- 1Movement Control Rehabilitation (MOCORE) Laboratory, Altorfer Complex Stevens Institute of Technology, Hoboken, NJ, United States
- 2Department of Biomedical Engineering, Stevens Institute of Technology, Hoboken, NJ, United States
- 3Department of Computer Science, Stevens Institute of Technology, Hoboken, NJ, United States
Previous studies have demonstrated how augmented feedback can accelerate motor learning. Still, how specific feedback features of complexity and intermittency can influence learning a challenging, force-driven motor task remains largely unknown. This study is an initial investigation of how variations in the complexity and intermittency of augmented visual guidance affect the performance of an isometric muscle control task with a computerized platform. This novel platform has been developed to rehabilitate upper-extremity function after neuromuscular dysfunction (e.g., spinal cord injury, stroke) while utilizing: 1) a position-adjustable arm brace for gravity support; 2) a myoelectric command interface; 3) virtual reality (VR) for motor training. Results from this study elucidate new motor control principles and suggest how augmented guidance may be leveraged in designing VR motor rehabilitation programs, which are highly flexible and customizable to individual users. This study demonstrated that simpler and more intermittent feedback typically resulted in better performance (i.e., shorter computerized motion pathlengths). Supplementary results suggested these feedback modes also reduced cognitive loading (i.e., alpha/beta band magnitudes in electroencephalography) but increased physical arousal (i.e., higher skin conductance). In sum, this study indicates that for complex, force-driven tasks, augmented guidance must be presented selectively to accelerate gains in motor performance. This study suggests that simple and intermittent feedback avoids cognitively overwhelming the user while encouraging physical engagement that supports better performance.
Introduction
Neuromuscular traumas, such as spinal cord or traumatic brain injuries, often affect upper-extremity functional capabilities (Johnson and Griswold, 2017; Wyndaele and Wyndaele, 2006). Therefore, physical rehabilitation is commonly prescribed to aid in the recovery of motor task skills by training strength, flexibility, and neuroplasticity (Howard, 2017; Hakim et al., 2017). Previous studies have demonstrated how augmented sensory-driven cues encoding spatial errors can significantly improve motor training (Sigrist et al., 2013a; Young and Schmidt, 1992). Feedback tools as rudimentary as a mirror can provide real-time visual feedback that effectively guides spatial positioning (Sewall et al., 1988). Computerized interfaces with customizable displays or audio and haptic modules offer greater flexibility in delivering enhanced sensory feedback. For rehabilitation, informative augmented feedback cues are often presented to guide persons towards a target trajectory (Sigrist et al., 2013a). Previous research has determined that levels of complexity (amount of information provided) (Sanford et al., 2020; Wulf and Shea, 2002) and intermittency (frequency of providing information) (Sadowski et al., 2013; Sanford et al., 2021) in visual feedback can uniquely affect the consistency of achieved motor patterns, including those representing muscle activation (Sanford et al., 2021; Sanford et al., 2020). However, the visual feedback features of complexity and intermittency, and their potential effects on learned performance, have not been thoroughly examined or leveraged for virtual reality (VR) motor rehabilitation despite the highly programmable nature.
Virtual reality can readily support augmented visual feedback paradigms and perceptions of embodiment through avatars (Kilteni et al., 2012). For example, target trajectories could be transparent overlays between avatar body segments representing the user’s actual position in space separate from segments indicating the desired position (Blana et al., 2016). Additionally, computerized training environments can accommodate various command interfaces, including those driven by myoelectric control. Muscle activation patterns, as measured using electromyography (EMG), can be used to control virtual devices or game objects (Garcia-Hernandez et al., 2019). Computerized training environments are highly effective for training muscle activity patterns that can be leveraged for high-order function, including control over myoelectric devices (Antfolk, 2010; Perry et al., 2018). Highly complex EMG patterns can be classified as an equivalent command space using flexible machine learning structures (Walsh et al., 2021; Toledo-Pérez et al., 2019) that are customizable to individual users. Thus, computerized environments are powerful platforms for customizing rehabilitation interfaces in both the training regime and usability for persons of varying ability levels.
Virtual reality is an increasingly popular tool for motor rehabilitation (Sveistrup, 2004), including for upper-extremity function (Lim et al., 2020), because of how VR cognitively engages and motivates its users through colorful displays, immersive environments, and gamified constructs (Howard, 2017; Garcia-Hernandez et al., 2019; Zimmerli et al., 2013). Yet, the gains in motor function when using VR rehabilitation compared to conventional therapies are negligible when controlling for therapeutic dosage (Prasad et al., 2018). Thus, the potential for VR rehabilitation therapies has not been realized. It is crucial to investigate how customizable VR training elements, such as augmented guidance features, can be optimized for motor learning. Visual guidance, in particular, should be considered, given how VR is a visually-driven medium and because visual feedback has a central role in accelerated motor learning (Salmoni et al., 1984; Lee et al., 2016).
Augmented visual feedback improves motor task performance through trajectory-level guidance for either motion or muscle activation targets (Sigrist et al., 2013a). Compared to other sensory modalities, such as audio and haptic, visual feedback best guides spatial positioning during movement exercises (Sigrist et al., 2013b; Nesbitt, 2003). Two visual feedback features, complexity and intermittency, can uniquely affect performance gains (Sanford et al., 2020; Sanford et al., 2021) and could be optimized for rehabilitation. The optimal visual features for a particular training regimen can depend upon a wide range of co-factors, primarily how challenging the task is and a person’s current level of experience (Wulf and Shea, 2002; Wulf, 2013). Thus, sensory guidance features for rehabilitation may need to be strategically adapted throughout multiple training sessions as task difficulty is adjusted to a person’s changing skill level (Ávila-Sansores et al., 2013; Zimmerli et al., 2013).
Visual feedback complexity increases as more visual information, e.g., more concurrent targets (Sigrist et al., 2013a; Sanford et al., 2020), is presented to the user. The greater complexity of the performance task necessitates greater visual feedback complexity (Wulf and Shea, 2002). Still, highly complex feedback could generate cognitive overloading that deteriorates learning potential (Bannert, 2002), especially if the person interprets the information as extraneous or irrelevant to the task directive (Proteau et al., 1992). Given our previous findings (Sanford et al., 2020), we hypothesize that complex feedback can support better tracking of a primary target if the supplementary information is represented synergistically as a body-level movement.
Feedback intermittency alters the frequency of visual guidance presented during concurrent feedback training (Schiffman et al., 2006; Sanford et al., 2021). While continuous feedback is the constant provision of a person’s motor actions against desired trajectories, intermittent feedback is presented with reduced frequency. For example, bandwidth feedback reduces feedback frequency by removing augmented cues during periods of low error, i.e., error falls below a predetermined threshold. Intermittent feedback can progressively reduce the reliance on external guidance cues and better promote the development of intrinsic mechanisms, such as proprioceptive memory, resulting in better performance in retention (i.e., after training and with no augmented guidance) (Sadowski et al., 2013; Sanford et al., 2021). The guidance hypothesis suggests (Salmoni et al., 1984; Schmidt et al., 1989) that reducing the feedback frequency can enhance motor learning by promoting greater reliance on intrinsic mechanisms. Thus, we hypothesize that bandwidth feedback should result in greater post-training performance than continuous feedback, even for a myoelectric control task.
Previous studies have examined the effects of augmented sensory feedback for technology-enhanced motor rehabilitation platforms (Wannstedt and Herman, 1978; Kearney et al., 2019; Morone et al., 2021), including with focus on upper-extremity function (Carmeli et al., 2009; Van Dijk et al., 2005). However, identifying and applying specific feedback features (e.g., nature, timing, frequency, type) to most effectively precipitate desired motor learning outcomes for rehabilitation applications remains elusive (Molier et al., 2010). In this study, we investigated how the complexity and intermittency of augmented visual guidance can facilitate improved functional performance of a muscle-based (myoelectric command) training task for upper-extremity rehabilitation. We utilize a novel computerized platform that incorporates myoelectric control of a virtual robot avatar to perform reach-to-touch tasks while the participant receives augmented visual guidance during training. The task employs a position-adjustable brace of the upper extremity to support users, such as those with a spinal cord injury who are challenged to move their limbs against gravity (Ajiboye and Weir, 2005; Minkel, 2000). The brace holds the arm isometrically to support resistance strength and coordination training at varied arm positions (Oranchuk et al., 2019). Thus, we are fundamentally investigating the effects of variations in augmented guidance for the performance of a force-driven rehabilitation task (Alavi et al., 2015; Tiboni et al., 2018). Another crucial and novel element of our investigation is the examination of concurrent feedback, as previous bandwidth investigations have utilized terminal feedback (Salmoni et al., 1984; Schmidt et al., 1989). Concurrent feedback distinguishes itself by providing performance feedback during the action, while terminal feedback entails providing feedback about performance after the movement is completed (Park et al., 2000).
Furthermore, we measure and evaluate user-centered response variables to potentially explain an underlying mechanism in how augmented guidance may induce the observed performance patterns. Specifically, we assess participant perceptions in agency (Aoyagi et al., 2019) over the command interface. In addition, we measure the physiological stresses that are endured at cognitive [electroencephalography measures for loading (Kumar and Kumar, 2016)] and physical [electrodermal activity indicative of body arousal (Critchley, 2002)] levels. These physiological stresses indicate well-being during training, which may help further identify participant tolerance of various visual feedback modes and may be an additional dimension of person-specific customization of VR-based training.
Materials and methods
Human participation
Thirteen healthy participants signed an informed consent approved by the local Institutional Review Board. They were recruited from a university campus (Seven Females: 21.2 ± 2.0 years, 165.1 ± 3.7 cm, 56.5 ± 2.6 kg. Six Males: 22.3 ± 2.1 years, 179.3 ± 5.9 cm, 77.5 ± 6.4 kg). All participants were right-hand dominant, and the right arm was used for training. All participants were naïve to both the brace device and muscle-driven command interfaces. Individuals were excluded from participating if they reported any of the following: 1) Clinical diagnosis of cognitive or neuromuscular impairment. 2) Previous surgery to an upper extremity or the spine/neck. 3) Hearing or vision issues not correctable to normal levels. 4) Proneness to epileptic seizures due to visual stimuli.
Supportive brace apparatus
A novel computerized platform for isometric training of muscle function has been developed for motor rehabilitation of the upper extremity (Figure 1). The first principal component of this platform is a position-adjustable brace that isometrically supports the upper arm undergoing training. The brace was custom constructed using 3D printing and essential hardware components, allowing the user to assume variable arm configurations. Support at varying configurations can enable physical therapists to develop training programs that promote muscle strength and coordination at different muscle lengths (Folland et al., 2005; Noorkõiv et al., 2015), even for persons with severe motor dysfunction. In cases of severe motor disability, the brace provides gravity support to assume different configurations while a person can focus training effort on preserved muscles executing the computerized task. Fundamentally, the person will exert directional efforts against the brace’s padded interior (contact-side). In addition, isometric resistance from the brace helps to amplify the skin-surface EMG signals used to drive the motion of virtual avatars (Berger and d’Avella, 2017; Gordon and Ferris, 2004).
