- 1Sensory Processes Research Laboratory, School of Optometry and Vision Science, University of New South Wales, Kensington, NSW, Australia
- 2Bandicoot Imaging Sciences, Sydney, NSW, Australia
Improving the digital presentation of fabrics enhances the online shopping experience and, in turn, reduces textile waste. In this study, we examined how the manipulation of simple surface reflectance models can bias the perception of fabric properties simulated online in a web browser. We showed that motion and three-dimensional (3D) folds (i.e., rumple) influence the perception of sheen for different fabric types (cotton knit and satin). Also, we found complex interactions between these parameters in their effects on perceived sheen and perceived color saturation. Moreover, we showed that changing the level of specular roughness significantly influences visual perception of sheen, color and lightness, which in turn, can categorically alter perceptual judgments of material type. In contrast to visual attributes, specular roughness did not influence visually perceived tactile characteristics of digital fabrics (thickness and stretch). The knowledge gained about perceptual biases of digital fabrics from this study will inform future considerations for optimizing the fidelity of textiles depicted in digital commerce.
Introduction
Shopping is an essential human activity that has not been able to resist the convenience afforded by the industrial transition to online internet platforms (Xiong et al., 2022). Despite the explosion in consumer dependence on online shopping platforms following the onset of the global pandemic (García-Salirrosas and Acevedo-Duque, 2022; García-Salirrosas et al., 2022), the purchase of more personalized products like apparel may not have enjoyed the full potential of an online experience. Conventional photographic samples of apparel displayed on retail websites are often limited in photographic presentation provided by a finite set of static views. Poor fidelity in visual representation of real fabrics can set up inaccurate expectations that result in returns and the already excessive global generation of textile waste (Kasavan et al., 2021) with Australia contributing 304,200 tons in 2018–2019 alone (Australian Government, 2021). To improve visual representations and reduce waste, real-time 3D rendering of surfaces and materials in a web browser has been adopted by many retailers. However, limited research has considered how rendering of different surface and reflectance properties may affect perception of fabrics presented on generic computer displays. Most psychological studies focusing on the online apparel industry have been limited to website presentation (Devderea and Toader, 2018; Wiranata and Hananto, 2020) and the quality of information available on products (Aldás-Manzano et al., 2011; Vasić et al., 2019). In this study, we assess how the visually simulated representation of fabrics on computer displays might influence how the quality of information concerning material properties of real-world fabrics is (mis-) perceived when viewed on generic computer displays commonly used for online shopping.
Image formation depends on complex interactions between illumination, surface and material properties, as well as viewing direction (Honson et al., 2020; Kiyokawa et al., 2023). An idealized bi-directional reflectance distribution function (BRDF) can be used to model this complex process of image formation (Nicodemus, 1965). The BRDF models the behavior or opaque surfaces that generate diffuse and specular shading in images (see Figure 1). Diffuse shading is viewpoint-independent shading generated by Lambertian reflectance and is dependent on the orientation of surface normals relative to the light source. In comparison, specular highlights are viewpoint-dependent and determined by the orientation of surface normals relative to viewing direction and the angle of incident illumination. Whereas diffuse shading carries information about 3D shape and matte surface color, specular reflections carry information about 3D surface shape and gloss, independent of color attributes (Fleming et al., 2003; Fleming et al., 2004).
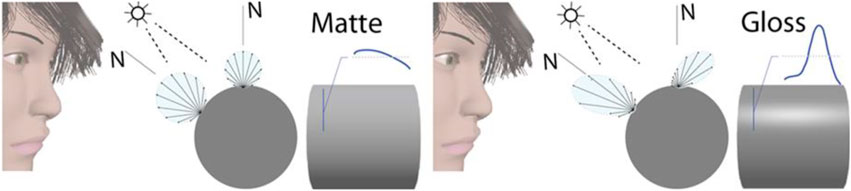
FIGURE 1. Surface optics involved in diffuse reflectance (left) and specular reflectance (right). The amount of photometric energy reflected diffusely by a surface depends on Lambertian reflectance (i.e., the orientation of surface normals, N, relative to the light source). The diffuse profile of a surface generates the experience of a surface’s matte 3D shape. Matte surfaces (left) reflect light symmetrically around surface normals. Glossy surfaces (right) reflect light disproportionately towards the side of surface normals oriented away from the primary light source. The width of the specular lobe determines the contrast and sharpness of specular highlights.
While the visual system recovers surface information from diffuse and specular image components, humans appear to perceptually decompose images into layered representations (intrinsic images) of their environmental causes (Barrow et al., 1978; Anderson and Marlow, 2022). According to this view, the perception of gloss and color of 3D surfaces depends on the visual system’s ability to separate diffuse and specular components into representations of 3D shape, color and gloss. Due to the co-dependence of different percepts on multiple image components (e.g., perceived 3D shape depending on both specular and diffuse shading), understanding how perception of surface and material properties is decomposed onto perceptual “layers” is formally challenging.
Despite the challenges in understanding material perception, some key constraints have been identified in previous literature. In the case of perceived gloss, Anderson and Kim (2009) proposed that photogeometric image constraints (e.g., the orientation of sharp specular edges relative to adjacent [diffuse] image shading) play a critical role in the perceptual attribution of image structure to environmental causes. Evidence suggests that image motion may also help improve the visual system’s ability to segment relevant image structure for material perception. Motion cues generated by optic flow might enable better perceptual differentiation between diffuse and specular shading (Sakano and Ando, 2010). For example, Hartung and Kersten (2002) showed that the pattern of visual motion generated by diffuse and specular reflectance is different, and this difference could be used to perceive the glossiness of a rotating teapot. A subsequent study proposed the distinction between matte and glossy surfaces depends on differences in the velocity field between these materials (Doerschner et al., 2011). In that study, optic flow attributed to specular reflectance was shown to have a different velocity profile relative to the velocities of the underlying diffuse shading as the surface rotates relative to the observer and light field. These differential motion velocities generated by specular and diffuse reflectance appear to enhance our perceptual ability to estimate the material properties of objects that move relative to observer in real-world scenes.
Although the identification of specular reflections is critical for the perception of gloss, failure to classify these highlights correctly may bias the perception of color attributes. Honson et al. (2020) found perceptual interactions on surface color and gloss perception across changes in simulated surface shape, roughness, and surface pose relative to illumination. Increasing specular roughness was found to reduce perceived glossiness and color saturation but increased perceived lightness. Differences in the visual system’s ability to perceptually separate diffuse and specular shading might account for this inverse relationship between perceived color value (i.e., lightness) and glossiness. Based on this view, perceptual segmentation of specular content should be optimal when specular highlights are sharp. Following any increase in specular roughness, proportional amounts of specular content may be misattributed to Lambertian reflectance (and diffuse shading), which may influence the apparent color saturation and lightness of 3D surfaces (Isherwood et al., 2021).
