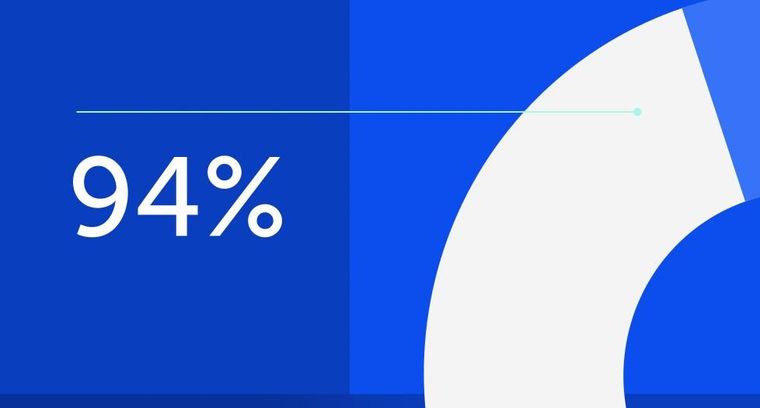
94% of researchers rate our articles as excellent or good
Learn more about the work of our research integrity team to safeguard the quality of each article we publish.
Find out more
ORIGINAL RESEARCH article
Front. Virtual Real., 04 August 2023
Sec. Augmented Reality
Volume 4 - 2023 | https://doi.org/10.3389/frvir.2023.1214520
This article is part of the Research TopicAugmenting Human Experience and Performance through Interaction TechnologiesView all 8 articles
Background: Multiple 3D visualization techniques are available that obviates the need for the surgeon to mentally transform the 2D planes from MRI to the 3D anatomy of the patient. We assessed the spatial understanding of a brain tumour when visualized with MRI, 3D models on a monitor or 3D models in mixed reality.
Methods: Medical students, neurosurgical residents and neurosurgeons were divided into three groups based on the imaging modality used for preparation: MRI, 3D viewer and mixed reality. After preparation, the participants needed to position, scale, and rotate a virtual tumour inside a virtual head of the patient in the same orientation as the original tumour would be. Primary outcome was the amount of overlap between the placed tumour and the original tumour to evaluate accuracy. Secondary outcomes were the position, volume and rotation deviation compared to the original tumour.
Results: A total of 12 medical students, 12 neurosurgical residents, and 12 neurosurgeons were included. For medical students, the mean amount of overlap for the MRI, 3D viewer and mixed reality group was 0.26 (0.22), 0.38 (0.20) and 0.48 (0.20) respectively. For residents 0.45 (0.23), 0.45 (0.19) and 0.68 (0.11) and for neurosurgeons 0.39 (0.20), 0.50 (0.27) and 0.67 (0.14). The amount of overlap for mixed reality was significantly higher on all expertise levels compared to MRI and on resident and neurosurgeon level also compared to the 3D viewer. Furthermore, mixed reality showed the lowest deviations in position, volume and rotation on all expertise levels.
Conclusion: Mixed reality enhances the spatial understanding of brain tumours compared to MRI and 3D models on a monitor. The preoperative use of mixed reality may therefore support the surgeon to improve spatial 3D related surgical tasks such as patient positioning and planning surgical trajectories.
In the preoperative neurosurgical workflow for intracranial tumour resection, magnetic resonance imaging (MRI) is often used for the evaluation of the pathology and surrounding anatomical structures. (Villanueva-Meyer, Mabray and Cha, 2017). Since MRI offers 2D planes of a 3D volume, neurosurgeons are required to mentally transform these images to the 3D anatomy of the patient in order to comprehend the spatial relationship between the different structures. Evaluation of the anatomical structures in 3D obviates the need for this transformation and might support the surgeon in the peri-operative workflow. (Swennen, Mollemans and Schutyser, 2009; Stadie and Kockro, 2013; Preim and Botha, 2014; Abhari et al., 2015; Wake et al., 2019). Most neuronavigation systems support a segmentation solution to create 3D models of the patients’ anatomy, which can be viewed on a monitor. However, 3D models on a monitor remain a 2D depiction of a 3D world. Moreover, other 3D visualization techniques are also available with recent development in mixed reality due to innovation in mixed reality-head-mounted displays (MR-HMDs).
