- Experimental Psychology Unit, Edith Cowan University, Perth, WA, Australia
Using motion capture to enhance the realism of social interaction in virtual reality (VR) is growing in popularity. However, the impact of different levels of avatar expressiveness on the user experience is not well understood. In the present study we manipulated levels of face and body expressiveness of avatars while investigating participant perceptions of animation realism and interaction quality when disclosing positive and negative experiences in VR. Moderate positive associations were observed between perceptions of animation realism and interaction quality. Post-experiment questions revealed that many of our participants (approximately 40%) indicated the avatar with the highest face and body expressiveness as having the most realistic face and body expressions. The same proportion also indicated the avatar with the highest face and body expressiveness as being the most comforting and enjoyable avatar to interact with. Our results suggest that higher levels of face and body expressiveness are important for enhancing perceptions of realism and interaction quality within a social interaction in VR using motion capture.
Introduction
Despite real-time social interaction in Virtual Reality (VR) via head-mounted display (HMD) being widely available since around 2016 (e.g., Rec Room, VR chat, and more recently Horizon Worlds), VR social interaction is yet to become a mainstream activity. One historical limitation of VR social interaction applications has been the restricted facial and body expressiveness for player avatars. However, motion capture is becoming more accessible and can enhance perceptions of animation realism (Fribourg et al., 2020; Rogers et al., 2022). Avatar expressiveness in VR is important for conveying real life social cues such as eye gaze and gesturing (Aburumman et al., 2022; Cao et al., 2020; Ferstl and McDonnell, 2018; Herrera et al., 2020; Kokkinara and McDonnell, 2015; Kyrlitsias and Michael-Grigoriou, 2022; Rogers et al., 2022; Roth et al., 2016; Seymour et al., 2021; Thomas et al., 2022; Wu et al., 2021).
There is currently a lack of research investigating how different levels of avatar expressiveness might affect the experience of VR social interactions. Given that companies such as Microsoft and Meta are now developing their own VR social applications, it is important to clarify how avatar expressiveness influences perceived realism and interaction. This will help to broaden understanding of how VR avatars are experienced during VR social interactions, and to better design these avatars for specific purposes, such as VR based training or therapy (Owens and Beidel, 2015; Baccon et al., 2019; Arias et al., 2021). Communication with real time motion captured avatars has been of long interest (Capin et al., 1997; Guye-Vuillème et al., 1999; Kalra et al., 1998; Maurel et al., 1998), however it is only in more recent years that technology has become more accessible and further developed to provide what might be considered a ‘good’ user experience (Seymour et al., 2021, 2018; Rogers et al., 2022). In our study we investigated how participants experience social interactions in VR when their partner’s avatar is controlled with motion captured facial and body movements in real time. We examined participant ratings of animation realism and interaction quality across different avatars with different levels of face and body expressiveness.
Behavioural Realism in VR
Behavioural realism is a broad term used to describe the extent to which features found within a virtual environment mimic a real-world equivalent. Within the context of VR, these features include environments, events, items, or characters (Oh et al., 2018; Wiley et al., 2018; Herrera et al., 2020; Arias et al., 2021). Perceptions of behavioural realism are based on assumptions related to our real-world experience. For example, a virtual ball that quickly falls to the earth after being thrown may be considered as having a high level of behavioural realism if it prompts the user to react as if it were real.
Avatars are the visual representation of social actors within the virtual environment (Kyrlitsias and Michael-GrigoriouLugrin et al., 2015; Seymour et al., 2018;, 2022). Two key qualities of avatars include their visual depiction and their movement capabilities (Grewe et al., 2021; Higgins et al., 2022; Latoschik et al., 2017; Stuart et al., 2022; Wu et al., 2021). While we recognize the importance of both these qualities, in our research we are focused on the visual expressiveness of the avatar. Motion capture technologies can affect the experience of social VR because motion captured avatars are able to convey a wider range of social cues during an interaction (Kokkinara and McDonnell, 2015; Lugrin et al., 2015; Latoschik et al., 2017; Herrera et al., 2020; Kyrlitsias and Michael-Grigoriou, 2022; Rogers et al., 2022). For instance, avatars that express interest with direct eye gaze to our presence and behaviour in VR are often rated as having a higher level of behavioural realism (Aburumman et al., 2022; Grewe et al., 2021; Wu et al., 2021).
Why is avatar expressiveness in VR important?
Avatar expressiveness is important because more expressive avatars are believed to provide a more engaging and interactive social experience that better parallels real-life social interaction (Kyrlitsias and Michael-Grigoriou, 2022; Rogers et al., 2022). Indeed, evidence suggests that social interaction with a full face and body motion-captured avatar is rated similarly to face-to-face interaction (Rogers et al., 2022). This indicates that motion capture is important for increasing the perceived animation realism of VR avatars.
The term social presence is used in the context of computer-mediated communication to describe the feeling that one is communicating with a real social actor (Biocca et al., 2003; Jung and Lindeman, 2021; Oh et al., 2018; Skarbez et al., 2017; Sterna and Zibrek, 2021). Many VR researchers aim for a higher level of perceived social presence because it can facilitate turn taking, gesturing and self-disclosure (Barreda-Ángeles and Hartmann, 2022; Felton and Jackson, 2021; Herrera et al., 2020; Oh et al., 2018; Smith and Neff, 2018). Social presence is assumed to be enhanced when avatar expressiveness is higher, as this helps to facilitate a stronger sense of overall behavioural realism.
In one study, Smith & Neff (2018) utilised rudimentary motion capture where participants were required to navigate two social tasks while face-to-face or in VR. Their results revealed that a basic motion tracked avatar with limited facial expressiveness was enough to evoke similar levels of social presence as face-to-face interaction. However, it remains unclear how different implementations of motion capture and actor expressiveness might affect perceived avatar animation realism and quality of social interaction in VR. Additionally, the relative importance of motion captured face and body gestures is not well understood. Therefore, we aim to extend this line of investigation by assessing participant experiences interacting with avatars with varying degrees of avatar expressiveness for face and body movement.