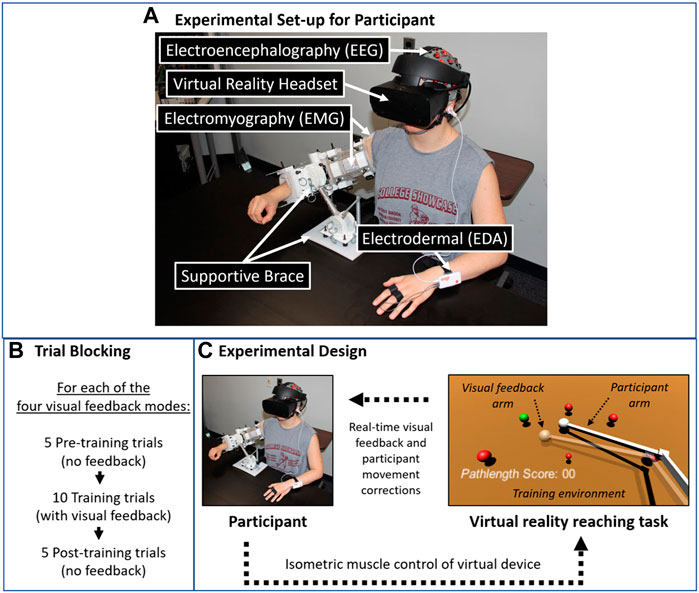
FIGURE 1. Experiment Overview. (A) Participant arm placed in supportive brace fastened to the table to apply isometric muscular exertions. Worn EMG sensors record myoelectric patterns to command the VR robot arm avatar. EEG and EDA signals additionally recorded to measure participant cognitive loading and physical arousal, respectively. (B) For each visual feedback mode, participants completed pre-training and post-training trials (with no feedback) before and after training trials (with visual feedback). (C) Isometric muscle control was used to command a virtual device through a variety of reaching tasks while receiving real-time visual feedback for making movement corrections to reduce error to the shortest path between targets.
The brace comprises an arm mount, an adjustable rod, and a secondary mount on the table directly in front of the participant. The arm mount component straps twice over the upper arm, once over the forearm, and allows adjusting and locking of the elbow angle. The arm mount has cut-outs for EMG sensors on the upper arm and forearm muscle mid-bellies. An adjustable rod attached to the forearm connects the arm mount to a second mount clamped to the table in front of the participant. The adjustable hinges on the mounts and rod allow the arm position to be adjusted at elbow and shoulder angles that are comfortable and within desired limits. In this study, we searched for arm positions deemed comfortable and neutral for each participant within the following angular ranges: shoulder ad/abduction (45–75°), shoulder internal rotation (0–45°), and elbow flexion (90–120°). We defined neutral as an arm position where participants perceived they could produce high forces in the four orthogonal directions (forward, back, left, right) used to command the virtual avatar (robot arm).
Measurement of physiological signals—electromyography, electroencephalography, and electrodermal activity
Fourteen wireless electromyography (EMG) sensors (Trigno Wireless EMG System, Delsys, Natick, MA, United States) were used to measure real-time muscle activity and provide the myoelectric inputs to control the virtual robot. EMG sensors were placed on the mid-belly of fourteen individual muscles of the arm and torso: brachioradialis, extensor digitorum, biceps brachii, triceps brachii, upper trapezius, middle trapezius, lower trapezius, infraspinatus, serratus anterior, latissimus dorsi, pectoralis major, anterior deltoid, lateral deltoid, and posterior deltoid. These muscles were identified as primary force-generating muscles in upper-extremity movements and targets for physical rehabilitation (Keenan, 1988; Reinold et al., 2007; Selkowitz et al., 2007; Fox et al., 2015). All EMG data were sampled at 1728 Hz.
A 64-channel electroencephalography (EEG) scalp-recording cap (g.USBamp, g. tec neurotechnology United States, Inc.) measured brain activity during all experiment phases. Power spectrum analyses were performed offline to identify mean power in alpha (8–12 Hz) and beta (13–30 Hz) frequency bands as measures of cognitive loading. Only seven of the thirteen participants were available to have EEG measurements taken during all experiment phases. All EEG data were sampled at 256 Hz. Electrodermal (EDA) activity was measured as a proxy for emotional and physical arousal based on increased skin conductivity (in microsiemen) of the left hand. Changes in galvanic skin response due to moisture were measured from electrode readings (Shimmer3 GSR + sensor, Shimmer, United States) at the index and middle fingers. Only four of the thirteen participants were available to have EDA measurements taken during all experiment phases. All EDA data were sampled at 51 Hz. All EMG, EEG, and EDA data were synchronized offline.
Survey measurement for perception of control
Immediately following each visual feedback block, participants completed a survey inquiring about their perception of control of the virtual avatar during training. Only ten of the thirteen eligible participants completed the survey. The survey asked participants to rate their level of agreement on a scale of 1 (disagree) to 100 (agree) with a single statement. The statement reflected the sense of agency (Moore and Obhi, 2012) and read as: I was in full control of the virtual prosthetic arm during training.
Utilizing support vector machines for electromyography classification
A support vector machine (SVM) (Toledo-Pérez et al., 2019) was used as the machine learning classifier for translating EMG activation patterns (14 muscle inputs) to direction outputs to be used as commands for the end-effector of the virtual robot arm. SVMs were trained uniquely for each participant. During pilot testing with our platform, we attempted to use a single SVM to output eight directional commands (four orthogonal, four diagonal). However, the single SVM produced challenges in EMG control of multiple degrees of freedom, as is well-cited (Parajuli et al., 2019), and participants reported poor intuitive control. Thus, we alternatively created an ad hoc command architecture using two SVM structures in parallel. One SVM was trained to identify forward and backward command directions, and the other was separately trained to identify right and left command directions. Training trials with diagonal data were included in both classifiers. A manual threshold was specified in series with classifier output to denote “no movement” of the end-effector when average EMG activity across all muscles was within 20% of the baseline (i.e., resting periods between target plateaus) EMG amplitude, presumably from sensor noise or hyperactivity at rest.
The main consequence of this command scheme was that participants primarily utilized sequences of diagonal movements (i.e., both SVM classifiers were producing command outputs concurrently) to move towards target locations. Our platform justified this approach since participants reported seamless and natural control of the robot avatars. This perception may partly explain endpoint stiffness regulation as a function of arm posture (McIntyre et al., 1996), and diagonal translations may better align with user endpoint forces. However, considerations of mapping arm posture to endpoint force synergies were beyond the scope of this study and held secondary to finding arm postures accommodating user comfort and stated preferences. More sophisticated approaches to the command interface may be enacted in future deployments, including those that better facilitate robust and concurrent control of multiple degrees of freedom. Still, the current scheme provided a sufficiently stable and consistent interface to discern performance effects due to variations in visual feedback features, as this study’s main objective.
For classifier training, each participant would be placed in the brace to perform voluntary isometric contractions in specific directions as instructed by the experimenter. First, the participant would perform maximum voluntary isometric contractions (MVIC) in the four orthogonal directions: forward, back, left, and right. From these trials, we identified the average EMG across all muscles signifying 100% MVIC for normalizing target force levels during training trials. Second, data to train an SVM were collected in sixteen individual trials within the VR environment. In each trial, participants would exert effort at one of two force level targets, 20% or 40% MVIC, in one of eight movement directions, the four orthogonal directions, and their corresponding four diagonal directions in that same plane. If they reached and exceeded the force target, the virtual end-effector would slowly start moving in the intended direction to encourage them to maintain that force level. Participants maintained an isometric hold for no longer than 12 s at the desired force level for each trial, resulting in over 20,000 data points collected per trial. A total of 10,000 sample points of EMG activity were extracted (centered within an isometric hold) for each movement direction and utilized for SVM training (“svm” function in Matlab). As demonstrated in our prior work that characterized machine learning classifier outputs for a similar version of this platform (Walsh et al., 2021), we achieved over 90% in classification accuracy, precision, and recall for SVM training when utilizing all fourteen muscle inputs. The 90% accuracy threshold was universally reached for all participants within the single session of training. Real-time input data to each SVM was provided as the root mean square filter with a window of 200 samples for the fourteen EMG sensors.
Virtual reality task environment for training and testing
The 3-D VR task environment (Figure 2) was primarily comprised of a robot arm whose end-effector moves within the transverse plane (forward-back-right-left) based on SVM outputs commanded by the participant’s myoelectric patterns. The remainder of the robot-arm linkage follows the end-effector according to inverse kinematics (Kucuk and Bingul, 2006). The end-effector moved towards target locations (marked by spheres) for both training and testing trials of functional performance. Participants were instructed to pursue targets as quickly as possible while adhering to the shortest pathlength between targets. Participants were informed that performance was positively assessed by minimizing the end-effector pathlength. Participants performed reach-to-touch tasks with the robot arm either in training trials with augmented visual guidance or during testing trials with no added feedback. With point-to-point reaching, efficient (shorter pathlength) trajectories are desired. For this motor task, modulated by EMG-level control, deviations from an ideal straight-line path are relatively small, but discernible. As such, augmented visual guidance of the original straight-line trajectory provides a clear and persistent reference path for participants to maintain during an entire training reach. Presumably, larger deviations, such as those induced by perturbations, would invoke motor planning and execution of a new target trajectory. For training trials, there are five target spheres arranged equidistantly (at 0°, 45°, 90°, 135°, and 180°) from the starting position for conducting a point-to-point reaching task (Yue et al., 2002). A color change of a random target sphere would cue the participant to command the end-effector to reach and contact that target before immediately returning to the starting position and pursuing the next target. The five targets were arranged randomly for testing trials, and participants could choose the order to contact all targets serially. Allowing participants to select the order of pursued targets strategically supports the development of motor control (Levin et al., 2015). Participants were informed about their pathlength during training through a “Pathlength Score” display to facilitate learning with knowledge of results (Cirstea and Levin, 2007) and score gamification (Kern et al., 2019). Pathlength score was explicitly computed as the ratio of the minimum pathlength (straight line distance) between targets over the actual pathlength traversed by the end-effector multiplied by 100. For the participant, score interpretations were intuitive, whereby the goal was to achieve a score as close to 100 as possible. Point-to-point reaching tasks have been used extensively for evaluating motor control (Won and Hogan, 1995; Ghafouri and Feldman, 2001), including monitoring the functional recovery of the upper-body with rehabilitative therapies (Dipietro et al., 2009; Celik et al., 2010; Zhou et al., 2013).
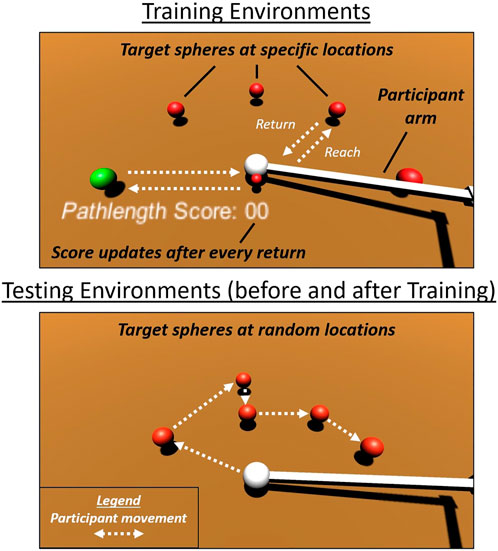
FIGURE 2. Three-dimensional virtual reality task environment shown for robot arm (white) under myoelectric control to make contact between its end-effector and target spheres. TOP) During point-to-point “training”, the targets were positioned equidistantly from a central initial position as the participant received augmented visual guidance in moving to (reach) and from (return) the targets. BOTTOM) During “testing”, augmented visual guidance was removed and targets were randomly positioned, and participants pursued targets in series and in the sequence order they choose.