Several extended reality modalities exist; virtual reality, augmented reality and mixed reality. In virtual reality the user is completely closed off from the real environment while with augmented reality the virtual models are merged into the real world. Mixed reality is the extension of augmented reality where these virtual 3D models are not only merged and anchored into the real world, but the user can additionally interact and manipulate them in real-time. It combines the physical and digital worlds, enabling users to perceive and interact with virtual content as if it were part of their actual surroundings. Due to the hand-, eye- and position tracking of the MR-HMDs they provide an active interaction between the user and virtual 3D models. (Westby, 2021). Moreover, MR-HMDs offer a stereoscopic view for a better depth perception and can show the anatomy on a 1:1 scale. Studies have shown additional value of mixed reality in neurosurgical education and preoperative assessment of Wilms tumours. (Pelargos et al., 2017; Wellens et al., 2019). However, little is known about the added value of these qualities in improving the spatial understanding of brain tumours compared to MRI or 3D models on a monitor.
In this study we will compare the spatial understanding of brain tumours when examining the pathology through either MRI, 3D models on a monitor or 3D models in mixed reality. Furthermore, we will compare these results on different levels of experience.
Participants were tested on their spatial understanding of the shape and size of a brain tumour and of its position within the head of the patient. An MR-HMD (HoloLens 2, Microsoft, Redmond, WA, United States) was used to mimic the patients’ head and tumour for the experiment. This device features a high-resolution, see-through display that allows users to view and interact with holographic images integrated into their surroundings. This display creates a mixed reality experience by overlaying virtual objects onto the real world, enabling users to perceive and interact with them in real-time. The virtual models that were created in our study were constructed from the MRI of the patient and were shown on a 1:1 scale, creating a virtual replica of reality. Participants were asked to position, scale, and rotate a virtual tumour inside the virtual head of the patient in the same orientation as the original tumour would be. This was performed in separate groups based on three different imaging modalities used for preparation: MRI, 3D models on a monitor, and 3D models in mixed reality.
Participants were included from the University Medical Center Utrecht based on three levels of experience: medical student, neurosurgical resident, and neurosurgeon. On each level a total of 12 participants were included and further subdivided into three groups: MRI, 3D viewer, and mixed reality. They were allocated in a 1:1:1 ratio in a parallel design, leaving 4 participants in each group on all levels. Participant characteristics of each group are shown in Table 1. Approval of the local ethics committee was not necessary for this study.
MRI group: An MRI T1 with contrast series was shown on a monitor through a conventional radiology viewer (RadiAnt DICOM Viewer 2021.2.2, Medixant, Poznań, Poland) in axial, sagittal, and coronal perspective, which could be scrolled through by the participant (Figure 1A).
For both 3D model groups we used a cloud environment (Lumi, Augmedit, Naarden, Netherlands) that is recently available and CE certified for preoperative use. It has an integrated automatic segmentation algorithm for MRI T1 with contrast series that creates surface-based 3D models of the skin, brain, ventricles and tumour, which we validated in previous studies. (Fick et al., 2021; van Doormaal et al., 2021). The algorithm uses image specific thresholds and sets up spheres with adaptive meshing to find the radiologic boundaries of the sought tissues. They enable robust handling of noisy and poor contrast regions. The 3D models were checked by a neurosurgeon and when needed manually altered to create the most optimal 3D representation for the experiment.
3D viewer group: The Lumi cloud environment was used with integrated Unity 3D viewer that could be accessed through a web browser. The participants could view the models in this viewer on a monitor from every angle and could zoom in- and out. A separate menu was available where each individual model could be turned on, off or shown in a transparent setting. The original MRI could be seen in relation to the models in axial, sagittal, and coronal perspective (Figure 1B).
Mixed reality group: The Lumi application on the HoloLens 2 was used where the 3D models could be viewed directly from the cloud environment. The participant could walk around the models, grab and rotate them to view them from every angle. The same menu with similar functionalities as in the 3D viewer was shown next to the models but now in mixed reality. The original MRI could be moved through the models in axial, sagittal, and coronal perspective and with a free-moving plane from every angle (Figure 1C).
