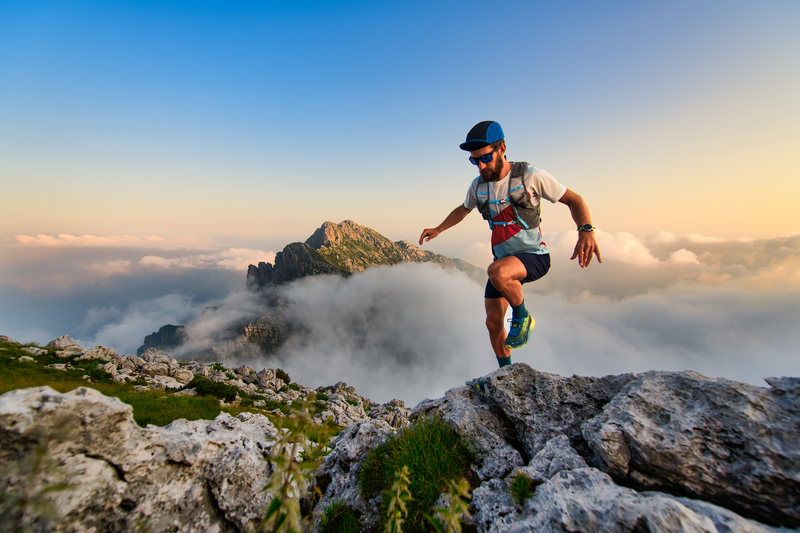
95% of researchers rate our articles as excellent or good
Learn more about the work of our research integrity team to safeguard the quality of each article we publish.
Find out more
ORIGINAL RESEARCH article
Front. Virtual Real. , 16 June 2022
Sec. Virtual Reality and Human Behaviour
Volume 3 - 2022 | https://doi.org/10.3389/frvir.2022.882526
This article is part of the Research Topic Human Spatial Perception, Cognition, and Behaviour in Extended Reality View all 13 articles
Virtual reality users are susceptible to disorientation, particularly when using locomotion interfaces that lack self-motion cues. Environmental cues, such as boundaries defined by walls or a fence, provide information to help the user remain oriented. This experiment evaluated whether the type of boundary impacts its usefulness for staying oriented. Participants wore a head-mounted display and performed a triangle completion task in virtual reality by traveling two outbound path segments before attempting to point to the path origin. The task was completed with two teleporting interfaces differing in the availability of rotational self-motion cues, and within five virtual environments differing in the availability and type of boundaries. Pointing errors were highest in an open field without environmental cues, and lowest in a classroom with walls and landmarks. Environments with a single square boundary defined by a fence, drop-off, or floor texture discontinuity led to errors in between the open field and the classroom. Performance with the floor texture discontinuity was similar to that with navigational barriers (i.e., fence and drop-off), indicating that an effective barrier need not be a navigational impediment. These results inform spatial cognitive theory about boundary-based navigation and inform application by specifying the types of environmental and self-motion cues that designers of virtual environments should include to reduce disorientation in virtual reality.
Small virtual environments (VEs) can often be explored by foot, whereby physical walking through the real environment corresponds directly to movement through the VE. However, most VEs are larger than the surrounding real environment, requiring a locomotion interface in order to completely explore the VE. Locomotion interfaces have proliferated in recent years, and their diversity is captured in a database called the “Locomotion Vault” (Di Luca et al., 2021). Fundamentally, locomotion interfaces separate movement through the real environment from movement through the VE. For example, joystick locomotion typically involves a stationary user moving smoothly through the VE.
The teleporting interface is widely used in VR applications due to its ease of use (Bozgeyikli et al., 2016; Langbehn et al., 2018) and low incidence of cybersickness (Christou and Aristidou, 2017; Langbehn et al., 2018; Moghadam et al., 2018; Weissker et al., 2018). To teleport, the user selects a position (and sometimes an orientation) in the VE and is then instantly transported to that location without the visual and body-based self-motion cues that accompany real world movement. The lack of self-motion cues when teleporting reduces cybersickness, but can also cause disorientation. For example, participants in one study (Cherep et al., 2020) performed a triangle completion task in which they traveled along two outbound path segments before attempting to point to the path origin (i.e., to complete the triangle). Triangle completion errors were smallest when participants traveled the outbound path by physically walking and turning, were larger when they teleported to translate (i.e., to change position) but rotated their own bodies to turn, and largest when they teleported to change their position as well as their orientation. The latter two conditions are referred to herein as partially concordant teleporting (teleport to translate, use the body to rotate) and discordant teleporting (teleport to translate and rotate). These labels reflect the level of concordance between movement of the body and movement through the VE. The disorientation consequences of teleporting occur across multiple scales of movement (Kelly et al., 2020), and they also impact the accuracy of acquired cognitive maps (i.e., knowledge of distances and directions between environmental locations) after exploring a large-scale VE (Lim et al., 2020). This study explores whether appropriate use of boundaries might mitigate spatial disorientation.
