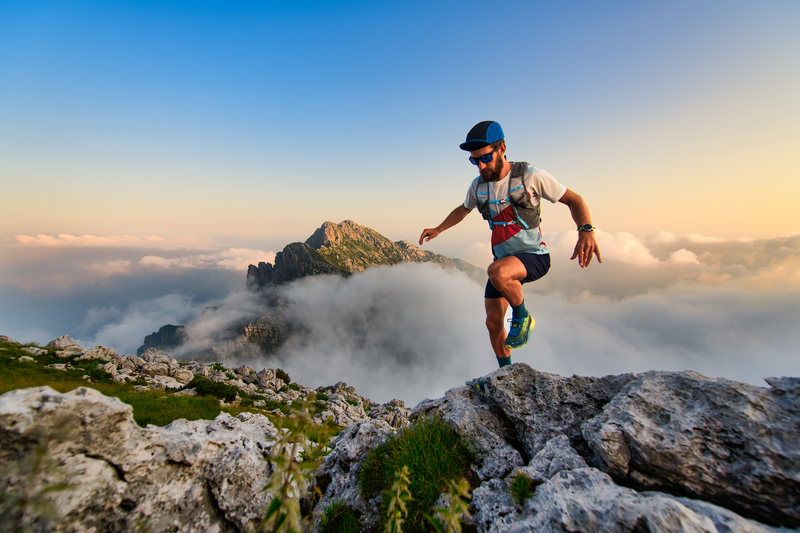
94% of researchers rate our articles as excellent or good
Learn more about the work of our research integrity team to safeguard the quality of each article we publish.
Find out more
ORIGINAL RESEARCH article
Front. Virtual Real. , 14 August 2023
Sec. Virtual Reality and Human Behaviour
Volume 4 - 2023 | https://doi.org/10.3389/frvir.2023.1189717
This article is part of the Research Topic Understanding and Improving the ‘Self’ Using Immersive Virtual Reality View all 8 articles
Mental imagery practice is widely used to help athletes prepare for competitions, as it can produce motor actions that enhance performance. The goal of imagery training for athletes is to create realistic images in their minds and to familiarize them with certain procedures, environments, and other aspects related to competition. Traditional imagery training methods use still images or videos, and athletes study the pictures or watch the videos in order to mentally rehearse. However, factors such as distractions and low realism can affect the training quality. In this paper, we present a Virtual Reality (VR) solution and a study that explores our hypotheses that H1: high-fidelity VR systems improve mental imagery skills, that H2: the presence of elements such as virtual onlookers or photographers in the VR environment arouse stronger emotional reactions and affect, and that H3: the presence of elements such as onlookers or photographers in the VR environment results in better mental imagery skill improvement. For that purpose, seven elite snow sports athletes were exposed to three training methods, Video, VR-Empty, and VR-Crowded. Our results show that a VR simulation with virtual onlookers (VR-Crowded) can significantly increase heart rate, which can induce increased emotional arousal. The results from validated questionnaires show no significant difference for the three training methods in terms of mental imagery and affect, but the results show an ascending trend for the athlete’s arousal from Video to the VR-Crowded condition. Gaze detection heat maps of interest areas for the two VR conditions support hypothesis H2 that environmental factors such as the presence of photographers, staff, and onlookers can increase head and eye movement, possibly indicating an increase in emotional arousal during imagery training. According to verbal feedback and interviews, athletes are more likely to use innovative training methods (e.g., the high-fidelity VR method) than traditional video-training methods.
Mental imagery, sometimes colloquially referred to as visualizing, seeing in the mind’s eye, or imagining the feel of, is a cognitive process widely used by athletes as a performance-enhancing technique to facilitate motor actions (Cumming and Williams, 2012). Imagery as mental practice can positively affect skilled motor performance, especially when practice conditions are “optimal” (Barber, 1975). The results from Cumming and Hall (2002) indicate that professional, competitive athletes perceive imagery to be more relevant for performance than recreational athletes. Mental imagery training is effective not only for adults, but also children and adolescents Frank et al. (2023). Furthermore, training and practice have been shown to increase the functional representativeness of the image, moving this toward actual execution and hence, exerting a greater impact on performance (Frank et al., 2014). Importantly, the degree of functional representation, or functional equivalence (cf. Holmes and Collins, 2001) is another important contributory factor, highlighting the importance of both imagery stimulus and practice conditions Collins and Carson (2017).
Based on the stimulus, creating realistic images in the mind is one of the most important criteria that determines the quality of imagery training (Bedir and Erhan, 2020). Generally, athletes can produce realistic images using specific training methods, and the method used determines the quality of mental imagery training. However, current imagery-training methods rely mainly on mental training, and are commonly not supported by technology. We were approached by coaches of New Zealand elite athletes, and asked how technology might be used to help athletes improve their skills of mental imagery. In this paper, we present a Virtual Reality (VR) training method for mental imagery, explore factors that can affect mental imagery skills in VR, evaluate the approach with elite athletes, and discuss the positive and negative effects.
For our study, we collaborated with Snow Sports New Zealand (SSNZ), the New Zealand sporting organisation representing adaptive snow sports, alpine ski racing, cross country skiing, freeskiing and snowboarding, to support the preparation of Olympic ski and snowboard athletes. The research motivation is that some athletes reported that they feel nervous and stressed prior to performance. Factors such as expectations, performance outcome, and the pre-race environment (audience, weather, photographers, etc.) can affect their performance. Therefore, we built a VR snow sports environment to assist athletes in the development and subsequent rehearsal of their pre-performance routine to improve mental imagery skills. We explored the impact of different training methods based on that VR environment on mental imagery skills and affect. Participants of the study wore a VR headset and virtually moved themselves from the warm-up room to the start-gate of a course. Depending on the experimental condition, various types and levels of stimuli were presented along the way, including an audience, other athletes, and photographers in the VR environment.
The main goal of the study was to compare different visual stimuli in a VR-based imagery training method to determine if the conditions affect mental imagery skills, and how the presence or absence of others in the environment affects imagery ability. For this purpose, seven elite snow sports athletes were exposed to three training methods, Video, VR-Empty (see Figures 1A, B), and VR-Crowded (see Figures 1C, D).
FIGURE 1. VR training methods: (A) Warm-up tent of VR-Empty, (B) Corridor of VR-Empty, (C) Warm-up tent of VR-Crowded, (D) Corridor of VR-Crowded. Created with Unity 2020.3.26®. Unity is a trademark or registered trademark of Unity Technologies.
Work on the impact of imagery has stressed the importance of vividness and controllability (Holmes and Collins, 2001) whilst imagery use across sports has been shown to co-act with these variables (Gregg and Hall, 2006). In parallel, the personal relevance of image content has always been seen as important to engaging the athlete and enhancing his/her vividness through generation of stronger response and emotional propositions (Lang, 1979; Cumming and Williams, 2012). Indeed, imagery vividness as measured by psychophysiological concommitants has long been shown as related to individual variables, including the importance of the image and relevance to trait emotionality [e.g., (Budnik-Przybylska et al., 2023)] and modality (Wang and Morgan, 1992) On this basis, we expected to see greater engagement and vividness of imagery from the VR condition due to its increased facilitation of an internal perspective (as opposed to photos or video) and personal relevance by offering an accurate simulation of an upcoming“peak event” environment, namely, the Olympic Games. As such, the VR manipulation more effectively addressed the “why” component identified by Cumming and Williams (2012). Based on this, our hypotheses for the different training methods are as follows.