Each participant had to examine five tumour cases through the imaging modality according to their allocated group. In every group, the participant had 1 min to go through the case to ensure each participant had the same preparation time before starting the experiment. All cases were identical among all participants and performed in the same consecutive order. After each case a task was performed in mixed reality with the HoloLens 2 in specially designed software for the current study. During the task, a transparent skin model and an opaque tumour model of the patient were shown. The tumour was projected outside the head of the patient and scaled and rotated in a random offset. The participant had to position, scale, and rotate the tumour inside the head of the patient as the original tumour.
The different functionalities could be controlled with a separate menu. Furthermore, a switch was implemented, that could be turned on or off, that reduced the movements of the user with a factor 10 on the virtual models so the participants could better control and fine-tune their task. During the experiment the participant could review the imaging of their assigned group. However, the mixed reality models of the task were not allowed to be placed next to the imaging modality of the assigned group. So the participants could not compare the original imaging and the mixed reality models directly next to each other. The task was completed once the participant was satisfied with the result.
None of the participants had any mixed reality experience. Therefore each participant received a standardized mixed reality training of Microsoft where the different functionalities that were needed for the experiment were practised. Furthermore, every participant performed a practice case that was identical to the real experiment in order to get used to the workflow and controls. These results were not included in the study. An axial, coronal, and sagittal perspective of the MRI and 3D model of each tumour case is shown in Supplementary Figure S1.
Primary outcome was the amount of overlap between the placed tumour and the original tumour. The overlap was defined as the Sørensen–Dice similarity coefficient (DSC), which calculates the amount of overlap between 2 volumetric sets using the formula
FIGURE 2. A visual representation of the calculated rotation error between the placed tumour and the original tumour. The original tumour (A) and the placed tumour (B) are shown with identical specified vectors where the shortest angle between the two vectors is identified as the rotation error (C). Note that this representation is in 2D whereas the used vectors and angle calculations in the experiment were performed in 3D.
Lastly, we measured time spent on the task and used the NASA Task Load Index (See Supplementary Figure S2). This is a standardized assessment tool to evaluate the perceived workload of the task in multiple dimensions on a 21-point Likert scale. (Hart and Staveland, 1988). We excluded the ‘physical demand’ section of the standardized assessment as this was not appropriate to our study. Each participant was instructed to answer each dimension of the assessment from the perspective of how they felt about reaching the goals of the task based on their original imaging.
To determine statistical significant differences between groups, the one-way ANOVA was used with a post-hoc Tukey test provided the data followed a normal distribution. This was checked visually with a histogram, a Q-Q plot and with the Shapiro-Wilk test. Skewed data was transformed or non-parametric tests were used; the Kruskal–Wallis test with a post-hoc Mann-Whitney-U test. A p-value of < .05 was deemed statistically significant. Statistics were performed in a statistical software package (SPSS version 28.0, SPSS Inc., Chicago, Illinois, United States).
The mean and standard deviation including level of significance of the amount of overlap of each group in all expertise levels are shown in Table 2. Among the medical students, the mean (SD) amount of overlap for the MRI, 3D viewer and mixed reality group was 0.26 (0.22), 0.38 (0.20) and 0.48 (0.20) respectively. The one-way ANOVA showed a significant difference between groups (p = .004). Post hoc comparisons showed that the mean score for the mixed reality group was significantly higher than the MRI group (0.22, 95% CI 0.07 to 0.39; p = .003).
TABLE 2. Mean (SD) amount of overlap of each group in all expertise levels. Only statistically significant differences are reported under p-value.
Among the residents, the mean (SD) amount of overlap for the MRI, 3D viewer and mixed reality group was 0.45 (0.23), 0.45 (0.19) and 0.68 (0.11) respectively. The one-way ANOVA showed a significant difference between groups (p = <.001). Post hoc comparisons showed that the mean score for the mixed reality group was significantly higher than the MRI (0.23, 95% CI 0.09 to 0.36; p = <.001) and 3D viewer group (0.22, 95% CI 0.08 to 0.36; p = <.001).
Among the neurosurgeons, the mean (SD) amount of overlap for the MRI, 3D viewer and mixed reality group was 0.39 (0.20), 0.50 (0.27) and 0.67 (0.14) respectively. The one-way ANOVA showed a significant difference between groups (p = <.001). Post hoc comparisons showed that the mean score for the mixed reality group was significantly higher than the MRI (0.29, 95% CI 0.13 to 0.44; p = <.001) and 3D viewer group (0.17, 95% CI 0.01 to 0.33; p = .029).