Visual feedback modes utilized for augmented training guidance
Augmented training guidance in this study was presented as visual cues to suggest participant deviations from optimal (shortest) pathlengths between initial positions and targets. A second “ghost” (semi-transparent) robot avatar was presented concurrently as a guide against the participant-controlled avatar during training. The end-effector position for the guide avatar was a projection of the participant-controlled avatar onto the optimal pathlength. Four modes of augmented visual feedback were created through concurrent variation of complexity (amount of visual information) and intermittency (frequency of visual information), with each feature tested at two levels (Figure 3).
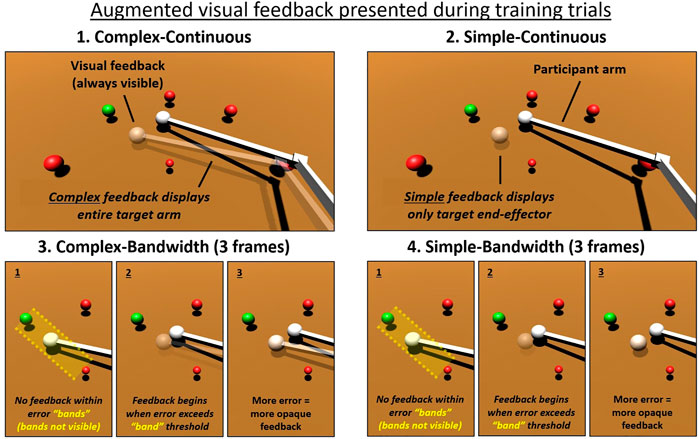
FIGURE 3. Visual Feedback Modes for Augmented Guidance during Training. All visual feedback modes project variations of a semi-transparent guide robot arm that follows the shortest (straight line) pathlengths between initial positions and active targets.
Guidance complexity was specified as either simple, by displaying only the end-effector of the guide robot, or complex, which also displayed the guide robot’s arm linkage. The arm linkage of the guide robot similarly follows inverse kinematics of its end-effector and does not inherently provide additional feedback about the performance error. However, our previous work (Sanford et al., 2020) suggested that additional visual cues, even if redundant due to biomechanical coupling, may facilitate better motor learning if they signified greater body representation, e.g., serial sequence of body segments. In this previous study, the performance variable of interest was the thigh angle during the squat. A serial body linkage improved motor performance by adding additional visual information about torso and shank segments. Thus, we seek to investigate if such feedback may positively contribute to motor learning despite the fundamental motor task difference of isometric, i.e., force-driven, avatar control.
Guidance intermittency was specified as either continuous, whereby the guide avatar is always present, or bandwidth, whereby augmented guidance was provided only if position error exceeded a particular threshold. This study specified the threshold as the mean error for a given participant during two practice training as part of initial accommodation. When this error is exceeded, a semi-transparent version of the guide arm appears and becomes opaquer in proportion to increasing error. The guide arm is fully opaque at twice the error magnitude of the threshold value. Pilot experimental sessions for this study and our previous work have indicated that modulating transparency of the guide arm in proportion to error magnitude ensured that intermittent transitions in feedback are not perceived as jarring to participants. The guide arm was presented at 20% transparency for continuous feedback modes. Pairing each unique level of one feature to another feature resulted in four visual feedback modes: 1) complex-continuous, 2) simple-continuous, 3) complex-bandwidth, and 4) simple-bandwidth. Since the motor learning benefits of augmented feedback have been generally established (Sigrist et al., 2013a), we chose to exclude a baseline training case with no visual feedback. As such, we limited our scope of examination to discriminating performance effects due to variations in features of augmented feedback. Furthermore, we randomize the order of presentation of visual feedback modes to ensure that any progressive learning effects across the session are distributed across modes with post-experiment analyses.
Experimental protocol
Each participant completed a single session that evaluated the effects of all four visual feedback modes within 4 hours. The participant donned the upper-arm brace and had all skin-surface physiological (EMG, EEG, EDA) sensors placed upon arrival. Immediately after, several accommodation procedures occurred, including 1) brace adjustment for comfort and neutrality, 2) participant selection of an avatar end-effector speed (three speed choices presented), 3) between one and 2 minutes gaining experience commanding the virtual robot. Before testing each visual feedback mode, a couple of practice trials were conducted to determine baseline average performance errors (optimal pathlength deviations) and bandwidth thresholds. For each of the visual feedback modes, each participant underwent a three-block trial sequence: 1) Five testing trials (pre-training), 2) Ten training trials (training with augmented visual guidance), 3) Five testing trials (post-training). As mentioned, the order of visual feedback modes was randomized for each participant. Each trial was separated by 15 s, and a 15-min rest break separated each three-block sequence for a visual feedback mode to mitigate fatigue effects. Participants were further queried intermittently throughout the session about their feelings and if they required an additional break. During the 15-min rest break, participants were relieved from wearing the headset. Participants were informed that they could undertake additional breaks, or exit the protocol, as needed (e.g., dizziness). No adverse events were observed or reported with the participants recruited for this study.
Data and statistical analysis
All statistical analyses were performed using the Statistics Toolbox of MATLAB® (Mathworks Inc., Natick, MA, United States). The mean values of all metrics for performance (completion time and pathlength score), perception (agency survey), and physiological engagement (alpha- and beta-band EEG activity for cognitive loading; EDA for physical arousal) were evaluated for each participant, visual feedback mode, and block of testing or training trials. This study’s analysis of central interest was the relative performance change between post-training and pre-training trials for each visual feedback mode. We further evaluated the change in physiological measures from pre-training to either training or post-training. We also observed participant sense of agency for each visual feedback mode used for training. A two-factor Friedman (two-way ANOVA by ranks) test was performed for each metric to identify significant differences across factors of complexity and intermittency, followed by a post hoc test when significant differences or interactions were observed. Additionally, we examined whether significant positive or negative coupling existed between performance (pathlength score) and either EEG or agency depending on the visual feedback mode. Specifically, we applied a simple linear regression to each scatter plot (one per feedback mode) of participant mean values pairing performance against either EEG or agency utilizing the “regstats” function in Matlab. We then further identified if the slope parameter was positive or negative and significantly different (p < 0.05) from zero based on t-stat p-values, also extracted from “regstats.”
Results
Pathlength score and completion time
Results for both performance variables (pathlength score, completion time) are reported as the mean across participant-level averages within each block of trials. For each participant, the performance results during post-training blocks are divided (normalized) from those for pre-training to suggest the relative change in performance due to training with a particular visual feedback mode. When performing a multi-variate analysis (MANOVA) for both performance variables (pathlength, completion time), a significant difference (p = 1.8 E-08) was observed across the independent variable of visual feedback modes. Figure 4 presents the results for pathlength score alone. For the factors of complexity and intermittency, the two-way ANOVA indicated a significant difference for pathlength score based on complexity but not for intermittency (Table 1). No significant interactions were observed between these factors for either performance metric. The normalized pathlength score was significantly higher (p = 0.0461) for simple feedback modes compared to complex modes. When examining individual feedback modes (Table 2), simple-continuous feedback generated better pathlength performance compared to both complex-continuous (p = 0.0293) and complex-bandwidth (p = 0.0449). Furthermore, all individual visual feedback modes demonstrated an improvement in pathlength score during post-training compared to pre-training (i.e., normalized value greater than 1). Figure 5 presents the mean completion times during post-training when normalized by pre-training averages. Significant differences in completion time were not observed between pairs of individual visual feedback modes.
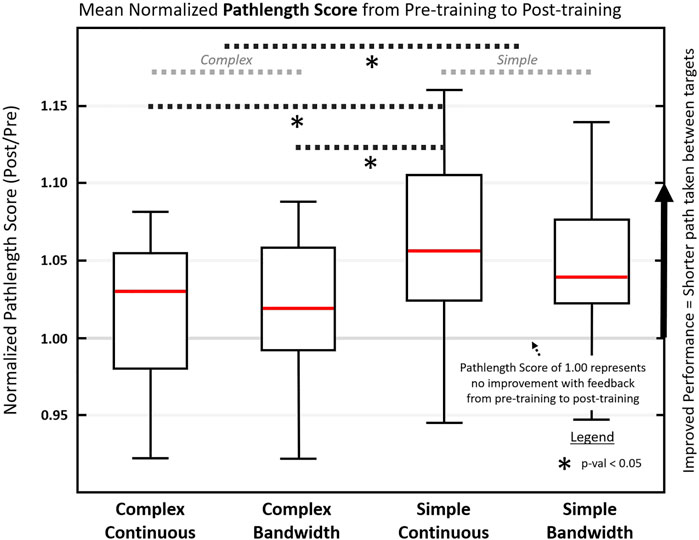
FIGURE 4. Pathlength score results indicate relative change in performance of pathlength minimization after training as score in post-training is divided (normalized) by pre-training for each participant.
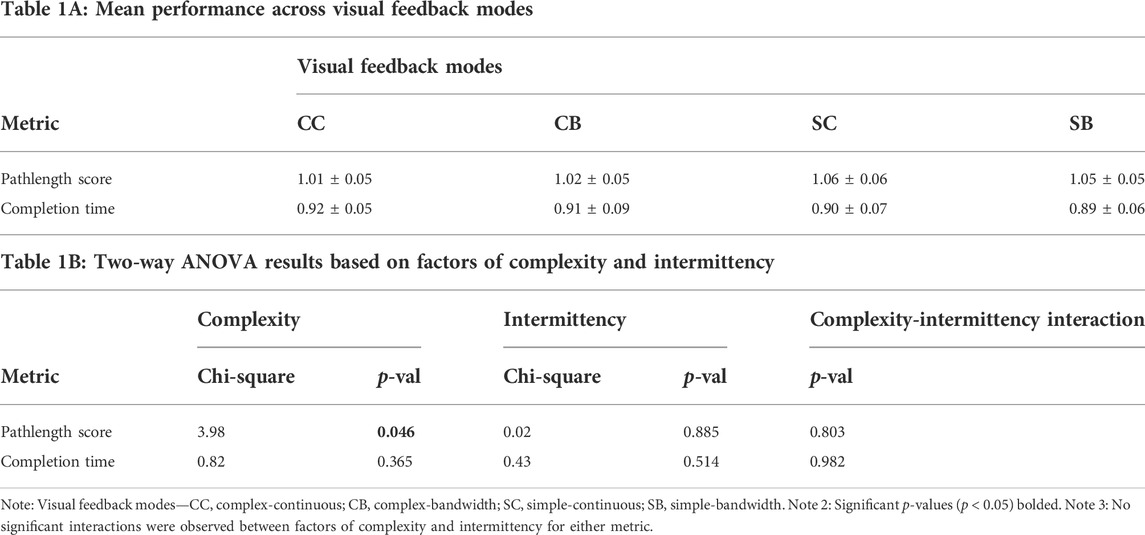
TABLE 1. Performance results as the relative change from pre-training to post-training (post/pre ratio per participant).