• H1: Using high-fidelity VR systems to display realistic 3D sports environments (VR-Empty & VR-Crowded) will improve mental imagery skills compared to still-image and video-based training methods (Video). Since immersive technologies provide more-visceral experiences, enhancing the sense of presence, we predict that this will allow people to more readily form mental images.
• H2: An environment with distracting elements (VR-Crowded) will arouse stronger emotional reactions and affect. We expect the crowded condition to be more like a real competition situation, since experienced participants would have stronger emotional reactions to crowds around them. In short, the more vivid and ‘accurate’ images will more effectively access memories of the real situation [cf. (Lang et al., 1980)].
• H3: An environment with distracting elements (VR-Crowded) will improve mental imagery skills more compared to an environment without distracting elements (VR-Empty). We believe the presence of crowds increases the realism of the experience, leading to an increase in improvement [cf. (Lang, 1979; Collins and Carson, 2017)].
Our results show that a crowded VR simulation with a rich set of stimuli can significantly increase heart rate, which signifies increased emotional arousal. Although the further results show no significant difference for mental imagery and affect, there is an increasing trend for athlete arousal from the Video to the VR-Crowded method. Gaze detection heat maps of interest areas for the two VR methods indicate that environmental factors such as the presence of photographers, staff, and an audience can increase head and eye movement, possibly indicating an increase in emotional arousal during imagery training.
The remainder of this paper first reviews related work on mental imagery skills and the use of immersive technologies in the sports field. We then present our study design and methods, including a description of the VR system. After presenting and discussing our results, we conclude the article with design recommendations and an outlook on future work directions.
The work presented in this article focuses on using VR for mental imagery ability improvement. We build on previous work from mental imagery in sports and the use of VR in sports training.
Mental imagery is a well known and widely used aid across performance domains. Indeed, its application is so ubiquitous that it could be described as the Swiss Army Knife of performance psychology. There is substantial literature supporting the efficacy of mental imagery and its many related applications, e.g., the learning of motor skills for dart throwing or basketball free throws, rehearsal of game plans in soccer or routines in figure skating, increasing the self-confidence of badminton players, or facilitating the adherence to rehabilitation programs Hall (2001); Martin et al. (1999); Simonsmeier et al. (2020).
Mental imagery training/intervention is able to help athletes for physical practice, which can effectively improve motor skills and performance in sports (Lindsay et al., 2021). Olsson et al. (2008) examined the use of imagery training for active high jumping athletes. They compared two groups of athletes to measure four variables: jumping height, number of failed attempts, take-off angle, and bar clearance. The results showed that there was a significant improvement in bar clearance for the group that trained with imagery, but not for the control group.
Weinberg et al. (2003) found that imagery for athletes is much more effective in closed sports (i.e., those which are much more stable and predictable) than in open sports (those which are more dynamic and unpredictable). In some closed sports, for example, high jump, it is much easier to know what to imagine because the athletes are likely to find it easy to prepare and imagine exactly what will take place. In open sports, athletes must be concerned with more factors that may impede their performance, such as the movements of the opposing team or whole body movements performed on a moving and unstable platform.
All applications share common features of mechanism. Importantly, the categorization of the purpose of the application of the imagery method has provided useful insights into the specific design and application of interventions. As one example, the Sport Imagery Questionnaire (Williams and Cumming, 2011) assesses imagery applications with a categorization of use across five domains, namely, cognitive specific, cognitive general, motivational specific, motivational general-arousal, and motivational general-mastery. One particularly pertinent application of this approach provides guidance on what types of imagery, and when and how interventions best suit athletes of different ages and developmental stages in particular sports, e.g., Gregg and Hall (2006).
Holmes and Collins (2001) stressed the importance of vividness and controllability of imagery applications. Practitioners need to seek optimal “functional equivalence” between the image and the real situation (Holmes and Collins, 2001) in that, when coupled with vividness and controllability, the performance impact of the imagery is greater. Notably, realistic emotional responses (both magnitude and valence) are important aspects. Consequently, the use of video or, as in this paper, VR, is increasingly sought after as a way to optimize this important component of athlete preparation. Furthermore, these impacts range from the general (e.g., better and more widely employable imagery skills) through to the specific, for example, “How can I get ready to meet the challenges of this competition?”
Studies in the sports field using VR have focused on three areas: virtual training (Adamovich et al., 2009; Calabrò et al., 2017; Akbaş et al., 2019), simulation development (Pereira et al., 2018), and performance analysis (Ouadahi et al., 2016; Neumann et al., 2018).
VR can provide a realistic simulation environment for the athlete in terms of training. Nozawa et al. (2019) designed an indoor training system for ski simulation which can be used to improve ski skills. Individualized training of technical and tactical concepts can be provided through VR, as well as motor abilities, regardless of the time and place, against a chosen opponent or in a specific situation (Akbaş et al., 2019). VR platforms allow the design of specific and complex training protocols, which are not feasible in real life (Argelaguet Sanz et al., 2015). Training routines can be designed to enhance particular motor skills and the training can be done progressively, for example, by increasing the level of difficulty of the task (Argelaguet Sanz et al., 2015).
Most sports training using VR is designed for motor skill improvement and only a few studies focus on the application of VR to sports psychology. VR can deceive the human brain’s predictive coding mechanism and create a real feeling of being present in the virtual body and space (Riva et al., 2019), which is a similar mechanism to mental imagery. Wellner et al. (2010) and Stinson and Bowman (2014) applied VR for pressure and anxiety management in sports training, as pressure and anxiety play important roles affecting sports performance (Ehrlenspiel, 2006).
In recent years, researchers have started to explore the relationship between using VR and the potential improvement of imagery training. Ross-Stewart et al. (2018) filmed a 360 video for NCAA baseball players, and played the video using VR goggles. Their findings indicate improvements in skill, goals and mastery imagery ability in both practice and competition. Bedir and Erhan (2020) designed a VR solution for training athletes in curling, bowling, and archery sports. The results show that VR-based imagery training athletes showed more improvement in a 4-week period than the athletes in the Visual Motor Behavior Rehearsal and Video Modeling group, in terms of both shot performance and imagery skills. This work also used recorded video played using VR headsets. Most of the work in imagery training is focused on finding the relationship between motor skills or performance and mental imagery, and seldom on sports competition preparation and mental rehearsal. To the best of our knowledge, no work so far has used an interactive, high-definition virtual environment for athletes to train their mental imagery abilities.
We implemented a controlled laboratory experiment to compare different visual stimuli in a VR-based imagery training method to determine if the conditions affect mental imagery skills, and how the presence or absence of others in the environment affects imagery ability. This study was approved by the Human Research Ethics Committee of the University of Canterbury, New Zealand.