The importance of non-verbal signals from face and body during social interactions
Prior studies that have investigated non-verbal social cues have demonstrated the valuable contribution of facial cues when conveying emotions (Ferstl and McDonnell, 2018; Kokkinara and McDonnell, 2015; Sel et al., 2015; Seymour et al., 2021; Shields et al., 2012; Solanas et al., 2018; Thomas et al., 2022; Willis et al., 2011). Similarly, but to a lesser extent, body gesturing also makes a valuable contribution to the communication of non-verbal information (Willis et al., 2011; Shields et al., 2012; Solanas et al., 2018). Importantly, when paired together, both face and body avatar expressiveness has been shown to improve social interactions (Shields et al., 2012; Fink et al., 2015; Solanas et al., 2018). While still an emerging area of research, several studies have investigated the importance of non-verbal social cues for VR social interactions using motion capture technology (Herrera et al., 2020; Kruzic et al., 2020; Thomas et al., 2022). For instance, Kruzic et al. (2020) used full body motion tracking to investigate the effect of avatar expressiveness when the interaction was conducted through a computer monitor. They found that high levels of face expressiveness increased interpersonal attraction, impression formation and nonverbal synchrony. In their study, participants interacted by playing a guessing game where using gesture was advantageous for performing the task. Whether their findings apply to other communicative contexts (e.g., getting acquainted interaction, or a self-disclosure interview) where gesture is not so explicitly warranted is still largely unknown. Additionally, Kruzic et al. (2020) did not have participants share the same virtual space together, so it remains unknown if their findings generalise to HMD-based VR.
Another recent study by Herrera et al. (2020) examined participant perceptions of avatars in HMD-based virtual reality that varied in the amount of arm movement possible and found no differences for interpersonal attraction across conditions. However, the avatars in the Herrera et al. (2020) study were driven by the VR hand controllers rather than specialised motion capture equipment, and importantly their methods did not include facial motion capture. The limited use of motion capture may have affected the participant’s self-reported experience across conditions. Overall, these studies reveal that avatar face and body expressiveness is a factor that may influence user experience, yet it remains unclear if an avatar with higher expressiveness can improve VR social interaction more than an avatar with lower expressiveness.
The present study
We aimed to clarify how an avatar’s face and body expressiveness affects social interactions in VR. We accomplished this by examining a series of self-disclosure interactions to a user-driven avatar across four within-subject conditions with varying degrees of both face and body expressiveness. Nonverbal social cues are often needed to enhance the quality of a social interaction by promoting feelings of enjoyment, comfort and closeness (Sel et al., 2015; Seymour et al., 2021; Shields et al., 2012; Solanas et al., 2018; Willis et al., 2011). Because avatars with higher levels of expressiveness were expected to convey more nonverbal social cues, we expected that perceived realism would be positively correlated with interaction quality.
Similar to prior research, we measured the perceived realism of the avatar’s movement during the social interactions (Biocca et al., 2003; Felton and Jackson, 2021; Ferstl and McDonnell, 2018; Kokkinara and McDonnell, 2015; Kyrlitsias and Michael-Grigoriou, 2022; Oh et al., 2018; Sterna and Zibrek, 2021; Thomas et al., 2022). In our study, we expected that perceived animation realism would be highest when participants interact with an avatar with the highest level of both face and body expressiveness. In the communication literature, nonverbal signals from the face are typically viewed to have more importance than nonverbal communication from the rest of the body (Sel et al., 2015; Seymour et al., 2021; Shields et al., 2012; Solanas et al., 2018; Willis et al., 2011). Therefore, we expected that an avatar with a higher level of face expressiveness paired with lower body expressiveness would be rated as having higher realism than an avatar with a higher level of body expressiveness paired with lower face expressiveness.
In addition to perceived animation realism, we also had participants provide ratings on the perceived quality of the interactions. Using similar ratings, Rogers et al. (2022) reported that their participants perceived a higher level of interaction quality for the disclosure of positive memories compared to the disclosure of negative memories. We expected to replicate this finding of Rogers et al. (2022). Given that prior research has shown both face and body social signals increase the quality of a social interaction (Sel et al., 2015; Solanas et al., 2018; Willis et al., 2011), we also expected to find higher participant perceptions of interaction quality when they interacted with an avatar with higher levels of face and body expressiveness. Finally, the expressiveness of an avatar’s facial animations was expected to be more important for the social interaction when compared to body expressiveness. People typically focus more on facial expressions over body expressions when interpreting non-verbal social cues (Proverbio et al., 2018; Shields et al., 2012; Solanas et al., 2018; Willis et al., 2011).
Materials and methods
Participants
A total of sixty-one undergraduate psychology students were recruited for this study (45 females and 16 males, M age = 29.9 years, SD = 10.2, Min = 17, Max = 58). Table 1 shows the extent of participant experience using technology to communicate with others and playing games over the past 12 months. Most participants had at least some experience with screen-based interactions (95%), and a notable portion of participants (25%) had at least some experience with VR interactions.
Materials: Hardware
Two computers linked via LAN were used for this study. The computer resources required to run motion-capture and VR are intensive, so we decided to use two computers to split the resource requirements of several applications running at once. The first computer was an Intel i7-9700k CPU, 32GB DDR4 RAM, Nvidia GeForce RTX 2080 GPU, and 2TB HDD storage. The second computer had the same specifications, but with a more powerful Intel i9-11900k CPU. For the VR HMD, we used a HP Reverb G2 VR Headset. This HMD provides 2160 × 2160 pixels per eye with 90 Hz refresh rate to permit a crisper image compared to most other commercial HMDs (at the time of our study). For the motion capture of body movement, the Noitom Perception Neuron Studio Inertial System was used. This comprises 17 wireless inertial sensors for body motion capture and 12 sensors for the motion capture of the hands. The sensor parameters are: Gyroscope range = +/- 2000 dps; Accelerate metering ± 32°g; Minimum resolution 0.02°; Static accuracy roll 0.7, pitch 0.7, yaw two; Data calculation rate 750Hz; Data output rate 60–240Hz; Inference type = dual probes; Operating frequency band 2400 MHz–2483MHz; Operating current 40 mA. For the motion capture of the face, an iPhone 11 running the LIVE face application was mounted on a tripod and connected to the first computer via USB cable.