There were no statistically significant differences between other groups on each expertise level.
Mean outcome scores of the position, rotation, and volume for each group in all expertise levels are illustrated in Figure 3. On each level of expertise the mixed reality group shows better accuracy for all components compared to the 3D viewer and MRI group. Medical students performed worst in the MRI group with high deviations, especially on rotation and position. This improved when working with the 3D viewer and further improved with mixed reality. On resident and neurosurgeon level the difference in performance between MRI and 3D viewer seems limited. All three groups among residents and neurosurgeons performed superior to the corresponding group among medical students with the biggest differences found in the MRI group.
FIGURE 3. Mean position, volume, and rotation deviation of the placed tumour compared to the original tumour for each group in all expertise levels.
Figure 4 illustrates the mean outcome measures per group on each expertise level on an example tumour. It shows on each expertise level how accurate the tumour is placed on average in each group.
FIGURE 4. Mean accuracy of all groups visualized on an example tumour. The opaque tumour is the original tumour and the transparent tumour is the placed tumour, which is deviated with the mean position, volume, and rotation of the according subgroup.
There were no statistically significant differences between groups in time spent on the task among medical students [F (2, 57) = 1.05, p = .356], residents [F (2, 57) = 2.07, p = .135], and neurosurgeons [F (2, 57) = 1.92, p = .156]. The means and standard deviations including level of significance of the secondary outcome measures are shown in Supplementary Table S1. The results of the NASA task load index are shown in Figure 5. The 3D viewer and mixed reality group had lower scores in perceived mental and temporal workload compared to the MRI group, especially on resident and neurosurgeon level. Furthermore, the amount of effort it took is lower as well in the 3D viewer and mixed reality group compared to MRI. However, how successful they felt on accomplishing the goal of the task (performance level) appear similar. Lastly, among neurosurgeons the 3D viewer shows a lower mental and temporal workload and less effort and frustration compared to mixed reality.
In this study we evaluated the effect of MRI, a 3D viewer, and mixed reality on the spatial understanding of brain tumours among medical students, residents and neurosurgeons. Mixed reality showed the best accuracy with the amount of overlap and on all secondary outcome measures regarding accuracy in each expertise level.
In current medical care, MRI is used to evaluate brain tumours and preparing a surgical plan. Additionally, several clinical applications support a segmentation solution to create 3D models of the patients’ anatomy that can be viewed on a monitor. Our results show the additional value of this visualization technique for the spatial understanding of brain tumours among residents and neurosurgeons is limited next to MRI. However, mixed reality does further improve the spatial understanding of brain tumours, which corroborates its additional value.
In the MRI group, medical students showed the most difficulty in accurate depiction of the brain tumour compared to residents and neurosurgeons. This indicates MRI requires a learning curve to interpret correctly. However, it seems this learning curve has a limit as the performance between residents and neurosurgeons appear similar. Since none of the participants had any significant prior HoloLens experience, the results of the mixed reality groups are without a notable learning curve. This means the differences found between MRI and mixed reality might only further increase when mixed reality becomes more frequently used. Moreover, the standard deviation of the amount of overlap in the mixed reality groups is relatively smaller compared to the other groups. This shows evaluation in mixed reality is not only more accurate, but also more consistent.
The NASA Task Load Index showed a higher mental workload in the MRI groups compared to the 3D viewer and mixed reality groups, especially among residents and neurosurgeons. The operating room is a complex and dynamic work environment with multitasking and frequent interruptions in surgical workflow. (Göras et al., 2019). Moreover, the mental workload for the surgeon during procedures is high. (Lowndes et al., 2020). Decreasing the mental workload and effort by using 3D models can create more room mentally to concentrate on other surgical tasks. Therefore the use of 3D might contribute to a safer and a more efficient workflow. (Goodell, Cao and Schwaitzberg, 2006; Huotarinen, Niemelä and Hafez, 2018). However, the amount of datapoints on this matter in our study is limited and should be interpreted with caution.