We recruited seven elite athletes (four male, three female) from the national high-performance snow sports organization in New Zealand as participants for the study. These seven participants represent almost all of the Olympic-level snow sports athletes from New Zealand. Participants were aged 15–22 (M = 19, SD = 2.2). We collected descriptive data including VR experience, level of expertise for snow sports, and competition experience. All participants had previous VR experience with an average rating of (M = 1.85, SD = 0.35) on a 5-point Likert scale, from 1 (Not at all), 3 (Usually), to 5 (Everyday). From the demographic information, 85.7% (6/7) participants were “Expert” in terms of snow skiing/boarding, and one participant self-reported as “Proficient.” Reported previous snow sports competition participation was distributed between “Frequently” (85.7%) and “Usually” (14.3%).
The present study adopted a within-subjects design with one independent variable, Training Method: Video, VR-Empty, and VR-Crowded. The video in the Video condition was captured and recorded from VR-Crowded and presented to athletes on a computer screen in 2D and from a first-person view. In VR-Crowded, 69 non-playable characters (NPCs), 51 of which were audience members, 16 were members of the media, and 2 were time keepers at the start gate, were present in the environment. In addition, there was a large display screen with a “live feed” of the participant, and cheering sounds from the crowd. In VR-Empty, we removed the sounds and large display, as well as the audience and media NPCs, leaving only the 2 time keepers in the start gate. Therefore, VR-Empty was a less-distracting version, where athletes could get training with fewer external environmental distractors.
The simulation was built using Unity1, and was run on a desktop PC (Intel i7, RTX 2080s). For the VR methods, a Meta Quest 22 VR headset was used and tethered to the PC. Participants used the VR controllers and arm swinging to move their avatars. An Empatica E43 wrist sensor was used to capture heart rate and galvanic skin response, which was recorded and synchronized with a time stamp. Frame rate was maintained around 80–90 Hz within the VR headset to reduce the chances of VR cybersickness (LaViola, 2000; Stanney et al., 2020). While the experience was designed for standing, participants were encouraged to sit down for safety and stability reasons. Swinging their arms at their sides in a normal walking cadence would propel the avatar forward along a predefined path. During the experience, a number of factors were recorded, including time, participant head position and orientation, view direction, hand position and orientation, and the Empatica data.
The participant’s avatar was an athlete in a winter Olympic event starting scenario. This scenario was a simulation of a warm up routine that Olympic athletes must perform before they start their event. Visual reference materials were provided to us by the snow sports organization, and the resulting VR experience was verified by them as well. Participants utilised a VR headset to interact as a virtual avatar which was movement-constrained to a predetermined path within the scene. Depending on the condition, as the participant moved through the scene, the NPCs created the ambience of a high-intensity competitive event. All other environmental factors were identical for both VR methods.
Participants moved their avatar from the “warm-up tent” to the “Start-gate tent” along the path (see Figure 2). The experience ended when they reached the end of the Start-gate tent, just before dropping in for their run (see Figure 3). The avatar body orientation updated automatically based on the position along the path, though they were free to look around. If a participant physically rotated beyond 120° from their forward direction, the VR view faded to black, and faded back in to the environment, but the avatar would now be facing in the opposite direction. Cues were provided to the user on how to return to the base orientation. This technique allowed participants to turn around, while reducing cybersickness by handling the rotation internally while in black.
FIGURE 2. Avatar Path. Red = Warm-up tent, Yellow = Corridor, Green = Start-gate tent. Created with Unity 2020.3.26®.
The participant’s avatar was animated using a procedural system, composed of a torso and trunk, run by a head-motion algorithm. The arms were connected via an inverse-kinematic rig driven by the VR controllers, and the legs were also connected via an inverse-kinematic rig, but the walk cycle was directed by procedural instructions. Each step was triggered by head motion (i.e., lateral sway extents), but completed by ray-casting the terrain to find a step placement. This allowed the avatar’s motion to be controlled by the development team, so pace, gait, step, sway, among other parameters, could be set to balance a more-realistic experience with a safer one, and to combat cybersickness.
The 3D environment and assets were created using 3ds Max4. The terrain was created using 3D procedural terrain tools, while the trees and details were scattered using Unity’s internal terrain tools. Terrain and environmental assets, such as the ski jump features, tents, scaffolding, props, etc., were modelled and textured in Autodesk Maya5, then imported into Unity.
The weather effects were created in Unity using particle simulation systems, with parameters controlled by the environment control scripts. Snow particles could be made to blow faster and more laterally based on “Wind” parameters. The Sun’s orientation could be controlled by “Time of Day” parameters. NPCs were created using Autodesk Maya, and refined with Pixologic Zbrush6. Each NPC was rigged with an internal skeleton structure, then animated. Each NPC was categorized and sorted into a set (e.g., “audience,” “photographer,” etc.), and entities of these categories could be shown/hidden in the opening menu to manipulate crowd sizes. The audio system was composed of multiple sound sources placed around the scene to create a spatial soundscape. This included NPC cheering, talking, music, wind and weather. These sound sources could be removed based on menu settings to correspond with crowd size or wind level. For the study, each participant experienced the same time of day (early afternoon) and weather conditions (clear).
To evaluate our hypotheses H1 to H3, objective and subjective data were collected. Participants completed questionnaires after each condition, and the physiological data, such as Galvanic Skin Response (GSR) and Heart Rate (HR), were measured before and during the user study. The facing direction was recorded for analyzing areas of interest (heat map) when participants walked through to the Start-gate tent.
To assess the impact of the different conditions on mental imagery skills (H1, H3), we used the Sport Imagery Ability Questionnaire (SIAQ) from Williams and Cumming (2011). The SIAQ was designed to assess an athlete’s ability to image any sport-specific cognitive and motivational imagery content, and is applicable for any intervention in relation to sport-related images. The SIAQ is a 15-item questionnaire to assess five types of athlete imagery abilities: skill imagery ability, strategy imagery ability, goal imagery ability, affect imagery ability, and mastery imagery ability. Athletes were asked to image each item, and then rate how easy they could image each scenario in relation to skiing or snowboarding. Ratings were made on a seven-point Likert type scale: 1 = Very hard to image, 7 = Very easy to image. Participants rated items such as “The positive emotions I feel while doing my sport” and “Remaining confident in a difficult situation.” The ratings were a combination of how well the athlete could see the image and how well the athlete could feel the image. The SIAQ can be evaluated and scored in two different ways: 1) Separate subscales of imagery ability, and 2) a global measure of sport imagery ability.
To evaluate H2, we measured the positive and negative affect after using the different training methods using the Positive and Negative Affect Schedule (PANAS) (Watson et al., 1988). The PANAS is a 20-item questionnaire to assess two types of affect: positive affect (10 items), e.g., Interested or Excited, and negative affect (10 items), e.g., Nervous or Scared. For each item, participants needed to indicate the extent to which they felt them after using the training method. For this, PANAS uses a five-point Likert scale: 1 = Very slightly or not at all to 5 = Extremely.
Since HR and GSR have been shown to be correlated with imagery and anxiety (Jordan and Lenington, 1979), and to further evaluate H2, we collected both measures before and during the experiment using the Empatica E4. The photoplethysmography (PPG) sensor on the device collects HR at a 64 Hz sampling rate, and the electrodermal activity (EDA) sensor on the device collects GSR data at a 4 Hz sampling rate. Gaze-direction data was also captured using the forward direction of the headset, which was used to analyze the interest areas while walking from the warm-up tent to the start-gate tent.