The primary theoretical motivation of this study was to evaluate whether motion and 3D geometry (i.e., fabric rumple) affect the perception of material properties. For the purpose of this study, we simulated the behaviour of textile fabrics that are commonly used in everyday human interaction. We rendered images based on 3D scans of genuine fabrics (satin and knitted cloth), which allowed us to assess the influence of different parameters of motion, 3D geometry, as well as diffuse and specular shading on material appearance. Psychophysical tasks were used to measure perceived sheen, lightness and color saturation of fabrics rendered with different chroma (blue versus red), 3D rumple (rumpled versus flat) and motion characteristics (animated image sequences versus still images). It is extremely difficult to physically match different fabric types in color attributes, so the physical material samples we scanned were selected to be close in saturation across the different fabric types and colors used. Although fabrics varied in lightness, this allowed us to ascertain generalizability in any effects of motion and rumple across different fabric types and colors. Based on the findings of a recent study (Isherwood et al., 2021), we expected to find complex interactions between shape and chroma on perceived material properties. Motion of fabrics was expected to improve recognition of specular reflections and facilitate the perception of fabric sheen and color. We also examined how varying specular reflectance can bias perceptual classifications of digital fabrics.
Materials and method
Participants
A total of 35 adult first-year vision science students were recruited who were non-experts in the textile industry. All participants had normal or corrected-to-normal visual acuity and color vision. All procedures adhered to the ethical principles outlined in the Declaration of Helsinki and were approved by the Human Research Ethics Advisory Panel at the University of New South Wales. Written informed consent was obtained from the participants prior to the beginning of the experiment.
Stimuli
Stimuli were real-time rendered images or animations that were based on physical samples of fabric materials. Physical samples (fabrics) were scanned by taking a series of at least 25 flash photographs from multiple angles with a DSLR camera (Figure 2), and Bandicoot proprietary software was used to create a graphical model of each material from these photographs. Images were rendered using the open-source graphical software platform known as Bivot (https://github.com/bandicoot-imaging-sciences/bivot) which was produced by Bandicoot Imaging Sciences (https://www.bandicootimaging.com.au). This software is a web-based platform that is capable of simulating surface reflectance using a customized BRDF based on the Disney Principled BRDF (Burley, Brent, and Walt Disney Animation Studios, 2012). The custom model was used to combine diffuse and specular reflectance models to simulate the appearance of natural opaque surfaces with spatially varying 3D shape, chromaticity and gloss.
In an initial study, a total of 4 unique material samples were rendered based on samples spanning approximately 110 × 70 mm in size and viewed frontally. There were two fabric types, with two samples of satins and two of knits. For each fabric type, two different hues (blue and red) were sampled. Hues were not identical across fabric types due to manufacturing constraints, but the hues allowed us to explore how any effect of hue might vary within each material class. We also systematically varied specular roughness over 2 levels and simulated surface motion (moving or statically presented). Hence, there were 16 conditions in total (2 fabric types x 2 hues x 2 motion states x 2 rumple levels). Static samples of the fabrics presented are shown in Figure 3.
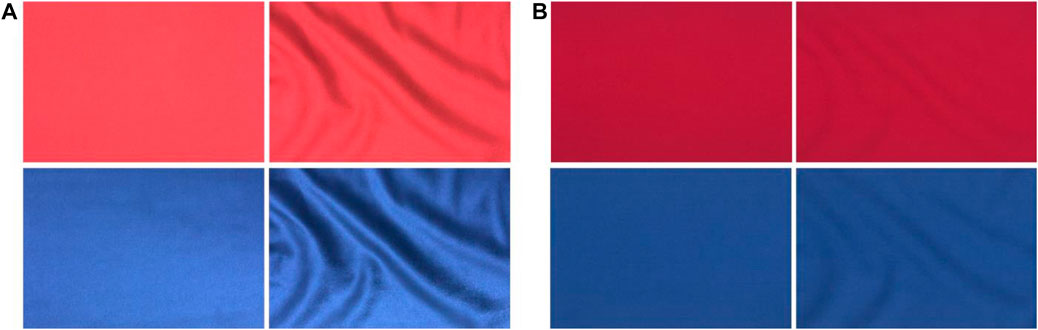
FIGURE 3. Rendered images of scanned samples varying in fabric types of satin (A) and knit (B). For each fabric type, and physical base color was either red or blue with each sample artificially rendered to be flat (left pairs) or generate digital folds (right pairs).
Animated renders were generated with the camera and lighting moving in a circle above the simulated material. The simulated vantage point was always approximately 0.3 m in front of the material across all conditions. A point light source was simulated to ensure that glossy details in the materials could be easily discerned by the viewer. The light source was offset by 40° relative to the viewing axis and simulated at approximately 1 m in front of the center of each material sample, with the simulated exposure adjusted to optimise the dynamic range of the luminance histogram of the image generated. This lighting configuration was used to produce dynamic renderings of surfaces that were similar to real-world photographs of the same surfaces. The simulated surfaces were slanted by approximately 9° in depth with the direction of slant in depth systematically rotating around the visual axis over a 360-degree range in counterphase to the dynamic changes in lighting direction. The resolution of the rendered images was 800 x 600 PPI (pixels per inch). Real-time rendered movie sequences were then able to be presented in a standard web browser using HTML5 and JavaScript.
In a follow-up study, we focussed on the moving blue fabrics with added rumple (i.e., digital folds). This was undertaken to examine the effects of specular roughness and amplitude on perceptual outcome measures. We systematically varied specular roughness over three levels (including 20%, 50%, and 80% of the maximum amount of specular roughness) and specular amplitude over three levels (0.050, 0.125, 0.200). Hence, there were 18 conditions in total (2 base fabric types x 3 specular amplitudes x 3 specular roughnesses). Figure 4 shows samples of the nine statically presented stimuli used in this follow-up study that was performed to assess how specular amplitude and roughness affect material judgments.
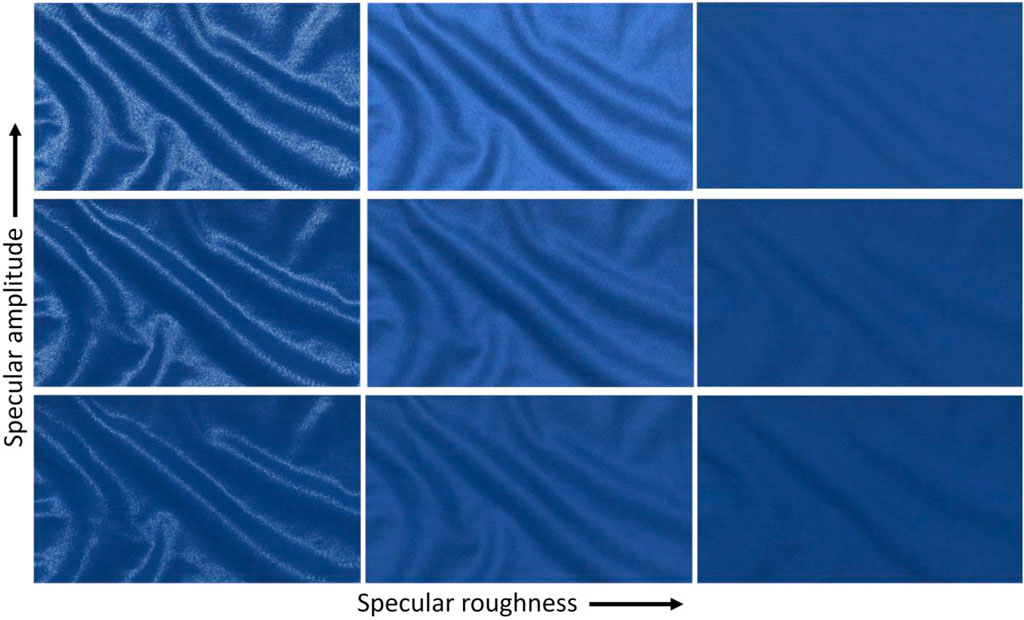
FIGURE 4. Rendered images of scanned samples of blue knit simulated to have digital folds. Samples were always moving onscreen, but with the specular component systematically varied in amplitude (between rows) and roughness (left to right). The base 3D geometry and diffuse texture were identical across all samples.
