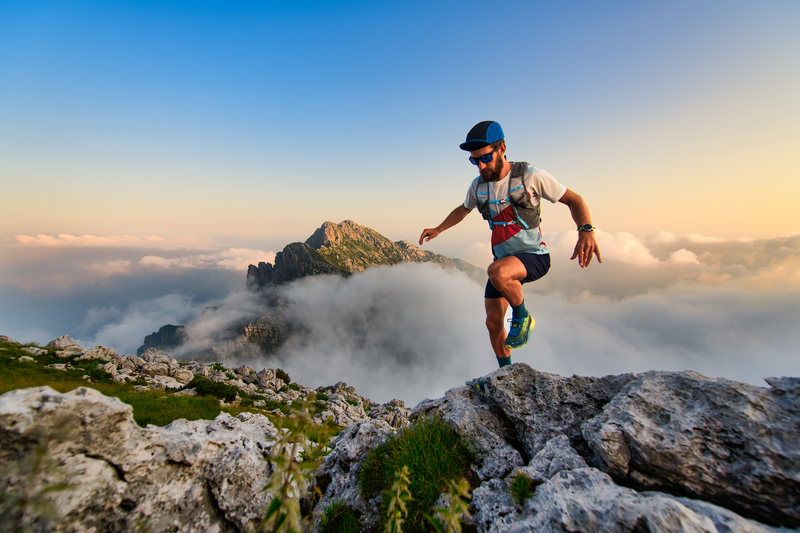
95% of researchers rate our articles as excellent or good
Learn more about the work of our research integrity team to safeguard the quality of each article we publish.
Find out more
ORIGINAL RESEARCH article
Front. Virtual Real. , 15 November 2022
Sec. Virtual Reality and Human Behaviour
Volume 3 - 2022 | https://doi.org/10.3389/frvir.2022.981625
This article is part of the Research Topic Human Spatial Perception, Cognition, and Behaviour in Extended Reality View all 13 articles
The frequent use of GPS-based navigation assistance is found to negatively affect spatial learning. Displaying landmarks effectively while providing wayfinding instructions on such services could facilitate spatial learning because landmarks help navigators to structure and learn an environment by serving as cognitive anchors. However, simply adding landmarks on mobile maps may tax additional cognitive resources and thus adversely affect cognitive load in mobile map users during navigation. To address this potential issue, we set up the present study experimentally to investigate how the number of landmarks (i.e., 3 vs. 5 vs. 7 landmarks), displayed on a mobile map one at a time at intersections during turn-by-turn instructions, affects spatial learning, cognitive load, and visuospatial encoding during map consultation in a virtual urban environment. Spatial learning of the environment was measured using a landmark recognition test, a route direction test, and Judgements of Relative Directions (JRDs). Cognitive load and visuospatial encoding were assessed using electroencephalography (EEG) by analyzing power modulations in distinct frequency bands as well as peak amplitudes of event-related brain potentials (ERPs). Behavioral results demonstrate that landmark and route learning improve when the number of landmarks shown on a mobile map increases from three to five, but that there is no further benefit in spatial learning when depicting seven landmarks. EEG analyses show that relative theta power at fronto-central leads and P3 amplitudes at parieto-occipital leads increase in the seven-landmark condition compared to the three- and five-landmark conditions, likely indicating an increase in cognitive load in the seven-landmark condition. Visuospatial encoding indicated by greater theta ERS and alpha ERD at occipital leads with a greater number of landmarks on mobile maps. We conclude that the number of landmarks visualized when following a route can support spatial learning during map-assisted navigation but with a potential boundary—visualizing landmarks on maps benefits users’ spatial learning only when the number of visualized landmarks shown does not exceed users’ cognitive capacity. These results shed more light on neuronal correlates underlying cognitive load and visuospatial encoding during spatial learning in map-assisted navigation. Our findings also contribute to the design of neuro-adaptive landmark visualization for mobile navigation aids that aim to adapt to users’ cognitive load to optimize their spatial learning in real time.
Imagine that your new colleague at work asks for directions from your workplace to the main train station. You will probably give route directions such as, “go straight until you see a school, then turn left,” or “turn right at the church.” Schools and churches are examples of prominent features in an environment known as landmarks. Landmarks are defined as “geographic objects that structure human mental representations of space” (Richter and Winter, 2014). They mark a salient feature in the environment and serve as points of reference that allow for spatial orienting and structuring of the environment (Presson and Montello, 1988). Ample evidence has shown that landmarks facilitate wayfinding efficiency (Wenig et al., 2017; Yesiltepe et al., 2021) and spatial memory of environments (Credé et al., 2019; Ligonnière et al., 2021).
Despite the long-standing literature on the importance of landmarks for human navigation (Richter and Winter, 2014), existing mobile navigation systems typically do not directly refer to landmarks when providing turn-by-turn wayfinding directions. The omission of landmarks in navigation systems could be one reason why navigation systems are often found to negatively affect spatial learning (Parush et al., 2007; Anacta et al., 2017; Wenig et al., 2017; Ligonnière et al., 2021). When guided by turn-by-turn directions, navigators tend to passively follow the given route shown on mobile maps and do not actively make spatial decisions (Fenech et al., 2010; Clemenson et al., 2021). Turn-by-turn directions drive navigators’ attention away from environmental features and lead to divided attention between navigation-assistive devices and the traversed environments (Gardony et al., 2013, 2015). Guided by such navigation devices, navigators are thus not supported in the active cognitive investment of encoding environmental information such as landmarks, route directions, and spatial relations of landmarks in the environment into memory (Parush et al., 2007; McKinlay, 2016; Dahmani & Bohbot, 2020; Sugimoto et al., 2021). As a consequence, overreliance on navigation systems may be detrimental to users’ spatial skills (Ishikawa, 2019; Ruginski et al., 2019). Considering the increasing reliance of navigators on mobile map applications in a digital age (Zenrin, 2017; Ishikawa, 2019), the importance of spatial learning abilities in healthy aging (Merhav and Wolbers, 2019; Ramanoël et al., 2022) and for education (Uttal and Cohen, 2012), there is a need to counter the negative effects of using GPS-based navigation systems on users’ spatial learning.
The inclusion of landmarks in mobile maps for pedestrian navigation has been proposed to counter the negative effects of using GPS-based navigation systems on users’ spatial learning (Raubal and Winter, 2002; Duckham et al., 2010; Liu et al., 2022). Indeed, Wunderlich and Gramann, (2021) showed that pedestrian navigation assistance that presents landmarks at intersections with verbal directions improved navigators’ spatial knowledge acquisition compared to standard navigation instructions in mobile applications that communicate turn-by-turn directions using metric distance information (e.g., “turn right in 200 m”). However, few studies have empirically examined the effects of landmark visualization in mobile maps on wayfinders’ spatial learning (Li, 2020; Münzer et al., 2020). Although landmarks can help users to process their environments for learning, they also require cognitive resources in users. The depiction of landmarks on mobile maps could therefore increase users’ cognitive load during an already demanding wayfinding task, especially in unfamiliar environments (Montello, 2005; Farr et al., 2012). Hence, excessive landmark information on mobile maps may possibly lead to users’ cognitive overload, considering their limited cognitive capacity (Baddeley, 2003). Cognitive overload may not only diminish the benefits of displayed landmark information in spatial learning but also may lead to decreased navigation efficiency (Münzer et al., 2012) and/or failure to acquire accurate spatial knowledge (Wen et al., 2013). Therefore, it is important to investigate how landmarks displayed on mobile maps affect cognitive load during navigation and spatial learning.
Cognitive load is defined as the total amount of resources being used in information processing in the present task (Sweller, 1988; Sweller et al., 1998; Baddeley, 2003). Cognitive resources are available for three types of cognitive load: 1) intrinsic cognitive load, which is associated with the nature of the task itself; 2) extraneous cognitive load, which arises when cognitive resources are used for irrelevant information; and 3) germane cognitive load, which is characterized as learning relevant information (Sweller et al., 1998). Indeed, the literature on cognitive capacity suggests that learning performance plateaus (or even drops) when the number of learning items exceeds learners’ limited cognitive capacity—typically four items (or chunks) (Luck and Vogel, 1997; Cowan, 2001; Baddeley, 2003; Alvarez and Cavanagh, 2004). Navigators’ cognitive load is thus an essential aspect to consider when visualizing landmarks on mobile maps; the number of landmarks should be displayed such that it optimizes spatial learning and wayfinding performance but not lead to overload.
Based on the previous literature on working memory capacity, we selected three, five, and seven landmarks as manipulations of low, medium, and high cognitive load in the present study, respectively. We used these three sets of landmarks as a starting point to investigate the potential impact of the number of landmarks shown on mobile map displays and how they affect cognitive load and spatial learning. Prior research that investigated cognitive load during navigation commonly used a dual-task paradigm where participants complete a working memory task while learning an environment during navigation. Cognitive load in such paradigms is assessed by either measuring the impact of the secondary (working memory) task on performance in the primary spatial learning task (Meneghetti et al., 2021) and/or using self-reports (e.g., NASA-Task Load Index, Hart and Staveland, 1988). However, self-reports are typically administered after participants have completed the navigation tasks and therefore do not capture cognitive load in real time while dual-task paradigms interrupt the spatial learning and navigation process.
We thus turned to electroencephalography (EEG), an established method to measure human electrocortical activity that allows for assessing brain dynamic features that might reflect cognitive load without interrupting the navigation task at hand. EEG measures neural activity with high temporal resolution (milliseconds) and thus captures the neural dynamics accompanying cognitive processes, such as spatial orienting (Gramann et al., 2010, 2021), spatial learning (Gehrke et al., 2018), visual processing (Wang et al., 2018), and memory processing (Onton et al., 2005; Maurer et al., 2015). Therefore, EEG is a more sensitive way to capture cognitive processes and their subcomponents (Cohen, 2014), compared to behavioral assessments and introspective self-reports.
Previous EEG studies have shown that one’s level of cognitive load is associated with power modulations in distinct frequency bands e.g., (Klimesch, 1999). Changes in EEG power reflect changes in synchronization of neuronal activity at different frequencies. Several frequencies in the EEG power spectrum, most notably the theta (4–8 Hz) and alpha (8–12 Hz) bands, have been associated with spatial navigation (Kahana et al., 1999; Bohbot et al., 2017; Do et al., 2021), memory processes (Klimesch, 1999; Klimesch et al., 2008; Sauseng et al., 2010), and attention (Pennekamp et al., 1994; Gevins and Smith, 2003; Sauseng et al., 2005; Doesburg et al., 2009). Specifically, a large body of research has found that theta frequency band power recorded over the frontal cortex increases in response to stimulus presentation (i.e., event-related synchronization, ERS), signaling increasing load during cognitive tasks (Smith et al., 2001; Jensen and Tesche, 2002; Gevins and Smith, 2003; Scharinger et al., 2017; Ratcliffe et al., 2022). ERS in the theta band in frontal regions has been proposed to reflect cognitive resource expenditure and relate to the integration and control of a variety of cognitive processes, such as visuospatial and verbal working memory (Sauseng et al., 2010). Previous research has also found that alpha frequency band power (8–12 Hz) in parietal regions decreases (i.e., event-related desynchronization, ERD) with increasing cognitive load (Stipacek et al., 2003; Doesburg et al., 2009). Decreased alpha power in parietal regions may indicate individuals’ maintenance of attention and working memory toward a focal task (Pfurtscheller et al., 1996; Sauseng et al., 2005; Fukuda et al., 2015) and a higher state of arousal (Carp and Compton, 2009).