Materials: Software
The character (avatar) used for this study was designed with the Reallusion Character Creator v3.44 software, using the Headshot plugin to make the avatar appear like the researcher controlling it. This avatar was then transferred to iClone v7.93 software, where it was prepared for its integration into VR on the second computer. The movement data obtained from the Perception Neuron sensors were sent wirelessly to a transceiver and streamed to the Perception Neuron Axis Studio software installed on the first computer. The Axis Studio software maps the sensor data onto a character rig for the following sensor locations: For both left and right sides the shoulders, upper arm, lower arm (forearm), hands, fingers, upper leg, lower leg, and feet. Also, for the head, upper torso, and lower torso. Axis Studio links directly to the iClone software using the iClone Motion Live plugin and maps the movement data of the body rig from Axis Studio onto the iClone character model. The LIVE face application installed on the iPhone 11 also streams the movement data obtained from the face and renders this data directly onto the face of the character model using the Motion Live plugin within iClone. The integration of these motion capture applications into the iClone software allows for a face and body animated character (via motion capture) in real time that we used for the VR social interactions. For more information on the iClone software see: www.reallusion.com/iclone/.
Unreal Engine version 4.26 was used to create the virtual environment, see Figure 1. The virtual environment was designed to replicate a small virtual office room, furnished with a book cabinet, table and a few adornments to make the room appear more realistic and comforting. In the virtual world, the participants and avatar were on opposite sides of a virtual table and were approximately 2 m apart from each other. The iClone software communicated directly to the second computer through the Unreal Engine Live-link plugin. This plugin allowed us to transfer and link the avatar designed on the first computer to the second computer where it was animated within the virtual environment in real time. Our overall pipeline for running social interactions in VR was the same as that used by Rogers et al. (2022).
Materials: Measures
Two brief scales were used to measure the perception of animation realism and the quality of the social interactions. The perceived animation realism scale was adapted from the Networked Minds Social Presence inventory (Biocca et al., 2003) and the Temple Presence inventory (Lombard et al., 2009). The items we used included: 1) I felt like I was interacting with a real person, 2) Their facial expressions looked natural/realistic, 3) Their body movements looked natural/realistic. The social interaction quality measure was previously used by Rogers et al. (2022) where participants were asked to rate their level of enjoyment, comfort, and closeness experienced during the interaction. All items were scored within the range of: 1) Not at all 2) A little bit 3) Quite a lot 4) A lot, and 5) Extremely.
Initial reliability tests were conducted to assess the internal reliability of the perceived animation realism and quality interaction scales. The reliability for each scale was within acceptable range (Cronbach’s Alpha values range from 0.79–0.93), see Table 2. The items of each scale were summed and averaged to create a composite score for perceived animation realism and perceived quality of the interaction.
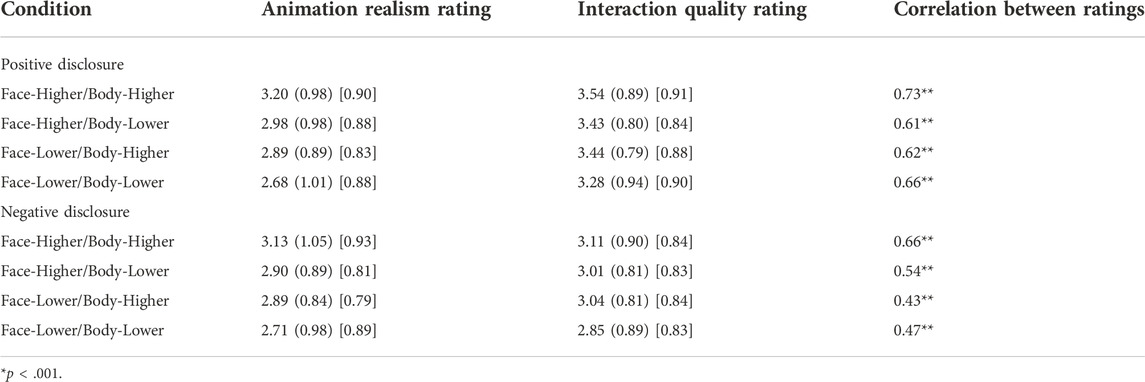
TABLE 2. Means, standard deviations (), Cronbach’s Alpha values [] including the correlations between animation realism and interaction quality scales.
Final post experiment questions were used to measure the participant preferences for the avatars. The same questions were presented as the previous two scales, but instead of participants rating each on the Likert response scale, participants indicated which of the four avatars they felt was the highest for the particular question. The preamble for this set of questions started with “Now that you have interacted with all four different versions of the avatar:” and then listed the questions as follows–“You enjoyed the most”, “You felt the most comfortable”, “You felt the strongest sense of closeness to your conversational partner”, “The avatar most felt like a real person”, “The avatar facial expressions looked the most natural/realistic”, “The avatar body movements looked the most natural/realistic”. Participants responded to these questions by choosing between interaction 1–4. We included these questions because we wanted to check if participants noticed any differences in avatar expressiveness after experiencing all interactions.
Procedure
Participants were first instructed to complete a pre-interaction survey. The survey required that participants provide their age and gender, as well as the extent of face-to-face, phone call, screen-based or VR communications they had over the past 12 months, and computer gaming. To prepare for the interactions, the participants were also required to write down four positive and four negative experiences. The details they were instructed to provide were to state 1) What the event was, 2) When it happened, 3) How it happened, and 4) How it made them feel. While the participants were completing these tasks, two researchers were preparing the motion-capture setup ready for the interaction. The first researcher (IB) prepared themselves by placing the sensors in correct positions along their body while standing in front of the iPhone mounted tripod, and the second researcher (AF) prepared the VR room for the interactions. For the interactions, the avatar was controlled by the first researcher a few meters across from where the participant was seated. This was decided so the participant could hear the researcher speak when conducting the interactions, see Figure 2.
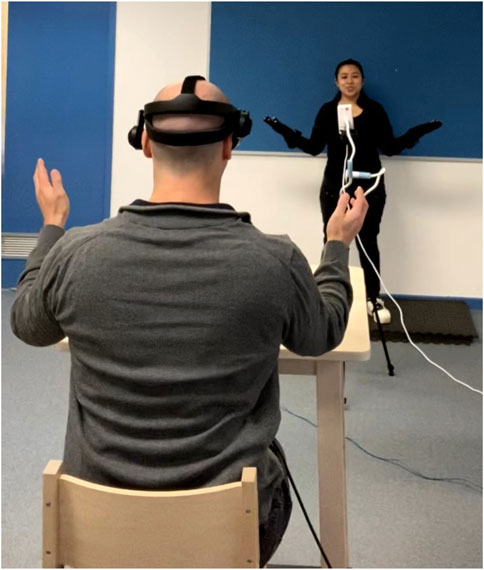
FIGURE 2. Participants were seated at a table approximately 3 m away from the researcher (IB) who was controlling the avatar with the motion-capture suit and iPhone 11.