Procedure
In all studies, participants engaged online in this research. Before participating, the researcher explained the instructions to participants for the study and asked the participants to score perceptual attributes using rating scales. Participants accessed the experiment through a web link using their own desktop computer device. This link initiated the JavaScript application on their computer to present a series of pages in randomized order. Prior to commencing the experiment, participants altered the size of the webpage in their browser by zooming in and out using their mouse wheel and the [CTRL] key on their keyboard to set the size of a template image to match the size of a physical credit card held against the display. A set of training images was offered to the participants to ensure that they were familiar enough with the requirements of the rating task. After feeling confident in understanding the task, participants clicked a button in their browser to commence their participation by viewing the series of pages that each displayed a simulated fabric image in real-time on their display.
Participants pressed the [Next >>] button after making each set of ratings out of 10, advancing them to the subsequent stimulus trial in the series. Stimuli were presented on the display in random order. Participants were asked to complete entries available in a row of response fields shown below the stimulus on each page. In the initial study with different colored fabrics, a total of nine participants judged:
1. Sheen (0 = completely matte vs. 10 = reflects light like a mirror)
2. Saturation (0 = grey/colorless vs. 10 = fully saturated in color)
3. Lightness (0 = Black vs. 10 = White)
In the follow-up study, where we varied specular roughness for the blue fabrics, a total of 26 participants followed the same procedure above, but additional questionnaire items were required to be completed using response fields below each of the blue rumpled digital fabrics simulated on each page. Participants had to also provide the following judgments:
4. Thickness (0 = 1/6 diam. Of a human hair or 50 denier vs. 10 = the thickest canvas or 500 denier)
5. Stretch (0 = cannot be stretched vs. 10 = highly elastic like the most stretchable Lycra or Spandex)
6. Material composition (Most like: Cotton, Polyester, Satin, Wool, Vinyl, Leather, Velvet, or Linen)
Data analysis
For statistical analysis, an R script was used to perform repeated-measures ANOVAs to examine the effects of rumple and motion on the perceived sheen, lightness, and color saturation (and perceived thickness and stretch in the follow-up study). Moreover, intercorrelations between outcome measures were performed to identify any linear relationships between perceptual experiences.
Results
Figure 5 shows the effect of fabric type, fabric color, digital folds, and motion on the perception of sheen. A four-way repeated-measures ANOVA found a significant main effect of fabric type on perceived sheen [F (1,8) = 61.44, p < 0.001]. There was no main effect of fabric color on perceived sheen [F (1,8) = 0.02, p = 0.886]. There was a significant main effect of rumple on perceived sheen [F (1,8) = 11.98, p < 0.01]. There was also a significant main effect of motion on perceived sheen [F (1,8) = 16.08, p < 0.005]. The only interaction effect that was found to be significant was a two-way interaction between fabric type and rumple on perceived sheen [F (1,8) = 19.10, p < 0.005]. All other two-way and three-way interactions were not significant.
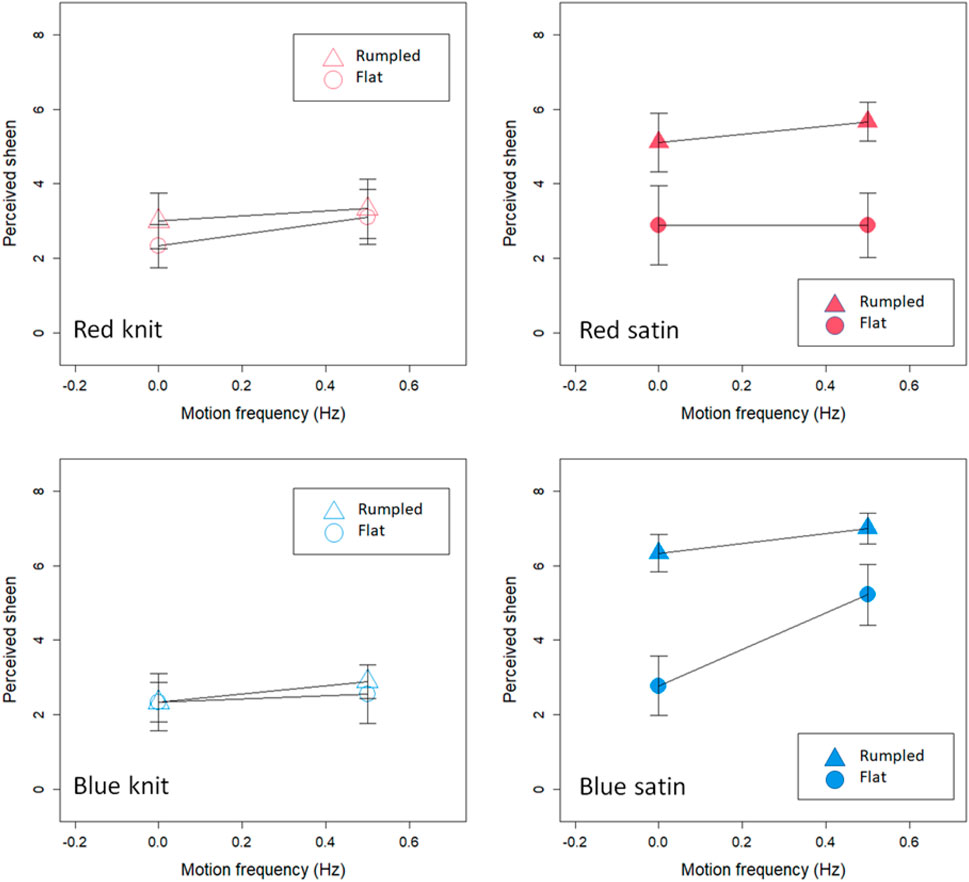
FIGURE 5. Means and standard errors for perceived sheen plotted as a function of motion frequency for red knit (upper left), red satin (upper right), blue knit (lower left) and blue satin (lower right).