We conducted a debriefing (Kriz, 2010; Crookall, 2014) with every participant after the experiment to connect their experiences with our observations, and to create a deeper understanding of their feedback. The researchers present at the experiments encouraged the athletes to comment on the simulation, reflect on their survey answers, and talk about their general impressions of the three different methods. We specifically asked in how far and which of the modes emotionally aroused them, on whether they considered the simulation useful for their pre-drop routine, which method(s) they preferred and why, and on whether they had any suggestions for improving the simulation. All debriefings were recorded on video for later analysis and review.
Participants were asked to read the information sheet and fill out an informed consent form once they arrived. Then they filled out a demographic questionnaire using a tablet. After that, the experimenter helped them put the Empatica E4 on their non-dominant wrist, and there was a 5-min break which allowed the participant’s physiological signals to settle so that the correct baseline data were collected during the idle time portion of the study.
After the break, verbal instructions were given using a script and they were guided to sit on a chair in the experiment area. The experimenter introduced the application and the experimental process, and explained how to use the devices involved in the study. The order of exposure to the three methods was randomized for each participant. If the first method was Video, the participants were asked to put on the headphones and watch the video clip. If the first method was VR-Empty or VR-Crowded, the experimenter helped them put on the VR headset, gave them the relevant devices, and let them get familiar with the system and interaction devices. Each method consisted of a single trial lasting about 10 min.
After each method, participants were required to fill out the questionnaires about their experience with the system. For the imagery questionnaire (SIAQ), the participants were asked to follow the following instruction, i.e., “For each item, bring the image to your mind with your eyes CLOSED. Then rate how easy it is for you to form this image. Please be as accurate as possible and take as long as you feel is necessary to arrive at the proper ratings for each image. There are no right or wrong answers, because we are simply interested in your response.” when answering each question. The experimenter then changed the configuration for the next method. Finally, participants were given one additional survey to gather information about their preference of training methods. The experimenter then performed the debriefing with the participants.
This section begins with a discussion of the pre-processing we did on the collected data to generate the variables we used in our statistical tests. Then we report the results of the statistical analysis of the objective data: HR and GSR. These results are followed by the heat map generated from the gaze-direction data, and analysis of subjective responses for the SIAQ, PANAS, and the user preference questions. We used the collected data sets from seven athletes. All statistics were computed using IBM SPSS Statistics7 v27. For statistical analysis, we adopted a One-way repeated-measures ANOVA test and Friedman test, and α = 0.05.
Each athlete has a unique cardio-pulmonary function, which means their physiological data (such as heart rate and galvanic skin response) may differ from one another. Therefore, their baseline data will also differ. Since the time taken for each athlete to complete the activity varied, we allowed the number of samples used for computing the averages to be different. This was based on whether the participant completed the entire experience or stopped early. The data were recorded in four sessions: once in a baseline session (recorded before the user study), and during the three method sessions. Therefore, the HR and GSR data each have two values, Pre and Post, for each method. For each subject, we first reduced the time-series data for each session to a single value for each variable (HR and GSR) by taking the mean. We then calculated the difference between Pre and Post for each resulting set of variables (HR and GSR) to get two new variables, Change in HR and Change in GSR.
In this section, we report on the results from the physiological signals HR and GSR, and from the gaze-direction heat map for interesting area.
A one-way repeated measures ANOVA was conducted to determine whether there were differences in Change in HR for the three different training methods. There were no outliers and the data was normally distributed, as assessed by boxplot and Shapiro-Wilk test (p > 0.05), respectively. The assumption of sphericity had not been violated, as assessed by Mauchly’s test of sphericity, χ2(2) = 0.6, p = 0.74. Change in HR was statistically different for the three methods,
The data was not normally distributed, as assessed by Shapiro-Wilk test (p < 0.05). A Friedman test was run to determine if there were differences in Change in GSR for the three different training methods, however the differences were not statistically significant, χ2(2) = 2.0, p = 0.36 (see Figure 5).
The gaze-direction data in terms of headset direction was recorded in both VR methods, and the experimenter recorded the focus point of athletes from the monitor during the study. In Figure 6, we present a heat map of focus points from athletes which reflects the area of interest when they walked from the Warm-up tent to the Start-gate tent. The Yellow and Red colors represent the areas of interest in VR-Empty and VR-Crowded, respectively. From the starting point (warm-up), the athletes showed more interest in other athletes that were gathered around watching the live broadcast on the TV. When they walked out on the way to the Start-gate tent, athletes were interested in the staff, photographers, and the live-streaming screen on the side in method VR-Crowded. In contrast, athletes focused more on the path in the VR-Empty method, as there were no other athletes or photographers around, and the live-streaming screen was turned off. The athletes still sometimes looked around, and looked at snow sports gear on the ground, the (blank) screen, and other facilities.
FIGURE 6. Heat map showing areas of interest for VR-Empty and VR-Crowded. Created with Unity 2020.3.26®.
This section focuses on the subjective data gathered from the SIAQ and the PANAS.
The SIAQ included an overall measure, Global Imagery Ability (Global), and five types of athlete imagery ability: Skill Imagery Ability (Skill), Strategy Imagery Ability (Strategy), Goal Imagery Ability (Goal), Affect Imagery Ability (Affect), and Mastery Imagery Ability (Mastery). The statistical results are shown in Table 2 and Figure 7. Global was calculated three times, once after using each of the three training methods, and the data were normally distributed, as assessed by boxplot and a Shapiro-Wilk test (p > 0.05). A one-way repeated measures ANOVA was conducted to determine whether there were differences in Global for the three methods. The assumption of sphericity was met, as assessed by Mauchly’s test of sphericity, χ2(2) = 0.84, p = 0.66. Global did not elicit statistically significant differences between the three training methods,
A Shapiro-Wilk test result (p < 0.05) indicated that the data was not normally distributed. A Friedman test was run to check for significant differences, however the differences were not statistically significant, χ2(2) = 0.69, p = 0.70.
There were no outliers and the data were normally distributed, as assessed by boxplot and Shapiro-Wilk test (p > 0.05), respectively. The assumption of sphericity was met, as assessed by Mauchly’s test of sphericity, χ2(2) = 1.9, p = 0.39. A one-way repeated measures ANOVA was conducted to determine whether there were statistically significant differences in Strategy Imagery Ability for the three different training methods, however the differences were not statistically significant, F(2, 12) = 1.4, p = 0.29,
A Shapiro-Wilk test result (p < 0.05) indicated that the data was not normally distributed. A Friedman test was run to check the significant differences, however the differences were not statistically significant, χ2(2) = 0.92, p = 0.63.
A Shapiro-Wilk test result (p < 0.05) indicated that the data was not normally distributed. A Friedman test was run to check the significant differences, however the differences were not statistically significant, χ2(2) = 1.04, p = 0.60.
The data were normally distributed, as assessed by the Shapiro-Wilk test (p > 0.05). The assumption of sphericity had not been violated, as assessed by Mauchly’s test of sphericity, χ2(2) = 0.98, p = 0.61. A one-way repeated measures ANOVA was conducted to determine whether there were statistically significant differences in Mastery Imagery Ability for the three different training methods, however the differences were not statistically significant, F(2, 12) = 0.97, p = 0.41,
The PANAS includes positive and negative parts. The results are shown in Figure 8 and the statistical data is shown in Table 3.