After the participants finished their pre-interaction survey, the researcher placed the HMD comfortably over the participant’s eyes. Participants were asked to close their eyes while the researcher navigated their position in the virtual environment. This was done to avoid causing motion sickness. Participants were positioned on the opposite of the virtual table, facing the avatar, and were approximately 2 m apart from each other within the virtual environment. The participant did not possess an embodied avatar of their own. Once the participant was placed in the correct position, small adjustments were made to ensure that the avatar in front of them was initially perceived by the participant as maintaining eye-contact. This was confirmed with participants via verbal feedback from them. After these adjustments were made to the participant’s satisfaction, the first interaction was started.
Participants experienced four consecutive interactions where they were asked to recount their positive and negative experiences via the same four questions they answered initially (i.e., What, when, how it happened, and how it made them feel). The order of the positive and negative experiences was counter-balanced for each participant by having every second participant disclose their negative experiences first. The four avatar expressiveness conditions are distinguished by the level of expressiveness for the face and body: higher-face/higher-body, higher-face/lower-body, lower-face/higher-body, and lower-face/lower-body. The order of these conditions was counter-balanced across participants to reduce any order effects that may occur after familiarisation with the VR environment. The allocation of the ordering of conditions for each participant was determined using a Latin square method so that the ordering of conditions was spread evenly across the sample (Richardson, 2018).
Higher face expressiveness consisted of motion tracking of the eyes, brows, cheeks, lips, and chin, whereas lower facial expressiveness had no tracking for the brows, cheeks, and lips (see Figures 3A,B). Higher body expressiveness consisted of full body motion tracking using the Perception Neuron sensors, with the actor making hand gestures throughout the interaction. In the lower body expressiveness condition, the actor deliberately made minimal body movements by standing still, and keeping arms by their side, without gesturing throughout the interaction (see Figures 3C,D). The face expressiveness of the avatars was manipulated by changing the settings within the iclone7 software to restrict motion capture input from the brows, cheeks and lips. However, body expressiveness could not be manipulated through the Perception Neuron Axis Studio software, so the experimenter (IB) was instructed to voluntarily restricted their arm and body joint movements for the manipulations. A video with examples of avatar expressiveness across conditions can be found at: https://youtu.be/diH9AwK4gzs.
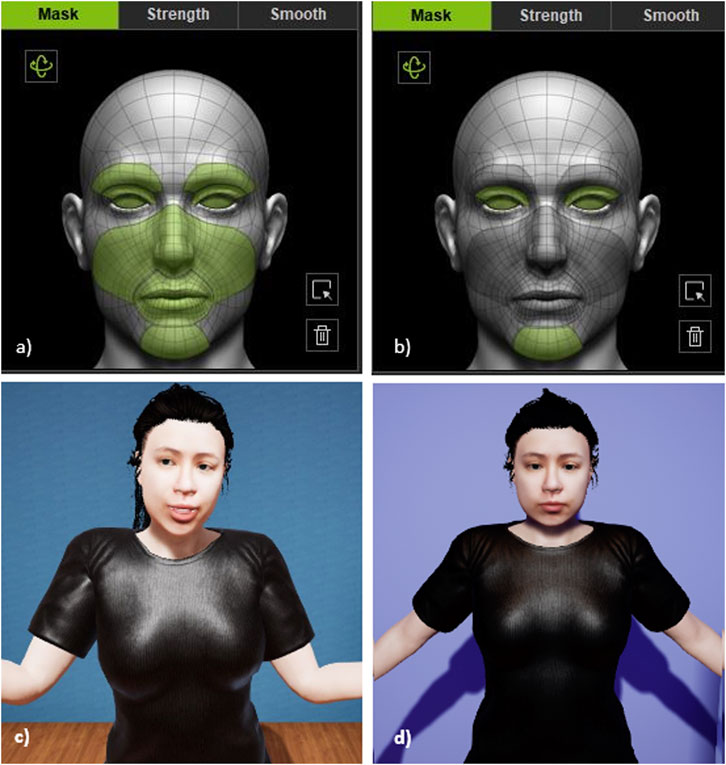
FIGURE 3. (A) shows the configuration with all facial features turned on for the high facial expressiveness condition using the iClone7 software. (B) shows the lower facial expressiveness configuration with the brow, cheeks and lips turned off to limit expressivity. (C) shows the avatar with high body expressiveness while in motion within the virtual room. Finally, (D) is how the avatar with low body expressiveness appeared.
After each positive/negative pair of disclosures, the second researcher helped the participant to remove the HMD and asked them to report on their experience via online survey. The participants reported on their perceived animation realism and interaction quality immediately after each pairing of negative and positive disclosures.
The positive/negative disclosure pairings typically lasted for approximately 5–10 min depending on the details the participant wished to share, for a total of about 40 min total across all four pairings. At the conclusion of all disclosures, participants filled in the final post experiment questions to indicate which avatar they most enjoyed interacting with, felt most comfortable with and felt the most closeness to. They also reported which avatar appeared most like a real person, had the most realistic face expressions, as well as had the most realistic body expressions.
Results
Ratings of avatar animation realism and quality of interaction
Descriptive statistics and correlations for the perceived avatar animation realism and quality of interaction ratings are provided in Table 2. Moderate positive correlations were observed between the perceived animation realism and interaction quality ratings. Two 4 × 2 repeated measures ANOVAs were carried out upon both the animation realism ratings and quality of interaction ratings. Two within-subjects factors included the expressiveness condition (Face-Higher/Body-Higher, Face-Higher/Body-Lower, Face-Lower/Body-Higher, Face-Lower/Body-Lower), and disclosure type (positive, negative).