These results suggest that the perception of sheen was improved with the introduction of digital folds and motion cues, compared with static presentations of fabrics. The improvement in perceived sheen was overall greater for the satin fabrics; digital folds introduced into these fabrics generated greater curvature that increased the sharpness and overall contrast of specular highlights (increasing perceived sheen). However, motion cues generally increased perceived sheen of all samples, especially the blue satin fabrics that were flat. It would appear that motion and rumple provide synergistic information for perceptually processing specular highlights in the inference of sheen (supported by the significant interaction between rumple and motion). Indeed, the increase in perceived sheen by motion was greater for the flat presentations of blue satin, but the increase in sheen was still lower than the perceived sheen achieved when digital folds and motion cues were combined. This suggests that digital rumple influenced the perception of sheen more than motion. This dominance of rumple over motion for influencing perceived sheen was also observed with the red satin, where there was limited effect of motion on perceived sheen (which could be attributed to the lower overall perceived sheen generated by this fabric color).
Figure 6 shows the effect of fabric type, fabric color, digital folds, and motion on the perception of color saturation. A four-way repeated-measures ANOVA found a significant main effect of rumple on perceived saturation [F (1,8) = 6.006, p < 0.05]. There was no main effect of fabric color on perceived saturation [F (1,8) = 0.65, p = 0.443]. There was also no main effect of fabric type [F (1,8) = 0.653, p = 0.442] nor of motion [F (1,8) = 0.324, p = 0.585] on perceived saturation. Furthermore, no significant two-way and three-way interaction effects were found. These results suggest that similar to perceived sheen, addition of fabric rumple increased the perceived color saturation of fabrics. The result also confirms that perceived saturation was perceptually matched across different fabric types and colors.
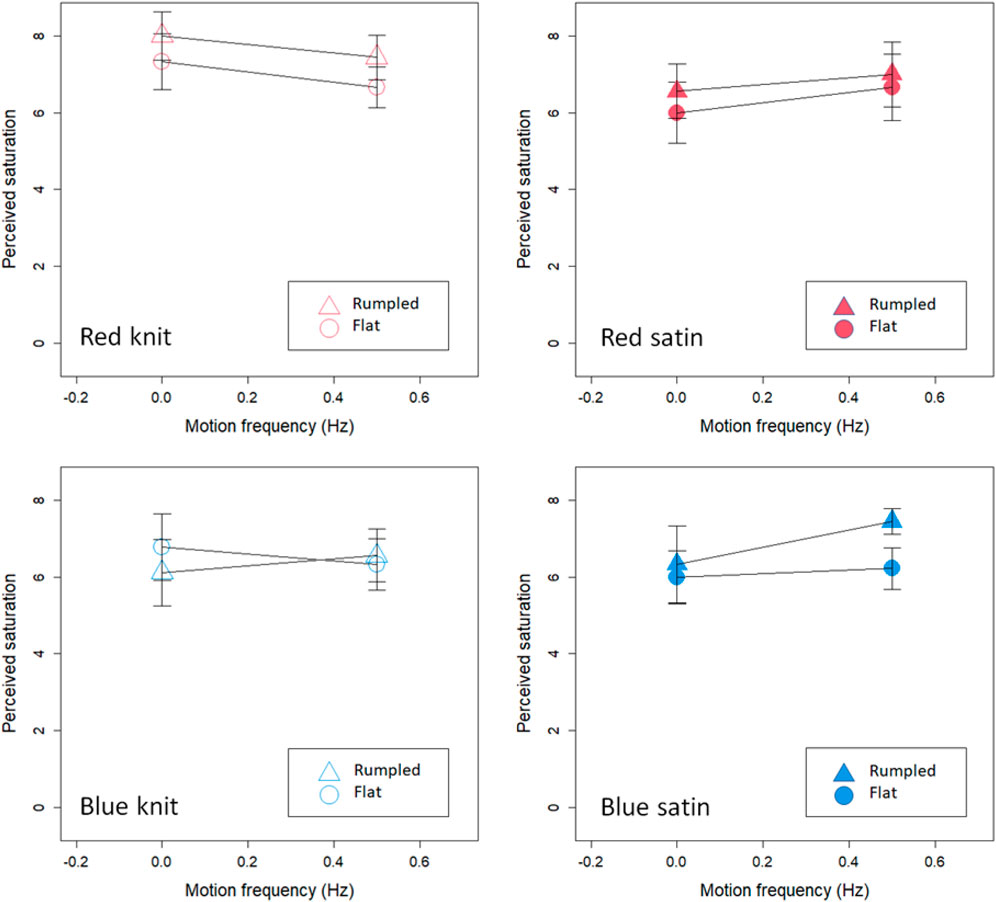
FIGURE 6. Means and standard errors for perceived saturation plotted as a function of motion frequency for red knit (upper left), red satin (upper right), blue knit (lower left) and blue satin (lower right).
Figure 7 shows the effect of fabric type, fabric color, digital folds, and motion on the perception of fabric lightness. A four-way repeated-measures ANOVA found a significant main effect of fabric type on perceived lightness [F (1,8) = 23.88, p < 0.01]. Also, there was a significant main effect of fabric color on perceived lightness [F (1,8) = 6.87, p < 0.05]. There was no main effect of rumple on perceived lightness [F (1,8) = 0.522, p = 0.49], and there was also no main effect of motion on perceived lightness [F (1,8) = 0.295, p = 0.602]. There was a significant two-way effect between fabric color and fabric type [F (1,8) = 7.59, p < 0.05] and a significant three-way effect between fabric color, fabric type and rumple [F (1,8) = 7.12, p < 0.05]. No other interaction effects were found.