In addition to self-motion cues, human navigators also rely on environmental cues, including boundaries and landmarks. Environmental boundaries were found to be particularly useful at reducing disorientation when using the teleporting interface (Cherep et al., 2020). Although the theory that environmental shape (commonly defined by boundaries) is processed in a dedicated module within the human mind has fallen out of favor (Cheng, 2008; Twyman and Newcombe, 2010), boundaries are undoubtedly important navigational cues (Hartley et al., 2004; Doeller and Burgess, 2008; Kelly et al., 2009; Chen et al., 2015; Zhou and Mou, 2019; Cherep et al., 2020). One reason for their importance is their ubiquitousness in the environment: the walls of a surrounding rectangular room can be seen from nearly any perspective. Animal research has identified specialized neurons, termed “boundary vector cells,” in the rodent brain which respond specifically to environmental boundaries such as walls (Lever et al., 2009). Detailed discussion of these cells can be found later in this section, but evidence indicates that humans may possess a similar representational system (Lee et al., 2018; Shine et al., 2019).
Given that boundaries can mitigate disorientation inherent in some locomotion interfaces, VE designers should be motivated to include boundaries in order to combat disorientation. One remaining hurdle before recommending this approach is that the definition of a useful boundary is unclear. Kevin Lynch’s classic architectural analysis of cities (Lynch, 1964), for example, noted that boundaries between city regions can be “hard, definite, precise” or “soft or uncertain” (p. 69). More concretely, must a boundary be defined by a vertical barrier (e.g., a wall) in order for it to positively impact navigation, or would a texture-defined boundary on the ground plane (e.g., a flooring transition) suffice? These questions are central to the current project, and research in psychology and neuroscience provides some clues to help narrow the focus.
One related study used a spatial memory task to compare the effect of boundaries that did or did not impede movement (Negen et al., 2020). Prior to the spatial memory task, participants in one condition were instructed to move their hand through a virtual wall, which showed them that it was not a navigational impediment. In another condition a plywood board was co-located with the virtual wall so that participants experienced it as a navigational barrier. Yet another condition presented a virtual wall that extended beneath a transparent floor and therefore could not impede movement. Participants then learned and later recalled locations of objects placed near the boundary. Recalled locations were biased away from all three types of boundaries (passable, non-passable, and below the floor), and the bias patterns were distinct from a fourth condition in which landmarks (specifically traffic cones) replaced the walls. These results indicate that boundaries have a similar effect on spatial cognition whether or not they are perceived as impediments to movement, and that the effect of boundaries differs from that of landmarks (i.e., the traffic cones). Although that study investigated spatial memory and not navigation, it is possible that a boundary defined by walls, a drop-off, or a texture discontinuity on the ground will all serve as effective cues for reducing disorientation in VR.
Animal neuroscience research has developed an exquisite picture of how space is represented in animal brains (Barry and Burgess, 2014). One component of this system is the boundary vector cell (Lever et al., 2009), which may also exist in the human brain (Lee et al., 2018; Shine et al., 2019). Boundary vector cells respond selectively based on the direction and distance of environmental boundaries, and likely play a key role in orienting the animal to the surrounding environment. In rodents, boundary vector cells respond strongly to walls (Lever et al., 2009), drop-offs (Stewart et al., 2014), and floor texture discontinuities (Wang et al., 2020). If humans possess a similar mechanism for representing boundaries, and if that boundary representation directly impacts spatial orientation, then one might hypothesize that walls, drop-offs, and floor texture discontinuities will all serve to reduce disorientation within the environment. Of course, extrapolating from animal neuroscience to human behavior involves many assumptions that should be approached with a good deal of caution.
In contrast to the animal research, human neuroscience research on boundaries suggests that texture discontinuities may be processed differently from navigational barriers. One study (Julian et al., 2016) used transcranial magnetic stimulation (TMS) to selectively interfere with neural activity in the occipital place area, which is centrally involved in visual scene processing. TMS negatively affected spatial memory for locations relative to walls but did not affect memory for locations relative to a texture discontinuity on the ground, indicating that texture boundaries are represented outside of the occipital place area. This does not rule out the possibility that texture discontinuities could still be useful for navigation, but it does show that texture discontinuities and walls are processed in distinct neural pathways.