For animation realism ratings, a significant main effect was found for condition (F (3,180) = 8.43, p < 0.001, ηp2 = 0.12). The pattern of means from highest to lowest followed our a priori expectations in order of: Face-Higher/Body-Higher (M = 3.16), Face-Higher/Body-Lower (M = 2.94), Face-Lower/Body-Higher (M = 2.89), Face-Lower/Body-Lower (M = 2.69). Planned contrasts revealed Face-Higher/Body-Higher to be significantly larger than Face-Higher/Body-Lower (F (1,60 = 4.66, p = 0.04, ηp2 = 0.07). No significant difference was observed between the ratings for the Face-Higher/Body-Lower and Face-Lower/Body-Higher conditions (F (1,60 = 0.28, p = 0.60). Lastly, ratings in the Face-Lower/Body-Higher condition were significantly larger than in the Face-Lower/Body-Lower condition (F (1,60 = 4.27, p = 0.04, ηp2 = 0.07). No significant main effect was found for disclosure type (F (1,60) = 1.18, p = 0.28, ηp2 = 0.02), and there was no interaction effect between condition and disclosure type (F (3,180) = 1.30, p = 0.28, ηp2 = 0.02). In sum, statistically the Face-Higher/Body-Higher was highest, the Face-Higher/Body-Lower and Face-Lower/Body-Higher were comparable, while Face-Lower/Body-Lower was the lowest.
For interaction quality ratings, while a significant main effect of condition was found (F (1,180 = 2.98, p = 0.03, ηp2 = 0.05), we note that the partial eta squared effect size was small, and none of the planned contrasts between the conditions were statistically significant (all ps ≥ 0.08). Overall, this suggests that interaction quality was not meaningfully different among the conditions. There was however a relatively large significant effect for disclosure type (F (1,60 = 45.98, p < 0.001, ηp2 = 0.43), with participants reporting greater interaction quality when disclosing positive experiences (M = 3.42) compared with negative experiences (M = 3.01).
Participant perceptions and preferences regarding the expressiveness conditions
In a set of post-experiment questions, participants rated which of the four avatar expressiveness conditions they felt was the highest regarding face and body realism, as well as which appeared most like a real person, see Figure 4. Non-parametric chi-square tests of homogeneity (also referred to as goodness of fit tests) were used to assess the frequencies. The avatar with higher face and body expressiveness was most frequently chosen as being the most like a real person (χ2 (3) = 10.67, p = 0.01), with the most natural facial expressions (χ2 (3) = 8.05, p = 0.05) and most natural body movements (χ2 (3) = 16.05, p = 0.001). Overall, more participants (39–45%) selected the higher facial and body animation as having the greatest perceived realism across the three realism items, compared to the other expressiveness conditions (8–26%), see Figure 4.
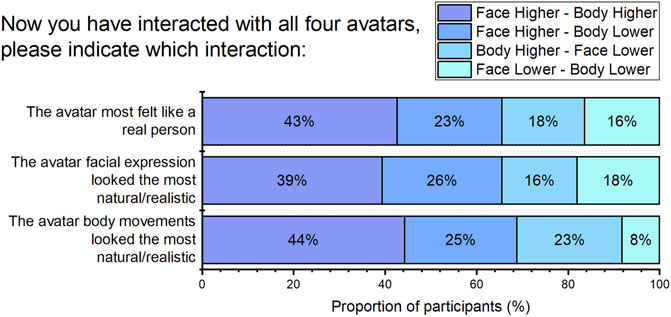
FIGURE 4. The post-experiment survey reporting the percentage of participants indicating a preference for a particular avatar based on the perceived animation realism questions.
In post experiment questions, participants rated the four avatar expressiveness conditions regarding which of those interactions they experienced the most enjoyment, comfort and closeness, see Figure 5. Non-parametric chi-square tests of homogeneity were used examine these frequencies. More participants selected the avatar with higher facial and body animation (36–41%) for greatest enjoyment (χ2 (3) = 9.23, p = 0.03) and comfort (χ2 (3) = 8.70, p = 0.03), compared to the other expressiveness conditions (16–25%). However, there was no significant difference in the proportion of participant selections for closeness (χ2 (3) = 4.11, p = 0.25) across the four expressiveness conditions.
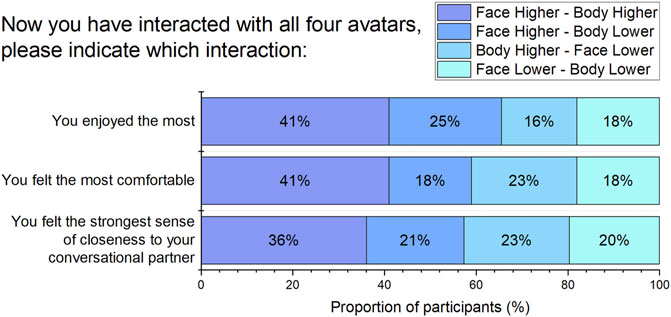
FIGURE 5. The post-experiment survey reporting The percentage of participants indicating a preference for a particular avatar based on the interaction quality questions.
Discussion
We investigated how different levels of face and body avatar expressiveness impacted upon participant perceptions of animation realism and interaction quality when disclosing positive and negative personal information in VR. In our study the avatar that participants interacted with was controlled in real-time by an experimenter via full face and body motion capture. Consistent with our expectations, we found that perceptions of animation realism and interaction quality were positively correlated (correlations ranged from 0.43 to 0.73). This supports the intuitive notion that a person who perceives more realistic avatar animation generally also tends to perceive a higher quality social experience. However, there also may be an influence of perceived interaction quality upon perceived animation realism. That is, a person experiencing a more engaging social interaction might be inclined to rate the animation realism more favourably. Teasing apart the precise nature of influence for this association is an avenue for future research.
In our study we systematically manipulated the extent of face and body expressiveness of the avatar to try and better understand the impact of varying levels of expressiveness on perceptions of realism and interaction quality. Overall, we found that avatars with a higher level of both face and body expressiveness (i.e., Face-Higher/Body-Higher condition) were rated by most participants (approximately 40%, see Figures 4, 5) as having the highest level of perceived avatar animation realism and interaction quality. This is consistent with prior communication literature that suggests both face and body movements contribute valuable social signals and complement each other to produce an overall more naturalistic communicative experience (Kruzic et al., 2020; Shields et al., 2012; Solanas et al., 2018; Willis et al., 2011). We also found that participants had higher interaction quality ratings for disclosing positive experiences compared with negative experiences, replicating a finding from Rogers et al. (2022).