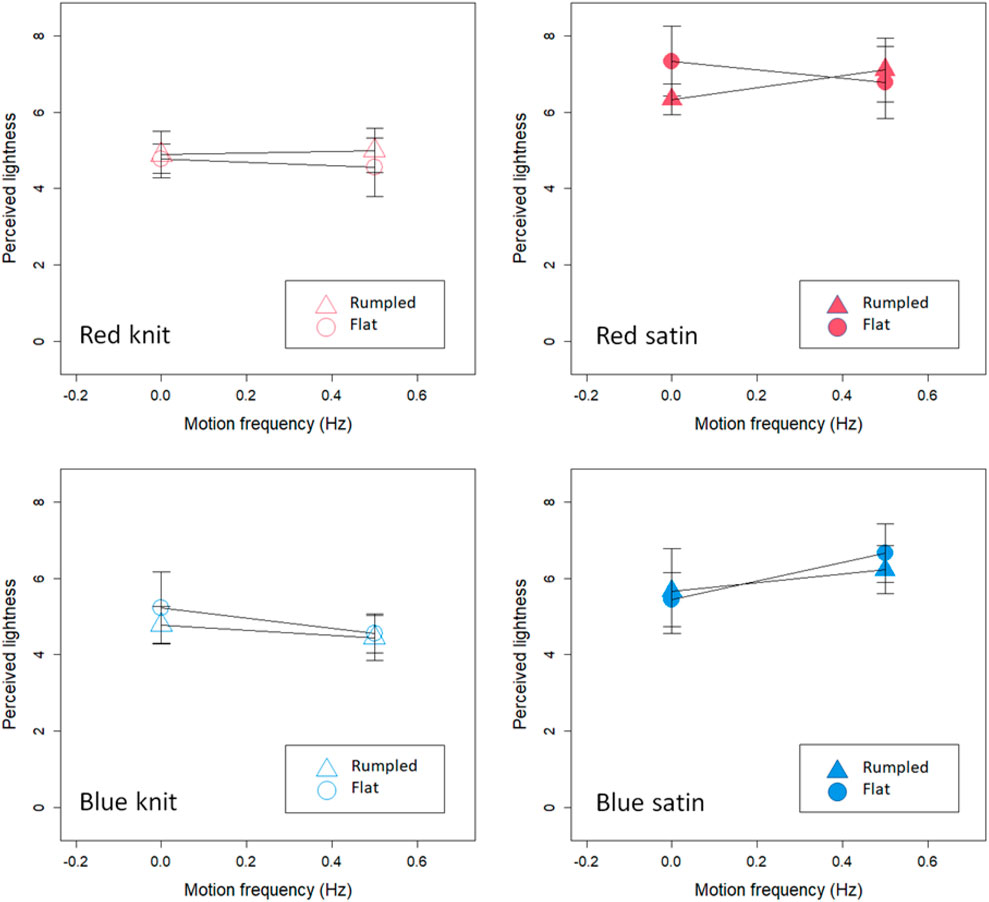
FIGURE 7. Means and standard errors for perceived lightness plotted as a function of motion frequency for red knit (upper left), red satin (upper right), blue knit (lower left) and blue satin (lower right).
Although we attempted to equate our selection of fabrics for saturation and lightness, equating fabrics on the basis of lightness was not possible. Indeed, we find perceived differences in lightness according to fabric type and color, but not for different conditions of motion and rumple. Hence, while we saw effects of rumple on perceived sheen and color saturation, we did not observe those effects on perceived lightness. Instead, perceived lightness depended on differences in the physical reflectance of the samples.
Given our finding of a compelling perceptual effect of simulated digital folds on the perception of sheen and color saturation (and the effect of motion on the perception of sheen), we decided to explore the potential categorical distortions these simulation properties may have on more complex material properties of fabrics (namely, the perception of fabric thickness, stretchability and the type of material from which it appears to be constructed).
To this end, we analyzed our results from a separate cohort of participants who provided these judgements. A summary of the means and standard errors for all of these conditions is shown in Figure 8 below. For the perception of sheen on both samples, a three-way repeated-measures ANOVA found significant main effects of specular amplitude [F (2,50) = 39.82, p < 0.001] and specular roughness [F (2,50) = 139.5, p < 0.001] on perceived sheen. However, unlike in the initial study, there was no main effect of fabric type on the perceived sheen [F (1,25) = 1.074, p = 0.31]. There were significant two-way interactions between fabric type and specular roughness [F (2,50) = 20.09, p < 0.001] and between specular amplitude and specular roughness [F (4,100) = 10.24, p < 0.001] on perceived sheen. Nonetheless, there was no two-way interaction between fabric type and specular amplitude on perceived sheen [F (2,50) = 0.797, p = 0.456]. Additionally, a three-way interaction was found to be significant between fabric type, specular amplitude, and specular roughness on perceived sheen [F (4,100) = 3.048, p < 0.05].
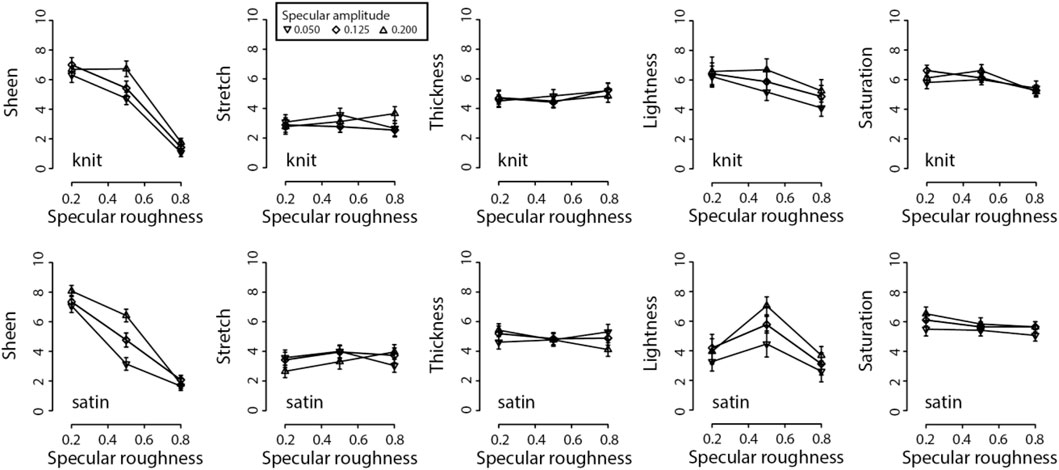
FIGURE 8. Means and standard errors for perceptual judgments (sheen, stretch, thickness, lightness and saturation) plotted as a function of specular roughness. Results for different fabric types are plotted separately as obtained using the cotton knit based fabric (upper row) and satin (lower row). Separate lines in each set of axes represent different specular amplitude levels.
We also considered tactile-related percepts (even though participants did not touch samples). For the perception of stretch on both samples, a three-way repeated-measures ANOVA found a significant main effect of fabric type on perceived stretch [F (1,25) = 6.957, p < 0.05]. Nevertheless, there was no main effect of specular amplitude [F (2,50) = 0.225, p = 0.8] or specular roughness [F (2,50) = 0.523, p = 0.596] on perceived stretch. Although a significant two-way interaction between specular amplitude and specular roughness was found for perceived stretch [F (4,100) = 4.782, p < 0.01], there were no other two-way or three-way interaction effects on perceived stretch.
For the perception of thickness on both samples, a three-way repeated-measures ANOVA found no significant main effect of fabric type [F (1,25) = 0.658, p = 0.425] and specular amplitude [F (2,50) = 0.464, p = 0.632] on perceived thickness. Moreover, no significant main effect was found for specular roughness on the perception of thickness [F (2,50) = 0.145, p = 0.865]. A significant two-way interaction between specular amplitude and specular roughness was found on perceived thickness [F (4,100) = 3.056, p < 0.05]. However, there were no other two-way or three-way interaction effects on perceived thickness.
For consistency with the initial study, we also considered effects on perceived color attributes. A three-way repeated-measures ANOVA found a significant main effect of fabric type [F (1,25) = 61.01, p < 0.001] and specular amplitude [F (2,50) = 27.82, p < 0.001] and specular roughness [F (2,50) = 41.55, p < 0.001] on perceived lightness. In addition, there was a significant two-way interaction between fabric type and specular roughness [F (2,50) = 24.08, p < 0.001] as well as between specular amplitude and specular roughness [F (4,100) = 5.744, p < 0.001] on perceived lightness. However, no other two-way or three-way interaction effects were found on perceived lightness.