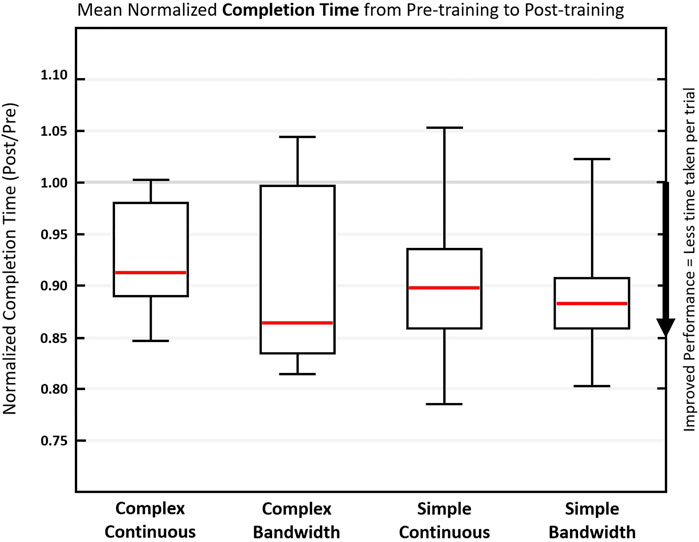
FIGURE 5. Results indicate relative change in trial completion time performance after training as mean time in post-training is divided (normalized) by time in pre-training for each participant.
Electroencephalography
Figure 6 presents EEG data for alpha and beta powers measured across all channels, observed as relative changes from pre-training to training (Train/Pre ratio) or pre-training to post-training (Post/Pre ratio). Significant differences in EEG were observed based on intermittency (Table 3) and across individual feedback modes (Table 4). Significant differences were observed only for Post/Pre for the alpha band. Continuous feedback resulted in significantly higher (p = 0.0116) EEG activity than bandwidth (intermittent) feedback. No significant interactions were observed between factors of complexity and intermittency. Additionally, complex-continuous (p = 0.0318) and simple-continuous (p = 0.0014) resulted in significantly higher EEG activity compared to simple-bandwidth. For the beta band, complex-bandwidth generated significantly higher (p = 0.0384) EEG activity during training than simple-bandwidth. Figure 7 presents a brain map of EEG alpha band activity averaged over all participants for simple-continuous and simple-bandwidth (Post/Pre). The simple modes are further examined since they produce better performance than complex modes. The higher alpha band activity preserved in post-training was generally distributed across the entire brain, including motor and sensory areas, suggesting a shift to continuous feedback produced a uniform effect on brain activity.
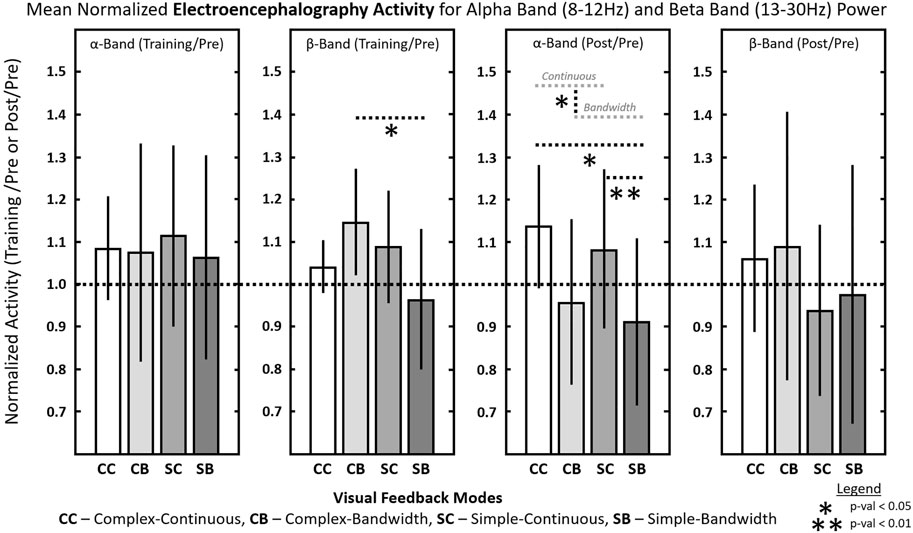
FIGURE 6. Electroencephalography results indicate change in alpha or beta band power during either training or post-training from pre-training.
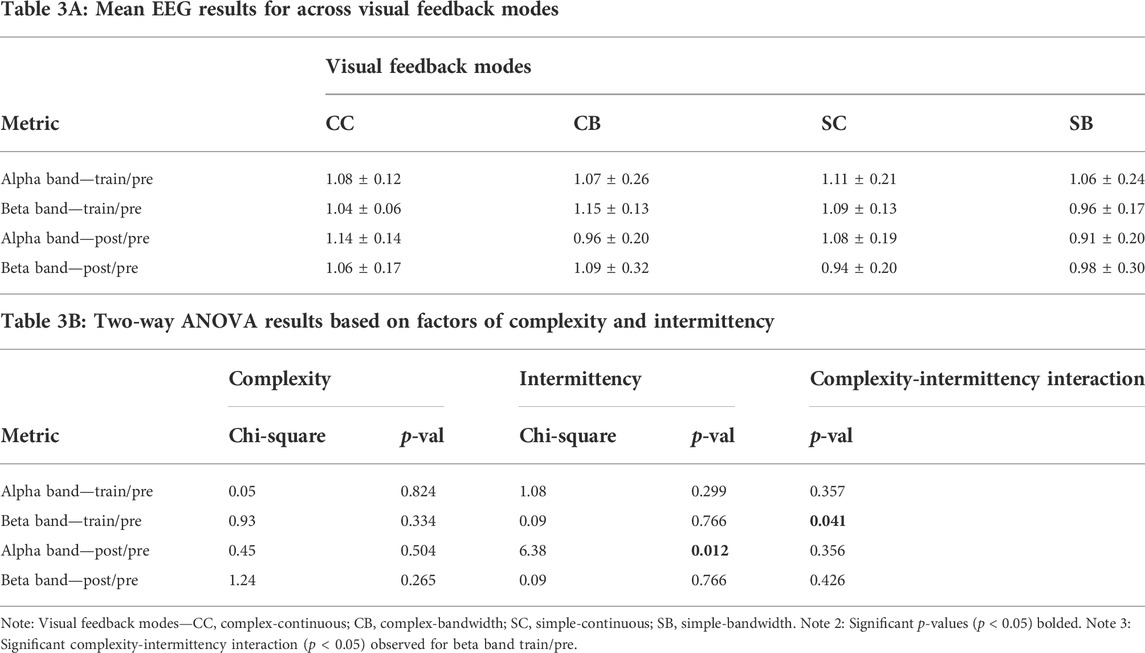
TABLE 3. Electroencephalography alpha band (8–12 Hz) and beta band (13–30 Hz) power as the relative change from pre-training to training (train/pre) and pre-training to post-training (post/pre).
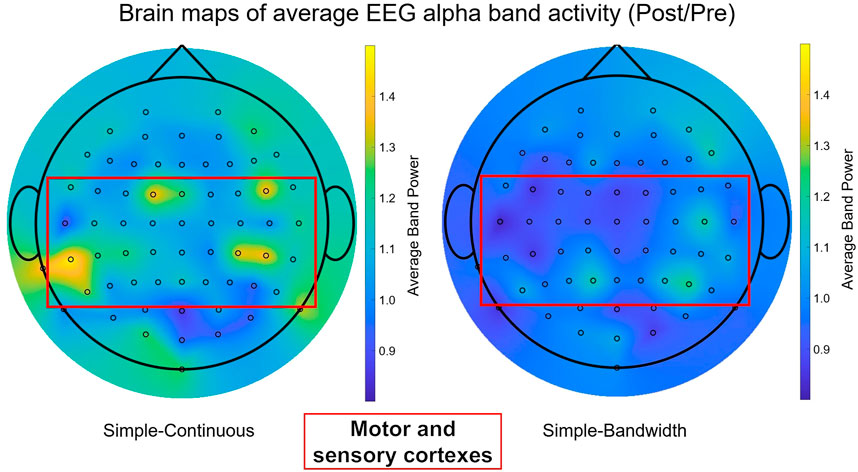
FIGURE 7. Brain map of EEG activity averaged for all participants. Electroencephalography (EEG) results to indicate changes in average alpha band activity during post-training from pre-training for simple-continuous (LEFT) and simple-bandwidth (RIGHT).
Electrodermal activity
Figure 8 presents the relative changes in electrodermal activity for each visual feedback mode, from pre-training to training. Significant differences were observed for the factor of complexity and across individual feedback modes (Table 5). No significant interactions were observed between factors of complexity and intermittency. Simple feedback resulted in significantly higher (p = 0.0239) skin conductance during training than complex feedback. Furthermore, complex-bandwidth feedback resulted in significantly lower conductance that either simple-continuous (p = 0.0377) or simple-bandwidth (p = 0.0218).
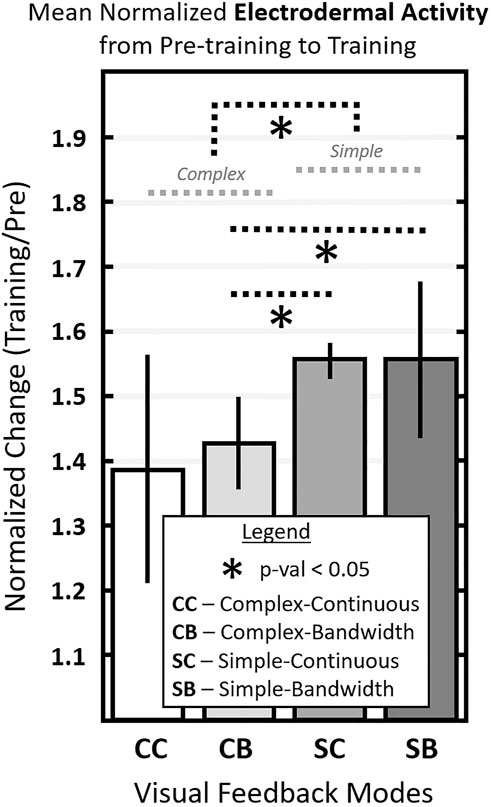
FIGURE 8. Relative change in electrodermal activity results during training compared to pre-training with each feedback mode.