Returning to disorientation in VR, boundaries defined by walls or a fence have been shown to reduce disorientation caused by the teleporting interface (Cherep et al., 2020). However, past research has not systematically evaluated whether boundaries defined in other ways, such as drop-offs or texture discontinuities on the ground, are similarly beneficial. This information would not only be useful to the VE designer interested in reducing disorientation by adding useful cues to the environment, but can also add to our understanding of how people recognize and treat something as a navigationally-relevant boundary. Therefore, the current study explored the impact of these boundaries on disorientation in VR. Participants performed a triangle completion task in five VEs varying in the available environmental cues. Participants traveled the outbound path of the triangle using two teleporting interfaces, partially concordant teleporting and discordant teleporting, which differ in the availability of rotational self-motion cues. A baseline walking condition has been used in some related studies, but was excluded here because triangle performance is much more sensitive to environment manipulations when locomotion occurs by teleporting compared to walking (Cherep et al., 2020, 2021).
The desired sample size for this experiment was determined through a power analysis. This study was focused primarily on comparison across VEs containing different boundary cues, so the effect size of the VE manipulation was estimated by averaging the effect size from Experiments 2, 3, and 5 reported by Cherep et al. (2020) because those experiments compared performance between a VE with no useful cues and a VE with useful boundary cues. Only effect sizes associated with the partially concordant teleporting interface were included because the effects were smaller and thus more conservative than those associated with the discordant teleporting interface. This process yielded a d = 0.427. Using a one-tailed, paired sample t-test in G*Power, a sample size of 36 was required to achieve a power of 0.80 with an alpha of 0.05. Due to potential data loss from technical issues and outliers, the target sample was set at 40 participants.
A total of 40 individuals (19 men, 21 women) participated in the experiment in exchange for a gift card worth 16 US dollars. Participants were recruited through a mass e-mail to students at Iowa State University. To be eligible, participants were required to be 18 years or older, able to walk short distances, and without history of photosensitive seizures.
The study followed a 2 (interface: partially concordant teleporting or discordant teleporting) by 5 (VE: open field, drop-off, fence, texture, or classroom) within-participant design. Participants completed two blocks of trials corresponding to the two interfaces, and block order was counterbalanced. Within each interface block, participants completed five blocks of trials corresponding to the five VEs. VE block order was counterbalanced using a balanced Latin square, and the same VE block order was used for the two interface blocks. Within each VE block, participants completed 8 triangle completion trials corresponding to 8 unique path turn angles (see below). Each participant completed 80 triangle completion trials in total.
Triangle completion paths were defined by a sequence of three vertical posts, green, yellow, and red. For each combination of interface and VE, participants performed 8 triangle completion trials corresponding to 8 unique turn angles (−135°, −101.25°, −67.5°, −33.75°, +33.75°, +67.5°, +101.25°, and +135°). Turn angle was manipulated in order to prevent stereotyped responses. Order of turn angle presentation was randomized within each block. Path leg length was randomly selected on each trial from a range of 1.4–2.0 m. Green post locations were randomly selected from 8 possible locations arranged in an elongated ring, shown in the bottom-center panel of Figure 1. Yellow post locations generally led the participant toward the center of the space, and red post locations generally led the participant back out from the center. Two sample paths are depicted in Figure 1.
FIGURE 1. Top row shows screenshots of the open field VE (left), drop-off VE (center), and texture VE (right). Bottom row shows screenshots of the fence VE (left) and classroom VE (right), as well as a top-down view of the triangle completion stimuli (center). The screenshots show views from outside of the space used in the triangle completion task. The top-down view shows all possible locations of the green post, as well as two sample trials marking the locations of the yellow and red posts.
Virtual environments were experienced through an HTC Vive head-mounted display (HMD) outfitted with a Vive Deluxe Audio Strap. The HMD was wirelessly connected to a gaming capable PC. The experiment took place within a 6 by 7 m research lab that was mostly empty except for equipment and small furniture placed at the edges of the room.
There were two teleporting interfaces used to travel the outbound path when performing the triangle completion task. Task videos showing the two interfaces are available on the Open Science Framework (https://osf.io/ckua3/). To travel using the partially concordant teleporting interface, the participant teleported to translate (i.e., to change position) and turned the body to rotate. Pressing and holding the touch-pad button on top of the controller caused a small white ring to appear on the ground plane of the VE (see Figure 2A), connected to the controller by a thin red line (similar to a laser pointer). Pointing the controller changed the position of the circle on the ground plane. Once the participant had selected their intended position in the VE, releasing the touch-pad button caused the participant to be instantly teleported to that location without any translational self-motion cues. Rotating in the VE was accomplished by rotating the body, which generated body-based and visual self-motion cues normally associated with rotation.
FIGURE 2. (A): To use the partially concordant teleporting interface, the participant positioned the concentric white circles to match the position of the post. (B): To use the discordant teleporting interface, the participant positioned and oriented the magenta ring to match the position and orientation of the green post and the arrow at the base of the post.
When using the discordant teleporting interface, the participant traveled the outbound path of the triangle by teleporting to translate and rotate. When pressing and holding the touch-pad button on the controller, a magenta circle with an arrow on one side appeared on the ground plane (see Figure 2B), connected to the controller by a thin red line. Pointing the controller changed the position of the circle, and sliding the thumb around the edge of the circular touch pad changed the orientation of the circle. In this way, the participant selected their intended position and orientation within the VE. Upon releasing the touch-pad button, the participant was instantly teleported to the selected position and orientation with no associated self-motion cues.