Cognitive load also manifests through the modulation of components in event-related potentials (ERPs). ERPs refer to averaged time-varying EEG activity that is time-locked to a particular event during a task (Fu and Parasuraman, 2006). Of the ERP research on cognitive load, a large body has investigated the P3 component (Kok, 2001; Fu and Parasuraman, 2006; Polich, 2007; Ghani et al., 2020). The P3 component is a relatively large and slow positive deflection that appears approximately 300–800 ms after stimulus onset (Watter et al., 2001). The P3 has a maximum amplitude over the posterior cortex (Kok, 2001; Polich, 2007). Prior research has suggested that P3 amplitude reflects the demands of a task on cognitive resources (e.g., attention, working memory) and indicates the cognitive load of the task (Kok, 2001). Moreover, previous studies have shown that greater task complexity, higher stimulus complexity, and overall information provided to participants leads to more pronounced P3 amplitudes (Kok, 2001; Watter et al., 2001; Polich, 2007; Ghani et al., 2020). For example, in single-task/attention focus paradigms (Van der Stelt et al., 1998) and in focused compared to divided attention (Heinze et al., 1990), P3 amplitude was greater when participants paid attention to target stimuli compared to unattended stimuli. In dual-task paradigms, the P3 amplitude evoked by a secondary task is typically reduced when the difficulty of a primary task is increased, indicating a reallocation of processing resources away from the secondary task to the primary task (Kramer et al., 1991; Watter et al., 2001).
Taken together, the findings of previous studies using EEG point toward several possible indices of cognitive load. To investigate the changes in cognitive load affected by the number of landmarks, we thus examined theta oscillations at fronto-central leads, alpha oscillations at parieto-occipital leads, and P3 amplitude over the parieto-occipital cortex.
Considering that landmarks seen in the environment and visualized on maps include both visual features (e.g., color, shape, texture, etc.,) and spatial features (e.g., geometries, distance, density, etc.,) that are both essential for navigation support, it is particularly important to assess navigators’ visuospatial information processing during map-assisted navigation. Psychophysiological studies have suggested that theta oscillations in the occipital and parietal regions underlie visual and spatial information encoding, respectively. Increased theta oscillations in the occipital region have been frequently implicated in visual processing and selective visual attention (Gladwin and De Jong, 2005; McDermott et al., 2017; Wang et al., 2018). Similarly, increased theta oscillations in the parietal region have been found during computerized spatial tasks as well as spatial navigation tasks in naturalistic virtual environments (Plank et al., 2010; Delaux et al., 2021; Do et al., 2021). Research on alpha oscillations in the occipital region during visuospatial processing shows the opposite—alpha power has been found to decrease with increased visual attention (Klimesch et al., 1998; Nelli et al., 2017; Wang et al., 2018; Delaux et al., 2021).
ERP studies have found that early visual attention can modulate P1 (80–120 ms) amplitude (Fu and Parasuraman, 2006) in posterior areas as measured at occipital and parietal leads. The posterior P1 is related to early visual encoding of visual stimuli presented to viewers, and its amplitude in the occipital region has been shown to increase with greater visual attention allocation to these visual stimuli (Luck et al., 1990; Hillyard and Anllo-Vento, 1998; Awh et al., 2000; Handy et al., 2001; Fu and Parasuraman, 2006). To further investigate whether and how the number of landmarks on mobile maps affects visuospatial encoding, we thus examined theta and alpha oscillations as well as P1 amplitude at occipital sites.
Recording EEG during navigation in the real world is challenging, as EEG experiments usually require markers for event-related analyses of the signal. There is also little control over stimulus context and presentation (e.g., participants’ familiarity of the environment, mobile map consulting, etc.,) and external environmental factors (e.g., traffic, weather, etc.,) in real-world settings. We thus turned to virtual reality (VR), which reproduces real-life environments and can be used with EEG. VR displays three-dimensional (3D) dynamic images with high quality (Sanchez-Vives and Slater, 2005). VR can also integrate movement inputs by combining it with other interfaces, such as joysticks, foot pedals, or treadmills, which provides a more immersive experience and naturalistic sensory feedback (Gramann, 2013) compared to desktop-based experiments. With such unique characteristics, VR provides high ecological validity and is commonly employed in experiments that investigate navigation processes (Darken and Peterson, 2002). Indeed, studies have shown that spatial learning outcomes and cognitive load in virtual environments are fairly similar to spatial learning and cognitive load in the real world (Armougum et al., 2019; Clemenson et al., 2020; Pastel et al., 2022).
Furthermore, VR technology enables more control over experimental protocols. Researchers can create novel virtual cities with similar styles and manipulate augmented objects in the virtual environments while keeping other features in the environment constant. This is not possible in the real world. Therefore, in our current experiment, we employed VR technology to create three virtual urban environments with European-style architecture. We depicted three, five, and seven landmarks on mobile maps during navigation for each city. We also integrated VR technology with an EEG device to measure navigators’ brain activity during navigation in virtual environments.
We utilized a within-participant design with presentation of three different numbers of landmarks (three, five, and seven) to investigate how the number of landmarks displayed on a mobile map affects navigators’ 1) spatial learning, 2) cognitive load, and 3) visuospatial encoding when they were asked to follow a given route in an urban virtual environment. The current paper investigated cognitive load and visuospatial encoding in navigators and focused on electrocortical activity in the fronto-central, parieto-occipital, and occipital regions while they were viewing mobile maps. We tested the following hypotheses:
Spatial learning (H1): As the behavioral outcome of the load induction of landmark depiction, we expected navigators’ spatial learning to be better when the number of landmarks depicted on the map along a given route increases from three to five. Further, we expected that depicting seven landmarks along the route would generally exceed navigators’ cognitive capacities and thus counter the benefit of showing landmarks on the mobile map. Therefore, participants’ spatial learning performance is not expected to further increase or may even decrease when the number of landmarks increases from five to seven as a result of increased cognitive load.
Cognitive load (H2): Following the findings of previous studies (e.g., Gevins and Smith, 2003), we expected that when participants view the mobile map, 1) theta ERS would increase at fronto-central leads; 2) alpha ERD at parieto-occipital leads would be more pronounced; and 3) P3 amplitude at parieto-occipital leads would increase along with increasing numbers of landmarks shown on the mobile map. This is because cognitive load increases when navigators have to process more landmarks.
Visuospatial encoding (H3): We expected theta ERS in posterior leads to increase, and P1 amplitude and alpha ERD in occipital leads to be more pronounced during map viewing. This is because navigators need to encode more visuospatial information in the brain with increasing numbers of landmarks displayed on the mobile map.
We used a three-sided cave automatic virtual environment (CAVE) to simulate stereoscopic vision with frame sequential projection (1,280 pixel × 800 pixel resolution at 120 Hz frequency). Participants navigated through three virtual urban environments at 3.8 m/s using a foot-operated controller (3D Rudder, Aix-en-Provence, France). Tilting the controller with their feet towards the front and back resulted in forward and backward translation, respectively. Tilting the controller towards the left and right resulted in left and right rotation, respectively. Participants were allowed to turn their heads when navigating along the route. There are six cameras in the CAVE that track the head direction of participants through the donned 3D glasses. The perspective view in the virtual cities changes according to participants’ head direction. The three city models used for navigation were developed using ArcGIS City Engine 2018 (Esri, CA, United States). We employed three European-style urban environments including low-rise buildings with heights between 5 m and 25 m, streets, trees, and open spaces (Figure 1). The sizes of the three cities are 426′562 m2, 510′910 m2, and 516′868 m2, respectively. The experimental tasks were rendered using Unity 3D 2018.4 LTS (Unity Technologies; San Francisco, CA, United States) and MiddleVR for Unity 1.0 (Truchtersheim, France).
FIGURE 1. (A) Bird’s eye view of one of the virtual cities; (B) participants’ view of the environment during navigation; and (C) a participant wearing stereoscopic goggles, seated on a chair approx. 30 cm away from the center of the VR system (CAVE). The participant placed her feet on a foot-operated controller, which allowed her to navigate through the environment, and was connected to the EEG during the navigation experiment. The virtual city displayed on the CAVE screen was in mono-mode to present a first-person perspective of the virtual environments during navigation.
Participants completed three within-participant landmark conditions (three, five, and seven landmarks), which were visualized on a digital map while they navigated along a given route through three different virtual urban environments, respectively. The three navigation routes each consisted of five intersections with lengths between 800 m and 900 m. Each route further contained seven salient buildings that served as landmarks: the starting building (home), five landmarks at the five intersections, and the destination building (goal). Displaying visually salient buildings (e.g., of varying size, shapes, and colors, Itti and Koch, 2001) on mobile maps can help navigators to identify the buildings easily in the environment (Kapaj et al., 2021). Including visually salient buildings as landmarks on mobile maps can help reduce uncertainty and confusion, should there be a conflict between transient landmarks and turn directions displayed on the mobile maps (Gardony et al., 2015; Tenbrink et al., 2020).
In the 3-landmark condition, the start building, destination, and the salient building at the third intersection were displayed on the map (Figure 2A). In the 5-landmark condition, the landmarks at the first and fourth intersections were visualized on the map in addition to the three landmarks in the 3-landmark condition (Figure 2B). In the 7-landmark condition, the landmarks at the second and fifth intersections were visualized on the mobile map in addition to the five landmarks in the 5-landmark condition (Figure 2C). The selections of landmark positions for each landmark condition were done to ensure that the distributions of landmarks along the route were equally spaced. The assignment of the three landmark conditions to the three cities was counterbalanced. The order in which participants underwent the landmark conditions was also counterbalanced.
FIGURE 2. The three different landmark conditions in three different virtual environments. The (A–C) depict the map condition with three, five, and seven landmarks displayed on the mobile map, respectively.
Along each navigation route, participants were shown a mobile map at specific points along the route: in the middle of a straight segment of the followed route where the next intersection was visible; shortly before the next intersection; and shortly after the past intersection, resulting in 17 views of the mobile map in total (Figure 3A). The mobile map was displayed in the center of the VR screen for 5 s. It showed the current intersection and the route direction oriented with participants’ heading direction. It provided turn-by-turn directions (i.e., a black line) and participants’ current location (i.e., a blue dot), following the design of current navigation system displays (Figure 3B). Depending on the landmark condition, the respective 3D landmark at the intersections was visualized on the mobile map (Figure 3B). The landmarks visualized on the map are exactly like the landmarks seen in the navigated environments, including their first-person viewing perspectives along the route. To reflect a real-life scenario of mobile map consultation (Brügger et al., 2019), the virtual urban environment faded away when the map was displayed, and participants’ navigation through the virtual environment was disabled. The 17 map-onset events were used for event-related EEG analyses (i.e., ERP and frequency band power analysis).
