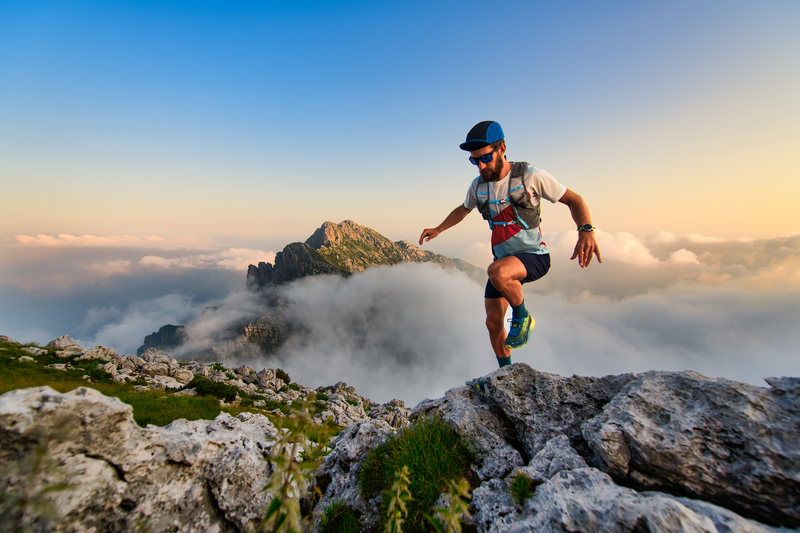
95% of researchers rate our articles as excellent or good
Learn more about the work of our research integrity team to safeguard the quality of each article we publish.
Find out more
ORIGINAL RESEARCH article
Front. Virtual Real. , 19 July 2022
Sec. Virtual Reality and Human Behaviour
Volume 3 - 2022 | https://doi.org/10.3389/frvir.2022.895487
Background: Numerous studies have investigated emotion in virtual reality (VR) experiences using self-reported data in order to understand valence and arousal dimensions of emotion. Objective physiological data concerning valence and arousal has been less explored. Electroencephalography (EEG) can be used to examine correlates of emotional responses such as valence and arousal in virtual reality environments. Used across varying fields of research, images are able to elicit a range of affective responses from viewers. In this study, we display image sequences with annotated valence and arousal values on a screen within a virtual reality theater environment. Understanding how brain activity responses are related to affective stimuli with known valence and arousal ratings may contribute to a better understanding of affective processing in virtual reality.
Methods: We investigated frontal alpha asymmetry (FAA) responses to image sequences previously annotated with valence and arousal ratings. Twenty-four participants viewed image sequences in VR with known valence and arousal values while their brain activity was recorded. Participants wore the Oculus Quest VR headset and viewed image sequences while immersed in a virtual reality theater environment.
Results: Image sequences with higher valence ratings elicited greater FAA scores than image sequences with lower valence ratings (F [1, 23] = 4.631, p = 0.042), while image sequences with higher arousal scores elicited lower FAA scores than image sequences with low arousal (F [1, 23] = 7.143, p = 0.014). The effect of valence on alpha power did not reach statistical significance (F [1, 23] = 4.170, p = 0.053). We determined that only the high valence, low arousal image sequence elicited FAA which was significantly higher than FAA recorded during baseline (t [23] = −3.166, p = 0.002), suggesting that this image sequence was the most salient for participants.
Conclusion: Image sequences with higher valence, and lower arousal may lead to greater FAA responses in VR experiences. While findings suggest that FAA data may be useful in understanding associations between valence and arousal self-reported data and brain activity responses elicited from affective experiences in VR environments, additional research concerning individual differences in affective processing may be informative for the development of affective VR scenarios.
Physiological input can be brought into games and virtual reality (VR) experiences to create a more personalized, dynamic experience. The increasing popularity of physiological data monitoring in games and the simultaneous advancement in consumer-grade physiological tracking technologies has led to a great increase in affective gaming research, effectively enhancing the player experience (Robinson et al., 2020). Research in affective experiences, however, remains in its infancy, as there exist few standard protocols for evaluation (Robinson et al., 2020).
Numerous studies have investigated affective responses to better understand user emotions elicited during various VR experiences. Affective responses in VR have been investigated through self-reported data (Felnhofer et al., 2015; Lin, 2017; Voigt-Antons et al., 2021) as well as physiological data (Kerous et al., 2020; Kisker et al., 2021; Schöne et al., 2021). Self-reported data often examines affect from the perspective of a dimensional model of affect (Poria et al., 2017).
Russell’s circumplex model of affect (Russell, 1980) includes two dimensions commonly explored in affective research: valence (pleasantness; unpleasant to pleasant) and arousal (intensity; low to high). Valence represents the level of pleasantness of an affective stimulus, while arousal represents the level of intensity or excitement elicited from an affective stimulus. A visualization of this model can be seen in Figure 1. For example, Bored is shown in the lower left quadrant of Figure 1, as it is an unpleasant, mild intensity affective state, while Astonished is shown in the upper right quadrant of Figure 1, as it is a pleasant, high intensity affective state. While VR research investigating valence and arousal dimensions of affect has increased in recent years (Marín-Morales et al., 2018), arousal is investigated more frequently than valence in head-mounted display (HMD) based VR experiences (Marín-Morales et al., 2020).
FIGURE 1. The valence-arousal circumplex model of affect as defined by Russell (Russell, 1980). The horizontal axis represents valence, while the vertical axis represents arousal.
While questionnaire data can increase knowledge concerning user emotional states, combining self-reported with objective, physiological data is recommended to increase interpretability of results, which may also increase efficacy of VR experiences in serious applications (Checa and Bustillo, 2020). Therefore, it is our belief that additional research is needed to elucidate findings related to affect by increasing efforts to understand associations between self-reported and physiological data.