The VEs were built in Unity. Screenshots of the 5 VEs are shown in Figure 1. The open field VE contained a large (90 m diameter) grass circle, beyond which was a brown dirt texture extending to the horizon. The fence VE added a 9 by 9 m square white fence (0.75 m tall) to the grass ground plane. The texture VE added a 9 by 9 m square on top of the grass ground plane. The square was textured with a wood flooring pattern that was flush with the ground plane. The drop-off VE included the same wood-textured square, except the square was elevated above the grass ground plane by 2.5 m, creating a drop-off at the edges of the square. The classroom VE (previously used in other similar studies; (Cherep et al., 2020)) displayed a 9 by 9 m room with typical classroom objects, such as windows, a door, and a blackboard. Tables and chairs that would normally be arranged in the center of a classroom were moved to the walls of the room, leaving the center of the room open for the triangle completion task. A practice VE, which was used to give participants an opportunity to familiarize themselves with the task and the interfaces, contained an endless ground plane textured with a grid pattern.
The path used for triangle completion was defined by a sequence of semi-transparent vertical posts, each 1 m tall and 0.25 m in diameter. Arrival at the first post triggered the disappearance of that post and the appearance of the next post in the sequence, and so on until reaching the third post. A green post marked the start of the path (i.e., the path origin), a yellow post marked the end of the first path leg, and a red post marked the end of the second path leg. At the base of each post was a blue arrow pointing to the location of the next post in the sequence. An example of the blue arrow can be seen at the base of the green post in Figure 2. In this example, the blue arrow at the base of the green post points in the direction of the yellow post, which is the next post in the path sequence. The blue arrow was necessary in order to give participants a target orientation when using the discordant teleporting interface (i.e., the post location specified their target location and the blue arrow specified their target orientation). The blue arrow at the base of the red post pointed in the same direction as the arrow at the base of the yellow post. The blue arrow was not necessary when using the partially concordant interface, but it was provided in order to avoid a potential confound. The blue arrow was not expected to provide a useful global orientation cue for performing the task: its orientation differed from the green post to the yellow post, it was only visible until the participant arrived at the post, and its orientation varied across trials (since each triangle completion trial used a different configuration of post locations).
Upon arrival at the research lab, the participant completed the informed consent form as well as a COVID-19 screening form. The researcher then provided a basic description of the triangle completion task and the two teleporting interfaces.
The researcher assisted the participant with donning and adjusting the HMD. The participant first experienced the training VE, where the participant practiced at least three trials of the triangle completion task following the researcher’s instructions. The participant’s task was to travel to a sequence of three posts and then, while standing at the location of the third post, to point to the unseen location of the first post (i.e., to complete the triangle). At the start of each trial, a green post appeared marking the location of the path origin. The participant then traveled to the location of the green post, which disappeared on arrival. Next, a yellow post appeared marking the end of the first path leg. The yellow post disappeared on arrival, and a red post appeared marking the end of the second path leg. The red post disappeared upon arrival and the participant then attempted to point to the location of the path origin (i.e., the green post) by positioning a small blue disk on the ground plane. The disk was connected to the controller by a thin red line. The participant pulled the trigger on the controller to log their response, and the researcher then pressed a key to advance to the next trial. Performance-based feedback was never provided. Participants typically spent less than 5 minutes performing practice trials within the training VE.
Once the participant was comfortable with the task and the teleporting interface, the researcher loaded the first of the five experimental VEs. Upon entering each VE, the researcher instructed the participant to briefly walk around to visually inspect the VE. This was done to ensure that the participant experienced and understood the spatial layout of the boundary (e.g., that the wood floor in the drop-off VE was elevated above the grass). While walking, the researcher described the key features of the VE and confirmed that the participant understood. For example, in the drop-off VE the researcher stated, “The grass is several feet below the wooden platform that you are standing on.” The participant then returned to the center of the lab and began the triangle completion task.
After completing eight trials in each VE, the participant was placed back into the practice VE to practice using the other teleporting interface, after which they repeated the same process in each of the five experimental VEs. Participation lasted up to 1 hour.
Two participants (1 man, 1 woman) did not complete all conditions of the experiment within the allotted time and their data were therefore excluded prior to analysis. Analyses were conducted using data from the remaining 38 participants (18 men, 20 women). All data are provided on the Open Science Framework (https://osf.io/ckua3/).