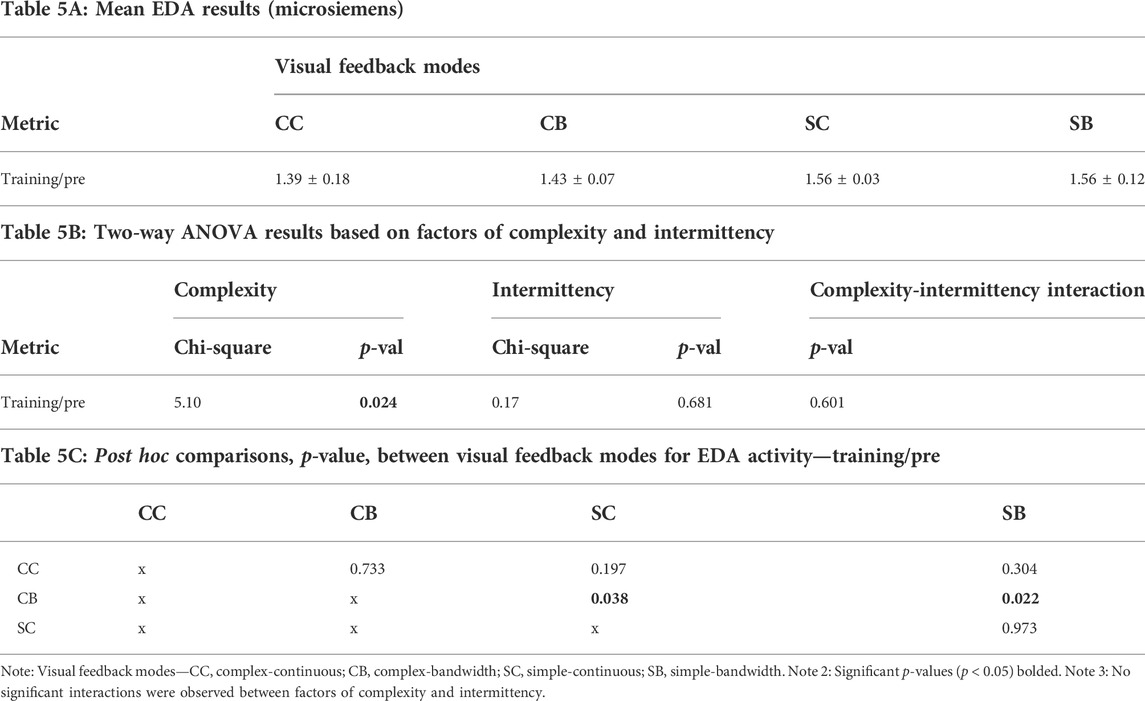
TABLE 5. Electrodermal activity during training with each feedback mode. Results presented as the mean skin conductance during training divided by pre-training (train/pre).
Agency (survey) results
The mean survey score (80.65) for the perception of control (agency) was normalized for each participant by subtracting the mean across visual feedback modes to highlight better the model-level differences in survey scores (Figure 9A). The mean values for each feedback mode were: complex-continuous = 83.5/100, complex-bandwidth = 81.6/100, simple-continuous = 81.5/100, simple-bandwidth = 76/100. A significant difference (p = 0.0249) was observed between complex-continuous and simple-bandwidth. No significant differences were observed based on factors of complexity or intermittency. Figure 9B plots agency against pathlength performance (relative change in score from pre-training to post-training).
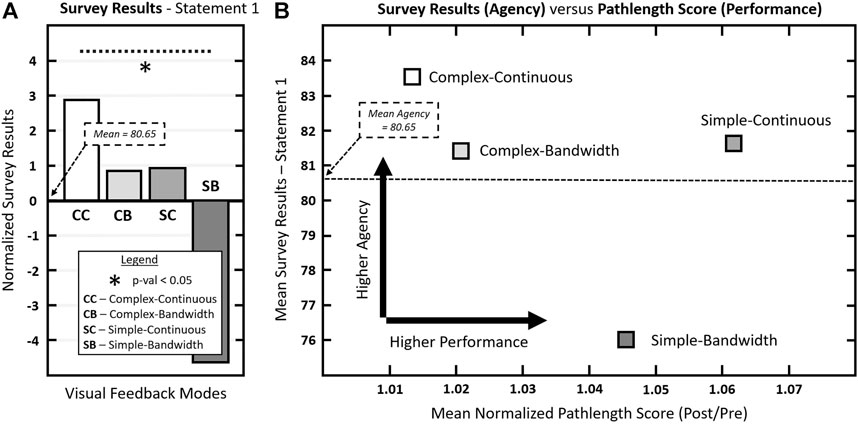
FIGURE 9. Survey results. (A) Survey score results for agency for each visual feedback mode. (B) Agency results versus relative change (pre to post) in pathlength score performance.
Electroencephalography and agency versus pathlength score per visual feedback mode
EEG and agency results were both evaluated against Pathlength Score across participants for each feedback mode (Figure 10). Participant mean values for EEG alpha band power and agency data were scatter-plotted against respective Pathlength Score values for post-training/pre-training for each visual feedback mode. A simple linear regression model was fitted to these data for each mode. We sought to verify whether the slope parameter was significantly different from zero to indicate apparent performance dependencies, either positive or negative, on cognitive loading (EEG) or action-driven perception (agency). The linear regression model parameters for the y-intercept and slope were extracted for each feedback mode. For three feedback modes, slopes were positive for EEG versus performance results. For three feedback modes, slopes were negative for agency versus performance results. The p-values, based on the t-stat to indicate significant difference from zero, for regression parameters are provided for each feedback mode in Figure 10. Ultimately, a significant correlation, as indicated by a significantly non-zero slope parameter, was not observed here for any feedback mode. These results suggest either a lack of correlation between these variables across feedback modes or an insufficient number of participant data points.
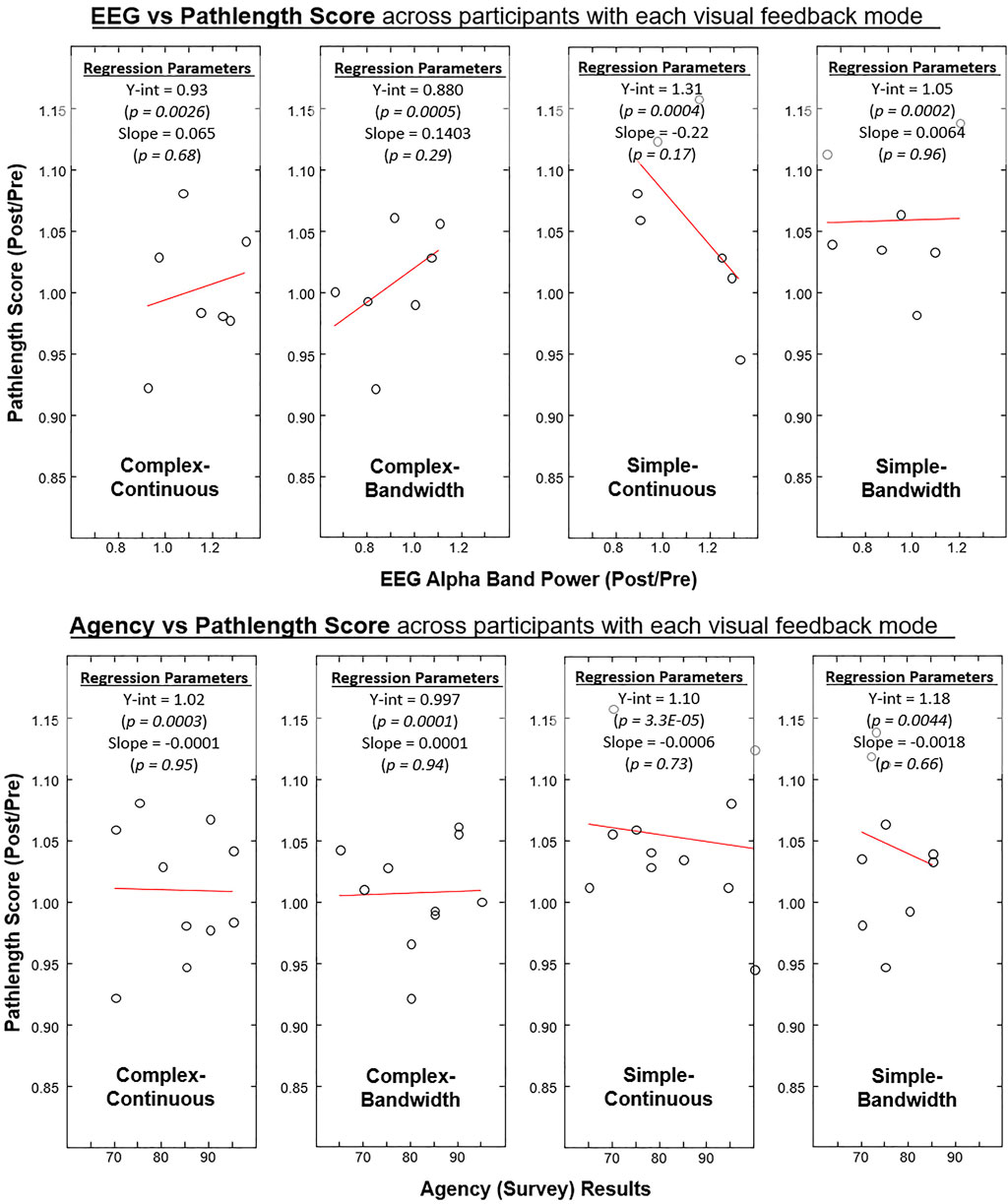
FIGURE 10. EEG and Agency versus Pathlength Score per Visual Feedback Mode. TOP) EEG alpha band power and pathlength score both presented as post-training/pre-training. BOTTOM) Agency (survey) results and pathlength score (post/pre). Linear regression parameters (y-int, slope) and respective p-values (based on t-test) to indicate significant difference from zero shown with each mode.
Discussion
This study primarily investigated changes in force-driven motor performance with variations in features of augmented visual feedback, namely, complexity and intermittency. We leveraged a novel rehabilitative platform utilizing a computerized interface (i.e., immersive virtual reality) and a position-adjustable arm brace that provides gravity support during isometric strength training at varied arm postures. Our results demonstrated that variations in visual feedback features could generate significant differences in post-training performance. Our results do not reflect true motor learning (Ronsse et al., 2011), which requires demonstration of long-term retention and skill acquisition; however, immediate (short-term) performance effects can be indicative of learning potential (Guadagnoli and Lee, 2004) and promise for neuromotor rehabilitation (Nieuwboer et al., 2009). Direct performance effects were characterized according to a relative change from pre-training to post-training for each participant and the feedback mode used for augmented guidance. For the point-to-point motor task in VR, simpler feedback (i.e., end-effector guide only) appeared to be more effective in improving performance. This more simple guide produced the best pathlength performance overall.
In this study, we presented more complex feedback for this force-driven task (i.e., participant held isometrically) with the inclusion of the links preceding the end-effector of the guide arm. In our previous work investigating visual feedback features for a motion-driven task (i.e., participants’ own motions drive computer display) (Sanford et al., 2020), we evaluated relatively simple and complex forms of feedback. More complex feedback entailed presentation of additional segmental motions to track concurrently. That study suggested that complex feedback representing the intrinsic coupling of all body segments for a high constrained movement (i.e., two-legged squat forming closed-chain with ground) facilitated better tracking of the primary target segment (i.e., thigh). In the current study, the target segment to track is the end-effector, and the motions of the links are constrained to the end-effector through inverse kinematics. While the presentation of these link positions is, in fact, extraneous to the primary target of the end-effector, its inclusion as additional real-time feedback tests whether presenting a kinematic synergy facilitates better motor learning. A significant distinction with this study from our previous work with the squat task is that the participant is held isometrically and cannot dynamically embody (Kilteni et al., 2012) the motion feedback being presented in real time. Thus, complex feedback might only be effectively leveraged towards improved motor performance for rehabilitation paradigms utilizing motion-driven inputs that allow the user to embody the avatar fully. It may be necessary and more challenging for a force-driven task to effectively display kinetic synergies as complex feedback for rehabilitating motor coordination (Berger and d’Avella, 2014). In this study, the additional information presented may have been perceived as distracting (Smith, 2019) or irrelevant to the primary objective (Proteau et al., 1992). Thus, our hypothesis regarding complexity was refuted for the presented motor task.