FIGURE 3. (A) Red dots along the black navigation route indicate the 17 map pop-up spots during navigation; (B) a mobile map that rotates along with the participant’s head direction, as seen by the participant at the location of the green dot in panel (A). The blue dot in panel (B) indicates the participant’s current location in the virtual city. The black line indicates the path the participant needs to follow. A 3D landmark building or an imprint of the building is shown on the map at a turning intersection, depending on the landmark condition.
The experiment was conducted in German or English based on participants’ language preferences. After giving their informed consent, participants were introduced to the procedure of the experiment. Subsequently, they completed a questionnaire assessing their self-reported spatial and navigation abilities using the Santa Barbara Sense of Direction Scale (SBSOD; Hegarty et al., 2002) and their spatial orientation skills using the Perspective Taking/Spatial Orientation Test (PT/SOT; Hegarty and Waller, 2004) before they were connected to the EEG. Next, participants were given the instructions for the navigation task. Participants then practiced walking in a training virtual city with the 3D rudder and using the electronic pointing device to answer the spatial learning tests. After the training trial, once participants had no further questions, the main experiment started.
The main experiment consisted of three blocks. Each experimental block comprised a map-assisted navigation task and spatial learning tests. During the navigation phase, participants were asked to follow the route indicated on the map as quickly as possible to a specific destination, and to learn the landmarks along the route that were displayed on the map. In all three landmark conditions, participants were also told that some landmarks at the intersections that were not visualized on the map would be tested after navigation. Participants finished the navigation task when they arrived at the destination. We instructed participants to learn the landmarks and the environments intentionally, which simulates real-world scenarios when navigators attempt to learn the environments to make independent route decisions for future wayfinding.
After each navigation trial in each city, participants’ spatial knowledge acquisition, which was subcategorized as landmark knowledge, route knowledge, and survey knowledge (Siegel and White, 1975), were assessed with a landmark recognition test, a route memory test, and a judgment of relative direction (JRD) test, respectively (Figure 4).
FIGURE 4. Spatial learning tests: Panels (A), (B), and (C) illustrate how a participant responded in the landmark recognition test, the route direction test, and the JRD test respectively. All tests were carried out in the CAVE using a 3D pointing device.
After the main experiment, participants completed the Corsi block-tapping task (CBTT) on a computer to assess their visuospatial capacity.
Seven buildings that were seen along the route (i.e., starting building, destination building, and five buildings at the intersections) and that served as landmarks on the map, and seven buildings from the same traversed environment but not seen along the route were presented to participants. Participants were asked whether they had seen the building along the route and to answer with “yes” or “no” using an electronic pointing device (Figure 5A). Signal detection theory (SDT, Parks, 1966) was used to analyze participants’ landmark recognition performance (Huang et al., 2012; Wunderlich and Gramann, 2020, 2021). Buildings that served as landmarks along the route were considered as “signal” while buildings not seen along the route were considered as “noise.” D-prime (d’) indicates participants’ recognition discriminability where a higher d’ score reflects better discriminability in landmark recognition.
FIGURE 5. Landmark and route learning improved when more than three landmarks were shown. No improvement was observed in JRD performance when more landmarks were shown. The means of d’, choice accuracy, and absolute response error in each landmark condition are presented in the three plots with the error bars representing the 95% CI of the mean.
For buildings that participants answered “yes,” they were additionally asked which direction they took in reference to these buildings. Participants used the electronic pointing device to choose between “left,” “right,” “straight,” and “destination” (Figure 5B). “Destination” indicated that participants recognized the last building along the route, which signaled the end of the navigation task. This was explained to participants during the training trial. The paradigm of the landmark recognition and route direction tests were adapted from tests used in previous navigation studies (Huang et al., 2012; Wunderlich and Gramann, 2020, 2021). Performance on the route direction test was calculated as the percentage of correctly answered trials over the total number (seven) of landmarks.
The assessment of JRDs is a well-established method to assess acquired (metric) survey knowledge of navigators (Huffman and Ekstrom, 2018). Participants were asked to imagine standing at a first landmark while facing a second landmark and to point to a third landmark. For each JRD, participants pointed the electronic pointing device to the estimated direction of the third landmark and confirmed their decision by pressing a button. Each JRD consisted of three of the seven landmarks seen on the route (Figure 5C). Fourteen JRDs were pseudo-randomly selected out of the 35 possible trials. The seven landmarks appeared six times out of the 14 JRD trials. Pointing accuracy was defined as the absolute angular difference between estimated direction and the actual direction of a target landmark relative to a reference landmark (i.e., JRD error).
We conducted a power analysis for a mixed-effect model prior to the experiment. We estimated a small-to-medium effect size (d = 0.3–0.5) for the three within-subjects conditions with 14 JRD trials in each condition. The power analysis suggested testing 50 participants to obtain a statistical power of 73% for a small effect and 89% for a medium effect, respectively.
We recruited 49 participants (29 females) between the ages of 18 and 35 years (M = 25.6 years, SD = 4.09) for this study. One participant was excluded from analysis due to a self-reported mental ailment during the experiment and requested to have their data excluded. All participants were reimbursed 30 CHF for their participation. We conducted this study in compliance with the ethical standards of the University of Zurich Ethics Board, the Swiss Psychological Society, and the American Psychological Association.
Participants’ brain activity was continuously recorded using a 64-channel EEG device with active electrodes (LiveAmp, Brain Products GmbH, Gilching, Germany). The impedance of the channels was set below 10 kOhm. Electrodes were placed according to the extended 10% system (Oostenveld and Oostendorp, 2002). All electrodes were referenced to FCz with a ground electrode at Fpz. The EEG was recorded at a 500 Hz sampling rate. The raw EEG signal was streamed wirelessly via a BlueTooth adapter (UBT21) and was recorded continuously for the navigation task and the CBTT task. The EEG signal and all trigger events were synchronized using Windows Operating System’s interprocess communication (I.P.C.).
The BeMoBIL pipeline (Klug et al., 2022) was used to preprocess and clean the EEG data in the MATLAB (Mathworks, Inc.) toolbox EEGLAB (Delorme and Makeig, 2004). We first downsampled the raw EEG data to 250 Hz, and then removed spectral peaks at 50 Hz, corresponding to power line frequency, using the ZapLine Plus function (Klug and Kloosterman, 2022). Then, we applied the automated rejection function clean_artifacts from EEGLAB to identify noisy channels with ten iterations. We removed channels that were detected as bad channels more than four times and interpolated them by spherical interpolation of neighboring channels and applied re-referencing to the common average. On the cleaned dataset, we performed an independent component analysis (ICA) using an adaptive mixture independent component analysis (AMICA) algorithm (Palmer et al., 2011). Subsequently, for each resultant independent component (IC), we computed an equivalent current dipole model using DIPFIT routines from EEGLAB (Oostenveld and Oostendorp, 2002). We used the ICLabel algorithm (Pion-Tonachini et al., 2019) with the default classifier to classify the resultant ICs as eye, brain, or other components and removed ICs that reflected eye movements with a probability of 70% or higher. Next, we applied a 1–30 Hz bandpass filter to remove higher-frequency signals that are not relevant to our analysis. After these preprocessing steps, we excluded the EEG data from one participant because of severe artifacts.
We corrected the event latencies in wireless synchronization according to the projector (33 ms) and EEG trigger (100 ms) latencies, and then extracted 17 map-onset epochs from the continuous data with a time window of 0–5 s with respect to map onset and with a pre-event baseline of −1 to 0 s. We then performed an automatic epoch artifact detection and rejection using the function pop_autorej in EEGLAB: Epochs that fluctuated more than ±80 μV were excluded (Duncan et al., 2009). We used a probability threshold of three in standard deviation for the detection of improbable data. A maximum of 10% of total trials were rejected per iteration (five iterations in total). On average, we excluded 4.19% of all trials (0.7 out of 17 epochs) based on these criteria.
To examine the general effect of the number of landmarks on cognitive load while participants were consulting mobile maps during navigation, we averaged the map-onset events along each navigation route.
We selected the following regions of interest (ROIs): fronto-central (FC1, FCz, FC2), parieto-occipital (PO1, POz, PO2), and occipital (O1, Oz, O2) regions for the analyses in the time-frequency domain and ERPs. The ROIs and electrode clusters were chosen based on previous literature reporting maximal effects of cognitive load for theta-frequency band power in the fronto-central region and for alpha band power in the parietal-occipital region (Dong et al., 2015; Scharinger et al., 2017; Wei and Zhou, 2020), as well as visuospatial processing in the occipital and parieto-occipital regions (Handy et al., 2001; Wei and Zhou, 2020).
We calculated frequency band power using the function spectopo from EEGLAB, which uses Matlab’s pwelch function to calculate power spectral density (PSD). For PSD estimation, we used a 2-s Hanning window that led to a frequency resolution of 0.5 Hz to capture spectral changes in the EEG data. We set four frequency bands with the following frequency ranges: delta (1–3.9 Hz), theta (4–7.9 Hz), alpha (8–12.9 Hz), and beta (13–29.9 Hz), and computed the absolute spectra of the four frequency bands within the 0–5 s epoch with stimulus onset. To reduce inter-individual deviation, we computed relative power indices for each band (i.e., delta, theta, alpha, and beta) as power in a given frequency band relative to the entire bandwidth (i.e., 1–30 Hz) (Wang et al., 2015; Nishiyori et al., 2021) using the following formula:
Relative theta power = [absolute theta power/absolute power of (theta + alpha + beta + delta)]* 100
To obtain baseline power, we calculated relative power indices during the time before the navigation experiment started when participants were sitting on a chair and viewing a dark blue screen. We extracted baseline epochs with a length of 1 s from this pre-experiment phase. Baseline epochs had 200 ms overlap with subsequent epochs.
We then calculated ERD (negative values) and ERS (positive values) with respect to the pre-experiment baseline (Pfurtscheller and Lopes da Silva, 1999; Krause et al., 2000; Dong et al., 2015) for theta and alpha bands using the following formula:
ERD or ERS = (relative power during map-event - relative power during baseline)/relative power during baseline.
We corrected single-trial EEG data epochs with a pre-stimulus baseline from −200 to 0 ms. Based on visual inspections of ERP plots, we selected the following time windows: P1 (80–150 ms) at occipital sites and P3 (450–700 ms) at the parietal-occipital region for individual peak detection. Peak amplitude was calculated by taking the mean of the maximum peak value in the respective search windows and the neighboring +1 and −1 sample points (in total three data samples) (Wunderlich and Gramann, 2020).
To assess the effect of the number of landmarks on cognitive load and visual processing, we entered the theta ERS, alpha ERD, and peak amplitudes of P1 and P3 in R and ran for each parameter a linear regression model, with the α level set at α = 0.05 for all analyses. Multilevel/hierarchical modeling is a generalized form of regression analysis that enables hypothesis testing for nested study designs such as multiple trials within participants (Gelman, 2006) and for missing values in predictors (Fitzmaurice and Molenberghs, 2008).