There are numerous ways to quantify triangle completion performance based on two-dimensional pointing responses. A detailed understanding of the responses is provided by separately analyzing the directional (i.e., angular) component of the response from the distance (i.e., axial) component of the responses. For example, error in perceived facing direction (e.g., misperception of the turn angle on the outbound path) will lead directly to angular error in the pointing response. On the other hand, error in encoding perceived travel distance (e.g., overperception of the outbound path distance) will lead to axial error in the pointing response. Therefore, the analyses focus on these two primary components of pointing error, angular error and axial error.
Travel time (the time elapsed between the start of a trial and when the participant reached the red post) and response time (the time elapsed between arrival at the red post and completion of the pointing response) were also analyzed but are not reported here in detail. In general, these measures responded little to the experimental manipulation of VE and primarily reflected the greater difficulty associated with the discordant teleporting interface through slower travel time and slower response time.
Absolute angular error was defined as the absolute angular deviation between the direction of the response and the direction of the path origin, relative to the participant’s location at the end of the path (i.e., the red post). This variable captures the directional component of the participant’s response, irrespective of the response distance. Visual inspection of signed angular error revealed no notable overall bias toward leftward or rightward responses, nor differences in bias across conditions, so the emphasis is on absolute angular error.
Absolute angular errors were not normally distributed, so a log transformation was used to remove skewness from the data (Osborne and Costello, 2008).1 The result was a more normal distribution with minimal skewness and more similar variances across conditions compared to the untransformed data. Figure 3 shows average untransformed absolute angular error. Plots showing log-transformed data are available on the Open Science Framework (https://osf.io/ckua3/). All participants were within three standard deviations of the log-transformed mean error, so all were included in the analyses.
FIGURE 3. Mean untransformed absolute angular error shown separately by condition. Higher values correspond to greater error (i.e., worse performance). Error bars represent +/−1 SEM. Asterisks represent significant differences using repeated contrasts. Note that not all possible pairs were tested, see Section 3.1 for details.
Analyses using non-parametric tests on the untransformed data and parametric tests on the log-transformed data led to similar conclusions. For example, comparisons of performance between pairs of VEs led to identical conclusions when conducted using Wilcoxon signed-rank tests with untransformed data and t-tests with log-transformed data. Only the results of parametric tests on log-transformed data are reported here. Unless stated otherwise in the analyses below, all analysis of variance (ANOVA) assumptions were tested and confirmed.
Absolute angular error was analyzed in a 2 (sex) by 2 (interface) by 5 (VE) mixed ANOVA. Primary findings from this analysis and from follow-up tests are summarized in Table 1. The effect of order was not significant and is therefore excluded from the reported analyses. Mauchly’s test of sphericity was violated for the VE variable, so the analysis proceeded using a Greenhouse-Geisser correction for comparisons involving VE. The main effect of interface was significant, F(1,36) = 27.642, p < 0.001,
TABLE 1. Summary of key findings from the statistical analyses. For repeated contrast results, OF, open field; DO, drop-off; F, fence; T, texture; and C, classroom.
Repeated contrasts comparing pairs of VEs were conducted separately for the two interfaces, in light of the significant interaction between interface and VE. When using the discordant interface, errors in the open field VE were significantly higher than those in the drop-off VE, which produced the next-highest errors, F(1,37) = 4.339, p = 0.044,
When using the partially concordant interface, repeated contrasts showed that errors in the open field VE did not differ from those in the drop-off VE, which did not differ from the fence VE, which did not differ from the texture VE, ps >0.114. Errors in the classroom VE were significantly smaller than those in the texture VE, F(1,37) = 17.240, p < 0.001,
Finally, errors were larger when using the discordant teleporting interface compared to the partially concordant interface in the open field, drop-off, fence, and texture VEs, ps <0.003, but did not significantly differ in the classroom VE, t(37) = 1.767, p = 0.085.
Absolute axial error was defined as the absolute value of the difference between the response distance and the distance to the green post (i.e., to the path origin), divided by the distance to the green post. This variable captures the accuracy of the distance component of the participant’s response, irrespective of the angular component, and is scaled relative to the correct distance. Larger values represent greater axial error.
Absolute axial errors were not normally distributed, so a log transformation was used to remove skewness from the data (Osborne and Costello, 2008).2 The result was a more normal distribution with minimal skewness and more similar variances across conditions. Figure 4 shows average untransformed absolute axial error. Plots showing log-transformed data are available on the Open Science Framework (https://osf.io/ckua3/). All participants were within three standard deviations of the log-transformed mean error, so all were included in the analyses.
FIGURE 4. Mean untransformed absolute axial error shown separately by condition. Higher values correspond to greater error (i.e., worse performance). Error bars represent +/−1 SEM. Asterisks represent significant differences using repeated contrasts. Note that not all possible pairs were tested, see Section 3.2 for details.