Perceptions of animation realism in real-time social VR across different levels of avatar expressiveness
Our study reveals that people appear sensitive to variations in avatar expressiveness. In our study we measured participant perceptions of avatar realism in two different ways. First, we had participants rate three items after each interaction to provide a composite score of perceived animation realism. This composite score was found to be highest for the Face-higher/Body-higher condition, and lowest for the Face-lower/Body-lower condition. However, the conditions that had a mixed profile (i.e., Face-higher/Body-lower and Face-lower/Body-higher) did not differ from one another. Second, at the conclusion of the study we measured participant perceptions of avatar realism by requiring them to pick which of the avatars was the most realistic across three questions. More participants (i.e., approximately 40%) indicated that the avatar with the highest level of both face and body expressiveness was perceived as the most realistic, when compared to the other expressiveness conditions. Prior research has indicated that non-verbal facial cues are more important for conveying social emotions during a social interaction compared to non-verbal body language (Willis et al., 2011; Cao et al., 2020). Overall, while our study indicates that when paired together higher face and body expressiveness increases a sense of realism, we were not able to reliably discern any superiority of face over body.
Perceptions of interaction quality in real-time social VR across different levels of avatar expressiveness
We measured perceptions of interaction quality in the same manner as perceptions of animation realism. That is, we obtained a composite measure of interaction quality after each interaction (via Likert ratings of enjoyment, comfort, and closeness). We also obtained a preference rating at the end of the study for each of the quality dimensions. While our analysis of the interaction quality composite scores obtained after each interaction revealed an overall significant main effect of avatar expressiveness, follow up analyses suggested no large/meaningful differences among the different expressiveness conditions. However, the preference ratings indicated that most participants selected the avatar with higher facial and body animation for greatest enjoyment and comfort (but not closeness). The results are not as clear as what was found for perceived animation realism, but the preference ratings do indicate that higher expressiveness appears to be a factor that can impact upon perceived interaction quality. This is consistent with other research examining social interaction in other modalities such as face-to-face, video-chat, or conversations with virtual agents (Kokkinara and McDonnell, 2015; Sel et al., 2015; Solanas et al., 2018; Thomas et al., 2022; Willis et al., 2011).
Limitations and future research
A limitation of this study is that the participant was not embodied by their own self-avatar. Embodiment is an important feature in VR because it can affect how we interact with each other (Aseeri et al., 2020; Foster, 2019; Fribourg et al., 2020; Peck and Gonzalez-Franco, 2021; Smith and Neff, 2018). For example, possessing a body can facilitate the synchronisation of body movements, allows reference (via pointing gestures) to features in the shared environment, and regulate emotions by adjusting our own behaviour (via self-soothing gestures) (Peck and Gonzalez-Franco, 2021). However, it is important to consider that there may be individuals who prefer not to embody their own avatar while in VR (Foster, 2019). This may be true for individuals who prefer greater levels of interpersonal distance.
An intriguing avenue for future research exists regarding how people may have different preferences for the varying levels of avatar expressiveness that is most comfortable for them. In our study, 20% of participants selected the avatar with the lowest levels of avatar expressiveness (i.e., lower-face/lower-body) as being the most enjoyable, comfortable, and providing the strongest sense of closeness. We are unable to conclude if this was due to an increase of interpersonal distance provided by the lower expressiveness avatar. Furthermore, our sample primarily (74%) identified as female, so we were unable to control for gender differences. Including a larger and more diverse sample in a future study, with a trait anxiety measure, and assessing perceptions of interpersonal distance, will help to improve our understanding of this finding. Indeed, future VR social interaction applications using motion capture may include configuration options to set the level of expressiveness of the embodied avatar, or the avatars being interacting with. What benefits and shortcomings such control over body language expressiveness will have for social interaction across a wide range of communicative contexts in VR is an intriguing area for future research. We acknowledge that there are many hypothetical ways that the level of expressiveness of virtual characters might be manipulated, and we have only used one method in this study. Future studies are needed to better understand how different forms of manipulation of expressiveness impacts upon user experience.
A further limitation of our study was that we focused purely on a single type of social interaction (i.e., a structured interaction protocol where the participant was required to disclose positive or negative personal information). This meant that the participant was required to do most of the talking. The participants may have been more focused on speaking rather than paying attention to the expressiveness of their partner’s avatar. Other types of interaction where the conversational partner has a more active communicative role might result in more pronounced differences in participant perceptions of avatar realism and interaction quality. Having the participants perform different roles (e.g., being an interviewer versus and interviewee) with their interaction partner may help to differentiate when different levels of avatar expressiveness are most ideal. Future research is required to further tease out the relative importance of different levels of avatar expressiveness across different kinds of communicative contexts.
Conclusion
Our study investigated how face and body expressiveness of avatars influences perceived animation realism and interaction quality when disclosing personal information in VR with real-time motion captured avatars. We found evidence to suggest that perceived animation realism and perceived interaction quality benefits from higher levels of face and body expressiveness. We also found significant moderate positive associations between ratings of perceived animation realism and interaction quality. We therefore conclude that avatar expressiveness is important for perceived realism and interaction quality. Future research is needed to investigate if individual differences in preferred levels of face and body expressiveness exist across different contexts involving VR social interactions.
Data availability statement
The datasets presented in this study can be found in online repositories. The names of the repository/repositories and accession number(s) can be found below: https://figshare.com/articles/dataset/The_Association_Between_Perceived_Animation_Realism_of_Avatars_and_the_Perceived_Quality_of_Social_Interaction_in_Virtual_Reality/20180009
Ethics statement
The studies involving human participants were reviewed and approved by Edith Cowan University ethics committee. The patients/participants provided their written informed consent to participate in this study.
Author contributions
AF, SR, RH, and CS contributed to the conception and design of the study, AF and IB collected data. AF conducted statistical analyses. AF and SR wrote the first draft of the manuscript. All authors contributed to manuscript revision, read, and approved the submitted version.
Conflict of interest
The authors declare that the research was conducted in the absence of any commercial or financial relationships that could be construed as a potential conflict of interest.