We developed the multilevel models using the lmer4 package in R version 4.0 (Bates et al., 2011). To identify the maximal appropriate random effects structure that would converge, we included by-participant and by-item intercepts and slopes in the random structure based on a within-participants design. Subsequently, we simplified the maximal random effects model until it converged. Our multilevel model can be described with the following equation:
The mixed-effect regression follows a hypothesis-driven confirmatory approach and models the effect of the number of landmarks on cognitive load measured by EEG and on spatial learning. We dummy-coded categorical variables (i.e., condition: number of landmarks) to 0 and 1 for each contrast. We then fitted separate models for each EEG feature (i.e., theta ERS, alpha ERD, and ERP component amplitudes) and spatial learning outcomes (i.e., landmark recognition, route direction, and JRDs).
Participants spent on average 8.11 min (SD = 1.63 min) to navigate from the starting position to the destination in the three cities. There is no significant difference in navigation time between the three landmark conditions (ps > 0.507). In the following sections, we describe the results of spatial learning and EEG measures in detail.
Overall, the 48 participants produced 144 d’s, 144 route direction choices, and 1981 JRD responses. Due to technical reasons in Unity, 35 JRD responses were lost. The mean d’s was 1.83 (SD = 0.76), the mean percentage of correct route direction choice was 62% (SD = 0.26), and the mean of the absolute JRD response error was 72.64° (SD = 48.06°). For a complete overview of the results of the multilevel models, see Supplementary Table S1 in the supplementary materials.
The multilevel regression models reveal significant effects of the number of landmarks on landmark recognition and route learning. For the landmark recognition task, recognition discriminability d’ increases by 0.51 when the number of landmarks increases from three to five (β = 0.51, 95% CI [0.30, 0.72], p < 0.001). There is no further increase in landmark recognition discriminability from five landmarks to seven landmarks [β = −0.11, 95% CI (−0.32, 0.10), p = 0.31]. D-prime increases by 0.4 when the number of landmarks increases from three to seven [β = 0.40, 95% CI (0.19, 0.61), p < 0.001].
For the route direction choice task, we find a similar pattern for the number of landmarks. Route direction memory significantly increases by 12% on average from three landmarks to five landmarks [β = 0.12, 95% CI (0.57, 0.67), p < 0.001], and does not improve further from five landmarks to seven landmarks [β = −0.02, 95% CI (−0.09, 0.06), p = 0.71]. Route direction memory also significantly improves by 10% on average from three landmarks to seven landmarks [β = 0.10, 95% CI (0.02, 0.18), p = 0.01].
For the JRD performance, the linear mixed effect model revealed no significant main effect of the number of landmarks (ps > 0.68). Figure 5 displays the relationship between the number of landmarks and spatial learning (i.e., landmark recognition, route direction knowledge, and JRDs).
The multilevel linear regression models reveal that theta ERS in the fronto-central region is significantly greater in the 7-landmark condition compared to theta ERS in the 3- and 5-landmark conditions [7 vs. 3: β = 0.07, 95% CI (0.01, 0.14), p = 0.026; 7 vs. 5: β = 0.10, 95% CI (0.04, 0.16), p = 0.002]. We do not observe significant differences in theta ERS in the fronto-central region between the 3-landmark and 5-landmark conditions [5 vs. 3: β = −0.03, 95% CI (−0.09, 0.04), p = 0.391].
We do not find statistically significant differences in alpha ERD in the parieto-occipital region between the three different landmark conditions [5 vs. 3: β = −0.03, 95% CI (-0.09, 0.02), p = 0.220; 7 vs. 3: β = −0.04, 95% CI (−0.10, 0.01), p = 0.120; 7 vs. 5: β = -0.01, 95% CI (−0.06, 0.05), p = 0.738].
Supporting our hypothesis, theta ERS in the parieto-occipital leads increases significantly with increasing numbers of landmarks [5 vs. 3: β = 0.06, 95% CI (0.01, 0.11), p = 0.027; 7 vs. 3: β = 0.15, 95% CI (0.10–0.20), p < 0.001; 7 vs. 5: β = 0.09, 95% CI (0.04, 0.14), p = 0.001]. The same pattern of theta ERS is observed in the occipital region [5 vs. 3: β = 0.06, 95% CI (0.01, 0.11), p = 0.027; 7 vs. 3: β = 0.15, 95% CI (0.10, 0.20), p < 0.001; 7 vs. 5: β = 0.08, 95% CI (0.02, 0.14), p = 0.008].
Alpha ERD in the occipital region is smallest in the 3-landmark condition and significantly smaller compared to the 5- and 7-landmark condition [5 vs. 3: β = -0.09, 95% CI (−0.16, −0.03), p = 0.006; 7 vs. 3: β = -0.07, 95% CI (−0.14, −0.01), p = 0.031], which is again in line with our hypothesis. No significant difference is observed between the 5-landmark and 7-landmark condition [7 vs. 5: β = 0.02, 95% CI (−0.05, 0.09), p = 0.541].
Figure 6 below depicts the averaged theta ERS and alpha ERD across the three landmark conditions. Supplementary Table S2 in the supplementary materials presents a complete overview of the results of the multilevel models for ERD/ERS.
FIGURE 6. Mean ERD/ERS values of the map-event window (i.e., 0–5 s) for mean frontal-central theta ERS and mean parieto-occipital alpha ERD indicating cognitive load changes (top panel), and mean parieto-occipital theta ERS, occipital theta ERS and occipital alpha ERD indicating visuospatial encoding (bottom panel). Error bars indicate ±1.96 standard error (i.e., 95% CI) of the mean. Means in the 3-landmark condition are depicted in black, serving as a baseline in each violin plot. Significant differences between means at p < 0.05 are shown with different colors within the same violin plot. Means presented in the same color within the same violin plot indicate no significant difference between the means at p ≥ 0.05.
The linear mixed-effect models reveal that P3 amplitude in the parieto-occipital region in the 7-landmark condition is significantly greater than in the 3- and 5-landmark conditions, which is in accordance with our hypothesis. P3 amplitude increases by 143% on average from the 3-landmark to 7-landmark condition [7 vs. 3: β = 1.43, 95% CI (0.47, 2.39), p = 0.004] and by 139% on average from the 5-landmark to 7-landmark condition [7 vs. 5: β = 1.39, 95% CI (0.44, 2.35), p = 0.004]. We do not find a significant difference between the 3- and 5-landmark conditions [5 vs. 3: β = 0.04, 95% CI (−0.92, 1.00), p = 0.936].
We do not find any significant difference between the three conditions in P1 amplitude in the occipital region and in the parietal-occipital region (ps > 0.139).
Figure 7 plots the group-mean amplitude of the ERPs and the detected peak amplitude for each landmark condition. Supplementary Table S3 in the supplementary materials presents a complete overview of the results of the multilevel models of the ERPs.
FIGURE 7. (A,B) Grand averaged amplitudes of ERPs for each landmark condition at (A) parieto-occipital leads (PO3, POz, PO4), and (B) occipital leads (O1, Oz, O2). The ERP signals served as the basis for individual peak detection—areas shaded in gray indicate the time window where (A) the P3 (450–700 ms), and (B) P1 (80–150 ms) were extracted for each participant. (C,D) Violin plots display the distribution of detected peak amplitudes together with mean and ±1.96 standard error (i.e., 95% CI) in each landmark condition for (C) parieto-occipital P3, and (B) occipital P1. The line plot in the top panel and means in the bottom panel highlighted in purple indicate significant differences at p < 0.05.
In the current paper, we set up to examine cognitive load and visuospatial encoding measured with EEG in a naturalistic, map-assisted urban navigation experiment, carried out in virtual reality. We were driven by the research question of whether and how varying the number of landmarks displayed along a route on a mobile map during navigation would affect navigators’ spatial learning, cognitive load, and visuospatial encoding. We found that navigators’ landmark and route learning improve without neurophysiological indications of increased cognitive load; that is, we do not find a significant increase in frontal theta ERS or parieto-occipital P3 amplitude when the number of landmarks increases from the lowest number (three) to a medium (five) number of landmarks, which does not support our hypothesis of cognitive load. However, cognitive load increases significantly when the maximum assessed number of landmarks (seven) is shown on the mobile map as indicated by increased theta ERS and increased P3-amplitudes of the event-related potential, which is in line with our cognitive load hypothesis. This might explain why we do not find further benefits in navigators’ spatial learning: Navigators experienced cognitive overload in the 7-landmark condition and could not improve their spatial learning beyond a medium level of cognitive load. The results partly support our initially stated hypotheses informed by prior research (based mostly on stationary studies using artificial stimuli) on cognitive capacity and learning, cognitive load, and visuospatial encoding in the context of navigation and wayfinding. In the following sections, we discuss our main results in more detail.
Our current finding that spatial learning performance appears to plateau at five (medium number) landmarks shown on a mobile map in a map-assisted navigation task suggests that for most participants, their cognitive capacity was saturated at five landmarks, which is in line with our hypothesis of spatial learning. This finding is consistent with prior research on cognitive capacity suggesting that learning performance reaches a plateau after the learnt items exceed capacity (Luck and Vogel, 1997; Baddeley, 2003). In our current navigation study, following a given route through an environment is associated with intrinsic cognitive load as the navigation task (i.e., controlling the foot pedal to steer body movement) had to coordinate with the changing visual information provided by the environment and the landmark learning task, thus rendering the navigation task a dual- or multiple-task setting. Increasing the number of depicted landmarks from three to five benefits spatial learning and possibly induces germane cognitive load due to the relevance of landmarks to the spatial learning task at hand. Depicting landmarks on mobile maps at intersections may make navigators more aware of and let them pay more attention to landmarks at navigation decision points and landmark-based actions, which in turn leads to better spatial memory of the traversed environment (Gardony et al., 2013). However, increasing the depicted landmarks from five to seven does not further improve spatial learning, possibly due to overload in germane cognitive load.
The effects of the number of shown landmarks can be generalized to landmark and route knowledge but cannot be applied to survey knowledge. In our current study, participants’ JRDs demonstrate high pointing errors. JRD performance in the current study is poorer than the performance reported in most prior related studies e.g., (Zhang et al., 2014; Credé et al., 2020). One possible explanation for this is that navigating in a novel environment only once is not sufficient for most navigators to obtain reliable configurational layout knowledge (Frankenstein et al., 2012; Huffman and Ekstrom, 2018). Previous studies have found that JRDs improve significantly with increasing exposure to the environment (Zhang et al., 2014; Huffman and Ekstrom, 2018). Another possibility for the poor JRD performance is the lack of body-based cues during navigation in our current experiment, such as motor, vestibular, and proprioceptive cues (Gramann, 2013). All of these body-based cues have been known to facilitate survey knowledge acquisition (Chrastil and Warren, 2013).
Previous cognitive load research found a relationship between increasing task demands and increasing frontal midline theta ERS (Krause et al., 2000; Maurer et al., 2015; Scharinger et al., 2017). Similarly, we found that fronto-central midline theta increases with the number of landmarks visualized on a mobile map during navigation in visually complex urban VR environments.