Indicative of neural processes, electroencephalography (EEG) is often used to investigate correlates of emotional responses in VR environments, such as valence and arousal (Marín-Morales et al., 2020). Several VR studies have examined emotional correlates through the measurement of frontal alpha asymmetry (FAA) (Rodrigues et al., 2018; Kisker et al., 2021; Schöne et al., 2021); a score measuring the difference between right and left prefrontal cortical activity (Harmon-Jones and Gable, 2018).
Perhaps the most investigated model, the valence model of affect (Davidson et al., 1990) proposes that relatively greater left prefrontal cortical activity is associated with both positive emotions and approach, while relatively greater right prefrontal cortical activity is associated with negative emotions and withdrawal. However, Harmon-Jones and Gable (2018) suggest an FAA model based on motivational direction alone, as the negatively valenced emotion of anger is associated with greater relative left frontal cortical activity, while Lacey et al. (2020) propose that FAA may instead indicate effortful control of emotions rather than emotions themselves. While multiple models of FAA exist, none have proven to be universally valid (Kisker et al., 2021). Despite the lack of an agreed upon model of FAA, for the purposes of this study, we consider the valence model of affect (Davidson et al., 1990) in order to interpret our results.
Because images can elicit a dynamic range of affective responses from viewers, numerous studies across varying fields of research have used images to examine emotional responses (Quigley et al., 2014; Kurdi et al., 2017). In the present study, we aimed to investigate FAA responses to image sequences with known self-reported valence and arousal values. Therefore, we used image sequences comprised of images from the Open Affective Standardized Image Set (OASIS) (Kurdi et al., 2017). These image sequences have been evaluated in a previous study (Mousas et al., 2021) in which users rated the level of arousal and valence of each image sequence. The image sequences are described in more detail in Section 2.5.
To examine FAA responses to the image sequences in virtual reality, we presented the images on a screen within a virtual movie theater environment, as described in Section 2.2. In this way, we could investigate FAA responses to the image sequences with known self-reported values within a virtual environment. Our goal was to investigate the relationship between FAA responses image sequences with annotations of valence and arousal ratings, in order to understand how FAA responses related to the self-reported data from the image sequences.
Similar work by Schöne et al. (2021) also investigated FAA within VR using affective stimuli that the authors developed. Schöne et al. (2021) classified their affective stimuli as positive, neutral or negative based on its appetitive/aversive nature. Appetitive stimuli is that which is positively valenced and which may result in approach behavior, while aversive stimuli is negatively valenced and may result in avoidance behavior (Hayes et al., 2014). While members of their research team agreed upon the classifications of their affective stimuli, our study differs in that we examine affective image sequences previously annotated with user-rated values of valence and arousal. In our study, participants wore an EEG headset while viewing these image sequences in a VR theater environment.
While we conducted additional exploratory analyses based on our findings, we list our primary research questions and hypotheses here:
• RQ1: Will FAA differ between image sequences with different valence and arousal levels?
– We hypothesize that FAA will be greater during positively valenced image sequences, as the valence model of affect would predict.
• RQ2: Will left prefrontal cortical activity be greater than right activity during high valence image sequences?
– We hypothesize that during high valence image sequences, left prefrontal cortical activity will be greater than right activity.
• RQ3: Will FAA during baseline differ from FAA during the image sequences?
– We hypothesize that FAA during baseline will significantly differ from FAA during the high valence and high arousal image sequence, as this image sequence is the most positive and intense.
Our contribution seeks to build a greater knowledge base concerning objective affective data in virtual environments.
Students at Purdue University were invited to participate in this study via email announcements. The study was approved by the Purdue University institutional review board (IRB), and participants provided written consent before participation. We collected data from 29 participants in total. Five participants were removed from the analysis due to poor data quality from either an inadequate EEG headset fit or head movement during the experiment, leaving 24 participants (14 male, 10 female; age: M = 24.38, SD = 3.50; age range: 19 − 33) for our statistical analysis. All participants were right-handed with the exception of two (one: left-handed, one: ambidextrous). We examined FAA values recorded during four image sequence events (see Section 3). An a priori power analysis was conducted using G*Power3 (Faul et al., 2007) to test the difference between four image sequence measurements (two levels of valence and two levels of arousal) using a repeated measures Analysis of Variance (ANOVA), a medium effect size 0.25 (partial η2 = 0.06) (Cohen, 1988), and an alpha of 0.05. This analysis showed that 23 participants were necessary to achieve a power of 0.80. Our group sample size is greater than that of Schöne et al. (2016), who displayed images to participants while investigating FAA through EEG, and similar to Kisker et al. (2021), who used EEG to investigate FAA during an immersive VR experience. Participants received a 10 United States dollar Amazon gift card as compensation.
We presented our virtual environment (VE) to participants using the Oculus Quest VR headset. The Oculus Quest VR headset has a visual resolution of 1,440 × 1,600 per eye, a refresh rate of 72 Hz, and user adjustable interpupillary distance (IPD). While slight viewing distortion may exist in HMDs, all participants used the IPD settings on the headset and determined a comfortable viewing setting. No participant stated that the images were unclear.
We used a movie theater environment downloaded from the Unity Asset store and placed image sequences on the screen, mimicking a movie playing at a theater, as seen in Figure 2. Our decision to place participants in a virtual theater environment was based on work by He et al. (2018), who stated that making participants feel like they were in a theater could create a strong immersive experience for them, in turn inducing a strong emotional effect (Ding et al., 2018). Additionally, Kim et al. (2018) showed that such an immersive experience could more significantly impact emotional responses of participants compared to a non-immersive experience.