Publisher’s note
All claims expressed in this article are solely those of the authors and do not necessarily represent those of their affiliated organizations, or those of the publisher, the editors and the reviewers. Any product that may be evaluated in this article, or claim that may be made by its manufacturer, is not guaranteed or endorsed by the publisher.
Supplementary material
The Supplementary Material for this article can be found online at: https://www.frontiersin.org/articles/10.3389/frvir.2022.981400/full#supplementary-material
References
Aburumman, N., Gillies, M., Ward, J. A., and Hamilton, A. F. de C. (2022). Nonverbal communication in virtual reality: Nodding as a social signal in virtual interactions. Int. J. Hum. Comput. Stud. 164, 102819. doi:10.1016/J.IJHCS.2022.102819
Arias, S., Wahlqvist, J., Nilsson, D., Ronchi, E., and Frantzich, H. (2021). Pursuing behavioral realism in Virtual Reality for fire evacuation research. Fire Mat. 45, 462–472. doi:10.1002/FAM.2922
Aseeri, S., Marin, S., Landers, R. N., Interrante, V., and Rosenberg, E. S. (2020). “Embodied realistic avatar System with body motions and facial expressions for communication in virtual reality applications,” in Proceedings - 2020 IEEE conference on virtual reality and 3D user interfaces, 581–582. doi:10.1109/VRW50115.2020.00141
Baccon, L. A., Chiarovano, E., and Macdougall, H. G. (2019). Virtual reality for teletherapy: Avatars may combine the benefits of face-to-face communication with the anonymity of online text-based communication. Cyberpsychol Behav. Soc. Netw. 22, 158–165. doi:10.1089/CYBER.2018.0247
Barreda-Ángeles, M., and Hartmann, T. (2022). Psychological benefits of using social virtual reality platforms during the Covid-19 pandemic: The role of social and spatial presence. Comput. Hum. Behav. 127, 107047. doi:10.1016/J.CHB.2021.107047
Biocca, F., Harms, C., and Burgoon, J. K. (2003). Toward a more robust theory and measure of social presence: Review and suggested criteria. Presence. (Camb). 12, 456–480. doi:10.1162/105474603322761270
Cao, Q., Yu, H., and Nduka, C. (2020). “Perception of head motion effect on emotional facial expression in virtual reality,” in Proceedings - 2020 IEEE conference on virtual reality and 3D user interfaces, 751–752. VRW. doi:10.1109/VRW50115.2020.00226
Capin, T. K., Noser, H., Thalmann, D., Pandzic, I. S., and Thalmann, N. M. (1997). Virtual human representation and communication in VLNet. IEEE Comput. Graph. Appl. 17, 42–53. doi:10.1109/38.574680
Felton, W. M., and Jackson, R. E. (2021). Presence: A review. Int. J. Human–Computer. Interact. 38, 1–18. doi:10.1080/10447318.2021.1921368
Ferstl, Y., and McDonnell, R. (2018). A perceptual study on the manipulation of facial features for trait portrayal in virtual agents. Front. Virtual Real 18, 281–288. doi:10.1145/3267851.3267891
Fink, B., Weege, B., Neave, N., Pham, M. N., and Shackelford, T. K. (2015). Integrating body movement into attractiveness research. Front. Psychol. 6, 220. doi:10.3389/fpsyg.2015.00220
Foster, E. M. (2019). “Face-to-Face conversation: Why embodiment matters for conversational user interfaces,” in Proceedings of the 1st international conference on conversational user interfaces - CUI ’19. doi:10.1145/3342775
Fribourg, R., Argelaguet, F., Lécuyer, A., and Hoyet, L. (2020). Avatar and sense of embodiment: Studying the relative preference between appearance, control and point of view. IEEE Trans. Vis. Comput. Graph. 26, 2062–2072. doi:10.1109/TVCG.2020.2973077
Grewe, C. M., Liu, T., Kahl, C., Hildebrandt, A., and Zachow, S. (2021). Statistical learning of facial expressions improves realism of animated avatar faces. Front. Virtual Real. 0, 11. doi:10.3389/FRVIR.2021.619811
Guye-Vuillème, A., Capin, T. K., Pandzic, I. S., Magnenat Thalmann, N., and Thalmann, D. (1999). Nonverbal communication interface for collaborative virtual environments. Virtual Real. 4 (1 4), 49–59. doi:10.1007/BF01434994
Herrera, F., Oh, S. Y., and Bailenson, J. N. (2020). Effect of behavioral realism on social interactions inside collaborative virtual environments. Presence. (Camb). 27, 163–182. doi:10.1162/PRES_A_00324
Higgins, D., Zibrek, K., Cabral, J., Egan, D., and McDonnell, R. (2022). Sympathy for the digital: Influence of synthetic voice on affinity, social presence and empathy for photorealistic virtual humans. Comput. Graph. X. 104, 116–128. doi:10.1016/J.CAG.2022.03.009
Jung, S., and Lindeman, R. W. (2021). Perspective: Does realism improve presence in VR? Suggesting a model and metric for VR experience evaluation. Front. Virtual Real. 0, 98. doi:10.3389/FRVIR.2021.693327
Kalra, P., Magnenat-Thalmann, N., Moccozet, L., Sannier, G., Aubel, A., and Thalmann, D. (1998). Real-time animation of realistic virtual humans. IEEE Comput. Graph. Appl. 18, 42–56. doi:10.1109/38.708560
Kokkinara, E., and McDonnell, R. (2015). “Animation realism affects perceived character appeal of a self-virtual face,” in Proceedings of the 8th ACM SIGGRAPH conference on motion in games, 221–226. MIG 2015 221–226. doi:10.1145/2822013.2822035
Kruzic, C., Kruzic, D., Herrera, F., and Bailenson, J. (2020). Facial expressions contribute more than body movements to conversational outcomes in avatar-mediated virtual environments. Sci. Rep. 10 (1 10), 20626–20723. doi:10.1038/s41598-020-76672-4
Kyrlitsias, C., and Michael-Grigoriou, D. (2022). Social interaction with agents and avatars in immersive virtual environments: A survey. Front. Virtual Real. 2. doi:10.3389/FRVIR.2021.786665
Latoschik, M. E., Roth, D., Gall, D., Achenbach, J., Waltemate, T., and Botsch, M. (2017). “The effect of avatar realism in immersive social virtual realities,” in Proceedings of the ACM symposium on virtual reality software and technology. VRST Part F131944. doi:10.1145/3139131.3139156
Lombard, M., Ditton, T. B., and Weinstein, L. (2009) In Proceedings of the 12th annual international workshop on presence. Measuring presence: The Temple presence inventory, 1–15.