Absolute axial error was analyzed in a 2 (sex) by 2 (interface) by 5 (VE) mixed ANOVA. Primary findings from this analysis and from follow-up tests are summarized in Table 1. The effect of order was not significant and is therefore excluded from the reported analyses. Mauchly’s test of sphericity was violated for the VE variable, so the analysis proceeded using a Greenhouse-Geisser correction for comparisons involving VE. The main effect of interface was significant, F(1,36) = 74.286, p < 0.001,
Repeated contrasts comparing pairs of VEs were conducted separately for the two interfaces, in light of the significant interaction between interface and VE. When using the discordant interface, errors in the open field VE were significantly larger than those in the drop-off VE, which produced the next-highest errors, F(1,37) = 10.815, p = 0.002,
When using the partially concordant interface, repeated contrasts showed that errors in the open field VE were worse than those in the drop-off VE, F(1,37) = 7.076, p = 0.011,
Finally, errors were larger when using the discordant teleporting interface compared to the partially concordant interface in the open field, drop-off, fence, and texture VEs, ps <0.001, but did not significantly differ in the classroom VE, t(37) = 0.955, p = 0.346.
Considering the collective results from the angular and axial errors reported above, the effect of VE on triangle completion performance was mostly similar across the two teleporting interfaces, with some small but important deviations. Across both teleporting interfaces, the open field VE generally led to the worst triangle completion performance, the classroom VE generally led to the best triangle completion performance, and the drop-off, fence, and texture VEs fell in between the open field and the classroom. One exception was the absolute angular error measure when using the partially concordant teleporting interface, where the open field was no worse than the single-boundary VEs (i.e., drop-off, fence, and texture VEs), although the numerical trend was consistent with predictions. Angular errors were generally smaller when using the partially concordant interface, and so participants apparently benefited less from the direction cues provided by the environmental boundaries. The finding that the open field and classroom VEs generally led to the worst and best performance, respectively, is not especially surprising. The open field possessed no orientation cues (boundaries or landmarks) and the classroom contained more orientation cues than any of the other VEs. Although past research has shown that the classroom VE leads to better performance than the open field VE (Cherep et al., 2020, 2021), the current study adds new data comparing boundaries defined by a fence, drop-off, and texture.
Triangle completion performance in the drop-off, fence, and texture VEs, all of which contained a single square boundary, generally fell in between the open field and classroom VEs. When using the partially concordant teleporting interface, the drop-off, fence, and texture VEs all produced comparable performance. However, when using the discordant teleporting interface, the drop-off VE was worse than the fence and texture VEs. This is surprising for a couple of reasons. First, the drop-off VE is quite similar to the texture VE: both are defined by a wood ground texture within the square boundary and grass ground texture beyond. The key difference is that the grass and wood textures are on the same plane in the texture VE, whereas the grass texture lies below the level of the wood texture in the drop-off VE. The drop-off VE therefore provides an additional cue defining the square boundary, which furthermore represents a navigational impediment. Given that the drop-off VE contains an extra boundary cue compared to the texture VE, it is unclear why the drop-off VE led to worse performance than the fence VE and the texture VE when using the discordant teleporting interface.
The texture VE led to performance that was no worse than and occasionally better than performance in the fence VE and the drop-off VE. This provides clear evidence that texture-defined boundaries can be as effective as boundaries that impede navigation. This finding echoes other human behavioral research reporting that boundaries do not need to impede movement in order to be useful cues for spatial memory (Negen et al., 2020). The current findings are also in alignment with animal neuroscience research showing that boundary vector cells in the rodent brain respond to texture boundaries as well as walls and drop-offs (Lever et al., 2009; Stewart et al., 2014; Wang et al., 2020), and preliminary research that humans possess analogous neural representations (Lee et al., 2018; Shine et al., 2019). On the other hand, human neuroscience research indicates that boundaries defined by textures and those defined by walls are processed in distinct regions of the brain in the service of scene recognition (Julian et al., 2016). Whether the current findings conflict with this research would require a model explaining how scene recognition informs navigation.
The size of the VEs and the triangular paths were relatively small, which may have impacted the availability of visual cues during travel. For example, participants had to keep their head tilted downward in order to fixate the posts forming the triangle, which may have made visual cues on the ground plane (e.g, intersections between the boundary and the ground plane) more prominent in the visual field. Future research using larger VEs and larger paths should evaluate whether the current results generalize across scale.
On average, men performed the triangle completion task with lower error than did women. A larger study by Cherep et al. (2021) reported similar results when participants performed triangle completion in VR using the same two teleporting interfaces, although sex differences did not occur when participants physically walked the outbound path. That study also found sex differences in spatial measures such as mental rotation and perspective taking, as well as sex differences in video game hours. Ultimately, the authors concluded that perspective taking ability was most predictive of individual differences in performance. Spatial skills are known to be malleable (Baenninger and Newcombe, 1989; Voyer et al., 2000; Uttal et al., 2013; Jirout and Newcombe, 2015; Lauer et al., 2019), so it is possible that differences in spatial experiences underlie the sex difference reported here. However, the current study did not assess spatial ability, spatial experience, or video game experience. Future studies should include individual difference measures such as these.