For the perception of color saturation, a three-way repeated-measures ANOVA found a significant main effect of specular roughness [F (2,50) = 6.951, p < 0.01] on perceived saturation. Nevertheless, there was no significant main effects of fabric type [F (1,25) = 0.362, p = 0.553] or specular amplitude (F (2,50) = 2.957, p = 0.0611) on perceived saturation. There were no two-way or three-way interaction effects on perceived saturation.
Correlation analysis of pairwise linear relationships between dependent variables is shown in Table 1 for our five perceptual outcome metrics (perceived sheen, thickness, stretch, saturation and lightness). A significant positive correlation was found between perceived sheen and perceived saturation [r (16) = 0.786, p < 0.001] and between perceived sheen and perceived lightness [r (16) = 0.591, p < 0.01]. Also, there was a positive correlation between perceived lightness and perceived saturation [r (16) = 0.479, p < 0.05]. Nevertheless, there was neither a significant correlation between perceived sheen and perceived thickness [r (16) = −0.124, p = 0.62] nor between perceived sheen and perceived stretch [r (16) = −0.186, p = 0.47]. No significant correlation was found between either perceived thickness and perceived stretch [r (16) = −0.376, p = 0.12] nor between perceived thickness and perceived saturation [r (16) = −0.186, p = 0.46]. Also, there was no significant correlation between perceived thickness and perceived lightness [r (16) = −0.176, p = 0.48]. In addition, no significant correlation was found between perceived stretch and perceived saturation [r (16) = −0.298, p = 0.23], nor between perceived stretch and perceived lightness [r (16) = 0.301, p = 0.22].
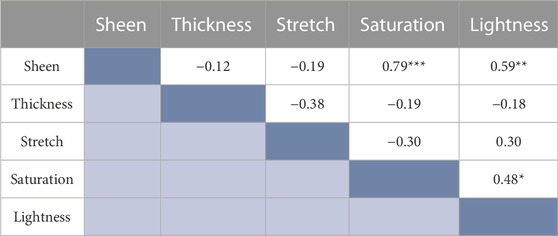
TABLE 1. Correlation matrix showing Pearson’s correlation between perceptual estimates. (*p < 0.05; **p < 0.01; ***p < 0.001).
In addition to the five primary outcome measures we explored so far, we also obtained material classification judgments from our participants across all conditions. We determined the proportions of each of the eight possible alternatives that the cohort of participants selected. Pie charts presented in Figure 9 show the relative distribution of these proportions across changes in specular roughness (left to right) for the base cotton or satin fabrics used in the simulation (upper and lower rows, respectively). Whereas the proportions for judging simulations as depicting cotton increased with increasing specular roughness, the proportions for judging simulations as depicting satin decreased with increasing specular roughness.
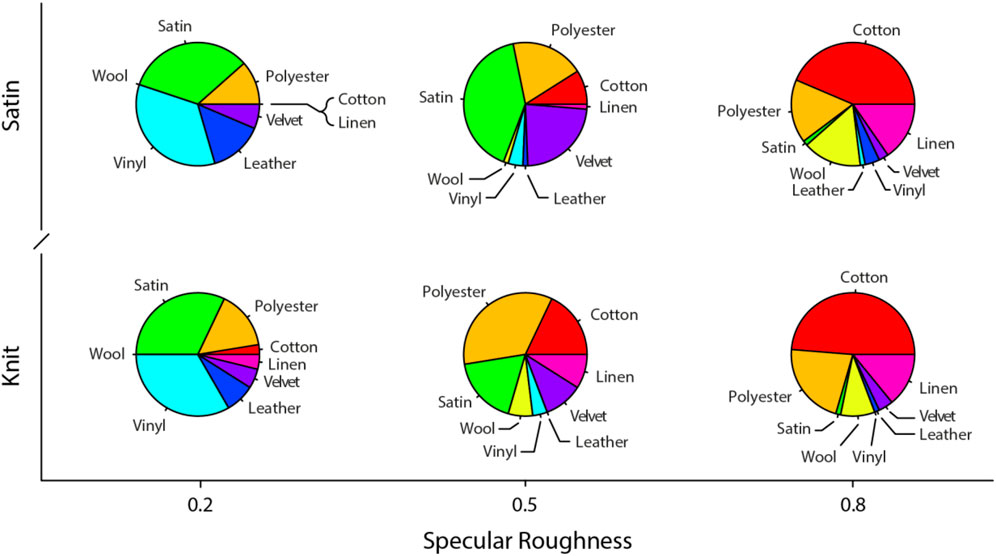
FIGURE 9. Pie charts show the proportion of total material classification judgments made by participants for each of the three levels of specular roughness and each of the base fabric types (knit and satin). Note how the proportion of cotton judgments increases with increasing specular roughness, while the proportion of satin judgments declines with increasing specular roughness for both fabric types.
A chi-square test of independence was performed to examine the relationship between different physical base fabric types used and the judgments of material composition. We also examined the relationship between different levels of specular roughness and judgments of material composition. Any support for the null hypothesis would be obtained when there is no systematic variation in proportions of different material judgments across changes in material type or specular roughness. Consistent with the null hypothesis, there was no significant relationship between the different base fabric types (knit and satin) and the judgments of material composition (X2 (7, N = 26) = 0.1, p = 1). However, we did find a significant relationship between the high and low levels of specular roughness and judgments of material composition (X2 (7, N = 26) = 26, p < .001). These findings indicate that specular roughness appears to strongly influence visual judgements of material composition.
To examine the role of specular roughness (and specular amplitude) on material judgments more closely, we considered whether more generative aspects of the simulation might contribute to the experience of material classifications. Figure 10 plots the individual specular roughness and amplitude values for individual samples judged by each of the participants (small points), with larger points showing the overall mean specular roughness and amplitude across all participants for each of the eight possible material classifications. A paired t-test found there was a significant mean difference in specular roughness for samples classified as cotton versus satin (t22 = 15.37, p < 0.001) or cotton versus vinyl (t22 = 13.26, p < 0.001).