Although a direct, one-to-one relationship between color and emotion does not exist, the color red is thought to contain the most energy (Lindström et al., 2006). Therefore, it is possible the red seats and carpet of the virtual environment provided an energizing atmosphere for participants, which may have influenced feelings towards image sequences. Because all participants experienced the same virtual theater environment, we assume any effects from the color of the environment are comparable between participants. Participants were positioned a few rows back, in the middle of the theater environment. Images were positioned in the virtual environment with no degree of tilt; the z-axis rotation parameter was set at zero. Our image sequence viewing application was developed using the Unity game engine.
To collect brain activity data from participants, we used the consumer-grade Emotiv Epoc X EEG headset1 (Emotiv Systems Inc., San Francisco, CA, United States), which has demonstrated EEG data consistent with conventional EEG recordings (Bobrov et al., 2011; Duvinage et al., 2013) as mentioned in Le et al. (2020), and relatively good research performance (Sawangjai et al., 2019). Additionally, this EEG headset is quick to set up and comfortable for participants, making it potentially more ecologically valid than wet electrode set-ups. The Emotiv Epoc X is a 14 channel headset based on the international 10–20 system which includes electrode sites AF4, AF3, F3, F4, F7, F8, FC5, FC6, O1, O2, P7, P8, T7 and T8. Additional electrodes, M1 and M2, served as the reference and ground electrodes, respectively. Felt pads used to make contact between the electrode and the participant’s scalp were fully soaked in saline solution prior to inserting into each electrode compartment of the headset. The Emotiv Epoc X records data at a 256 Hz sampling rate which ensured that alpha power data was free from aliasing. Emotiv acquisition filters consist of built-in 5th order Sinc filters, with bandwidths set to 0.16–43 Hz; the maximum bandwidth available. In line with best practices in human EEG (Sinha et al., 2016), broadband EEG signals were acquired in situ with alpha bands extracted in data analysis. Line noise at 50 and 60 Hz were filtered using built-in 5th order Sinc notch filters. We used F3 and F4 electrodes to calculate FAA, as this electrode pair is both common in FAA research (Smith et al., 2017; Kuper et al., 2019; David et al., 2021), and unobstructed by the VR headset during recording.
Raw EEG data was recorded in Emotiv’s software, EmotivPro, and exported to EEGLAB (Delorme and Makeig, 2004) in MATLAB (MathWorks) for preprocessing. Data was filtered between 2 Hz (high pass) and 30 Hz (low pass) using eegfiltnew. Next, bad channels were rejected and then interpolated. An average reference was applied to the data, and Independent Component Analysis (ICA) was run on the data with the goal of removing artifacts from the data without removing those portions of data which are affected by artifacts (Delorme and Makeig, 2004). Eye components were removed from the data with 70% probability. Continuous EEG data was segmented into 2 s epochs for each event. Epochs that were ±100 μV were rejected. spectopo from EEGLAB was used on the cleaned EEG data to determine the power spectra in the 8–13 Hz alpha range. To determine FAA, the natural log-transformed alpha power of the left electrode (F3) was subtracted from the natural log-transformed alpha power of the right electrode (F4) using the following formula: (ln [Right] − ln [Left]) (Smith et al., 2017).
As an exploratory analysis, temporal dynamics of FAA bursts were investigated using time series wavelet decomposition analyses adapted from studies by Allen and Cohen (2010). FAA burst analysis codifies fluctuations of FAA activity over shorter time scales than standard FAA measures, and is used to assess micro-scale FAA changes underlying conventional FAA measures. For example, FAA burst analysis has been utilized in understanding resting state neural dynamics in major depressive disorder (MDD). Participants exhibiting lifetime presentation of MDD have shown a high number of total negative FAA bursts as well as increases in temporal precision of positive FAA bursts relative to participants without MDD (Allen and Cohen, 2010). For our FAA burst analysis, EEG signals were mapped to analytic signals by way of the Hilbert transform (Equation 1):
The analytic signal F(t) is a complex-valued function analogous to Fourier representations with negative frequencies discarded. Instantaneous power reflective of the squared magnitude of the total EEG signal at each time sample was calculated from the resulting analytic signal as (Equation 2):
FAA through time between two given electrodes was then calculated as (Equation 3):
Density estimation of FAA values was performed to estimate the underlying FAA distribution. Positive FAA bursts corresponding to greater relative right EEG power across all frequency bands were taken as values greater than the 90th percentile of the FAA distribution. Likewise, negative FAA bursts corresponding to greater relative left EEG power were taken as values less than the 10th percentile of the FAA distribution.
After identification of positive and negative FAA bursts, wavelet decomposition was performed to extract instantaneous alpha power for assessment of time-frequency dynamics of FAA bursts. The time-frequency decomposed signal Φ(t, f) was calculated by convolving the 2s epoch FAA time series with a complex Morlet mother wavelet of the form (Equation 4):
with t being t, f being frequency, and j being the imaginary number
Average alpha power was calculated as the mean power per frequency in the alpha band and converted to a decibel scale.
In this study, we investigated four image sequences: V2A2: low valence and low arousal; V2A8: low valence and high arousal; V8A2: high valence and low arousal; and V8A8: high valence and high arousal, shown in Figure 3. The order of image sequence presentation for each participant was randomized within our application. Each image sequence included 10 images and was displayed for 4 seconds, following image presentation recommendations by Chen et al. (2006), for a total of 40 s per image sequence. Please see Mousas et al. (2021) for more information concerning the development of the image sequences.
The images used in our image sequences were originally taken from the Open Affective Standardized Image Set (OASIS) (Kurdi et al., 2017), which provides an open-access database of 900 color images comprising a variety of themes. The OASIS dataset additionally contains normative ratings of both valence and arousal dimension of affect, collected from an online study with 822 participants (aged 18–74) in 2015, with an equal gender distribution. In our previous work (Mousas et al., 2021) we developed a system to generate image sequences with target valence and arousal values from the annotated image dataset, and validated our image sequences through a user study.