Our hypothesis regarding intermittency is also refuted as continuous feedback outperformed bandwidth feedback. However, this result is consistent with our previous work investigating intermittency effects with the squat task (Sanford et al., 2021). In both studies, the guidance hypothesis (Park et al., 2000) was not confirmed, suggesting these computerized rehabilitation protocols may not be able to facilitate the development of intrinsic mechanisms within a single session. Thus, follow-up sessions may be necessary to confirm the relevance of the guidance hypothesis to these specific motor paradigms. Furthermore, the guidance hypothesis with intermittent feedback is often predicated on knowledge of results with terminal feedback (Schmidt et al., 1989). Thus, a novel element of the current study is the inclusion of intermittency with concurrent feedback. However, given the proven effectiveness of concurrent feedback to generate immediate improvement in performance (Park et al., 2000), it is crucial to examine ways to leverage concurrent feedback in creating VR rehabilitation protocols that can further accelerate gains in motor function. As in our previous motion-driven squat protocol, we did examine “potential learning” in relative retention in performance in post-training with no feedback after receiving augmented guidance during training. In the squat protocol, this potential learning was greater with bandwidth protocols. In the force-driven task, due to only testing four visual feedback modes instead of six as in the motion task, additional trials were added to create a new pre-training (baseline) phase. Therefore, potential learning was evaluated as the relative difference between the new pre-training (baseline) and the post-training phases (retention). During the VR reaching task, training with concurrent bandwidth feedback induced significantly lower cognitive activity than continuous feedback, regardless of complexity. Amongst simple feedback, determined superior for performance, higher performance was related to lower cognitive stress (alpha band power), lower sense of agency (survey score), and higher physical stress. Relative decreases in alpha band activity can indicate greater focus on external objects during VR interactions (Magosso et al., 2019). This focus shift to external cueing can produce greater performance and retention than a greater internal focus (Wulf, 2013). Furthermore, other studies have shown that lower alpha band activity (Kiefer et al., 2014) and lower cognitive activity identified via fMRI (Weaver, 2015) can suggest greater potential in motor learning. This lower alpha band activity may reflect participant experiences of completed movements that feel more automatic and require less conscious effort (Fitts and Posner, 1967). While long-term retention is only demonstrable with multiple sessions, relatively lower alpha band activity coupled with greater performance gains suggests the promise of simple-bandwidth feedback in learning motor tasks that are more complex, i.e., predicated on muscle-level synergies.
In this study, we observed two sets of user-centered metrics as potential explanatory variables for the performance with various modes of training feedback. First, we observed explicit agency from the survey responses, indicating that participants perceived complex-continuous augmented guidance provided better control of the virtual arm than simple-bandwidth. Complex-continuous theoretically provided the most guidance, i.e., the guide robot arm is displayed fully (end-effector and arm links) and constantly during training. It is plausible that participants assumed the guide arm generated greater control or projected their intended actions on the guide arm versus the actual arm. Our laboratory’s previous findings measured and leveraged agency implicitly for simple computerized reach (Nataraj et al., 2020a; Nataraj et al., 2020b; Nataraj et al., 2022) and grasp (Liu et al., 2021; Nataraj et al., 2021) and demonstrated significant positive correlation with improved performance. Results to that end were inconclusive in this study; however, these preliminary results suggest that a constrained myoelectric task may generate a perceptional inversion whereby participants felt lower agency with feedback conditions that improved performance. Participants are not always aware of what is most beneficial to them for motor learning (Wulf, 2013). Participants may make selections based on comfort and neglect the possibility that challenging scenarios, which may be uncomfortable, will be more advantageous for motor learning.
Physiological measures such as EEG and EDA provide a more objective basis to discern fundamental user-centered responses. As inferred through cognitive loading, increased engagement can produce better performance in a VR environment (Cho et al., 2015). When significant differences were discernible, this study confirmed, as expected, that simpler and intermittent feedback resulted in lower cognitive loading, indicated by reduced EEG power. Since simpler feedback generally produced better performance, it may be inferred that complex feedback, as presented here, may have resulted in overloading that diminished performance (Bannert, 2002). Alternatively, simpler feedback generally produced greater physical arousal, as indicated by higher electrodermal activity (Critchley, 2002). For this study, the simpler feedback may have supported the user to be more physically engaged, without mental distraction, towards improved motor performance.
The major limitations of our study to demonstrate how variations in augmented visual guidance for training affect motor learning include constraints on the task, motor transference, and long-term retention. The task control space was limited to 2D due to challenges in attaining robust multi-dimensional control through the enacted pattern classifiers. More advanced machine learning methods may be enacted for 3D myoelectric control (Liarokapis et al., 2013; Rabin et al., 2020). However, the deployment of such approaches must be balanced against the feasibility considerations of time to train within single sessions (Walsh et al., 2021) and classification accuracy (Gu et al., 2018). Feasibility is crucial for clinical populations with reduced and compromised muscle sets to identify myoelectric commands (Huang et al., 2016; Walsh et al., 2021). Ultimately, improved motor skill acquisition (Soderstrom and Bjork, 2015; Utley, 2018) must be demonstrated by testing functional abilities in generalizable contexts that differ from training. Functional gains with isometric testing must be exhibited through improved abilities to perform dynamic tasks that better represent activities of daily living (Akima et al., 1999; Garcia-Hernandez et al., 2019). Additional modifications could be pursued to facilitate better motor control, even within the training paradigm (Bank et al., 2017). We did employ a measure of strategy to support motor control objectives by allowing users to self-select the order or target pursuits during testing blocks. However, more complex tasks (e.g., 3D control, additional tasks beyond point-to-point contact) are more versatile for synergistic control. Synergistic control involves manipulating the end-effector through forward dynamics (Ferris and Schlink, 2017), whereby the user enacts control upon a robot arm’s elbow and shoulder joints.
This study’s findings with neurotypical participants demonstrate the feasibility of the platform utilizing myoelectric control of virtual avatars and show how performance and physiological responses are sensitive to augmented visual guidance features during motor training. The implications of this work include the development of VR applications that systematically vary feedback features and better ensure desired performance and physiological responses that support improved functional outcomes. Future research directions should further consider how augmented guidance can be altered to optimize outcomes for clinical populations. A key question is whether persons with losses in either motor or cognitive capacity can similarly absorb and leverage augmented feedback in this platform for improved motor performance. For rehabilitation and authentic motor learning, repeated measures (i.e., follow-up sessions) with the same group of affected participants would be needed for validation. In any case, this line of research promotes approaches for advanced rehabilitation platforms that are more user-personalized and holistic (i.e., consideration of performance, perceptional, and physiological responses).
Conclusion
In optimizing VR training for a force-driven motor task, the complexity and intermittency of augmented visual guidance can significantly influence the resultant motor performance. When training upper-extremity function, additional visual feedback about the forearm and upper arm may be unnecessary when the primary objective is end-effector accuracy. For a virtual reaching task, training with simpler feedback (i.e., about end-effector only) resulted in significantly greater motor performance (e.g., minimal pathlengths, shorter completion times) and higher arousal (electrodermal activity). Furthermore, training with feedback presented more intermittently (i.e., bandwidth) resulted in improved muscle-level control in conjunction with lower cognitive (alpha band) activity. These post-training results with simple-bandwidth feedback indicated that participants were more positively allocating resources to physical engagement and performance. Future studies should investigate longitudinal comparisons of VR-based therapies that systematically leverage augmented visual guidance to conventional treatments and non-optimized VR protocols to determine if these performance advantages exist for similar therapeutic dosages. Furthermore, advanced feedback control systems to adapt VR rehabilitation systems for greater personalization for individual users may consider varying training features according to online measures of physiological variables (e.g., EEG, EDA).
Data availability statement
The raw data supporting the conclusion of this article will be made available by the authors, without undue reservation.
Ethics statement
The studies involving human participants were reviewed and approved by the Institutional Review Board (IRB) of Stevens Institute of Technology. The patients/participants provided their written informed consent to participate in this study.
Author contributions
SS designed the experimental protocol, collected the data, and wrote the manuscript. BC helped develop the VR environments. ML and SD helped with data collection and article revisions. RN was the principal investigator supervising the project.
Funding
Charles V. Schaefer, Jr. School of Engineering and Science, Stevens Institute of Technology provided funds to support the fundamental development of this research. VA SPiRE Grant RX003582 supported the translation of the described platform for use with persons having spinal cord injury. Stevens Institute of Technology Provost Office provided the Excellence Doctoral Fellowship support for SS’s final year of dissertation study culminating in the work presented in this study.
Conflict of interest
The authors declare that the research was conducted in the absence of any commercial or financial relationships that could be construed as a potential conflict of interest.
Publisher’s note
All claims expressed in this article are solely those of the authors and do not necessarily represent those of their affiliated organizations, or those of the publisher, the editors and the reviewers. Any product that may be evaluated in this article, or claim that may be made by its manufacturer, is not guaranteed or endorsed by the publisher.
References
Ajiboye, A. B., and Weir, R. F. H. (2005). A heuristic fuzzy logic approach to EMG pattern recognition for multifunctional prosthesis control. IEEE Trans. Neural Syst. Rehabil. Eng. 13 (3), 280–291. doi:10.1109/TNSRE.2005.847357
Akima, H., Takahashi, H., Kuno, S. Y., Masuda, K., Masuda, T., Shimojo, H., et al. (1999). Early phase adaptations of muscle use and strength to isokinetic training. Med. Sci. Sports Exerc. 31 (4), 588–594. doi:10.1097/00005768-199904000-00016
Alavi, N., Herrnstadt, G., Randhawa, B. K., Boyd, L. A., and Menon, C. (2015). Bimanual elbow exoskeleton: Force based protocol and rehabilitation quantification.” in 2015 37th Annual International Conference of the IEEE Engineering in Medicine and Biology Society. EMBC, 4643
Antfolk, C. (2010). Using EMG for real-time prediction of joint angles to control a prosthetic hand equipped with a sensory feedback system. J. Med. Biol. Eng. 30 (6), 399. doi:10.5405/jmbe.767
Aoyagi, K., et al. (2019). Mprovement of sense of agency during upper-limb movement for motor rehabilitation using virtual reality.” in 41st Annual International Conference of the IEEE Engineering in Medicine and Biology Society. Berlin, Germany: EMBC, 118–121. doi:10.1109/EMBC.2019.8856796
Ávila-Sansores, S., Orihuela-Espina, F., and Enrique-Sucar, L. (2013). “Patient tailored virtual rehabilitation,” in Converging clinical and engineering research on neurorehabilitation (Berlin, Heidelberg, 879–883. doi:10.1007/978-3-642-34546-3_143
Bank, P. J. M., Dobbe, L. R. M., Meskers, C. G. M., de Groot, J. H., and de Vlugt, E. (2017). Manipulation of visual information affects control strategy during a visuomotor tracking task. Behav. Brain Res. 329, 205–214. doi:10.1016/j.bbr.2017.04.056
Bannert, M. (2002). Managing cognitive load—Recent trends in cognitive load theory. Learn. Instr. 12 (1), 139–146. doi:10.1016/S0959-4752(01)00021-4
Berger, D. J., and d’Avella, A. (2014). Effective force control by muscle synergies. Front. Comput. Neurosci. 8, 46. doi:10.3389/fncom.2014.00046
Berger, D. J., and d’Avella, A. (2017). “Towards a myoelectrically controlled virtual reality interface for synergy-based stroke rehabilitation,” in Converging Clinical and Engineering Research on Neurorehabilitation II. Biosystems & Biorobotics. Editors J. Ibáñez, J. González-Vargas, J. Azorín, M. Akay, and J. Pons (Cham: Springer) 15. doi:10.1007/978-3-319-46669-9_156
Blana, D., Kyriacou, T., Lambrecht, J. M., and Chadwick, E. K. (2016). Feasibility of using combined EMG and kinematic signals for prosthesis control: A simulation study using a virtual reality environment. J. Electromyogr. Kinesiol. 29, 21–27. doi:10.1016/j.jelekin.2015.06.010
Carmeli, E., Vatine, J.-J., Peleg, S., Bartur, G., and Elbo, E., “Upper limb rehabilitation using augmented feedback: Impairment focused augmented feedback with handtutor,” in 2009 Virtual Rehabilitation International Conference, 2009, pp. 220.