Our finding that parieto-occipital P3 amplitude increases with increasing numbers of landmark depictions is also consistent with previous literature. It was shown that parieto-occipital P3 amplitude increases along with increasing demands on cognitive capacity (Kok, 2001; Polich, 2007; Scharinger et al., 2017; Wei and Zhou, 2020). Importantly, our findings on frontal theta power converge with those of parieto-occipital P3 amplitude. They are aligned with prior research demonstrating that more pronounced theta power occurs along with more pronounced P3 amplitude during cognitive tasks (Spencer and Polich, 1999; Scharinger et al., 2017; Wei and Zhou, 2020). By demonstrating a similar effect on parieto-occipital P3 amplitude and fronto-central theta ERS in the context of navigation and wayfinding, our research extends the existing literature on cognitive load and cognitive capacity, which were conducted mostly in highly controlled laboratory settings using simple stimuli. However, we did not observe differences in alpha ERD between the three landmark conditions. This could be attributed to conflicting relationships found between alpha ERD and cognitive load in recent years (Palva and Palva, 2007; Jensen and Mazaheri, 2010). It has been proposed recently that alpha ERS and alpha ERD might support two different cognitive mechanisms—attention orientation and attention maintenance, respectively (Capilla et al., 2014; Puma et al., 2018). As our current experimental design does not distinguish between these two mechanisms, they might have occurred at the same time in our extracted alpha power oscillations during map viewing and the two processes might have canceled each other out.
Notably, theta ERS is determined not only by the amount of displayed information that needs processing but also by the cognitive effort being spent to complete a cognitive task (Onton et al., 2005). Similarly, P3 amplitude is related to not only intrinsic task demands, but also to the internal expenditure of cognitive resources on the task at hand (Näätänen, 1992; Kok, 2001). Our results in the context of navigation and wayfinding show no increase in cognitive load when the number of landmarks increases from three to five, suggesting that the medium amount of chosen landmarks (five) does not tax additional cognitive resources, compared to showing the lowest evaluated amount of landmarks (three). This pattern differs when the highest number of landmarks (seven) was shown. We found increased cognitive load and thus that more cognitive resources were indeed consumed, compared to the other two landmark conditions.
Taking the findings of spatial learning outcomes and cognitive load together, it appears that showing five landmarks on a mobile map improves spatial learning performance compared to just showing three without significantly taxing additional cognitive resources. Presenting seven landmarks, and thus more information on a mobile map to navigators, however, does not further improve spatial learning, probably due to cognitive overload. Our research thus identified a potential boundary condition to the proposed benefit of visualizing landmarks in mobile maps on spatial learning—visualizing landmarks on maps benefits users’ spatial learning only when the number of visualized landmarks shown does not exceed users’ cognitive capacity during navigation. As such, this paper contributes to the ongoing debate in the field of map-assisted navigation by showing, on the one hand, that landmarks have an important role because they do facilitate spatial learning (Liao et al., 2017). On the other hand, we show that depicting increasing numbers of landmarks on mobile maps does not necessarily lead to corresponding increases in spatial learning, and that the role of landmarks in navigation and spatial learning should not be overly exaggerated (Montello, 2017). Future work can further examine other potential boundary conditions to the landmark effect on spatial cognition during navigation. To further ensure that our findings on EEG/ERP modulations reflect cognitive load in navigation contexts, future studies should also combine other instruments for measuring cognitive load, such as self-reports on cognitive load using the NASA-TLX questionnaire (Hart and Staveland, 1988) and pupil diameter assessments with mobile eye trackers (Krejtz et al., 2018).
Our finding of increased theta ERS at parieto-occipital leads with increasing numbers of depicted landmarks, which is in line with our hypothesis of visuospatial encoding, replicates the results of previous literature on posterior theta oscillations during spatial navigation in urban virtual environments (Fischer et al., 2020; Do et al., 2021). Our results on theta ERS and alpha ERD at occipital leads are also congruent with the literature on visual stimuli encoding and visual attention (Wang et al., 2018; Delaux et al., 2021). These findings together suggest that when a greater number of landmarks is available for visuospatial encoding on a mobile map during navigation, brain activity related to visuospatial encoding increases similarly. However, an increase of available visuospatial information on mobile maps does not necessarily lead to an increase in cognitive load. This, therefore, extends the cartographic literature interested in the relationship of how visual information on maps is presented to map viewers and how this affects visual processing demand (Garlandini and Fabrikant, 2009), cognitive load (Bunch and Lloyd, 2006), decision making (Korporaal et al., 2020), and spatial behavior (Brügger et al., 2019). Specifically, our findings provide further insights to the field of cognitive cartography regarding the way in which landmark depiction is visually processed by map users during map-assisted navigation, and how this visual information can assist navigation and spatial learning. Our findings on visuospatial processing of map information and cognitive load also contribute to the literature on mobile maps by emphasizing that different cognitive processes occur during map-assisted navigation (Montello, 2002; Lobben, 2004) and spatial learning (Allen, 2003). These two different cognitive processes should be considered separately when designing cognitively supportive mobile maps.
The current work provides the first evidence for the impact of the number of landmarks visualized in a mobile map on cognitive load and visuospatial encoding during navigation and wayfinding in virtual urban environments. The use of three vs. five vs. seven landmarks based on classic studies on cognitive load theory e.g., (Baddeley, 2003), turns out to be a very useful stepping stone for further research on the role of landmarks in map-assisted wayfinding. The current findings need to be considered together with our specific navigation settings, including the route following task, the map style, the route length, the number of traversed intersections, and the chosen types of 3D landmarks (i.e., point features). Future studies following our paradigm could examine the relationship between spatial learning and cognitive load without depicting landmarks on a mobile map, which would resemble the state-of-the-art of available navigation systems, or presenting beyond seven landmarks. Another series of studies could, for example, examine how the length of a route to be followed affects cognitive load and spatial learning.
Based on our chosen experimental set up, we could assess only 17 map onset events for each participant and per condition, even though we used a within-participant design to control for inter-subject variability in brain activity and to increase statistical power. Classic prior research with desktop setups have typically used a large number of repetitions of a given event (such as stimulus presentation) to measure cognitive processing. However, using such a method to increase the numbers of events for analysis can be challenging in naturalistic settings (Wascher et al., 2014). In the case of our pedestrian wayfinding paradigm, showing a mobile map repeatedly could directly interfere with navigators’ wayfinding performance (i.e., trying to avoid obstacles, etc.,). To alleviate this problem, future studies could try using eye blinks as events as they are self-generated by navigators (Wascher et al., 2014; Wunderlich and Gramann, 2020) and therefore do not interrupt the navigation task. Another option would be to employ a VR-system coupled with an eye-tracking system that could be leveraged to generate large numbers of eye fixation events for a fine-grained cognitive load analysis while maintaining high ecological validity.
Our current empirical research on the effect of landmark visualization on cognitive load and visuospatial encoding further exemplifies the important role of landmarks in map-assisted navigation and wayfinding. Specifically, varying the number of landmarks depicted on mobile maps used in a naturalistic VR navigation setting was found to affect spatial learning as well as cognitive load and visuospatial information encoding measured by EEG. Moreover, our findings also suggest a potential boundary effect to the proposed benefit of depicting landmarks on mobile maps during navigation on spatial learning. To support effective (i.e., accurate) spatial learning, a mobile map with a medium number of landmarks (i.e., five landmarks) seems to be optimal for spatial learning without overtaxing cognitive resources. Alternatively, showing a low number of landmarks (i.e., three landmarks) compared to five landmarks leads to worse spatial learning. Showing a high number of landmarks (i.e., seven landmarks) compared to five landmarks does not further benefit spatial learning while taxing more cognitive resources. By examining the effect of increasing the number of landmarks depicted on mobile maps on cognitive load and visuospatial encoding in a naturalistic navigation task, the present research synthesizes the fields of navigation research, mobile map design, neuroergonomics, and spatial cognition with implications for the development of brain-machine interfaces used for navigation. Our findings also provide a starting point for the design of tailored navigation assistance systems that respond to users’ cognitive load to optimize spatial learning while still maintaining navigation efficiency.
The raw data supporting the conclusions of this article will be made available by the authors, without undue reservation.
The studies involving human participants were reviewed and approved by the Ethics Board at the University of Zurich, Switzerland. The patients/participants provided their written informed consent to participate in this study.
BC, KG, and SF designed the study. BC performed data collection. BC performed data analysis. AW, KG, and EL assisted with data analysis. BC drafted the manuscript and all authors were involved in revising the manuscript. The authors read and approved the final manuscript.
This work was supported by the H2020 European Research Council (ERC) Advanced Grant GeoViSense (740426). https://cordis.europa.eu/project/id/740426. The funder had no role in the study design, data collection and analysis, decision to publish, or preparation of the manuscript.
We thank Armand Kapaj for his assistance in the data collection and Ian Ruginski for his advice on the experimental design and assistance with multilevel modeling.
The authors declare that the research was conducted in the absence of any commercial or financial relationships that could be construed as a potential conflict of interest.
All claims expressed in this article are solely those of the authors and do not necessarily represent those of their affiliated organizations, or those of the publisher, the editors and the reviewers. Any product that may be evaluated in this article, or claim that may be made by its manufacturer, is not guaranteed or endorsed by the publisher.
The Supplementary Material for this article can be found online at: https://www.frontiersin.org/articles/10.3389/frvir.2022.981625/full#supplementary-material
Allen, G. L. (Editor) (2003). Human Spatial Memory: Remembering Where (1st ed.). New York, NY: Psychology Press. doi:10.4324/9781410609984
Alvarez, G. A., and Cavanagh, P. (2004). The capacity of visual short-term memory is set both by visual information load and by number of objects. Psychol. Sci. 15 (2), 106–111. doi:10.1111/j.0963-7214.2004.01502006.x
Anacta, V. J. A., Schwering, A., Li, R., and Muenzer, S. (2017). Orientation information in wayfinding instructions: Evidences from human verbal and visual instructions. GeoJournal 82 (3), 567–583. doi:10.1007/s10708-016-9703-5
Armougum, A., Orriols, E., Gaston-Bellegarde, A., Marle, C. J.-L., and Piolino, P. (2019). Virtual reality: A new method to investigate cognitive load during navigation. J. Environ. Psychol. 65, 101338. doi:10.1016/j.jenvp.2019.101338
Awh, E., Anllo-Vento, L., and Hillyard, S. A. (2000). The role of spatial selective attention in working memory for locations: Evidence from event-related potentials. J. Cogn. Neurosci. 12 (5), 840–847. doi:10.1162/089892900562444
Baddeley, A. (2003). Working memory: Looking back and looking forward. Nat. Rev. Neurosci. 4 (10), 829–839. doi:10.1038/nrn1201
Bates, D., Maechler, M., Bolker, B., Walker, S., Christensen, R. H. B., Singmann, H., et al. (2011). Data From: Package ‘lme4.’ linear mixed-effects models using S4 classes, R Package Version, http://dk.archive.ubuntu.com/pub/pub/cran/web/packages/lme4/lme4.pdf.