A Shapiro-Wilk test (p > 0.05) indicated that the data was normally distributed. The assumption of sphericity was met, as assessed by Mauchly’s test of sphericity, χ2(2) = 0.12, p = 0.94. A one-way repeated measures ANOVA was conducted to determine whether there were statistically significant differences in Positive Affect for the three different training methods, however the differences were not statistically significant, F(2, 12) = 0.75, p = 0.49,
A Shapiro-Wilk test (p > 0.05) indicated that the data was normally distributed. The assumption of sphericity was met, as assessed by Mauchly’s test of sphericity, χ2(2) = 0.45, p = 0.8. A one-way repeated measures ANOVA was conducted to determine whether there were statistically significant differences in Negative Affect for the three different training methods, however the differences were not statistically significant, F(2, 12) = 0.13, p = 0.88,
The athletes were asked to choose which of the VR-based training methods they preferred, and the results showed that 6 of the 7 athletes preferred the VR-Crowded training method. The one athlete who chose VR-Empty as the preferred VR method explained that she liked VR-Crowded more than VR-Empty, but the VR-Crowded method was too realistic, and the virtual people around the competition environment made her feel uncomfortable.
We performed a debrief and interview with each participant after completing all three methods, and the recorded debriefings were automatically transcribed. The resulting transcripts were reviewed by the researchers to collect feedback on the following subject areas: emotional arousal, usefulness of the training method, preferred training method and suggestions for improvement.
When reflecting on whether the simulation induced emotional arousal, 5 of 7 of the athletes confirmed that they were aroused by the VR methods.
Four of these athletes experienced a higher arousal in the VR-Crowded method, for example, stating “I did not know that it was going to be like this,” “It felt a lot more real than I thought. But yeah, kind of reminded me of most normal competition jitters.” or “They’re super close and you do not know what to do.” (when referring to the camera people).
One athlete reported a higher arousal in the VR-Empty method and stated “With nobody around that one made you feel like, I do not know, a bit more under pressure. Yeah. A bit more intense with just nothing going on.”
Finally, one athlete preferred the VR-Crowded method but overall did not perceive a difference between the three methods. For this highly experienced and multiple-medal winning athlete, their established mental preparation and pre-performance routine is to focus on the performance, and not to be distracted by external factors. In fact, this athlete is so well trained that mental preparation is part of the performance.
With regard to the usefulness of the training method, three athletes clearly indicated that they considered the simulation as helpful and could see the benefit in preparing for a real event. One athlete specifically pointed out that seeing the first feature of the course from the start gate helps visualize the whole course from training.
All 7 athletes preferred the VR-based methods. Several different reasons were mentioned for this. For example, one athlete said that it helped imagining, and continued “When I saw that, I had forgotten, what it is like, you know? And that’s the stuff that you miss. I think like that method is a lot better for that. All those extra things that happen and that you have to block out.” Another athlete clearly pointed to the added control that the VR methods offered, saying “I quite like that the VR method allows you to actually control and look around yourself and be in that moment yourself and not just watching somebody else.”
All suggestions for improving the training method, focused on how to improve the perceived realism of the VR-based methods. One athlete asked to include a waiting line in front of the start gate and actually see other athletes leave. Possibly, this could include unexpected delays, due to an accident happening on the course or a sudden weather change. Another athlete commented on the number of people cheering and said that there would normally not be such a crowd, and if at all there would be other athletes. Another athlete, though, said that there should be way more people cheering.
This section discusses the results in relation to our hypotheses.
• H1: Using high-fidelity VR systems to display realistic 3D sports environments (VR-Empty & VR-Crowded) will improve mental imagery skills compared to still-image and video-based training methods (Video).
• H2: An environment with distracting elements (VR-Crowded) will arouse stronger emotional reactions and affect.
• H3: An environment with distracting elements (VR-Crowded) will improve mental imagery skills more compared to an environment without distracting elements (VR-Empty).
We first discuss the objective data, followed by the subjective data analysis and interview results.
Imagery content influences autonomic responses, making it comparable to real activity (Deschaumes-Molinaro et al., 1992). Therefore, physiological changes during a mental-imagery activity might be similar to the changes in the actual real activity. Physiological data such as HR and GSR provide a picture of emotional and physiological states and have been shown to be correlated with effects of depression, anxiety, anger, and stress (Yoo et al., 2005), which might be induced during either mental or physical activity.
Jones and Johnson (1980) reported that high- and low-activity images were found to elicit clear differential cardiac responses, with significantly greater cardiac acceleration produced for high-activity images. The differences in Change in HR between VR-Crowded and Video, and between VR-Crowded and VR-Empty were significant, as shown in Figure 4.
The difference of Change in HR between VR-Empty and VR-Crowded is particularly interesting, as it supports H2. In VR-Crowded, the VR settings were different, in that it provided more environmental details to simulate a real snow sports competition. The athletes in the warm-up room were talking to each other, watching the live broadcast, and preparing for the competition. Once the participant walked out of the tent, the audience and photographers on both sides were watching the athlete walk from the Warm-up tent to the Start-gate tent. Cheering sounds and background music simulated the real competition, including the camera view on the big screen for live broadcasting. The results from Table 1 and Figure 4 meet our expectations, and show that environmental factors, such as an audience and photographers, provide additional stimuli that can accelerate the athlete’s heart rate.
The correct stimulus and simulation for mental imagery training play an important role that decides the quality of the training (Barber, 1975). The Change in HR of VR-Empty in Figure 4 is a turning point. The significant difference in HR change between VR-Empty and VR-Crowded is even more significant, compared to Video vs. VR-Empty. The Change in HR of Video is higher than VR-Empty (though not statistically so), indicating a trend that the environmental factors play an important role, as the video was recorded from the VR-Crowded method.
The GSR is an index for emotional arousal changes. The level of human emotional arousal changes in response to the surrounding environment (e.g., if something is scary, threatening, joyful, or otherwise emotionally relevant), and the subsequent change in emotional response that we experience, increases eccrine sweat gland activity. Previous research has shown the link between GSR and emotional arousal (Critchley, 2002; Salimpoor et al., 2009; Boucsein, 2012). Both positive (“happy” or “joyful”) and negative (“threatening” or “saddening”) stimuli can result in an increase in arousal, and in an increase in skin conductance. The GSR signal is therefore not representative of the type of emotion, but the intensity of it (Farnsworth, 2018).
Although the statistical differences for the three methods in Change in GSR were not significant, the results still provide trends supporting emotional arousal during mental imagery training. It is clear from Figure 5 that the trend of Change in GSR is ascending from Video through VR-Empty to VR-Crowded. This supports H2 and shows the intensity of emotions during imagery training. Both VR methods show strong emotional intensity changes because VR training provides an innovative and immersive training experience compared to the traditional video method. The athletes present either anxious/stressed, or excited/joyful responses during the VR methods, because the GSR index only reflects the intensity of the emotion, not the type of emotion, which might also explain why the Change in HR of VR-Empty is lower than Video, but the Change in GSR of VR-Empty is higher than Video.