Although the current study did not include conditions to evaluate the necessity of boundaries, past research (Cherep et al., 2020) found that landmarks alone were insufficient to stay oriented when teleporting. In that study, performance in a VE with landmarks only was no better than an open field devoid of cues. Yet, the landmarks were beneficial when presented in the context of a boundary: triangle completion performance was better in a VE with landmarks positioned beyond the boundary of a circular fence, compared to a VE containing only the circular fence without landmarks.
Past research indicates that VE designers should include spatial boundaries to facilitate navigation and avoid disorientation associated with some locomotion interfaces (Cherep et al., 2020). Regarding the type of spatial boundary to include, the current findings indicate that a drop-off, a fence, and a texture boundary are all similarly valuable. Although the drop-off boundary was somewhat less effective than the fence and texture boundaries, the drop-off was still effective and differences among the boundary types were relatively small. The classroom VE, which contained boundaries as well as numerous landmarks, led to the best performance. The classroom VE also eliminated the difference between locomotion interfaces, indicating that sufficient visual information can overcome the disorienting effects of VR.
Pre-registration, complete data, videos, and supplemental figures are available on the Open Science Framework: https://osf.io/ckua3/. Some of this research was previously reported at the 2021 Symposium on Spatial User Interaction (Kelly et al., 2021).
The datasets presented in this study can be found in online repositories. The names of the repository/repositories and accession number(s) can be found below: https://osf.io/ckua3/.
The studies involving human participants were reviewed and approved by the Iowa State University Institutional Review Board. The patients/participants provided their written informed consent to participate in this study.
The research was conceptualized by JK, TD, LC, and SG. JK supervised data collection and analyzed the data. JK drafted the paper. TD, LC, and SG provided feedback and revisions on the paper.
This material is based upon work supported by the National Science Foundation under Grant Number CHS-1816029. The open access publication fees for this article were covered by the Iowa State University Library.
The authors declare that the research was conducted in the absence of any commercial or financial relationships that could be construed as a potential conflict of interest.
All claims expressed in this article are solely those of the authors and do not necessarily represent those of their affiliated organizations, or those of the publisher, the editors and the reviewers. Any product that may be evaluated in this article, or claim that may be made by its manufacturer, is not guaranteed or endorsed by the publisher.
Thanks to Moriah Zimmerman for assistance with creating the virtual environments, to Jason Terrill, Nicole Powell, and Owen Perrin for assistance with data collection, and to Melynda Hoover for helpful discussion and technical assistance.
1A constant value of 1.5 was added to all absolute angular error values prior to the log transformation (Ekwaru and Veugelers, 2018), as this was found to minimize skewness.
2A constant value of 0.02 was added to all absolute axial error values prior to the log transformation (Ekwaru and Veugelers, 2018), as this was found to minimize skewness.
Baenninger, M., and Newcombe, N. (1989). The Role of Experience in Spatial Test Performance: A Meta-Analysis. Sex. Roles 20, 327–344. doi:10.1007/BF00287729
Barry, C., and Burgess, N. (2014). Neural Mechanisms of Self-Location. Curr. Biol. 24, R330–R339. doi:10.1016/j.cub.2014.02.049
Bozgeyikli, E., Raij, A., Katkoori, S., and Dubey, R. (2016). “Point and Teleport Locomotion Technique for Virtual Reality,” in Proc. Annual Symposium on Computer-Human Interaction in Play (New York: ACM), 205–216. doi:10.1145/2967934.2968105
Chen, X., He, Q., Kelly, J. W., Fiete, I. R., and McNamara, T. P. (2015). Bias in Human Path Integration Is Predicted by Properties of Grid Cells. Curr. Biol. 25, 1771–1776. doi:10.1016/j.cub.2015.05.031
Cheng, K. (2008). Whither Geometry? Troubles of the Geometric Module. Trends Cognitive Sci. 12, 355–361. doi:10.1016/j.tics.2008.06.004
Cherep, L. A., Lim, A. F., Kelly, J. W., Acharya, D., Velasco, A., Bustamante, E., et al. (2020). Spatial Cognitive Implications of Teleporting through Virtual Environments. J. Exp. Psychol. Appl. 26, 480–492. doi:10.1037/xap0000263
Cherep, L., Kelly, J., Miller, A. J., Lim, A., and Gilbert, S. B. (2021). Individual Differences in Teleporting through Virtual Environments. J. Exp. Psychol. Appl. doi:10.31234/osf.io/b6cyd
Christou, C. G., and Aristidou, P. (2017). Steering versus Teleport Locomotion for Head Mounted Displays. In Augmented Reality, Virtual Reality, and Computer Graphics. Lecture Notes in Computer Science, eds. L. D. Paolis, P. Bourdot, and A. Mongelli (London: Springer-Verlag), 10325, 431–446. doi:10.1007/978-3-319-60928-7_37
Di Luca, M., Seifi, H., Egan, S., and Gonzalez-Franco, M. (2021). Locomotion Vault: The Extra Mile in Analyzing VR Locomotion Techniques. New York, NY, USA: Association for Computing Machinery.