Lugrin, J. L., Latt, J., and Latoschik, M. E. (2015). “Avatar anthropomorphism and illusion of body ownership in VR,” in 2015 IEEE virtual reality (VR), 229–230. doi:10.1109/VR.2015.7223379
Maurel, W., Thalmann, D., Wu, Y., and Thalmann, N. M. (1998). Biomechanical models for soft tissue simulation. Berlin: Springer. doi:10.1007/978-3-662-03589-4
Oh, C. S., Bailenson, J. N., and Welch, G. F. (2018). A systematic review of social presence: Definition, antecedents, and implications. Front. Robot. AI 5, 114. doi:10.3389/frobt.2018.00114
Owens, M. E., and Beidel, D. C. (2015). Can virtual reality effectively elicit distress associated with social anxiety disorder? J. Psychopathol. Behav. Assess. 37, 296–305. doi:10.1007/s10862-014-9454-x
Peck, T. C., and Gonzalez-Franco, M. (2021). Avatar embodiment. A standardized questionnaire. Front. Virtual Real. 0, 44. doi:10.3389/FRVIR.2020.575943
Proverbio, A. M., Ornaghi, L., and Gabaro, V. (2018). How face blurring affects body language processing of static gestures in women and men. Soc. Cogn. Affect. Neurosci. 13, 590–603. doi:10.1093/SCAN/NSY033
Rogers, S. L., Broadbent, R., Brown, J., Fraser, A., and Speelman, C. P. (2022). Realistic motion avatars are the future for social interaction in virtual reality. Front. Virtual Real. 0, 163. doi:10.3389/FRVIR.2021.750729
Roth, D., Lugrin, J. L., Galakhov, D., Hofmann, A., Bente, G., Latoschik, M. E., et al. (2016). Avatar realism and social interaction quality in virtual reality. Proc. - IEEE Virtual Real., 277–278. doi:10.1109/VR.2016.7504761
Sel, A., Calvo-merino, B., Tuettenberg, S., and Forster, B. (2015). When you smile, the world smiles at you: ERP evidence for self-expression effects on face processing. Soc. Cogn. Affect. Neurosci. 10, 1316–1322. doi:10.1093/SCAN/NSV009
Seymour, M., Riemer, K., and Kay, J. (2018). Actors, avatars and agents: Potentials and implications of natural face technology for the creation of realistic visual presence. J. Assoc. Inf. Syst. 19.
Seymour, M., Yuan, L., Dennis, A. R., and Riemer, K. (2021). Have we crossed the uncanny valley? Understanding affinity, trustworthiness, and preference for realistic digital humans in immersive environments. J. Assoc. Inf. Syst. 22, 591–617. doi:10.17705/1jais.00674
Shields, K., Engelhardt, P. E., and Ietswaart, M. (2012). Processing emotion information from both the face and body: An eye-movement study. Cogn. Emot. 26, 699–709. doi:10.1080/02699931.2011.588691
Skarbez, R., Brooks, F. P., and Whitton, M. C. (2017). A survey of presence and related concepts. ACM Comput. Surv. 50, 1–39. doi:10.1145/3134301
Smith, H. J., and Neff, M. (2018). “Communication behavior in embodied virtual reality,” in Proceedings of the 2018 CHI conference on human factors in computing systems. doi:10.1145/3173574
Solanas, M. P., Zhan, M., Vaessen, M., Hortensius, R., Engelen, T., and de Gelder, B. (2018). Looking at the face and seeing the whole body. Neural basis of combined face and body expressions. Soc. Cogn. Affect. Neurosci. 13, 135–144. doi:10.1093/SCAN/NSX130
Sterna, R., and Zibrek, K. (2021). Psychology in virtual reality: Toward a validated measure of social presence. Front. Psychol. 12, 705448. doi:10.3389/FPSYG.2021.705448
Stuart, J., Aul, K., Stephen, A., Bumbach, M. D., and Lok, B. (2022). The effect of virtual human rendering style on user perceptions of visual cues. Front. Virtual Real. 0, 58. doi:10.3389/FRVIR.2022.864676
Thomas, S., Ferstl, Y., McDonnell, R., and Ennis, C. (2022). “Investigating how speech and animation realism influence the perceived personality of virtual characters and agents,” in Proceedings - 2022 IEEE conference on virtual reality and 3D user interfaces, 11–20. VR. doi:10.1109/VR51125.2022.00018
Wiley, J., Pan, X., De, A. F., and Hamilton, C. (2018). Why and how to use virtual reality to study human social interaction: The challenges of exploring a new research landscape. Br. J. Psychol. 109, 395–417. doi:10.1111/BJOP.12290
Willis, M. L., Palermo, R., and Burke, D. (2011). Judging approachability on the face of it: The influence of face and body expressions on the perception of approachability. Emotion 11, 514–523. doi:10.1037/A0022571
Keywords: virtual reality, avatar, animation realism, face realism, body realism, social interaction, motion capture, avatar expressiveness
Citation: Fraser AD, Branson I, Hollett RC, Speelman CP and Rogers SL (2022) Expressiveness of real-time motion captured avatars influences perceived animation realism and perceived quality of social interaction in virtual reality. Front. Virtual Real. 3:981400. doi: 10.3389/frvir.2022.981400
Received: 29 June 2022; Accepted: 10 November 2022;
Published: 01 December 2022.
Edited by:
Qi Sun, New York University, United StatesReviewed by:
Anthony Steed, University College London, United KingdomPing Hu, Stony Brook University, United States
Copyright © 2022 Fraser, Branson, Hollett, Speelman and Rogers. This is an open-access article distributed under the terms of the Creative Commons Attribution License (CC BY). The use, distribution or reproduction in other forums is permitted, provided the original author(s) and the copyright owner(s) are credited and that the original publication in this journal is cited, in accordance with accepted academic practice. No use, distribution or reproduction is permitted which does not comply with these terms.
*Correspondence: S. L. Rogers, c2hhbmUucm9nZXJzQGVjdS5lZHUuYXU=