The heat map shows the results for areas of interest, allowing for a comparison of the two VR methods. This analysis aims to investigate whether external environmental factors, such as the presence of photographers, can influence athletes during imagery training. The findings from the heat map reveal that athletes are prone to distractions caused by environmental factors, including other athletes in the warm-up room, staff members, and photographers positioned alongside the path. Notably, a majority of athletes directed their attention towards the prominent live-stream screen situated on the route to the Start-gate tent. This screen provided them with a third-person view of themselves as captured by the broadcast camera. In the case of the VR-Crowded method, athletes also found themselves drawn towards the sounds of cheering individuals lining the path. This auditory stimulus partially contributed to their focus on the staff members. Therefore, these observations once again support Hypothesis H2 and underscore the significance of the VR-Crowded method in introducing crucial environmental factors that can increase head and eye movement, possibly indicating an increase in emotional arousal during imagery training.
Although the differences between the three methods either in Global Imagery Ability or the five sub-imagery abilities were not significant, the results support H1 and show an increasing trend (see Figure 7) between the three methods such as the Global, Skill, Strategy, and Mastery. The average difference between VR-Empty and VR-Crowded in Affect (5 ± 1.33 to 6 ± 0.94) and Mastery (4.57 ± 0.88 to 5.05 ± 0.89) is greater than 1. The results support H3 and indicate that using high-activity VR solutions with environmental factors can help mental imagery training.
Supporting H2, the results from the PANAS show that the athletes reported both higher positive and negative affect after VR-Crowded compared to both the VR-Empty and Video methods. This is interesting, as Video and VR-Crowded offer the same amount of distracting elements. The only differences between VR-Crowded and Video are the display, i.e., 3D vs. 2D, and the fact that athletes in VR-Crowded were in control of their gaze and walking pace through the environment. We observed that some athletes in the VR-Crowded method were trying to interact with the virtual staff and other athletes along the path, such as waving their hands and trying to give “high fives.” In our opinion, this shows a higher sense of presence Witmer and Singer (1998), i.e., their subjective experience of being at that place with the audience while physically being at another, as compared to the Video method. Necessary conditions for presence in a virtual environment are being able to focus one’s attention on it Witmer and Singer (1998). This may lead to higher involvement and immersion. Future research will have to investigate if a virtual environment such as VR-Crowded leads to a higher sense of presence, and whether this increase leads to higher emotional arousal.
While all athletes preferred the VR methods for training, only 5 of the 7 athletes reported emotional arousal. One athlete reported no change in emotional arousal, but still preferred the VR-crowded method over the others. Considering the athlete’s extensive experience in competing in such events, we are of the opinion that our VR methods are specifically useful for more novice athletes with none or limited competition experience. A recent meta-analysis on the effect of imagery training for adolescents and children, though, did not find any difference in the effect with regard to expertise Frank et al. (2023). It is recommended that future research investigates the competition age of the athlete and its affect on the use of VR as a mental imagery enhancing tool.
It is interesting to note that, compared to the Video method, the VR methods supported a stronger feeling of really being there and being in control. The latter is specifically interesting, as the path the athletes walked from the warm-up tent to the start gate was predefined, and control was limited to looking around freely and deciding on the speed of movement using arm swing. Still, these little additional control possibilities seem to have positively impacted the perceived presence and mental imagery of the situation.
Finally, we received some conflicting suggestions on how to improve the realism of the simulation. One athlete suggested there should be more people cheering, while another suggested to reduce the number. This seems to be strongly related to the previous experiences of each athlete. As a result, it seems important that simulations be adaptable, able to be adjusted to the various situations and a specific athlete’s expectations for upcoming competitions. VR seems like a good solution for providing this flexibility and personalization.
Seven elite athletes participated in this study, who represented almost the complete team of high-performance snow sport athletes in New Zealand. Due to this low number of participants, only the Change in HR is partially supporting H2 in a statistically significant way. The rest of the results, and support for the three hypotheses, were not statistically significant. However, trends within the data did support the other hypotheses.
Within this elite athlete population, we wanted to explore the effect of VR mental imagery, and the high ecological validity of using elite athletes is a major plus here. More work is needed to better quantify the impact of imagery training using VR and to rule out that the VR methods were preferred due to their novelty and enhanced immersion. With the inherent difficulties of small population groups within elite sport, we therefore, see great benefit in this current study, as it has allowed us to increase the ecological validity well beyond using a more generalized subject pool (e.g., university students).
In this study, we also found some technical issues that need to be improved for future studies. The athletes used two controllers to control the virtual character from a first-person point of view. Moving was based on arm swing, and the angle between the upper arm and lower arm of the control rig might not have been natural, depending on the size of the actual athlete. We will add an arm calibration procedure to auto-adapt the virtual avatar’s arm length for the next study.
The locomotion method needs to be re-assessed, as some athletes reported that it would be more realistic if their actual movement matched the virtual movement. Therefore, tracking the movement of the feet of the participants could solve this issue.
The fact that we tested with elite athletes provided us with insights from the target group and we are confident to recommend VR with appropriate and realistic environmental settings for mental imagery training of athletes. It is an innovative method that can provide an immersive training experience and help them mentally prepare for real sports competitions. VR, as used within this study, can induce emotional arousal which is a key factor that can affect the quality of imagery training, and then potentially impact sports performance. Going forward, we recommend combining imagery training with other psychological tools, such as motivational interviewing, which might strengthen the positive impacts through increased personal salience [see, for example, Functional Imagery Training, (Rhodes and May, 2022)].
The added benefits of performing VR training lies in the ability to vary important elements of the environment, in order to play through “What if … ” scenarios. The current study only varied the makeup and size of the crowd in the warm-up area, as well as the impact media personnel have on athletes. Other important factors can also be readily manipulated, such as time of day, weather visibility, start delays (for example, due to crashes), and equipment malfunctions. By providing these “knobs” to coaches, athletes can experience and acclimate to many more events that can impose additional distractions, possibly degrading performance.
We developed a VR environment to train and improve mental imagery skills of elite snow sports athletes. We designed and implemented a user study to explore the impact of different visual stimuli on imagery ability, and measured their positive and negative affect. Physiological data such as heart rate and galvanic skin response were collected and gaze-direction data was recorded. A debriefing was held to better understand the feedback and experience of the athletes.
The results from the athletes show that a VR environment with the representation of an audience around the athletes can significantly increase heart rate, which indicates an elevation in emotion arousal. The results from questionnaires (SIAQ and PANAS) indicate positive trends and feedback using VR for mental imagery training, which partly supports our hypotheses. The gaze-direction heat maps of areas of interest for the two VR methods indicate that environmental factors in the VR environment, such as photographers, staff, and audience, can also induce emotional arousal and affect the mental imagery skills and positive affect of athletes.
According to feedback gathered during interviews with the athletes, they reported a clear preference for the VR training methods over the traditional video training method, as these offer a higher level of control. Also, it seems important to make these adaptable, as upcoming competitions might, for example, differ in the number of people in between the warm-up tent and the start gate, the length of this walk, or the visibility of the first feature from the start gate. We look forward to continuing this important journey of helping elite athletes reach their full potential through the improved, flexible, innovative training approaches we have employed.