Celik, O., O’Malley, M. K., Boake, C., Levin, H. S., Yozbatiran, N., and Reistetter, T. A. (2010). Normalized movement quality measures for therapeutic robots strongly correlate with clinical motor impairment measures. IEEE Trans. Neural Syst. Rehabil. Eng. 18 (4), 433–444. doi:10.1109/tnsre.2010.2047600
Cho, K. H., Kim, M. K., Lee, H.-J., and Lee, W. H. (2015). Virtual reality training with cognitive load improves walking function in chronic stroke patients. Tohoku J. Exp. Med. 236 (4), 273–280. doi:10.1620/tjem.236.273
Cirstea, M. C., and Levin, M. F. (2007). Improvement of arm movement patterns and endpoint control depends on type of feedback during practice in stroke survivors. Neurorehabil. Neural Repair 21 (5), 398–411. doi:10.1177/1545968306298414
Critchley, H. D. (2002). Review: Electrodermal responses: What happens in the brain. Neuroscientist 8 (2), 132–142. doi:10.1177/107385840200800209
Dipietro, L., Krebs, H. I., Fasoli, S. E., Volpe, B. T., and Hogan, N. (2009). Submovement changes characterize generalization of motor recovery after stroke. cortex 45 (3), 318–324. doi:10.1016/j.cortex.2008.02.008
Ferris, D. P., and Schlink, B. R. (2017). Robotic devices to enhance human movement performance. Kinesiol. Rev. (Champaign). 6 (1), 70–77. doi:10.1123/kr.2016-0040
Folland, J. P., Hawker, K., Leach, B., Little, T., and Jones, D. A. (2005). Strength training: Isometric training at a range of joint angles versus dynamic training. J. Sports Sci. 23 (8), 817–824. doi:10.1080/02640410400021783
Fox, I. K., Davidge, K. M., Novak, C. B., Hoben, G., Kahn, L. C., Juknis, N., et al. (2015). Nerve transfers to restore upper extremity function in cervical spinal cord injury: Update and preliminary outcomes. Plastic Reconstr. Surg. 136 (4), 780–792. doi:10.1097/prs.0000000000001641
Garcia-Hernandez, N., Garza-Martinez, K., Parra-Vega, V., Alvarez-Sanchez, A., and Conchas-Arteaga, L. (2019). Development of an EMG-based exergaming system for isometric muscle training and its effectiveness to enhance motivation, performance and muscle strength. Int. J. Hum. Comput. Stud. 124, 44–55. doi:10.1016/j.ijhcs.2018.11.010
Ghafouri, M., and Feldman, A. G. (2001). The timing of control signals underlying fast point-to-point arm movements. Exp. Brain Res. 137 (3), 411–423. doi:10.1007/s002210000643
Gordon, K. E., and Ferris, D. P. (2004). Proportional myoelectric control of a virtual object to investigate human efferent control. Exp. Brain Res. 159 (4), 478–486. doi:10.1007/s00221-004-1970-6
Gu, Y., Yang, D., Huang, Q., Yang, W., and Liu, H. (2018). Robust EMG pattern recognition in the presence of confounding factors: Features, classifiers and adaptive learning. Expert Syst. Appl. 96, 208–217. doi:10.1016/j.eswa.2017.11.049
Guadagnoli, M. A., and Lee, T. D. (2004). Challenge point: A framework for conceptualizing the effects of various practice conditions in motor learning. J. Mot. Behav. 36 (2), 212–224. doi:10.3200/jmbr.36.2.212-224
Hakim, R. M., Tunis, B. G., and Ross, M. D. (2017). Rehabilitation robotics for the upper extremity: Review with new directions for orthopaedic disorders. Disabil. Rehabilitation Assistive Technol. 12 (8), 765–771. doi:10.1080/17483107.2016.1269211
Howard, M. C. (2017). A meta-analysis and systematic literature review of virtual reality rehabilitation programs. Comput. Hum. Behav. 70, 317–327. doi:10.1016/j.chb.2017.01.013
Huang, S., Wensman, J. P., and Ferris, D. P. (2016). Locomotor adaptation by transtibial amputees walking with an experimental powered prosthesis under continuous myoelectric control. IEEE Trans. Neural Syst. Rehabil. Eng. 24 (5), 573–581. doi:10.1109/TNSRE.2015.2441061
Johnson, W., and Griswold, D. (2017). Traumatic brain injury: A global challenge. Lancet Neurol. 16 (12), 949–950. doi:10.1016/s1474-4422(17)30362-9
Kearney, E., Shellikeri, S., Martino, R., and Yunusova, Y. (2019). Augmented visual feedback-aided interventions for motor rehabilitation in Parkinson’s disease: A systematic review. Disabil. Rehabil. 41 (9), 995–1011. doi:10.1080/09638288.2017.1419292
Keenan, M. A. (1988). Management of the spastic upper extremity in the neurologically impaired adult. Clin. Orthop. Relat. Res. 233, 116–125. doi:10.1097/00003086-198808000-00014no
Kern, F., Winter, C., Gall, D., Käthner, I., Pauli, P., and Latoschik, M. E. (2019). “Immersive virtual reality and gamification within procedurally generated environments to increase motivation during gait rehabilitation,” in 2019 IEEE Conference on Virtual Reality and 3D User Interfaces (VR), 500–509. doi:10.1109/VR.2019.8797828
Kiefer, A. W., Gualberto Cremades, J., and Myer, G. D. (2014). Train the brain: Novel electroencephalography data indicate links between motor learning and brain adaptations. J. Nov. Physiother. 4 (2), 198. doi:10.4172/2165-7025.1000198
Kilteni, K., Groten, R., and Slater, M. (2012). The sense of embodiment in virtual reality. Presence. (Camb).Nov 21 (4), 373–387. doi:10.1162/PRES_a_00124
Kucuk, S., and Bingul, Z. (2006). Robot kinematics: Forward and inverse kinematics. INTECH Open Access Publisher.
Kumar, N., and Kumar, J. (2016). Measurement of cognitive load in HCI systems using EEG power spectrum: An experimental study. Procedia Comput. Sci. 84, 70–78. doi:10.1016/j.procs.2016.04.068
Lee, S., Kim, Y., and Lee, B.-H. (2016). Effect of virtual reality-based bilateral upper extremity training on upper extremity function after stroke: A randomized controlled clinical trial: Bilateral upper extremity training in post stroke. Occup. Ther. Int. 23 (4), 357–368. doi:10.1002/oti.1437
Levin, M. F., Weiss, P. L., and Keshner, E. A. (2015). Emergence of virtual reality as a tool for upper limb rehabilitation: Incorporation of motor control and motor learning principles. Phys. Ther.Mar 95 (3), 415–425. doi:10.2522/ptj.20130579
Liarokapis, M. V., Artemiadis, P. K., Kyriakopoulos, K. J., and Manolakos, E. S. (2013). A learning scheme for reach to grasp movements: On EMG-based interfaces using task specific motion decoding models. IEEE J. Biomed. Health Inf. 17 (5), 915–921. doi:10.1109/JBHI.2013.2259594
Lim, D. Y., Hwang, D. M., Cho, K. H., Moon, C. W., and Ahn, S. Y. (2020). A fully immersive virtual reality method for upper limb rehabilitation in spinal cord injury. Ann. Rehabil. Med. 44 (4), 311–319. doi:10.5535/arm.19181
Liu, M., Wilder, S., Sanford, S., Saleh, S., Harel, N. Y., and Nataraj, R. (2021). Training with agency-inspired feedback from an instrumented glove to improve functional grasp performance. Sensors 21 (4), 1173. doi:10.3390/s21041173
Magosso, E., De Crescenzio, F., Ricci, G., Piastra, S., and Ursino, M. (20192019). EEG alpha power is modulated by attentional changes during cognitive tasks and virtual reality immersion. Comput. Intell. Neurosci. 2019, 1–18. doi:10.1155/2019/7051079
McIntyre, J., Mussa-Ivaldi, F. A., and Bizzi, E. (1996). The control of stable postures in the multijoint arm. Exp. Brain Res. 110 (2), 248–264. doi:10.1007/bf00228556
Minkel, J. L. (2000). Seating and mobility considerations for people with spinal cord injury. Phys. Ther. 80 (7), 701–709. doi:10.1093/ptj/80.7.701
Molier, B. I., Van Asseldonk, E. H., Hermens, H. J., and Jannink, M. J. (2010). Nature, timing, frequency and type of augmented feedback; does it influence motor relearning of the hemiparetic arm after stroke? A systematic review. Disabil. Rehabil. 32 (22), 1799–1809. doi:10.3109/09638281003734359
Moore, J. W., and Obhi, S. S. (2012). Intentional binding and the sense of agency: A review. Conscious. Cogn. 21 (1), 546–561. doi:10.1016/j.concog.2011.12.002
Morone, G., Ghanbari Ghooshchy, S., Palomba, A., Baricich, A., Santamato, A., Ciritella, C., et al. (2021). Differentiation among bio-and augmented-feedback in technologically assisted rehabilitation. Expert Rev. Med. Devices 18 (6), 513–522. doi:10.1080/17434440.2021.1927704
Nataraj, R., Hollinger, D., Liu, M., and Shah, A. (2020). Disproportionate positive feedback facilitates sense of agency and performance for a reaching movement task with a virtual hand. PLoS One 15 (5), e0233175. doi:10.1371/journal.pone.0233175
Nataraj, R., Sanford, S., Liu, M., and Harel, N. Y. (2022). Hand dominance in the performance and perceptions of virtual reach control. Acta Psychol. (Amst). 223, 1034942022. doi:10.1016/j.actpsy.2022.103494
Nataraj, R., Sanford, S., Shah, A., and Liu, M. (2020). Agency and performance of reach-to-grasp with modified control of a virtual hand: Implications for rehabilitation. Front. Hum. Neurosci. 14, 126. doi:10.3389/fnhum.2020.00126
Nataraj, R., Sanford, S., and Taha, M. O. (2021) Stable chitosan-based nanoparticles using polyphosphoric acid or hexametaphosphate for tandem ionotropic/covalent crosslinking and subsequent investigation as novel vehicles for drug delivery. Front. Bioeng. Biotechnol. 8, 1544. doi:10.3389/fbioe.2020.00004
Nesbitt, K. (2003). Designing multi-sensory displays for abstract data. Available at: https://ses.library.usyd.edu.au/handle/2123/4135.