Bohbot, V. D., Copara, M. S., Gotman, J., and Ekstrom, A. D. (2017). Low-frequency theta oscillations in the human hippocampus during real-world and virtual navigation. Nat. Commun. 8, 14415–14417. doi:10.1038/ncomms14415
Brügger, A., Richter, K.-F., and Fabrikant, S. I. (2019). How does navigation system behavior influence human behavior? Cogn. Res. 4 (1), 5. doi:10.1186/s41235-019-0156-5
Bunch, R., and Lloyd, R. (2006). The cognitive load of geographic information. Prof. Geogr. 58, 209–220. doi:10.1111/j.1467-9272.2006.00527.x
Capilla, A., Schoffelen, J. M., Paterson, G., Thut, G., and Gross, J. (2014). Dissociated α-band modulations in the dorsal and ventral visual pathways in visuospatial attention and perception. Cereb. Cortex 24 (2), 550–561. doi:10.1093/CERCOR/BHS343
Carp, J., and Compton, R. J. (2009). Alpha power is influenced by performance errors. Psychophysiology 46 (2), 336–343. doi:10.1111/j.1469-8986.2008.00773.x
Chrastil, E. R., and Warren, W. H. (2013). Active and passive spatial learning in human navigation: Acquisition of survey knowledge. J. Exp. Psychol. Learn. Mem. Cognition 39 (5), 1520–1537. doi:10.1037/a0032382
Clemenson, G. D., Maselli, A., Fiannaca, A. J., Miller, A., and Gonzalez-Franco, M. (2021). Rethinking GPS navigation: Creating cognitive maps through auditory clues. Sci. Rep. 11 (1), 1–10. doi:10.1038/s41598-021-87148-4
Clemenson, G. D., Wang, L., Mao, Z., Stark, S. M., and Stark, C. E. L. (2020). Exploring the spatial relationships between real and virtual experiences: What transfers and what doesn’t. Front. Virtual Real. 1. doi:10.3389/frvir.2020.572122
Cohen, M. X. (2014). Analyzing neural time series data: Theory and practice. Cambridge, MA: MIT Press.
Cowan, N. (2001). The magical number 4 in short-term memory: A reconsideration of mental storage capacity. Behav. Brain Sci. 24 (1), 87–114. doi:10.1017/S0140525X01003922
Credé, S., Thrash, T., Hölscher, C., and Fabrikant, S. I. (2019). The acquisition of survey knowledge for local and global landmark configurations under time pressure. Spatial Cognition Comput. 19, 190–219. doi:10.1080/13875868.2019.1569016
Credé, S., Thrash, T., Hölscher, C., and Fabrikant, S. I. (2020). The advantage of globally visible landmarks for spatial learning. J. Environ. Psychol. 67, 101369. doi:10.1016/j.jenvp.2019.101369
Dahmani, L., and Bohbot, V. D. (2020). Habitual use of GPS negatively impacts spatial memory during self-guided navigation. Sci. Rep. 10 (1), 63101–63114. doi:10.1038/s41598-020-62877-0
Darken, R. P., and Peterson, B. (2002). “Spatial orientation, wayfinding, and representation,” in Handbook of virtual environments (Boca Raton: CRC Press). doi:10.1201/9780585399102
Delaux, A., de Saint Aubert, J. B., Ramanoël, S., Bécu, M., Gehrke, L., Klug, M., et al. (2021). Mobile brain/body imaging of landmark-based navigation with high-density EEG. Eur. J. Neurosci. 54, 8256–8282. doi:10.1111/ejn.15190
Delorme, A., and Makeig, S. (2004). Eeglab: An open source toolbox for analysis of single-trial EEG dynamics including independent component analysis. J. Neurosci. Methods 134 (1), 9–21. doi:10.1016/j.jneumeth.2003.10.009
Do, T. T. N., Lin, C. T., and Gramann, K. (2021). Human brain dynamics in active spatial navigation. Sci. Rep. 11 (1), 1–12. doi:10.1038/s41598-021-92246-4
Doesburg, S. M., Green, J. J., McDonald, J. J., and Ward, L. M. (2009). From local inhibition to long-range integration: A functional dissociation of alpha-band synchronization across cortical scales in visuospatial attention. Brain Res. 1303, 97–110. doi:10.1016/j.brainres.2009.09.069
Dong, S., Reder, L. M., Yao, Y., Liu, Y., and Chen, F. (2015). Individual differences in working memory capacity are reflected in different ERP and EEG patterns to task difficulty. Brain Res. 1616, 146–156. doi:10.1016/J.BRAINRES.2015.05.003
Duckham, M., Winter, S., and Robinson, M. (2010). Including landmarks in routing instructions. J. Locat. Based Serv. 4 (1), 28–52. doi:10.1080/1748972100378560210.1080/17489721003785602
Duncan, C. C., Barry, R. J., Connolly, J. F., Fischer, C., Michie, P. T., Näätänen, R., et al. (2009). Event-related potentials in clinical research: Guidelines for eliciting, recording, and quantifying mismatch negativity, P300, and N400. Clin. Neurophysiol. 120 (11), 1883–1908. doi:10.1016/J.CLINPH.2009.07.045
Farr, A. C., Kleinschmidt, T., Yarlagadda, P., and Mengersen, K. (2012). Wayfinding: A simple concept, a complex process. Transp. Rev. 32 (6), 715–743. doi:10.1080/01441647.2012.712555
Fenech, E. P., Drews, F. A., and Bakdash, J. Z. (2010). The effects of acoustic turn-by-turn navigation on wayfinding. Proc. Hum. Factors Ergonomics Soc. Annu. Meet. 54 (23), 1926–1930. doi:10.1177/154193121005402305
Fischer, L. F., Mojica Soto-Albors, R., Buck, F., and Harnett, M. T. (2020). Representation of visual landmarks in retrosplenial cortex. ELife 9, e51458. doi:10.7554/eLife.51458
Fitzmaurice, G., Davidian, M., Verbeke, G., and Molenberghs, G. (2008). “Advances in longitudinal data analysis: An historical perspective,” in Longitudinal data analysis. London, United Kingdom: Chapman and Hall/CRC, 17–42. doi:10.1201/9781420011579-9
Frankenstein, J., Mohler, B. J., Bülthoff, H. H., and Meilinger, T. (2012). Is the map in our head oriented North?. Psychol. Sci. 23 (2), 120–125. doi:10.1177/0956797611429467
Fu, S., and Parasuraman, R. (2006). “Event-related potentials (ERPs) in neuroergonomics,” in Neuroergonomics: The brain at work, Human Technology Interaction Series. Editors R. Parasuraman, and M. Rizzo (New York, NY: Oxford University Press), 32–50. doi:10.1093/acprof:oso/9780195177619.003.0003
Fukuda, K., Mance, I., and Vogel, E. K. (2015). α power modulation and event-related slow wave provide dissociable correlates of visual working memory. J. Neurosci. 35 (41), 14009–14016. doi:10.1523/JNEUROSCI.5003-14.2015
Gardony, A. L., Brunyé, T. T., Mahoney, C. R., and Taylor, H. A. (2013). How navigational aids impair spatial memory: Evidence for divided attention. Spat. Cogn. Comput. 13 (4), 319–350. doi:10.1080/13875868.2013.792821
Gardony, A. L., Brunyé, T. T., and Taylor, H. A. (2015). Navigational aids and spatial memory impairment: The role of divided attention. Spat. Cogn. Comput. 15 (4), 246–284. doi:10.1080/13875868.2015.105943210.1080/13875868.2015.1059432
Garlandini, S., and Fabrikant, S. I. (2009). “Evaluating the effectiveness and efficiency of visual variables for geographic information visualization,” in International Conference on Spatial Information Theory. Berlin, Heidelberg: Springer, 195–211. doi:10.1007/978-3-642-03832-7_12
Gehrke, L., Iversen, J. R., Makeig, S., and Gramann, K. (2018). The invisible maze task (IMT): Interactive exploration of sparse virtual environments to investigate action-driven formation of spatial representations. Lect. Notes Comput. Sci. Incl. Subser. Lect. Notes Artif. Intell. Lect. Notes Bioinformatics)LNAI 11034, 293–310. doi:10.1007/978-3-319-96385-3_20
Gelman, A. (2006). Multilevel (hierarchical) modeling: What it can and cannot do. Technometrics 48 (3), 432–435. doi:10.1198/004017005000000661
Gevins, A., and Smith, M. E. (2003). Neurophysiological measures of cognitive workload during human–computer interaction. Theor. Issues Ergonomics Sci. 4 (1–2), 113–131. doi:10.1080/14639220210159717
Ghani, U., Signal, N., Niazi, I. K., and Taylor, D. (2020). ERP based measures of cognitive workload: A review. Neurosci. Biobehav. Rev. 118, 18–26. doi:10.1016/j.neubiorev.2020.07.020
Gladwin, T. E., and De Jong, R. (2005). Bursts of occipital theta and alpha amplitude preceding alternation and repetition trials in a task-switching experiment. Biol. Psychol. 68 (3), 309–329. doi:10.1016/J.BIOPSYCHO.2004.06.004
Gramann, K. (2013). Embodiment of spatial reference frames and individual differences in reference frame proclivity. Spat. Cogn. Comput. 13, 1–25. doi:10.1080/13875868.2011.589038
Gramann, K., Hohlefeld, F. U., Gehrke, L., and Klug, M. (2021). Human cortical dynamics during full-body heading changes. Sci. Rep. 11 (1), 1–12. doi:10.1038/s41598-021-97749-8
Gramann, K., Onton, J., Riccobon, D., Mueller, H. J., Bardins, S., and Makeig, S. (2010). Human brain dynamics accompanying use of egocentric and allocentric reference frames during navigation. J. Cogn. Neurosci. 22 (12), 2836–2849. doi:10.1162/jocn.2009.21369
Handy, T. C., Soltani, M., and Mangun, G. R. (2001). Perceptual load and visuocortical processing: Event-related potentials reveal sensory-level selection. Psychol. Sci. 12 (3), 213–218. doi:10.1111/1467-9280.00338
Hart, S. G., and Staveland, L. E. (1988). “Development of NASA-TLX (task load index): Results of empirical and theoretical research”. In Adv. Psychol. Editors P. A. Hancock, and N. Meshkati (Amsterdam: North-Holland), 52, 139–183. doi:10.1016/S0166-4115(08)62386-9
Hegarty, M., Richardson, A. E., Montello, D. R., Lovelace, K., and Subbiah, I. (2002). Development of a self-report measure of environmental spatial ability. Intelligence 30 (5), 425–447. doi:10.1016/S0160-2896(02)00116-2
Hegarty, M., and Waller, D. (2004). A dissociation between mental rotation and perspective-taking spatial abilities. Intelligence 32 (2), 175–191. doi:10.1016/j.intell.2003.12.001
Heinze, H. J., Luck, S. J., Mangun, G. R., and Hillyard, S. A. (1990). Visual event-related potentials index focused attention within bilateral stimulus arrays. I. Evidence for early selection. Electroencephalogr. Clin. Neurophysiology 75 (6), 511–527. doi:10.1016/0013-4694(90)90138-a
Hillyard, S. A., and Anllo-Vento, L. (1998). Event-related brain potentials in the study of visual selective attention. Proc. Natl. Acad. Sci. U. S. A. 95 (3), 781–787. doi:10.1073/pnas.95.3.781
Huang, H., Schmidt, M., and Gartner, G. (2012). Spatial knowledge acquisition with mobile maps, augmented reality and voice in the context of GPS-based pedestrian navigation: Results from a field test. Cartogr. Geogr. Inf. Sci. 39 (2), 107–116. doi:10.1559/15230406392107
Huffman, D. J., and Ekstrom, A. D. (2018). Which way is the bookstore? A closer look at the judgments of relative directions task. Spat. Cogn. Comput. 19, 93–129. doi:10.1080/13875868.2018.1531869
Ishikawa, T. (2019). Satellite navigation and geospatial awareness: Long-term effects of using navigation tools on wayfinding and spatial orientation. Prof. Geogr. 71 (2), 197–209. doi:10.1080/00330124.2018.1479970
Itti, L., and Koch, C. (2001). Computational modelling of visual attention. Nat. Rev. Neurosci. 2 (3), 194–203. doi:10.1038/35058500
Jensen, O., and Mazaheri, A. (2010). Shaping functional architecture by oscillatory alpha activity: Gating by inhibition. Front. Hum. Neurosci. 4, 186. doi:10.3389/fnhum.2010.00186
Jensen, O., and Tesche, C. D. (2002). Frontal theta activity in humans increases with memory load in a working memory task. Eur. J. Neurosci. 15 (8), 1395–1399. doi:10.1046/j.1460-9568.2002.01975.x
Kahana, M. J., Sekuler, R., Caplan, J. B., Kirschen, M., and Madsen, J. R. (1999). Human theta oscillations exhibit task dependence during virtual maze navigation. Nature 399 (6738), 781–784. doi:10.1038/21645
Kapaj, A., Lanini-Maggi, S., and Fabrikant, S. I. (2021). “The influence of landmark visualization style on expert wayfinders’ visual attention during a real-world navigation task,” in Proceeding of the GIScience 2021 Short Paper Proceedings, Poznań, Poland, September 27–September 30, 2021.