The datasets presented in this article are not readily available because ethics approval requires all data to be stored on a secure and password protected machine within the HIT Lab NZ and to be securely destroyed after 10 years of the project. It further requires that the data is not made available to researchers outside the research team. Requests to access the datasets should be directed to SL, c3RlcGhhbi5sdWtvc2NoQGNhbnRlcmJ1cnkuYWMubno=.
The studies involving humans were approved by Human Research Ethics Committee–University of Canterbury, Christchurch, New Zealand. The studies were conducted in accordance with the local legislation and institutional requirements. Written informed consent for participation was not required from the participants or the participants’ legal guardians/next of kin because all participants were elite athletes of SnowSport NZ and the participation in the experiment and the later use of the system was part of their preparation for future competitions and signed off by their coaches.
YW conducted the user study, analysed the data and wrote the first draft of the article. SL co-designed the user study, managed the design of the VR system. SL, HL, and RL wrote sections of the manuscript. RM developed the VR system and wrote the system section of the paper. SF designed the visual elements of the VR system. CR and DC co-designed the user study and participated in the debriefing. All authors contributed to the article and approved the submitted version.
The development of the VR system used in this study was funded by High Performance Sports NZ.
We would like to thank the participants of our user studies for their help and feedback on our work. We would also like to thank the reviewers of our submission for their detailed and constructive feedback on earlier drafts.
Author DC was employed by Grey Matters Performance Ltd.
The remaining authors declare that the research was conducted in the absence of any commercial or financial relationships that could be construed as a potential conflict of interest.
All claims expressed in this article are solely those of the authors and do not necessarily represent those of their affiliated organizations, or those of the publisher, the editors and the reviewers. Any product that may be evaluated in this article, or claim that may be made by its manufacturer, is not guaranteed or endorsed by the publisher.
2https://www.meta.com/nz/quest/products/quest-2/
3https://www.empatica.com/en-gb/research/e4/
4https://www.autodesk.co.nz/products/3ds-max/
5https://www.autodesk.co.nz/products/maya/
7https://www.ibm.com/analytics/spss-statistics-software
Adamovich, S. V., Fluet, G. G., Tunik, E., and Merians, A. S. (2009). Sensorimotor training in virtual reality: A review. NeuroRehabilitation 25, 29–44. doi:10.3233/nre-2009-0497
Akbaş, A., Marszałek, W., Kamieniarz, A., Polechoński, J., Słomka, K. J., and Juras, G. (2019). Application of virtual reality in competitive athletes–a review. J. Hum. Kinet. 69, 5–16. doi:10.2478/hukin-2019-0023
Argelaguet Sanz, F., Multon, F., and Lécuyer, A. (2015). A methodology for introducing competitive anxiety and pressure in vr sports training. Front. Robotics AI 2, 10. doi:10.3389/frobt.2015.00010
Barber, T. X. (1975). “Ergogenic aids and muscular performance,” in American journal of clinical hypnosis. Editor william p. Morgan (New York, NY, USA: academic Press), 17, 279. doi:10.1080/00029157.1975.10403760
Bedir, D., and Erhan, S. E. (2020). The effect of virtual reality technology on the imagery skills and performance of target-based sports athletes. Front. Psychol. 11, 2073. doi:10.3389/fpsyg.2020.02073
Budnik-Przybylska, D., Syty, P., Kaźmierczak, M., Łabuda, M., Doliński, Ł., Kastrau, A., et al. (2023). Exploring the influence of personal factors on physiological responses to mental imagery in sport. Sci. Rep. 13, 2628. doi:10.1038/s41598-023-29811-6
Calabrò, R. S., Naro, A., Russo, M., Leo, A., De Luca, R., Balletta, T., et al. (2017). The role of virtual reality in improving motor performance as revealed by eeg: A randomized clinical trial. J. neuroengineering rehabilitation 14, 53–16. doi:10.1186/s12984-017-0268-4
Collins, D., and Carson, H. J. (2017). The future for PETTLEP: A modern perspective on an effective and established tool. Curr. Opin. Psychol. 16, 12–16. doi:10.1016/j.copsyc.2017.03.007
Critchley, H. D. (2002). Review: electrodermal responses: what happens in the brain. Neurosci. 8, 132–142. doi:10.1177/107385840200800209
Crookall, D. (2014). Engaging (in) gameplay and (in) debriefing. Simul. Gaming 45, 416–427. doi:10.1177/1046878114559879
Cumming, J., and Hall, C. (2002). Deliberate imagery practice: the development of imagery skills in competitive athletes. J. Sports Sci. 20, 137–145. doi:10.1080/026404102317200846
Cumming, J., and Williams, S. E. (2012). The role of imagery in performance Oxford, England: University of Oxford.
Deschaumes-Molinaro, C., Dittmar, A., and Vernet-Maury, E. (1992). Autonomic nervous system response patterns correlate with mental imagery. Physiology Behav. 51, 1021–1027. doi:10.1016/0031-9384(92)90086-h
Ehrlenspiel, F. (2006).Choking under pressure: attention and motor control in performance situations Ph.D. thesis. Potsdam, Germany: Universität Potsdam.
Farnsworth, B. (2018). What is GSR (galvanic skin response) and how does it work? https://imotions.com/blog/learning/research-fundamentals/gsr/#:∼:text=Galvanic%20Skin%20Response%20(GSR)%20is,arousal%20and%20other%20psychological%20processes.
Frank, C., Kluever, J., and Simonsmeier, B. A. (2023). Imagery training of motor actions in children and adolescents: A meta-analysis. Int. Rev. Sport Exerc. Psychol., 1–22. doi:10.1080/1750984X.2023.2167225
Frank, C., Land, W. M., Popp, C., and Schack, T. (2014). Mental representation and mental practice: experimental investigation on the functional links between motor memory and motor imagery. PLOS ONE 9, e95175. doi:10.1371/journal.pone.0095175
Gregg, M., and Hall, C. (2006). The relationship of skill level and age to the use of imagery by golfers. J. Appl. Sport Psychol. 18, 363–375. doi:10.1080/10413200600944140
Hall, C. R. (2001). Imagery in sport and exercise. 2nd edn. New York, NY, USA: John Wiley & Sons, 529–549.
Holmes, P. S., and Collins, D. J. (2001). The pettlep approach to motor imagery: a functional equivalence model for sport psychologists. J. Appl. sport Psychol. 13, 60–83. doi:10.1080/104132001753155958
Jones, G. E., and Johnson, H. J. (1980). Heart rate and somatic concomitants of mental imagery. Psychophysiology 17, 339–347. doi:10.1111/j.1469-8986.1980.tb00160.x
Jordan, C. S., and Lenington, K. T. (1979). Physiological correlates of eidetic imagery and induced anxiety. J. Ment. Imag.