Nieuwboer, A., Rochester, L., Müncks, L., and Swinnen, S. P. (2009). Motor learning in Parkinson’s disease: Limitations and potential for rehabilitation. Park. Relat. Disord. 15, S53–S58. doi:10.1016/s1353-8020(09)70781-3
Noorkõiv, M., Nosaka, K., and Blazevich, A. J. (2015). Effects of isometric quadriceps strength training at different muscle lengths on dynamic torque production. J. Sports Sci. 33 (18), 1952–1961. doi:10.1080/02640414.2015.1020843
Oranchuk, D. J., Storey, A. G., Nelson, A. R., and Cronin, J. B. (2019). Isometric training and long-term adaptations: Effects of muscle length, intensity, and intent: A systematic review. Scand. J. Med. Sci. Sports 29 (4), 484–503. doi:10.1111/sms.13375
Parajuli, N., Sreenivasan, N., Bifulco, P., Cesarelli, M., Savino, S., Niola, V., et al. (2019). Real-time EMG based pattern recognition control for hand prostheses: A review on existing methods, challenges and future implementation. Sensors 19 (20), 4596. doi:10.3390/s19204596
Park, J. H., Shea, C. H., and Wright, D. L. (2000). Reduced-frequency concurrent and terminal feedback: A test of the guidance hypothesis. J. Mot. Behav. 32 (3), 287–296. doi:10.1080/00222890009601379
Perry, B. N., Armiger, R. S., Yu, K. E., Alattar, A. A., Moran, C. W., Wolde, M., et al. (2018). Virtual integration environment as an advanced prosthetic limb training platform. Front. Neurol. 9, 785. doi:10.3389/fneur.2018.00785
Prasad, S., Aikat, R., Labani, S., and Khanna, N. (2018). Efficacy of virtual reality in upper limb rehabilitation in patients with spinal cord injury: A pilot randomized controlled trial. Asian Spine J. 12 (5), 927–934. doi:10.31616/asj.2018.12.5.927
Proteau, L. (1992). “Chapter 4 on the specificity of learning and the role of visual information for movement control,” in Advances in Psychology. Editors L. Proteau, and D. Elliott (North-Holland), 85, 67–103. doi:10.1016/S0166-4115(08)62011-7
Rabin, N., Kahlon, M., Malayev, S., and Ratnovsky, A. (2020). Classification of human hand movements based on EMG signals using nonlinear dimensionality reduction and data fusion techniques. Expert Syst. Appl. 149, 1132812020. doi:10.1016/j.eswa.2020.113281
Reinold, M. M., Macrina, L. C., Wilk, K. E., Fleisig, G. S., Dun, S., Barrentine, S. W., et al. (2007). Electromyographic analysis of the supraspinatus and deltoid muscles during 3 common rehabilitation exercises. J. Athl. Train. 42 (4), 464
Ronsse, R., Puttemans, V., Coxon, J. P., Goble, D. J., Wagemans, J., Wenderoth, N., et al. (2011). Motor learning with augmented feedback: Modality-dependent behavioral and neural consequences. Cereb. Cortex 21 (6), 1283–1294. doi:10.1093/cercor/bhq209
Sadowski, J., Mastalerz, A., and Niznikowski, T. (2013). Benefits of bandwidth feedback in learning a complex gymnastic skill. J. Hum. Kinet. 37 (1), 183–193. doi:10.2478/hukin-2013-0039
Salmoni, A. W., Schmidt, R. A., and Walter, C. B. (1984). Knowledge of results and motor learning: A review and critical reappraisal. Psychol. Bull. 95 (3), 355–386. doi:10.1037/0033-2909.95.3.355
Sanford, S., Liu, M., and Nataraj, R. (2021). Concurrent continuous versus bandwidth visual feedback with varying body representation for the 2-legged squat exercise. J. Sport Rehabil. 30, 794–803. doi:10.1123/jsr.2020-0234
Sanford, S., Liu, M., Selvaggi, T., and Nataraj, R. (2020). Effects of visual feedback complexity on the performance of a movement task for rehabilitation. J. Mot. Behav. 53, 243–257. doi:10.1080/00222895.2020.1770670
Schiffman, J. M., Luchies, C. W., Piscitelle, L., Hasselquist, L., and Gregorczyk, K. N. (2006). Discrete bandwidth visual feedback increases structure of output as compared to continuous visual feedback in isometric force control tasks. Clin. Biomech. (Bristol, Avon. 21 (10), 1042–1050. doi:10.1016/j.clinbiomech.2006.05.009
Schmidt, R. A., Young, D. E., Swinnen, S., and Shapiro, D. C. (1989). Summary knowledge of results for skill acquisition: Support for the guidance hypothesis. J. Exp. Psychol. Learn. Mem. Cognition 15 (2), 352–359. doi:10.1037/0278-7393.15.2.352
Selkowitz, D. M., Chaney, C., Stuckey, S. J., and Vlad, G. (2007). The effects of scapular taping on the surface electromyographic signal amplitude of shoulder girdle muscles during upper extremity elevation in individuals with suspected shoulder impingement syndrome. J. Orthop. Sports Phys. Ther. 37 (11), 694–702. doi:10.2519/jospt.2007.2467
Sewall, L. P., Reeve, T. G., and Day, R. A. (1988). Effect of concurrent visual feedback on acquisition of a weightlifting skill. Percept. Mot. Ski. 67 (3), 715–718. doi:10.2466/pms.1988.67.3.715
Sigrist, R., Rauter, G., Riener, R., and Wolf, P. (2013). Augmented visual, auditory, haptic, and multimodal feedback in motor learning: A review. Psychon. Bull. Rev. 20 (1), 21–53. doi:10.3758/s13423-012-0333-8
Sigrist, R., Rauter, G., Riener, R., and Wolf, P. (2013). Terminal feedback outperforms concurrent visual, auditory, and haptic feedback in learning a complex rowing-type task. J. Mot. Behav. 45 (6), 455–472. doi:10.1080/00222895.2013.826169
Smith, S. A. (2019). Virtual reality in episodic memory research: A review. Psychon. Bull. Rev. 26 (4), 1213–1237. doi:10.3758/s13423-019-01605-w
Soderstrom, N. C., and Bjork, R. A. (2015). Learning versus performance: An integrative review. Perspect. Psychol. Sci. 10 (2), 176–199. doi:10.1177/1745691615569000
Sveistrup, H. (2004). Motor rehabilitation using virtual reality. J. Neuroeng. Rehabil. 1 (1), 10–18. doi:10.1186/1743-0003-1-10
Tiboni, M., Borboni, A., Faglia, R., and Pellegrini, N. (2018). Robotics rehabilitation of the elbow based on surface electromyography signals. Adv. Mech. Eng. 10 (2), 168781401875459. doi:10.1177/1687814018754590
Toledo-Pérez, D. C., Rodríguez-Reséndiz, J., Gómez-Loenzo, R. A., and Jauregui-Correa, J. C. (2019). Support vector machine-based EMG signal classification techniques: A review. Appl. Sci. (Basel). 9 (20), 4402. doi:10.3390/app9204402
Van Dijk, H., Jannink, M. J., and Hermens, H. J. (2005). Effect of augmented feedback on motor function of the affected upper extremity in rehabilitation patients: A systematic review of randomized controlled trials. J. Rehabil. Med. 37 (4), 202–211. doi:10.1080/16501970510030165
Walsh, K. A., Sanford, S. P., Collins, B. D., Harel, N. Y., and Nataraj, R. (2021). Performance potential of classical machine learning and deep learning classifiers for isometric upper-body myoelectric control of direction in virtual reality with reduced muscle inputs. Biomed. Signal Process. Control 66, 102487. doi:10.1016/j.bspc.2021.102487
Wannstedt, G. T., and Herman, R. M. (1978). Use of augmented sensory feedback to achieve symmetrical standing. Phys. Ther. 58 (5), 553–559. doi:10.1093/ptj/58.5.553
Weaver, J. (2015). Motor learning unfolds over different timescales in distinct neural systems. PLoS Biol. 13 (12), e1002313, Dec. doi:10.1371/journal.pbio.1002313
Won, J., and Hogan, N. (1995). Stability properties of human reaching movements. Exp. Brain Res. 107 (1), 125–136. doi:10.1007/bf00228024
Wulf, G. (2013). Attentional focus and motor learning: A review of 15 years. Int. Rev. Sport Exerc. Psychol. 6 (1), 77–104. doi:10.1080/1750984X.2012.723728
Wulf, G., and Shea, C. H. (2002). Principles derived from the study of simple skills do not generalize to complex skill learning. Psychon. Bull. Rev. 9 (2), 185–211. doi:10.3758/bf03196276
Wyndaele, M., and Wyndaele, J.-J. (2006). Incidence, prevalence and epidemiology of spinal cord injury: What learns a worldwide literature survey? Spinal Cord. 44 (9), 523–529. doi:10.1038/sj.sc.3101893
Young, D. E., and Schmidt, R. A. (1992). Augmented kinematic feedback for motor learning. J. Mot. Behav. 24 (3), 261–273. doi:10.1080/00222895.1992.9941621
Yue, S., Henrich, D., Xu, W. L., and Tso, S. K. (2002). Point-to-point trajectory planning of flexible redundant robot manipulators using genetic algorithms. Robotica 20 (3), 269–280. doi:10.1017/s0263574701003861
Zhou, S.-H., Tan, Y., Oetomo, D., Freeman, C., Burdet, E., and Mareels, I. (2013). Point-to-point learning in human motor systems. American Control Conference, 5923
Keywords: virtual reality (VR), visual feedback, electromyography (EMG), rehabilitation, motor performance
Citation: Sanford S, Collins B, Liu M, Dewil S and Nataraj R (2022) Investigating features in augmented visual feedback for virtual reality rehabilitation of upper-extremity function through isometric muscle control. Front. Virtual Real. 3:943693. doi: 10.3389/frvir.2022.943693
Received: 14 May 2022; Accepted: 13 October 2022;
Published: 03 November 2022.
Edited by:
Daniel Thalmann, Swiss Federal Institute of Technology Lausanne, SwitzerlandReviewed by:
Marina Cidota, University of Bucharest, RomaniaMarkus Santoso, University of Florida, United States
Copyright © 2022 Sanford, Collins, Liu, Dewil and Nataraj. This is an open-access article distributed under the terms of the Creative Commons Attribution License (CC BY). The use, distribution or reproduction in other forums is permitted, provided the original author(s) and the copyright owner(s) are credited and that the original publication in this journal is cited, in accordance with accepted academic practice. No use, distribution or reproduction is permitted which does not comply with these terms.
*Correspondence: Raviraj Nataraj, cm5hdGFyYWpAc3RldmVucy5lZHU=