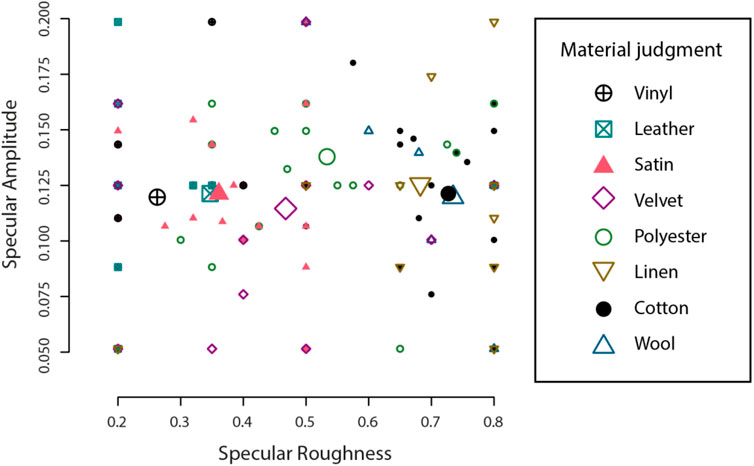
FIGURE 10. Effects of specular roughness and amplitude on material judgments performed under visual inspection. Large points show means, and small points show corresponding individual data from each participant for each of the eight material categories. Material classifications varied mostly along the dimension of specular roughness rather than specular amplitude. Note the large mean separation in specular roughness for percepts of satin and cotton.
Discussion
We explored human visual perception of digital fabrics that were dynamically rendered with different simulated reflectance properties in a web browser. Consistent with our original prediction, we found there were significant main effects of fabric shape (flat versus rumpled) and fabric motion on perceived sheen. These key findings suggest that motion and rumple have significant effects on increasing the perceived sheen of digital fabrics. There were also some complex interactions between fabric shape and motion on the visual perception of fabric sheen and color saturation. Digital folds were more dominant than motion cues in biasing the perception of sheen across the different fabric colors we considered. We also conducted a follow-up study where we only considered blue satin and knit fabric geometries that were digitally matched in hue. We found that altering specular roughness exerted a primary dimensional effect not just on perceived sheen, but also generated inter-correlated effects on perceived color saturation and lightness. These complex interactions accounted for observers’ categorical judgments of material type. This is important because we show that cotton knit could be made to look like satin and satin to look like cotton knit, simply by altering specular roughness (even though the geometries of their weave were very different). We also considered visually inferred tactile properties (e.g., thickness and stretch), but these perceptual attributes were unaffected by changes in specular roughness.
The findings have significant relevance for the understanding of perceived color and gloss considered in previous literature. Previous researchers have proposed that perception of gloss in the human visual system depends on measurable properties of specular reflections, including specular roughness/sharpness (Kim et al., 2012; Marlow et al., 2012; Marlow and Anderson, 2013; Cai et al., 2023). We found that specular roughness also influenced the perception of lightness and color saturation. Consistent with Honson et al. (2020), we found that increasing specular roughness reduced perceived color saturation. Increasing specular roughness increased perceived lightness of satin fabrics (consistent with Honson et al., 2020), but perceived lightness subsequently declined at higher levels of specular roughness. This difference could be attributed to the range of specular roughness levels in the current study extending further than what has previously been explored in earlier studies.
The findings of the current study also appear to expand on other research into the perception of simulated textiles. For example, Xue et al. (2014) studied the interaction between the visual features of skirts and their tactile properties on video and images on a computer. They mentioned that recall memory association between visual and haptic perception generated by daily contact with fabrics is stronger when watching skirts in videos that convey more detailed information about the fabric's tactile properties than still images of skirts. Dynamic motion displays provide observers with more information about the tactile properties of fabrics, compared with static displays. The researchers also found that color and sheen have little influence on perceived tactile properties. In the first study, we focused on how visual perception of surface properties of materials is altered by motion cues derived from genuine fabric samples. Extending the findings of Xue et al. (2014), we found that motion only had a significant effect on perceived sheen. However, we also found that simulated fabric motion had no effect on the perception of color saturation and lightness. Also, in our first study, consistent with Xue et al. (2014), we found that varying fabric color per se had no significant effect on perceived sheen and color saturation. Although variations in color did generate effects on perceived lightness, these effects were caused by actual differences in the physical lightness of fabrics.
We show that motion, and more so 3D digital folds (i.e., rumple) generate significant biases in the appearance of sheen and color saturation of fabrics presented in a web browser. We also showed that changing this simulated specular roughness of digital fabrics has profound effects on the subjective material classification of these simulated digital fabrics. It would be of great benefit to explore in future how motion, rumple and specular roughness together may be used to compensate for any inconsistencies in material appearance between digital fabrics and physical samples consumers may ultimately encounter following an online shopping experience. In the context of virtual reality, it would also be useful to assess the role of stereoscopic cues to the material appearance of digital fabrics, given the influence of stereopsis on perceived gloss (Blake and Bülthoff, 1990), shape (Ohara et al., 2022) and transparency (Kim et al., 2022). This will also have great benefits as an authoring tool in digital fashion design for mitigating waste generated during production.
Conclusion
This study examined biases in human perception of digital fabrics to determine how the industry can improve computer-based representations of textiles for the benefit of digital fashion design and the end-user online shopping experience. Images of digital fabrics rendered by Bivot software were used in an online experiment delivered in a standard web browser using HTML5 and JavaScript. We show evidence that digital folds and motion of simulated fabrics, as well as specular reflectance, have profound effects on visual processes underlying material appearance. Moreover, specular roughness was found to play a key role in the material classification of digital fabrics. It is anticipated that applying these findings to digital media will enhance digital fashion design and the online shopping experience (and hopefully help to reduce textile waste in the process).
Data availability statement
The raw data supporting the conclusion of this article will be made available by the authors, without undue reservation.
Ethics statement
All procedures adhered to the ethical principles outlined in the Declaration of Helsinki and were approved by the Human Research Ethics Advisory Panel at the University of New South Wales (UNSW Sydney). The participants provided their informed consent to participate in this study.
Author contributions
All authors listed have made a substantial, direct, and intellectual contribution to the work and approved it for publication.
Funding
This research was funded by an ARC Discovery Project grant awarded to JK (DP230100303).
Conflict of interest
MA, DM and DK are employees of Bandicoot Imaging Sciences, Australia. All participant recruitment, data collection and analysis were performed independently by UNSW Sydney.
The remaining authors declare that the research was conducted in the absence of any commercial or financial relationships that could be construed as a potential conflict of interest.
Publisher’s note
All claims expressed in this article are solely those of the authors and do not necessarily represent those of their affiliated organizations, or those of the publisher, the editors and the reviewers. Any product that may be evaluated in this article, or claim that may be made by its manufacturer, is not guaranteed or endorsed by the publisher.
Abbreviations
BRDF, Bi-directional Reflectance Distribution Function; 3D, Three Dimensional; DSRL, Digital Single-lens Reflex; PPI, Pixels Per Inch; HTML5, Hypertext Markup Language Revision 5, ANOVA, Analysis of Variance.
References
Aldás-Manzano, J., Currás-Pérez, R., and Sanz-Blas, S. (2011). Online information quality as determinant of perceived risk reduction in e-commerce: an application to apparel virtual stores. Int. J. Internet Mark. Advert. 6 (4), 352–372. doi:10.1504/ijima.2011.043656
Anderson, B. L., and Kim, J. (2009). Image statistics do not explain the perception of gloss and lightness. J. Vis. 9 (11), 10. doi:10.1167/9.11.10
Anderson, B. L., and Marlow, P. J. (2022). Perceiving the shape and material properties of 3D surfaces. Trends Cognitive Sci. 27, 98–110. doi:10.1016/j.tics.2022.10.005
Australian Government (2021). Minister's Priority List 2021-22. Department of Climate Change, Energy, the Environment and Water. Available at: https://www.dcceew.gov.au/environment/protection/waste/product-stewardship/ministers-priority-list/2021-22#:∼:text=New%20to%20the%20Minister's%20Priority,well%20as%20plastic%20oil%20containers.