Participants first signed our consent form upon arriving at the lab. Next, participants were fitted with the EEG headset. The researcher showed participants their brain signal visualization in real-time within the Emotiv software, and asked participants to try frowning, talking, and moving. In this way, participants were able to see their brain signal and understand how motion artifacts could decrease data quality. Participants were fitted with the Oculus Quest VR headset and could adjust the headset for comfort. A participant wearing both devices can be seen in Figure 4. Before starting the application, the researcher explained that participants should think about how they feel while viewing each image sequence.
Once the application was started, participants were invited to look around and become familiar with the VE, so that they might become immersed in the environment before beginning the image viewing task. Once the participant was ready to start the experiment, they faced the screen in the VE and the researcher started the experiment in Unity. After starting the experiment, the researcher left the room to ensure a private viewing experience (Davidson et al., 1990). First, participants viewed a fixation cross (white crosshair on black background) on the screen in the VE for 40 s, which served as an FAA baseline. Participants were instructed to minimize mental wandering during this time and to remain as still as possible. After the baseline period, image sequences were presented in a randomized order. Each image sequence was separated by a 5 s fixation cross. Participants were instructed to look at the fixation cross during these intervals. Participants spent about 35 minutes in the lab to complete the experiment. Please see Figure 5 for a flowchart of the study and Figure 6 for a visualization of the VR application components.
We investigated the following four image sequences: V2A2: low valence, low arousal; V8A2, high valence, low arousal; V2A8, low valence, high arousal; and V8A8, and high valence, high arousal. We used Greenhouse-Geisser corrections when Mauchley’s test of sphericity was violated. Bonferroni corrections were used for post hoc comparisons. Missing data and outliers were replaced with mean values prior to statistical analysis. Our statistical analyses are described below.
First, we used a two-way repeated measures Analysis of Variance (ANOVA) to explore differences in FAA between two levels of Arousal and Valence image sequences. The Shapiro-Wilk test indicated these values were normally distributed. These results can be found in Section 3.1.
As FAA scores alone do not indicate the contribution of left and right prefrontal cortices to FAA (Smith et al., 2017), we conducted an additional analysis to determine if greater FAA scores were indicative of increased left cortical activity, or decreased right cortical activity. We investigated alpha power from each side separately in order to learn about each hemisphere’s contribution to the FAA score. FAA is calculated by subtracting the natural log of the alpha power from the left electrode from the natural log of the alpha power from the right electrode. Therefore, examining alpha power of each electrode separately is informative in understanding which side has higher cortical activity. Because alpha power is inversely related to cortical activity, a higher alpha power from the right electrode would indicate lower cortical activity from the right electrode. According to Harmon-Jones and Gable (2018), greater left than right activity is associated with happiness and greater approach motivation; the tendency of an organism to expend energy to go towards a stimuli, while greater right than left activity is associated with fear and withdrawal motivation; the tendency to expend energy to move away from a stimuli.
To understand the contribution of left and right alpha power to FAA, we conducted three-way repeated measures ANOVAs with Side (left vs. right), Valence (high vs. low) and Arousal (high vs. low) as factors. Alpha power data was not normally distributed. Therefore, we transformed data following recommendations by Templeton (2011) prior to conducting our parametric analyses on the normally transformed data. These results can be found in Section 3.2. Additionally, we examined differences between FAA recorded during baseline and FAA recorded during each image sequence through one-tailed paired samples t-tests. These results can be found in Section 3.3.
In our FAA burst analyses, we investigated total FAA bursts, total positive FAA bursts, total negative FAA bursts, positive FAA burst power, and negative FAA burst power; all of which were normally distributed according to the Kolmogorov-Smirnov test of normality. A two-way analysis of variance (ANOVA) was was performed with Arousal and Valence of image sequences as factors. These results can be found in Section 3.4.
Below we describe our results concerning FAA, left and right prefrontal cortical activity, differences in FAA from baseline measurements, and FAA burst analyses.
While our results do not demonstrate a significant interaction effect between valence and arousal
We investigated the contribution of Side (left vs. right), Valence (high vs. low), and Arousal (high vs. low) on Alpha Power values with a three-way repeated measures ANOVA. From this analysis, we determined a main effect of Side on
To understand if FAA values during image sequence viewing were different from baseline FAA, we conducted one-tailed paired samples t-tests with FAA measured during V2A2, V2A8, V8A2, and V8A8 image sequence viewing. We determined a significant increase in FAA from baseline to V8A2 (t [23] = −3.166, p = 0.002), which showed that FAA during V8A2 (M = 0.36, SD = 0.36) was significantly higher than FAA during baseline (M = 0.15, SD = 0.37). Neither V2A8 (M = 0.19, SD = 0.29) nor V8V8 (M = 0.19, SD = 0.29) differed significantly from baseline; (t [23] = −0.670, p = 0.255) and (t [23] = −0.671, p = 0.254), respectively. Lastly, FAA during V2A2 (M = 0.26, SD = 0.37) did not significantly differ from than FAA during baseline (M = 0.15, SD = 0.37), (t [23] = −1.636, p = 0.058), as seen in Figure 7.
In examining the total number of positive and negative FAA bursts, no significant differences in burst count between arousal and valence levels were found, as seen in Figure 8. Furthermore, there were no significant differences in number of positive bursts, number of negative bursts, and burst power between arousal and valence levels, as seen in Table 1.
FIGURE 8. FAA temporal dynamics were analyzed using FAA burst measures. (A) A sample time-frequency wavelet decomposition from an FAA time series (red) from one epoch of one image sequence (V2A2). Time-frequency power was smoothed via convolution with a Gaussian kernel with a standard deviation of 2 to ease viewing. Power calculations were performed on raw data. (B). Total number of FAA bursts remained consistent between valence and arousal image sequence levels.