Klimesch, W., Doppelmayr, M., Russegger, H., Pachinger, T., and Schwaiger, J. (1998). Induced alpha band power changes in the human EEG and attention. Neurosci. Lett. 244 (2), 73–76. doi:10.1016/S0304-3940(98)00122-0
Klimesch, W. (1999). EEG alpha and theta oscillations reflect cognitive and memory performance: A review and analysis. Brain Res. Rev. 29 (2–3), 169–195. doi:10.1016/S0165-0173(98)00056-3
Klimesch, W., Freunberger, R., Sauseng, P., and Gruber, W. (2008). A short review of slow phase synchronization and memory: Evidence for control processes in different memory systems? Brain Res. 1235, 31–44. doi:10.1016/J.BRAINRES.2008.06.049
Klug, M., Jeung, S., Wunderlich, A., Gehrke, L., Protzak, J., Djebbara, Z., et al. (2022). The BeMoBIL Pipeline for automated analyses of multimodal mobile brain and body imaging data, 2022. bioRxiv. doi:10.1101/2022.09.29.510051
Klug, M., and Kloosterman, N. A. (2022). Zapline-plus: A zapline extension for automatic and adaptive removal of frequency-specific noise artifacts in M/EEG. Hum. Brain Mapp. 43, 2743–2758. doi:10.1002/HBM.25832
Kok, A. (2001). On the utility of P3 amplitude as a measure of processing capacity. Psychophysiology 38 (3), S0048577201990559–S0048577201990577. doi:10.1017/S0048577201990559
Korporaal, M., Ruginski, I. T., and Fabrikant, S. I. (2020). Effects of uncertainty visualization on map-based decision making under time pressure. Front. Comput. Sci. 2. doi:10.3389/fcomp.2020.00032
Kramer, A. F., Strayer, D. L., and Buckley, J. (1991). Task versus component consistency in the development of automatic processing: A psychophysiological assessment. Psychophysiology 28 (4), 425–437. doi:10.1111/j.1469-8986.1991.tb00726.x
Krause, C. M., Sillanmäki, L., Koivisto, M., Saarela, C., Häggqvist, A., Laine, M., et al. (2000). The effects of memory load on event-related EEG desynchronization and synchronization. Clin. Neurophysiol. 111 (11), 2071–2078. doi:10.1016/S1388-2457(00)00429-6
Krejtz, K., Duchowski, A. T., Niedzielska, A., Biele, C., and Krejtz, I. (2018). Eye tracking cognitive load using pupil diameter and microsaccades with fixed gaze. PLOS ONE 13 (9), e0203629. doi:10.1371/journal.pone.0203629
Li, R. (2020). Spatial learning in smart applications: Enhancing spatial awareness through visualized off-screen landmarks on mobile devices. Ann. Am. Assoc. Geogr. 110 (2), 421–433. doi:10.1080/24694452.2019.1670611
Liao, H., Dong, W., Peng, C., and Liu, H. (2017). Exploring differences of visual attention in pedestrian navigation when using 2D maps and 3D geo-browsers. Cartogr. Geogr. Inf. Sci. 44 (6), 474–490. doi:10.1080/15230406.2016.1174886
Ligonnière, V., Gyselinck, V., Lhuillier, S., Mostafavi, M. A., and Dommes, A. (2021). How does the visual and cognitive saliency of landmarks improve construction of spatial representations in younger and older adults? Spat. Cogn. Comput. 21 (4), 320–345. doi:10.1080/13875868.2021.1992410
Liu, J., Singh, A. K., and Lin, C.-T. (2022). Using virtual global landmark to improve incidental spatial learning. Sci. Rep. 12 (11), 1–14. doi:10.1038/s41598-022-10855-z
Lobben, A. K. (2004). Tasks, strategies, and cognitive processes associated with navigational map reading: A review perspective. Prof. Geogr. 56 (2), 270–281. doi:10.1111/j.0033-0124.2004.05602010.x
Luck, S. J., Heinze, H. J., Mangun, G. R., and Hillyard, S. A. (1990). Visual event-related potentials index focused attention within bilateral stimulus arrays. II. Functional dissociation of P1 and N1 components. Electroencephalogr. Clin. Neurophysiology 75 (6), 528–542. doi:10.1016/0013-4694(90)90139-b
Luck, S. J., and Vogel, E. K. (1997). The capacity of visual working memory for features and conjunctions. Nature 390 (6657), 279–281. doi:10.1038/36846
Maurer, U., Brem, S., Liechti, M., Maurizio, S., Michels, L., and Brandeis, D. (2015). Frontal midline theta reflects individual task performance in a working memory task. Brain Topogr. 28 (1), 127–134. doi:10.1007/s10548-014-0361-y
McDermott, T. J., Wiesman, A. I., Proskovec, A. L., Heinrichs-Graham, E., and Wilson, T. W. (2017). Spatiotemporal oscillatory dynamics of visual selective attention during a flanker task. NeuroImage 156, 277–285. doi:10.1016/j.neuroimage.2017.05.014
McKinlay, R. (2016). Technology: Use or lose our navigation skills. Nature 531 (7596), 573–575. doi:10.1038/531573a
Meneghetti, C., Labate, E., Toffalini, E., and Pazzaglia, F. (2021). Successful navigation: The influence of task goals and working memory. Psychol. Res. 85 (2), 634–648. doi:10.1007/s00426-019-01270-7
Merhav, M., and Wolbers, T. (2019). Aging and spatial cues influence the updating of navigational memories. Sci. Rep. 9 (1), 11469. doi:10.1038/s41598-019-47971-2
Montello, Daniel. R. (2005). “Navigation,” in The cambridge handbook of visuospatial thinking (Cambridge University Press), 257–294. doi:10.1017/cbo9780511610448.008
Montello, D. R. (2002). Cognitive map-design research in the twentieth century: Theoretical and empirical approaches. Cartogr. Geogr. Inf. Sci. 29 (3), 283–304. doi:10.1559/152304002782008503
Montello, D. R. (2017). Landmarks are exaggerated. Kunstl. Intell. 31 (2), 193–197. doi:10.1007/s13218-016-0473-5
Münzer, S., Lörch, L., and Frankenstein, J. (2020). Wayfinding and acquisition of spatial knowledge with navigation assistance. J. Exp. Psychol. Appl. 26 (1), 73–88. doi:10.1037/xap0000237
Münzer, S., Zimmer, H. D., and Baus, J. (2012). Navigation assistance: A trade-off between wayfinding support and configural learning support. J. Exp. Psychol. Appl. 18 (1), 18–37. doi:10.1037/a0026553
Näätänen, R. (1992). Attention and brain function. Hillsdale, NJ: Lawrence Erlbaum Associates. Available at: https://psycnet.apa.org/record/1992-98140-000.
Nelli, S., Itthipuripat, S., Srinivasan, R., and Serences, J. T. (2017). Fluctuations in instantaneous frequency predict alpha amplitude during visual perception. Nat. Commun. 8 (11), 1–12. doi:10.1038/s41467-017-02176-x
Nishiyori, R., Xiao, R., Vanderbilt, D., and Smith, B. A. (2021). Electroencephalography measures of relative power and coherence as reaching skill emerges in infants born preterm. Sci. Rep. 11 (11), 3609–3614. doi:10.1038/s41598-021-82329-7
Onton, J., Delorme, A., and Makeig, S. (2005). Frontal midline EEG dynamics during working memory. NeuroImage 27 (2), 341–356. doi:10.1016/j.neuroimage.2005.04.014
Oostenveld, R., and Oostendorp, T. F. (2002). Validating the boundary element method for forward and inverse EEG computations in the presence of a hole in the skull. Hum. Brain Mapp. 17 (3), 179–192. doi:10.1002/HBM.10061
Palmer, J., Kreutz-Delgado, K., and Makeig, S. (2011). Amica: An adaptive mixture of independent component analyzers with shared components. Techical Report. Swartz Center for Computatonal Neursoscience, University of California San Diego. Available at: http://sccn.ucsd.edu/∼jason/amica_a.pdf.
Palva, S., and Palva, J. M. (2007). New vistas for alpha-frequency band oscillations. Trends Neurosci. 30 (4), 150–158. doi:10.1016/j.tins.2007.02.001
Parks, T. E. (1966). Signal-detectability theory of recognition-memory performance. Psychol. Rev. 73 (1), 44–58. doi:10.1037/h0022662
Parush, A., Ahuvia, S., and Erev, I. (2007). Degradation in spatial knowledge acquisition when using automatic navigation systems. In Proceeding of the International Conference on Spatial Information Theory, Melbourne, VIC, September 19–September 23, 2007 (Berlin, Heidelberg: Springer), 238–254. doi:10.1007/978-3-540-74788-8_15
Pastel, S., Bürger, D., Chen, C. H., Petri, K., and Witte, K. (2022). Comparison of spatial orientation skill between real and virtual environment. Virtual Real. 26 (1), 91–104. doi:10.1007/s10055-021-00539-w
Pennekamp, P., Bösel, R., Mecklinger, A., and Ott, H. (1994). Differences in EEG-theta for responded and omitted targets in a sustained attention task. J. Psychophysiol. 8 (2), 131–141.