Barrow, H., Tenenbaum, J., Hanson, A., and Riseman, E. (1978). Recovering intrinsic scene characteristics. Comput. Vis. Syst. 2 (3-26), 2.
Blake, A., and Bülthoff, H. (1990). Does the brain know the physics of specular reflection? Nature 343, 165–168. doi:10.1038/343165a0
Cai, Y., Kiyokawa, H., Nagai, T., Haghzare, L., Arnison, M., and Kim, J. (2023). Effects of specular roughness on the perception of color and opacity. JOSA A 40 (3), A220–A229. doi:10.1364/josaa.479972
Devderea, C., and Toader, F. (2018). “Consumer behavior towards apparel E-commerce in RomaniaManag. Dyn. Knowl. Econ. 6(3), 471–487. doi:10.25019/MDKE/6.3.07
Doerschner, K., Fleming, R. W., Yilmaz, O., Schrater, P. R., Hartung, B., and Kersten, D. (2011). Visual motion and the perception of surface material. Curr. Biol. 21 (23), 2010–2016. doi:10.1016/j.cub.2011.10.036
Fleming, R. W., Dror, R. O., and Adelson, E. H. (2003). Real-world illumination and the perception of surface reflectance properties. J. Vis. 3 (5), 3. doi:10.1167/3.5.3
Fleming, R. W., Torralba, A., and Adelson, E. H. (2004). Specular reflections and the perception of shape. J. Vis. 4 (9), 10. doi:10.1167/4.9.10
García-Salirrosas, E. E., Acevedo-Duque, Á., Marin Chaves, V., Mejía Henao, P. A., and Olaya Molano, J. C. (2022). Purchase intention and satisfaction of online shop users in developing countries during the COVID-19 pandemic. Sustainability 14 (10), 6302. doi:10.3390/su14106302
García-Salirrosas, E. E., and Acevedo-Duque, Á. (2022). PERVAINCONSA scale to measure the consumer behavior of online stores of MSMEs engaged in the sale of clothing. Sustainability 14 (5), 2638. doi:10.3390/su14052638
Hartung, B., and Kersten, D. (2002). Distinguishing shiny from matte. J. Vis. 2 (7), 551. doi:10.1167/2.7.551
Honson, V., Huynh-Thu, Q., Arnison, M., Monaghan, D., Isherwood, Z. J., and Kim, J. (2020). Effects of shape, roughness and gloss on the perceived reflectance of colored surfaces. Front. Psychol. 11, 485. doi:10.3389/fpsyg.2020.00485
Isherwood, Z. J., Huynh-Thu, Q., Arnison, M., Monaghan, D., Toscani, M., Perry, S., et al. (2021). Surface properties and the perception of color. J. Vis. 21 (2), 7. doi:10.1167/jov.21.2.7
Kasavan, S., Yusoff, S., Guan, N. C., Zaman, N. S. K., and Fakri, M. F. R. (2021). Global trends of textile waste research from 2005 to 2020 using bibliometric analysis. Environ. Sci. Pollut. Res. 28, 44780–44794. doi:10.1007/s11356-021-15303-5
Kim, J., Hong, G., and Anstis, S. (2022). Size vs. stereo in illusory depth inversion. Front. Psychol. 12, 817745. doi:10.3389/fpsyg.2021.817745
Kim, J., Marlow, P. J., and Anderson, B. L. (2012). The dark side of gloss. Nat. Neurosci. 15 (11), 1590–1595. doi:10.1038/nn.3221
Kiyokawa, H., Nagai, T., Yamauchi, Y., and Kim, J. (2023). The perception of translucency from surface gloss. Vis. Res. 205, 108140. doi:10.1016/j.visres.2022.108140
Marlow, P. J., and Anderson, B. L. (2013). Generative constraints on image cues for perceived gloss. J. Vis. 13 (14), 2. doi:10.1167/13.14.2
Marlow, P. J., Kim, J., and Anderson, B. L. (2012). The perception and misperception of specular surface reflectance. Curr. Biol. 22 (20), 1909–1913. doi:10.1016/j.cub.2012.08.009
Nicodemus, F. E. (1965). Directional reflectance and emissivity of an opaque surface. Appl. Opt. 4 (7), 767–775. doi:10.1364/ao.4.000767
Ohara, M., Kim, J., and Koida, K. (2022). The role of specular reflections and illumination in the perception of thickness in solid transparent objects. Front. Psychol. 13, 766056. doi:10.3389/fpsyg.2022.766056
Sakano, Y., and Ando, H. (2010). Effects of head motion and stereo viewing on perceived glossiness. J. Vis. 10 (9), 15. doi:10.1167/10.9.15
Vasic, N., Kilibarda, M., and Kaurin, T. (2019). The influence of online shopping determinants on customer satisfaction in the Serbian market. J. Theor. Appl. Electron. Commer. Res. 14 (2), 0. doi:10.4067/s0718-18762019000200107
Wiranata, A. T., and Hananto, A. (2020). Do website quality, fashion consciousness, and sales promotion increase impulse buying behavior of e-commerce buyers? Indonesian J. Bus. Entrepreneursh. (IJBE) 6 (1), 74. doi:10.17358/ijbe.6.1.74
Xiong, L., Wang, H., and Wang, C. (2022). Predicting mobile government service continuance: a two-stage structural equation modeling-artificial neural network approach. Gov. Inf. Q. 39 (1), 101654. doi:10.1016/j.giq.2021.101654
Keywords: digital fabrics, perceived sheen, perceived color, specular roughness, online shopping, fashion design
Citation: Haghzare L, Ping X, Arnison M, Monaghan D, Karlov D, Honson V and Kim J (2023) Digital fabrics for online shopping and fashion design. Front. Virtual Real. 4:1236095. doi: 10.3389/frvir.2023.1236095
Received: 07 June 2023; Accepted: 13 July 2023;
Published: 21 August 2023.
Edited by:
Xiaogang Jin, Zhejiang University, ChinaCopyright © 2023 Haghzare, Ping, Arnison, Monaghan, Karlov, Honson and Kim. This is an open-access article distributed under the terms of the Creative Commons Attribution License (CC BY). The use, distribution or reproduction in other forums is permitted, provided the original author(s) and the copyright owner(s) are credited and that the original publication in this journal is cited, in accordance with accepted academic practice. No use, distribution or reproduction is permitted which does not comply with these terms.
*Correspondence: Leyla Haghzare, bC5oYWdoemFyZUB1bnN3LmVkdS5hdQ==
†These authors have contributed equally to this work