We investigated FAA during affective image sequence viewing in a VR theater environment. Twenty-four participants viewed image sequences with predetermined valence and arousal values while wearing a consumer-grade EEG headset. Our results show that image sequences with higher valence ratings elicited significantly higher FAA than image sequences with lower valence ratings, supporting our first hypothesis. Our results would seem to follow the theory that approach-motivation is associated with positive emotions (Davidson et al., 1990; Kisker et al., 2021). Interestingly, FAA was higher during image sequence viewing of low arousal than during image sequence viewing of high arousal. Considering that individual differences may impact both reports of affect, as well as affective responses (Kuppens et al., 2013), we imagine that future research could reveal that a relationship between valence and arousal may exist, perhaps in studies with a more diverse sample of participants.
In immersive environments, such as Cave Automatic Virtual Environments (CAVE), self-reported ratings such as “sad,” “funny,” “scary,” and “beautiful” have been shown to be higher for immersive environments than for the same stimuli presented in a 2D fashion (Visch et al., 2010). Considering that emotional intensity/arousal can be greater in immersive environments (Visch et al., 2010), and that VR can elicit stronger emotional responses than 2D viewing (Gorini et al., 2010), we understand FAA scores in this study may be different from image sequence viewing in 2D. Therefore, it is possible that participant arousal levels were higher due to the VR experience generally. Because ratings of valence and arousal can be modulated by differences in political and cultural attitudes (Kurdi et al., 2017), it is also possible that our FAA values reflected different perceptions of image context leading to differences in arousal, as both perception and information can mediate arousal (Diemer et al., 2015).
In a study investigating emotionally contagious sound stimuli (Papousek et al., 2014), participants who were higher in emotion regulation ability showed greater relative right frontal cortical activity when listening to anxiety-inducing stimuli (Lacey et al., 2020), indicating the role of individual differences in affective processing and FAA. As effortful control of emotions is also thought to play a role in FAA (Lacey et al., 2020), further investigation concerning individual differences in emotion regulation, and emotional reactivity would be helpful in clarifying our results. Additionally, negative affect elicited from negatively valenced stimuli can persist longer than positive affect (Davidson et al., 1990). Because the image sequences order was randomized, negative affect from previous image sequences may have influenced certain participants more than others. Considering that negative affective stimuli may cause participants to exert significant effort in order to engage with the stimuli, whereas, outside a laboratory setting, participants would instead choose not to engage at all (Lacey et al., 2020), it is possible that participants differed in their ability to exert effortful control to engage with negative stimuli.
In examining alpha power from left and right electrode sites, we determined that left prefrontal cortical activity was greater than right prefrontal cortical activity during the image viewing experience, which could be indicative of greater approach motivation or positive affect during the VR experience more generally. While our data suggests greater cortical activity during high valence than low valence image sequences, these results do not reach statistical significance at p = 0.053. Additionally, our second hypothesis is not supported, as we determined no difference in left and right prefrontal cortical activity during high valence image sequence viewing.
We determined that FAA during V8A2 image sequence viewing was significantly greater than FAA recorded during baseline. However, neither V8A8, V2A2, nor V2A8 image sequences elicited FAA significantly greater than FAA during baseline. Therefore, we consider our hypothesis only partially supported. Because we could only determine that FAA during the V8A2 image sequence was significantly greater than FAA during baseline, it is likely that the other image sequences did not elicit as strong of an emotional response. Considering that our results additionally showed that FAA was highest for high valence, and low arousal image sequences, it is possible that arousal may play a role in eliciting lower FAA.
All FAA values appear to increase from baseline, which suggests that the immersive, engaging properties of the virtual environment may have been stronger than the emotional attributes of the majority of image sequences. It is also possible that the consumer-grade EEG recording device may not have performed well enough to detect smaller changes in FAA, perhaps leading to statistically similar FAA values during image sequence viewing and baseline. Although this device was selected for its ecological validity, future research would benefit from a comparison study using a medical-grade EEG device.
Lastly, our additional FAA burst analysis suggests that temporal dynamics did not differ significantly across the four image sequences, suggesting new avenues for research investigating the time course of affective responses. While we used a strict burst threshold of 90th percentile of FAA distribution in line with previous work (Allen and Cohen, 2010), it is possible that lower-level bursting activity better encodes valence and arousal levels, especially with a consumer-grade EEG device.
Our study is limited in that we did not investigate presence, which may influence emotional responses in VR experiences (Diemer et al., 2015). Therefore, we do not know if FAA responses were influenced by potentially varying levels of presence among different participants. Because presence is considered necessary in order for real emotional responses to be elicited in VR (Parsons and Rizzo, 2008; Diemer et al., 2015), we assume that participants felt some level of presence, as we were able to determine differences in FAA with different image sequences ratings of valence and arousal. Additionally, our interstimulus intervals (ISIs) were fixed at 5 seconds, therefore, predictability of image sequence presentation may have impacted results as well.
In our future work, we would like to further examine the role of individual differences in affective responses to VR experiences, as individual differences appear fundamental in FAA (Lacey et al., 2020). Considering participants had no task other than paying attention to how they felt while viewing image sequences, it is notable that we were able to determine differences in FAA despite this passive experience. To examine how agency may influence FAA, we would like to investigate FAA responses to affective VR experiences in which participants have an active role in the environment. Because VR embodiment has been shown to increase emotional responses to virtual stimuli as seen through self-reported data (Gall et al., 2021), we would like to examine the role of VR embodiment in influencing FAA responses as well. Future work might additionally include electrodermal activity (EDA) in combination with EEG data in order to better understand affective responses to stimuli with predetermined arousal and valence values in VR.