First, for an in-depth analysis of the different spatial characteristics of the tumour, the task needed to be performed virtually. We used mixed reality since these 3D models are the best possible approximation to reality as they are created from the MRI of the patient and on a 1:1 scale. This meant the mixed reality group examined the tumour case and subsequently perform the task both in the HoloLens. However, within the mixed reality groups both the residents and neurosurgeons performed superior compared to medical students. This indicates that also in the mixed reality group, trained spatial awareness was a key factor in high performance.
Second, although the 3D models in the HoloLens are a virtual replica of reality, we can not extrapolate these results to the added value in spatial understanding of a real patient with certainty.
Lastly, the performed task is not a specific clinical task and is therefore difficult to relate to patient outcome.
Our results quantify the improvement in spatial understanding of brain tumours when viewed through MRI, 3D models on a monitor, and mixed reality. It corroborates the potential value of mixed reality for surgical tasks where accurate depiction of the spatial anatomy and anatomical relation between structures is vital. It might support the neurosurgeon in optimal patient positioning, planning surgical trajectories and intraoperative guidance. However, the additional value of mixed reality during these surgical tasks should be further explored in future research.
Mixed reality enhances the spatial understanding of brain tumours compared to MRI and 3D models on a monitor. The preoperative use of mixed reality in clinical practice might therefore support specific spatial 3D related tasks as optimal patient positioning and planning surgical trajectories.
The raw data supporting the conclusion of this article will be made available by the authors, without undue reservation.
Ethical review and approval was not required for the study on human participants in accordance with the local legislation and institutional requirements. Written informed consent for participation was not required for this study in accordance with the national legislation and the institutional requirements.
Conception and design: TF, JM, MK, JV, TV, and EH Acquisition of data: TF and MK Analysis: TF Interpretation of data: TF, JM, MK, JV, TV, and EH Drafting the article: TF Revising it critically: JM, MK, JV, TV, and EH Final approval of the version to be published: TF, JM, MK, JV, TV, and EH. All authors contributed to the article and approved the submitted version.
TvD is founder and CMO and JM is senior product developer of Augmedit bv, a start-up company that develops mixed reality tools for surgeons.
The remaining authors declare that the research was conducted in the absence of any commercial or financial relationships that could be construed as a potential conflict of interest.
All claims expressed in this article are solely those of the authors and do not necessarily represent those of their affiliated organizations, or those of the publisher, the editors and the reviewers. Any product that may be evaluated in this article, or claim that may be made by its manufacturer, is not guaranteed or endorsed by the publisher.
The Supplementary Material for this article can be found online at: https://www.frontiersin.org/articles/10.3389/frvir.2023.1214520/full#supplementary-material
Abhari, K., Baxter, J. S. H., Chen, E. C. S., Khan, A. R., Peters, T. M., de Ribaupierre, S., et al. (2015). Training for planning tumour resection: Augmented reality and human factors. IEEE Trans. Biomed. Eng. 62 (6), 1466–1477. doi:10.1109/TBME.2014.2385874
Fick, T., van Doormaal, J. A. M., Tosic, L., van Zoest, R. J., Meulstee, J. W., Hoving, E. W., et al. (2021). Fully automatic brain tumor segmentation for 3D evaluation in augmented reality. Neurosurg. Focus 51 (2), E14–E18. doi:10.3171/2021.5.FOCUS21200
Goodell, K. H., Cao, C. G. L., and Schwaitzberg, S. D. (2006). Effects of cognitive distraction on performance of laparoscopic surgical tasks. J. Laparoendosc. Adv. Surg. Tech. 16 (2), 94–98. doi:10.1089/lap.2006.16.94
Göras, C., Olin, K., Unbeck, M., Pukk-Härenstam, K., Ehrenberg, A., Tessma, M. K., et al. (2019). Tasks, multitasking and interruptions among the surgical team in an operating room: A prospective observational study. BMJ Open 9 (5), e026410. doi:10.1136/bmjopen-2018-026410
