. Virtual Real. 4:1242587. doi: 10.3389/frvir.2023.1242587
Received: 19 June 2023; Accepted: 13 November 2023;
Published: 28 November 2023.
Edited by:
Jeremy Cooperstock, McGill University, CanadaReviewed by:
Vincent Levesque, École de technologie supérieure (ÉTS), CanadaHojin Lee, Electronics and Telecommunications Research Institute (ETRI), Republic of Korea
Copyright © 2023 Venkatesan, Banakou, Slater and M.. This is an open-access article distributed under the terms of the Creative Commons Attribution License (CC BY). The use, distribution or reproduction in other forums is permitted, provided the original author(s) and the copyright owner(s) are credited and that the original publication in this journal is cited, in accordance with accepted academic practice. No use, distribution or reproduction is permitted which does not comply with these terms.
*Correspondence: Domna Banakou, ZG9tbmEuYmFuYWtvdUBueXUuZWR1