Our results suggest that FAA recorded while viewing image sequences in a VR theater environment may be influenced by both valence and arousal values. We determined that FAA was significantly greater during high valence image sequence viewing than during low valence image sequence viewing. However, FAA was significantly greater during low arousal image sequence viewing than high arousal image sequence viewing. Additionally, FAA elicited during the high valence, low arousal image sequence was significantly greater than FAA during baseline, further supporting the complex relationship that may exist between valence and arousal concerning FAA. While FAA data may be useful in understanding associations between valence and arousal self-reported data and brain activity responses elicited from affective experiences in VR environments, it will be important to consider individual differences in affective processing styles of participants, and further examine the relationship between valence and arousal in future research.
The datasets presented in this study can be found in online repositories. The names of the repository/repositories and accession number(s) can be found below: https://osf.io/6xb9z/?view_only = 6a41db5dccbd487e8b96a4477a7abfd0. Code for conducting FAA burst analyses can be found online at: https://github.com/bscoventry/AsymmetryBursts.
The studies involving human participants were reviewed and approved by the Purdue University Institutional Review Board. The patients/participants provided their written informed consent to participate in this study. Written informed consent was obtained from the individual(s) for the publication of any potentially identifiable images or data included in this article.
CK and CM developed the concept. CK developed the Unity environment, and conducted data collection. CK and BSC performed EEG preprocessing and analyses. CK, CM, and BSC completed statistical analyses. CK and BSC wrote the manuscript. CK and CM revised the manuscript. CM acquired funding for the study.
Publication of this article was funded by Purdue University Libraries Open Access Publishing Fund.
The authors declare that the research was conducted in the absence of any commercial or financial relationships that could be construed as a potential conflict of interest.
All claims expressed in this article are solely those of the authors and do not necessarily represent those of their affiliated organizations, or those of the publisher, the editors and the reviewers. Any product that may be evaluated in this article, or claim that may be made by its manufacturer, is not guaranteed or endorsed by the publisher.
1https://www.emotiv.com/epoc-x/.
Allen, J. J. B., and Cohen, M. X. (2010). Deconstructing the “Resting” State: Exploring the Temporal Dynamics of Frontal Alpha Asymmetry as an Endophenotype for Depression. Front. Hum. Neurosci. 4, 232. doi:10.3389/fnhum.2010.00232
Bobrov, P., Frolov, A., Cantor, C., Fedulova, I., Bakhnyan, M., and Zhavoronkov, A. (2011). Brain-computer Interface Based on Generation of Visual Images. PloS one 6, e20674. doi:10.1371/journal.pone.0020674
Checa, D., and Bustillo, A. (2020). A Review of Immersive Virtual Reality Serious Games to Enhance Learning and Training. Multimed. Tools Appl. 79, 5501–5527. doi:10.1007/s11042-019-08348-9
Chen, J.-C., Chu, W.-T., Kuo, J.-H., Weng, C.-Y., and Wu, J.-L. (2006). Tiling Slideshow. Proc. 14th ACM Int. Conf. Multimedia, 25–34. doi:10.1145/1180639.1180653
Cohen, J. (1988). Statistical Power Analysis for the Behavioral Sciences. 2 edn. New Jersey, USA: Lawrence Erlbaum Associates.
David, O. A., Predatu, R., and Maffei, A. (2021). REThink Online Video Game for Children and Adolescents: Effects on State Anxiety and Frontal Alpha Asymmetry. J. Cogn. Ther. 14, 399–416. doi:10.1007/s41811-020-00077-4
Davidson, R. J., Ekman, P., Saron, C. D., Senulis, J. A., and Friesen, W. V. (1990). Approach-withdrawal and Cerebral Asymmetry: Emotional Expression and Brain Physiology: I. J. Personality Soc. Psychol. 58, 330–341. doi:10.1037/0022-3514.58.2.330
Delorme, A., and Makeig, S. (2004). Eeglab: an Open Source Toolbox for Analysis of Single-Trial Eeg Dynamics Including Independent Component Analysis. J. Neurosci. methods 134, 9–21. doi:10.1016/j.jneumeth.2003.10.009
Diemer, J., Alpers, G. W., Peperkorn, H. M., Shiban, Y., and Mã¼hlberger, A. (2015). The Impact of Perception and Presence on Emotional Reactions: a Review of Research in Virtual Reality. Front. Psychol. 6, 26. doi:10.3389/fpsyg.2015.00026
Ding, N., Zhou, W., and Fung, A. Y. H. (2018). Emotional Effect of Cinematic Vr Compared with Traditional 2d Film. Telematics Inf. 35, 1572–1579. doi:10.1016/j.tele.2018.04.003
Duvinage, M., Castermans, T., Petieau, M., Hoellinger, T., Cheron, G., and Dutoit, T. (20132013). Cheron,. g., and Dutoit, Tperformance of the Emotiv Epoc Headset for P300-Based Applications. Biomed. Eng. OnLine 12, 56. doi:10.1186/1475-925x-12-56
Faul, F., Erdfelder, E., Lang, A.-G., and Buchner, A. (2007). G*Power 3: A Flexible Statistical Power Analysis Program for the Social, Behavioral, and Biomedical Sciences. Behav. Res. methods 39, 175–191. doi:10.3758/bf03193146
Felnhofer, A., Kothgassner, O. D., Schmidt, M., Heinzle, A.-K., Beutl, L., Hlavacs, H., et al. (2015). Is Virtual Reality Emotionally Arousing? Investigating Five Emotion Inducing Virtual Park Scenarios. Int. J. human-computer Stud. 82, 48–56. doi:10.1016/j.ijhcs.2015.05.004
Gall, D., Roth, D., Stauffert, J. P., Zarges, J., and Latoschik, M. E. (2021). Embodiment in Virtual Reality Intensifies Emotional Responses to Virtual Stimuli. Front. Psychol. 12, 674179. doi:10.3389/fpsyg.2021.674179
Gorini, A., Griez, E., Petrova, A., and Riva, G. (2010). Assessment of the Emotional Responses Produced by Exposure to Real Food, Virtual Food and Photographs of Food in Patients Affected by Eating Disorders. Ann. Gen. Psychiatry 9, 30–20. doi:10.1186/1744-859X-9-30
Harmon-Jones, E., and Gable, P. A. (2018). On the Role of Asymmetric Frontal Cortical Activity in Approach and Withdrawal Motivation: An Updated Review of the Evidence. Psychophysiology 55, e12879. doi:10.1111/psyp.12879
Hayes, D. J., Duncan, N. W., Xu, J., and Northoff, G. (2014). A Comparison of Neural Responses to Appetitive and Aversive Stimuli in Humans and Other Mammals. Neurosci. Biobehav. Rev. 45, 350–368. doi:10.1016/j.neubiorev.2014.06.018
He, L., Li, H., Xue, T., Sun, D., Zhu, S., and Ding, G. (2018). “Am I in the Theater? Usability Study of Live Performance Based Virtual Reality,” in Proceedings of the 24th ACM Symposium on Virtual Reality Software and Technology, 1–11.