Pfurtscheller, G., and Lopes da Silva, F. H. (1999). Event-related EEG/MEG synchronization and desynchronization: Basic principles. Clin. Neurophysiol. 110 (11), 1842–1857. doi:10.1016/s1388-2457(99)00141-8
Pfurtscheller, G., Stancák, A., and Neuper, Ch. (1996). Event-related synchronization (ERS) in the alpha band — An electrophysiological correlate of cortical idling: A review. Int. J. Psychophysiol. 24 (1), 39–46. doi:10.1016/S0167-8760(96)00066-9
Pion-Tonachini, L., Kreutz-Delgado, K., and Makeig, S. (2019). ICLabel: An automated electroencephalographic independent component classifier, dataset, and website. Neuroimage 198, 181–197. doi:10.1016/j.neuroimage.2019.05.026
Plank, M., Müller, H. J., Onton, J., Makeig, S., and Gramann, K. (2010). Spatial cognition. Encycl. Neurosci. August, 165–171. doi:10.1016/B978-008045046-9.01120-7
Polich, J. (2007). Updating P300: An integrative theory of P3a and P3b. Clin. Neurophysiol. 118 (10), 2128–2148. doi:10.1016/J.CLINPH.2007.04.019
Presson, C. C., and Montello, D. R. (1988). Points of reference in spatial cognition: Stalking the elusive landmark. Br. J. Dev. Psychol. 6 (4), 378–381. doi:10.1111/j.2044-835x.1988.tb01113.x
Puma, S., Matton, N., Paubel, P. V., Raufaste, É., and El-Yagoubi, R. (2018). Using theta and alpha band power to assess cognitive workload in multitasking environments. Int. J. Psychophysiol. 123, 111–120. doi:10.1016/J.IJPSYCHO.2017.10.004
Ramanoël, S., Durteste, M., Perot, V., Habas, C., and Arleo, A. (2022). An appraisal of the role of the neocerebellum for spatial navigation in healthy aging. Cerebellum. doi:10.1007/s12311-022-01389-1
Ratcliffe, O., Shapiro, K., and Staresina, B. P. (2022). Fronto-medial theta coordinates posterior maintenance of working memory content. Curr. Biol. 32, 2121–2129.e3. doi:10.1016/j.cub.2022.03.045
Raubal, M., and Winter, S. (2002). Enriching wayfinding instructions with local landmarks. Lect. Notes Comput. Sci. Incl. Subser. Lect. Notes Artif. Intell. Lect. Notes Bioinforma. 2478, 243–259. doi:10.1007/3-540-45799-2_17
Richter, K. F., and Winter, S. (2014). “Landmarks: Giscience for intelligent services,” in Landmarks: Giscience for intelligent services (Springer International Publishing). doi:10.1007/978-3-319-05732-3
Ruginski, I. T., Creem-Regehr, S. H., Stefanucci, J. K., and Cashdan, E. (2019). GPS-use negatively affects environmental learning through spatial transformation abilities. J. Environ. Psychol. 64, 12–20. doi:10.1016/j.jenvp.2019.05.001
Sanchez-Vives, M. V., and Slater, M. (2005). From presence to consciousness through virtual reality. Nat. Rev. Neurosci. 6 (4), 332–339. doi:10.1038/nrn1651
Sauseng, P., Griesmayr, B., Freunberger, R., and Klimesch, W. (2010). Control mechanisms in working memory: A possible function of EEG theta oscillations. Neurosci. Biobehav. Rev. 34 (7), 1015–1022. doi:10.1016/J.NEUBIOREV.2009.12.006
Sauseng, P., Klimesch, W., Stadler, W., Schabus, M., Doppelmayr, M., Hanslmayr, S., et al. (2005). A shift of visual spatial attention is selectively associated with human EEG alpha activity. Eur. J. Neurosci. 22 (11), 2917–2926. doi:10.1111/J.1460-9568.2005.04482.X
Scharinger, C., Soutschek, A., Schubert, T., and Gerjets, P. (2017). Comparison of the working memory load in N-back and working memory span tasks by means of EEG frequency band power and P300 amplitude. Front. Hum. Neurosci. 11, 6. doi:10.3389/fnhum.2017.00006
Siegel, A. W., and White, S. H. (1975). “The development of spatial representations of large-scale environments,”. In Adv. Child Dev. Behav.Editor H. W. Reese (JAI), 10, 9–55. doi:10.1016/S0065-2407(08)60007-5
Smith, M. E., Gevins, A., Brown, H., Karnik, A., and Du, R. (2001). Monitoring task loading with multivariate EEG measures during complex forms of human-computer interaction. Hum. Factors 43 (3), 366–380. doi:10.1518/001872001775898287
Spencer, K. M., and Polich, J. (1999). Poststimulus EEG spectral analysis and P300: Attention, task, and probability. Psychophysiology 36 (2), 220–232. doi:10.1111/1469-8986.3620220
Stipacek, A., Grabner, R. H., Neuper, C., Fink, A., and Neubauer, A. C. (2003). Sensitivity of human EEG alpha band desynchronization to different working memory components and increasing levels of memory load. Neurosci. Lett. 353 (3), 193–196. doi:10.1016/J.NEULET.2003.09.044
Sugimoto, M., Kusumi, T., Nagata, N., and Ishikawa, T. (2021). Online mobile map effect: How smartphone map use impairs spatial memory. Spat. Cogn. Comput. 22, 161–183. doi:10.1080/13875868.2021.1969401
Sweller, J. (1988). Cognitive load during problem solving: Effects on learning. Cogn. Sci. 12 (2), 257–285. doi:10.1207/s15516709cog1202_4
Sweller, J., van Merrienboer, J. J. G., and Paas, F. G. W. C. (1998). Cognitive architecture and instructional design. Educ. Psychol. Rev. 10 (3), 251–296. doi:10.1023/A:1022193728205
Tenbrink, T., Taylor, H. A., Brunyé, T. T., Gagnon, S. A., and Gardony, A. L. (2020). Cognitive focus affects spatial decisions under conditions of uncertainty. Cogn. Process. 21 (2), 287–302. doi:10.1007/s10339-020-00952-0
Uttal, D. H., and Cohen, C. A. (2012). Spatial thinking and STEM education. Psychol. Learn. Motiv. 57, 147–181. doi:10.1016/B978-0-12-394293-7.00004-2
Van der Stelt, O., Geesken, R., Gunning, W. B., Snel, J., and Kok, A. (1998). P3 scalp topography to target and novel visual stimuli in children of alcoholics. AlcoholFayettev. N.Y.) 15 (2), 119–136. doi:10.1016/s0741-8329(97)00106-7
Wang, Y. K., Jung, T. P., and Lin, C. T. (2018). Theta and alpha oscillations in attentional interaction during distracted driving. Front. Behav. Neurosci. 12, 3. doi:10.3389/fnbeh.2018.00003
Wang, Y., Sokhadze, E. M., El-Baz, A. S., Li, X., Sears, L., Casanova, M. F., et al. (2015). Relative power of specific EEG bands and their ratios during neurofeedback training in children with autism spectrum disorder. Front. Hum. Neurosci. 9 (2016), 723. doi:10.3389/FNHUM.2015.00723
Wascher, E., Heppner, H., and Hoffmann, S. (2014). Towards the measurement of event-related EEG activity in real-life working environments. Int. J. Psychophysiol. 91 (1), 3–9. doi:10.1016/j.ijpsycho.2013.10.006
Watter, S., Geffen, G. M., and Geffen, L. B. (2001). The n-back as a dual-task: P300 morphology under divided attention. Psychophysiology 38 (6), 998–1003. doi:10.1111/1469-8986.3860998
Wei, H., and Zhou, R. (2020). High working memory load impairs selective attention: EEG signatures. Psychophysiology 57 (11), e13643. doi:10.1111/psyp.13643
Wen, W., Ishikawa, T., and Sato, T. (2013). Individual differences in the encoding processes of egocentric and allocentric survey knowledge. Cogn. Sci. 37 (1), 176–192. doi:10.1111/cogs.12005
Wenig, N., Wenig, D., Ernst, S., Malaka, R., Hecht, B., and Schöning, J. (2017). “Pharos: Improving navigation instructions on smartwatches by including global landmarks,” in Proceedings of the 19th international conference on human-computer interaction with mobile devices and services, Vienna, Austria, September 4–September 7, 2017 (MobileHCI), 1–13. doi:10.1145/3098279.3098529
Wunderlich, A., and Gramann, K. (2020). Eye movement-related brain potentials during assisted navigation in real-world environments. Eur. J. Neurosci. 54, 8336–8354. doi:10.1111/ejn.15095
Wunderlich, A., and Gramann, K. (2021). Landmark-based navigation instructions improve incidental spatial knowledge acquisition in real-world environments. J. Environ. Psychol. 77, 101677. doi:10.1016/j.jenvp.2021.101677
Yesiltepe, D., Conroy Dalton, R., and Ozbil Torun, A. (2021). Landmarks in wayfinding: A review of the existing literature. Cogn. Process. 1, 369–410. doi:10.1007/s10339-021-01012-x
Zenrin (2017). Survey on the use of maps 2017. Tokyo: Zenrin Co., Ltd. Available at: https://www.zenrin.co.jp/product/article/map-17/index.html (Accessed April 19, 2018).
Keywords: cognitive load, assisted navigation, spatial learning, mobile maps, landmark visualization, virtual reality, EEG, visuospatial encoding
Citation: Cheng B, Wunderlich A, Gramann K, Lin E and Fabrikant SI (2022) The effect of landmark visualization in mobile maps on brain activity during navigation: A virtual reality study. Front. Virtual Real. 3:981625. doi: 10.3389/frvir.2022.981625
Received: 29 June 2022; Accepted: 01 November 2022;
Published: 15 November 2022.
Edited by:
Jonathan W. Kelly, Iowa State University, United StatesReviewed by:
Tad Brunye,Tufts University, United StatesCopyright © 2022 Cheng, Wunderlich, Gramann, Lin and Fabrikant. This is an open-access article distributed under the terms of the Creative Commons Attribution License (CC BY). The use, distribution or reproduction in other forums is permitted, provided the original author(s) and the copyright owner(s) are credited and that the original publication in this journal is cited, in accordance with accepted academic practice. No use, distribution or reproduction is permitted which does not comply with these terms.
*Correspondence: Bingjie Cheng, YmluZ2ppZS5jaGVuZ0BnZW8udXpoLmNo
Disclaimer: All claims expressed in this article are solely those of the authors and do not necessarily represent those of their affiliated organizations, or those of the publisher, the editors and the reviewers. Any product that may be evaluated in this article or claim that may be made by its manufacturer is not guaranteed or endorsed by the publisher.
Research integrity at Frontiers
Learn more about the work of our research integrity team to safeguard the quality of each article we publish.