Kriz, W. C. (2010). A systemic-constructivist approach to the facilitation and debriefing of simulations and games. Simul. Gaming 41, 663–680. doi:10.1177/1046878108319867
Lang, P. J. (1979). Emotional imagery: conceptual structure and pattern of somato-visceral response. Psychophysiology 16, 495–512. doi:10.1111/j.1469-8986.1979.tb01511.x
Lang, P. J., Kozak, M. J., Miller, G. A., Levin, D. N., and McLean, A. J. (1980). Emotional imagery: conceptual structure and pattern of somato-visceral response. Psychophysiology 17, 179–192. doi:10.1111/j.1469-8986.1980.tb00133.x
LaViola, J. J. (2000). A discussion of cybersickness in virtual environments. SIGCHI Bull. 32, 47–56. doi:10.1145/333329.333344
Lindsay, R. S., Larkin, P., Kittel, A., and Spittle, M. (2021). Mental imagery training programs for developing sport-specific motor skills: a systematic review and meta-analysis. Phys. Educ. Sport Pedagogy 28, 444–465. doi:10.1080/17408989.2021.1991297
Martin, K. A., Moritz, S. E., and Hall, C. R. (1999). Imagery use in sport: a literature review and applied model. Sport Psychol. 13, 245–268. doi:10.1123/tsp.13.3.245
Neumann, D. L., Moffitt, R. L., Thomas, P. R., Loveday, K., Watling, D. P., Lombard, C. L., et al. (2018). A systematic review of the application of interactive virtual reality to sport. Virtual Real. 22, 183–198. doi:10.1007/s10055-017-0320-5
Nozawa, T., Wu, E., and Koike, H. “Vr ski coach: indoor ski training system visualizing difference from leading skier,” in Proceedings of the 2019 IEEE Conference on Virtual Reality and 3D User Interfaces (VR), Osaka, Japan, March 2019, 1341–1342.
Olsson, C.-J., Jonsson, B., and Nyberg, L. (2008). Internal imagery training in active high jumpers. Scand. J. Psychol. 49, 133–140. doi:10.1111/j.1467-9450.2008.00625.x
Ouadahi, N., Chadli, S., Ababou, A., and Ababou, N. (2016). A simulator dedicated to strengthening exercises for windsurfers. Procedia Eng. 147, 532–537. doi:10.1016/j.proeng.2016.06.233
Pereira, M., Argelaguet, F., Millán, J. d. R., and Lécuyer, A. (2018). Novice shooters with lower pre-shooting alpha power have better performance during competition in a virtual reality scenario. Front. Psychol. 9, 527. doi:10.3389/fpsyg.2018.00527
Rhodes, J., and May, J. (2022). Applied imagery for motivation: a person-centred model. Int. J. Sport Exerc. Psychol. 20, 1556–1575. doi:10.1080/1612197X.2021.1987959
Riva, G., Wiederhold, B. K., and Mantovani, F. (2019). Neuroscience of virtual reality: from virtual exposure to embodied medicine. Cyberpsychology, Behav. Soc. Netw. 22, 82–96. doi:10.1089/cyber.2017.29099.gri
Ross-Stewart, L., Price, J., Jackson, D., and Hawkins, C. (2018). A preliminary investigation into the use of an imagery assisted virtual reality intervention in sport. J. Sports Sci. 6, 20–30. doi:10.17265/2332-7839/2018.01.003
Salimpoor, V. N., Benovoy, M., Longo, G., Cooperstock, J. R., and Zatorre, R. J. (2009). The rewarding aspects of music listening are related to degree of emotional arousal. PloS one 4, e7487. doi:10.1371/journal.pone.0007487
Simonsmeier, B. A., Androniea, M., Buecker, S., and Frank, C. (2020). The effects of imagery interventions in sports: A meta-analysis. Int. Rev. Sport Exerc. Psychol. 14, 186–207. doi:10.1080/1750984x.2020.1780627
Stanney, K., Lawson, B. D., Rokers, B., Dennison, M., Fidopiastis, C., Stoffregen, T., et al. (2020). Identifying causes of and solutions for cybersickness in immersive technology: reformulation of a research and development agenda. Int. J. Human–Computer Interact. 36, 1783–1803. doi:10.1080/10447318.2020.1828535
Stinson, C., and Bowman, D. A. (2014). Feasibility of training athletes for high-pressure situations using virtual reality. IEEE Trans. Vis. Comput. Graph. 20, 606–615. doi:10.1109/tvcg.2014.23
Wang, Y., and Morgan, W. P. (1992). The effect of imagery perspectives on the psychophysiological responses to imagined exercise. Behav. Brain Res. 52, 167–174. doi:10.1016/S0166-4328(05)80227-X
Watson, D., Clark, L. A., and Tellegen, A. (1988). Development and validation of brief measures of positive and negative affect: the panas scales. J. personality Soc. Psychol. 54, 1063–1070. doi:10.1037/0022-3514.54.6.1063
Weinberg, R., Butt, J., Knight, B., Burke, K. L., and Jackson, A. (2003). The relationship between the use and effectiveness of imagery: an exploratory investigation. J. Appl. Sport Psychol. 15, 26–40. doi:10.1080/10413200305398
Wellner, M., Sigrist, R., von Zitzewitz, J., Wolf, P., and Riener, R. (2010). Does a virtual audience influence rowing? Proc. Institution Mech. Eng. Part P J. Sports Eng. Technol. 224, 117–128. doi:10.1243/17543371jset33
Williams, S. E., and Cumming, J. (2011). Measuring athlete imagery ability: the sport imagery ability questionnaire. J. Sport Exerc. Psychol. 33, 416–440. doi:10.1123/jsep.33.3.416
Witmer, B. G., and Singer, M. J. (1998). Measuring presence in virtual environments: A presence questionnaire. Presence 7, 225–240. doi:10.1162/105474698565686
Yoo, H. J., Ahn, S. H., Kim, S. B., Kim, W. K., and Han, O. S. (2005). Efficacy of progressive muscle relaxation training and guided imagery in reducing chemotherapy side effects in patients with breast cancer and in improving their quality of life. Support. Care Cancer 13, 826–833. doi:10.1007/s00520-005-0806-7
Keywords: Virtual Reality (VR), mental imagery, positive and negative affect, sports training, elite athletes
Citation: Wu Y, Lukosch S, Lukosch H, Lindeman RW, McKee RD, Fukuden S, Ross C and Collins D (2023) Training mental imagery skills of elite athletes in virtual reality. Front. Virtual Real. 4:1189717. doi: 10.3389/frvir.2023.1189717
Received: 19 March 2023; Accepted: 31 July 2023;
Published: 14 August 2023.
Edited by:
Sameer Kishore, Middlesex University Dubai, United Arab EmiratesReviewed by:
Daniel Tony Bishop, Brunel University London, United KingdomCopyright © 2023 Wu, Lukosch, Lukosch, Lindeman, McKee, Fukuden, Ross and Collins. This is an open-access article distributed under the terms of the Creative Commons Attribution License (CC BY). The use, distribution or reproduction in other forums is permitted, provided the original author(s) and the copyright owner(s) are credited and that the original publication in this journal is cited, in accordance with accepted academic practice. No use, distribution or reproduction is permitted which does not comply with these terms.
*Correspondence: Stephan Lukosch, c3RlcGhhbi5sdWtvc2NoQGNhbnRlcmJ1cnkuYWMubno=
Disclaimer: All claims expressed in this article are solely those of the authors and do not necessarily represent those of their affiliated organizations, or those of the publisher, the editors and the reviewers. Any product that may be evaluated in this article or claim that may be made by its manufacturer is not guaranteed or endorsed by the publisher.
Research integrity at Frontiers
Learn more about the work of our research integrity team to safeguard the quality of each article we publish.