Hart, S. G., and Staveland, L. E. (1988). Development of NASA-TLX (task load index) results of empirical and theoretical research. Hum. Ment. Workload 52, 139–183. doi:10.1007/s10749-010-0111-6
Huotarinen, A., Niemelä, M., and Hafez, A. (2018). The impact of neurosurgical procedure on cognitive resources: Results of bypass training. Surg. Neurol. Int. 9 (1), 71. doi:10.4103/SNI.SNI_427_17
Lowndes, B. R., Forsyth, K. L., Blocker, R. C., Dean, P. G., Truty, M. J., Heller, S. F., et al. (2020). NASA-TLX assessment of surgeon workload variation across specialties. Ann. Surg. 271 (4), 686–692. doi:10.1097/SLA.0000000000003058
Pelargos, P. E., Nagasawa, D. T., Lagman, C., Tenn, S., Demos, J. V., Lee, S. J., et al. (2017). Utilizing virtual and augmented reality for educational and clinical enhancements in neurosurgery. J. Clin. Neurosci. 35, 1–4. doi:10.1016/j.jocn.2016.09.002
Preim, B., and Botha, C. (2014). Visual computing for medicine: Theory, algorithms, and applications. 2. Amsterdam, Netherlands: Elsevier Science. doi:10.1016/B978-0-12-415873-3.00035-3
Stadie, A. T., and Kockro, R. A. (2013). Mono-stereo-autostereo. Evol. 3-Dimensional Neurosurg. Planning’ 72, 63–77. S1. doi:10.1227/NEU.0b013e318270d310
Swennen, G. R. J., Mollemans, W., and Schutyser, F. (2009). Three-dimensional treatment planning of orthognathic surgery in the era of virtual imaging. J. Oral Maxillofac. Surg. 67 (10), 2080–2092. doi:10.1016/j.joms.2009.06.007
van Doormaal, J. A. M., Fick, T., Ali, M., Köllen, M., van der Kuijp, V., and van Doormaal, T. P. (2021). Fully automatic adaptive meshing based segmentation of the ventricular system for augmented reality visualization and navigation. World Neurosurg. 156, e9–e24. doi:10.1016/j.wneu.2021.07.099
Villanueva-Meyer, J. E., Mabray, M. C., and Cha, S. (2017). Current clinical brain tumor imaging. Clin. Neurosurg. 81 (3), 397–415. doi:10.1093/neuros/nyx103
Wake, N., Wysock, J. S., Bjurlin, M. A., Chandarana, H., and Huang, W. C. (2019). ‘“Pin the tumor on the kidney:” an evaluation of how surgeons translate CT and MRI data to 3D models’. Urology 131, 255–261. doi:10.1016/j.urology.2019.06.016
Wellens, L. M., Meulstee, J., van de Ven, C. P., Terwisscha van Scheltinga, C. E. J., Littooij, A. S., van den Heuvel-Eibrink, M. M., et al. (2019). Comparison of 3-dimensional and augmented reality kidney models with conventional imaging data in the preoperative assessment of children with Wilms tumors assessment of children with Wilms tumors. JAMA Netw. Open 2 (4), e192633. doi:10.1001/jamanetworkopen.2019.2633
Keywords: brain tumour, mixed reality, 3D visualization, spatial understanding, accuracy
Citation: Fick T, Meulstee JW, Köllen MH, Van Doormaal JAM, Van Doormaal TPC and Hoving EW (2023) Comparing the influence of mixed reality, a 3D viewer, and MRI on the spatial understanding of brain tumours. Front. Virtual Real. 4:1214520. doi: 10.3389/frvir.2023.1214520
Received: 29 April 2023; Accepted: 14 June 2023;
Published: 04 August 2023.
Edited by:
Giacinto Barresi, Italian Institute of Technology (IIT), ItalyReviewed by:
Pierre Boulanger, University of Alberta, CanadaCopyright © 2023 Fick, Meulstee, Köllen, Van Doormaal, Van Doormaal and Hoving. This is an open-access article distributed under the terms of the Creative Commons Attribution License (CC BY). The use, distribution or reproduction in other forums is permitted, provided the original author(s) and the copyright owner(s) are credited and that the original publication in this journal is cited, in accordance with accepted academic practice. No use, distribution or reproduction is permitted which does not comply with these terms.
*Correspondence: T. Fick, dC5maWNrLTNAdW1jdXRyZWNodC5ubA==
Disclaimer: All claims expressed in this article are solely those of the authors and do not necessarily represent those of their affiliated organizations, or those of the publisher, the editors and the reviewers. Any product that may be evaluated in this article or claim that may be made by its manufacturer is not guaranteed or endorsed by the publisher.
Research integrity at Frontiers
Learn more about the work of our research integrity team to safeguard the quality of each article we publish.