Kerous, B., Barteček, R., Roman, R., Sojka, P., Bečev, O., and Liarokapis, F. (2020). Social Environment Simulation in Vr Elicits a Distinct Reaction in Subjects with Different Levels of Anxiety and Somatoform Dissociation. Int. J. Human-Computer Interact. 36, 505–515. doi:10.1080/10447318.2019.1661608
Kim, A., Chang, M., Choi, Y., Jeon, S., and Lee, K. (2018). “The Effect of Immersion on Emotional Responses to Film Viewing in a Virtual Environment,” in 2018 IEEE Conference on Virtual Reality and 3D User Interfaces (VR) (IEEE), 601–602. doi:10.1109/vr.2018.8446046
Kisker, J., Lange, L., Flinkenflügel, K., Kaup, M., Labersweiler, N., Tetenborg, F., et al. (2021). Authentic Fear Responses in Virtual Reality: A Mobile Eeg Study on Affective, Behavioral and Electrophysiological Correlates of Fear. Front. Virtual Real. 106. doi:10.3389/frvir.2021.716318
Kuper, N., Käckenmester, W., Wacker, J., and Fajkowska, M. (2019). Resting Frontal Eeg Asymmetry and Personality Traits: A Meta-Analysis. Eur. J. Pers. 33, 154–175. doi:10.1002/per.2197
Kuppens, P., Tuerlinckx, F., Russell, J. A., and Barrett, L. F. (2013). The Relation between Valence and Arousal in Subjective Experience. Psychol. Bull. 139, 917–940. doi:10.1037/a0030811
Kurdi, B., Lozano, S., and Banaji, M. R. (2017). Introducing the Open Affective Standardized Image Set (Oasis). Behav. Res. 49, 457–470. doi:10.3758/s13428-016-0715-3
Lacey, M. F., Neal, L. B., and Gable, P. A. (2020). Effortful Control of Motivation, Not Withdrawal Motivation, Relates to Greater Right Frontal Asymmetry. Int. J. Psychophysiol. 147, 18–25. doi:10.1016/j.ijpsycho.2019.09.013
Le, T. P., Lucas, H. D., Schwartz, E. K., Mitchell, K. R., and Cohen, A. S. (2020). Frontal Alpha Asymmetry in Schizotypy: Electrophysiological Evidence for Motivational Dysfunction. Cogn. Neuropsychiatry 25, 371–386. doi:10.1080/13546805.2020.1813096
Lin, J.-H. T. (2017). Fear in Virtual Reality (Vr): Fear Elements, Coping Reactions, Immediate and Next-Day Fright Responses toward a Survival Horror Zombie Virtual Reality Game. Comput. Hum. Behav. 72, 350–361. doi:10.1016/j.chb.2017.02.057
Lindström, M., Ståhl, A., Höök, K., Sundström, P., Laaksolathi, J., Combetto, M., et al. (2006). Affective Diary: Designing for Bodily Expressiveness and Self-Reflection. CHI’06 Ext. Abstr. Hum. factors Comput. Syst., 1037–1042. doi:10.1145/1125451.1125649
Marín-Morales, J., Higuera-Trujillo, J. L., Greco, A., Guixeres, J., Llinares, C., Scilingo, E. P., et al. (2018). Affective Computing in Virtual Reality: Emotion Recognition from Brain and Heartbeat Dynamics Using Wearable Sensors. Sci. Rep. 8, 1–15. doi:10.1038/s41598-018-32063-4
Marín-Morales, J., Llinares, C., Guixeres, J., and Alcañiz, M. (2020). Emotion Recognition in Immersive Virtual Reality: From Statistics to Affective Computing. Sensors 20, 5163. doi:10.3390/s20185163
Mousas, C., Krogmeier, C., and Wang, Z. (2021). Photo Sequences of Varying Emotion: Optimization with a Valence-Arousal Annotated Dataset. ACM Trans. Interact. Intell. Syst. 11, 1–19. doi:10.1145/3458844
Papousek, I., Weiss, E. M., Schulter, G., Fink, A., Reiser, E. M., and Lackner, H. K. (2014). Prefrontal Eeg Alpha Asymmetry Changes while Observing Disaster Happening to Other People: Cardiac Correlates and Prediction of Emotional Impact. Biol. Psychol. 103, 184–194. doi:10.1016/j.biopsycho.2014.09.001
Parsons, T. D., and Rizzo, A. A. (2008). Affective Outcomes of Virtual Reality Exposure Therapy for Anxiety and Specific Phobias: A Meta-Analysis. J. Behav. Ther. Exp. psychiatry 39, 250–261. doi:10.1016/j.jbtep.2007.07.007
Poria, S., Cambria, E., Bajpai, R., and Hussain, A. (2017). A Review of Affective Computing: From Unimodal Analysis to Multimodal Fusion. Inf. Fusion 37, 98–125. doi:10.1016/j.inffus.2017.02.003
Quigley, K. S., Lindquist, K. A., and Barrett, L. F. (2014). Inducing and Measuring Emotion and Affect: Tips, Tricks, and Secrets. Handb. Res. Methods Soc. Personality Psychol., 220–252. doi:10.1017/cbo9780511996481.014
Robinson, R., Wiley, K., Rezaeivahdati, A., Klarkowski, M., and Mandryk, R. L. (2020). “let’s Get Physiological, Physiological!” a Systematic Review of Affective Gaming,” in Proceedings of the Annual Symposium on Computer-Human Interaction in Play, 132–147.
Rodrigues, J., Müller, M., Mühlberger, A., and Hewig, J. (2018). Mind the Movement: Frontal Asymmetry Stands for Behavioral Motivation, Bilateral Frontal Activation for Behavior. Psychophysiol 55, e12908. doi:10.1111/psyp.12908
Russell, J. A. (1980). A Circumplex Model of Affect. J. personality Soc. Psychol. 39, 1161–1178. doi:10.1037/h0077714
Sawangjai, P., Hompoonsup, S., Leelaarporn, P., Kongwudhikunakorn, S., and Wilaiprasitporn, T. (2019). Consumer Grade Eeg Measuring Sensors as Research Tools: A Review. IEEE Sensors J. 20, 3996–4024. doi:10.1109/jsen.2019.2962874
Schöne, B., Kisker, J., Sylvester, R. S., Radtke, E. L., and Gruber, T. (2021). Library for Universal Virtual Reality Experiments (Luvre): A Standardized Immersive 3d/360 Picture and Video Database for Vr Based Research. Curr. Psychol., 1–19. doi:10.1007/s12144-021-01841-1
Schöne, B., Schomberg, J., Gruber, T., and Quirin, M. (2016). Event-related Frontal Alpha Asymmetries: Electrophysiological Correlates of Approach Motivation. Exp. Brain Res. 234, 559–567. doi:10.1007/s00221-015-4483-6
Sinha, S. R., Sullivan, L. R., Sabau, D., Orta, D. S. J., Dombrowski, K. E., Halford, J. J., et al. (2016). American Clinical Neurophysiology Society Guideline 1: Minimum Technical Requirements for Performing Clinical Electroencephalography. Neurodiagnostic J. 56, 235–244. doi:10.1080/21646821.2016.1245527
Smith, E. E., Reznik, S. J., Stewart, J. L., and Allen, J. J. B. (2017). Assessing and Conceptualizing Frontal Eeg Asymmetry: An Updated Primer on Recording, Processing, Analyzing, and Interpreting Frontal Alpha Asymmetry. Int. J. Psychophysiol. 111, 98–114. doi:10.1016/j.ijpsycho.2016.11.005
Templeton, G. F. (2011). A Two-step Approach for Transforming Continuous Variables to Normal: Implications and Recommendations for Is Research. Commun. Assoc. Inf. Syst. 28, 4. doi:10.17705/1cais.02804
Visch, V. T., Tan, E. S., and Molenaar, D. (2010). The Emotional and Cognitive Effect of Immersion in Film Viewing. Cognition Emot. 24, 1439–1445. doi:10.1080/02699930903498186
Voigt-Antons, J.-N., Spang, R., Kojić, T., Meier, L., Vergari, M., and Möller, S. (2021). “Don’t Worry Be Happy-Using Virtual Environments to Induce Emotional States Measured by Subjective Scales and Heart Rate Parameters,” in 2021 IEEE Virtual Reality and 3D User Interfaces (VR) (IEEE), 679–686. doi:10.1109/vr50410.2021.00094
Keywords: virtual reality, mobile EEG, frontal alpha asymmetry, affective images, valence, arousal, emotion, frontal alpha asymmetry burst analysis
Citation: Krogmeier C, Coventry BS and Mousas C (2022) Affective Image Sequence Viewing in Virtual Reality Theater Environment: Frontal Alpha Asymmetry Responses From Mobile EEG. Front. Virtual Real. 3:895487. doi: 10.3389/frvir.2022.895487
Received: 13 March 2022; Accepted: 24 June 2022;
Published: 19 July 2022.
Edited by:
Maria Limniou, University of Liverpool, United KingdomReviewed by:
Ir. Ts. Dr. Norizam Sulaiman, Universiti Malaysia Pahang, MalaysiaCopyright © 2022 Krogmeier, Coventry and Mousas. This is an open-access article distributed under the terms of the Creative Commons Attribution License (CC BY). The use, distribution or reproduction in other forums is permitted, provided the original author(s) and the copyright owner(s) are credited and that the original publication in this journal is cited, in accordance with accepted academic practice. No use, distribution or reproduction is permitted which does not comply with these terms.
*Correspondence: Claudia Krogmeier, Y2tyb2dtZWlAcHVyZHVlLmVkdQ==
Disclaimer: All claims expressed in this article are solely those of the authors and do not necessarily represent those of their affiliated organizations, or those of the publisher, the editors and the reviewers. Any product that may be evaluated in this article or claim that may be made by its manufacturer is not guaranteed or endorsed by the publisher.
Research integrity at Frontiers
Learn more about the work of our research integrity team to safeguard the quality of each article we publish.