Doeller, C. F., and Burgess, N. (2008). Distinct Error-Correcting and Incidental Learning of Location Relative to Landmarks and Boundaries. Proc. Natl. Acad. Sci. 105, 5909–5914. doi:10.1073/pnas.0711433105
Ekwaru, J. P., and Veugelers, P. J. (2018). The Overlooked Importance of Constants Added in Log Transformation of Independent Variables with Zero Values: A Proposed Approach for Determining an Optimal Constant. Stat. Biopharm. Res. 10, 26–29. doi:10.1080/19466315.2017.1369900
Hartley, T., Trinkler, I., and Burgess, N. (2004). Geometric Determinants of Human Spatial Memory. Cognition 94, 39–75. doi:10.1016/j.cognition.2003.12.001
Jirout, J. J., and Newcombe, N. S. (2015). Building Blocks for Developing Spatial Skills: Evidence from a Large, Representative u.S. Sample. Psychol. Sci. 26, 302–310. doi:10.1177/0956797614563338
Julian, J. B., Ryan, J., Hamilton, R. H., and Epstein, R. A. (2016). The Occipital Place Area Is Causally Involved in Representing Environmental Boundaries during Navigation. Curr. Biol. 26, 1104–1109. doi:10.1016/j.cub.2016.02.066
Kelly, J. W., McNamara, T. P., Bodenheimer, B., Carr, T. H., and Rieser, J. J. (2009). The Shape of Human Navigation: How Environmental Geometry Is Used in the Maintenance of Spatial Orientation. Cognition 109, 281–286. doi:10.1016/j.cognition.2008.09.001
Kelly, J. W., Ostrander, A. G., Lim, A. F., Cherep, L. A., and Gilbert, S. B. (2020). Teleporting through Virtual Environments: Effects of Path Scale and Environment Scale on Spatial Updating. IEEE Trans. Vis. Comput. Graph. 26, 1841–1850. doi:10.1109/TVCG.2020.2973051
Kelly, J. W., Terrill, J., Zimmerman, M., Doty, T. A., Cherep, L. A., Hoover, M. T., et al. (2021). “Boundaries Facilitate Spatial Orientation in Virtual Environments,” in Symposium on Spatial User Interaction (New York, NY, USA: Association for Computing Machinery). SUI ’21. doi:10.1145/3485279.3488284
Langbehn, E., Lubos, P., and Steincke, F. (2018). “Evaluation of Locomotion Techniques for Roomscale Vr: Joystick, Teleportation, and Redirected Walking,” in Proc. Virtual Reality International Conference (New York: ACM), 1–9. doi:10.1007/978-3-319-08234-9_253-1
Lauer, J. E., Yhang, E., and Lourenco, S. F. (2019). The Development of Gender Differences in Spatial Reasoning: A Meta-Analytic Review. Psychol. Bull. 145, 537–565. doi:10.1037/bul0000191
Lee, S. A., Miller, J. F., Watrous, A. J., Sperling, M. R., Sharan, A., Worrell, G. A., et al. (2018). Electrophysiological Signatures of Spatial Boundaries in the Human Subiculum. J. Neurosci. 38, 3265–3272. doi:10.1523/jneurosci.3216-17.2018
Lever, C., Burton, S., Jeewajee, A., O’Keefe, J., and Burgess, N. (2009). Boundary Vector Cells in the Subiculum of the Hippocampal Formation. J. Neurosci. 29, 9771–9777. doi:10.1523/JNEUROSCI.1319-09.2009
Lim, A. F., Kelly, J. W., Sepich, N. C., Cherep, L. A., Freed, G. C., and Gilbert, S. B. (2020). “Rotational Self-Motion Cues Improve Spatial Learning when Teleporting in Virtual Environments,” in Symposium on Spatial User Interaction (New York: ACM). SUI ’20. doi:10.1145/3385959.3418443
Moghadam, K. R., Banigan, C., and Ragan, E. D. (2018). Scene Transitions and Teleportation in Virtual Reality and the Implications for Spatial Awareness and Sickness. IEEE Trans. Vis. Comput. Graph. 26, 2273–2287. doi:10.1109/TVCG.2018.2884468
Negen, J., Sandri, A., Lee, S. A., and Nardini, M. (2020). Boundaries in Spatial Cognition: Looking like a Boundary Is More Important Than Being a Boundary. J. Exp. Psychol. Learn. Mem. Cogn. 46, 1007–1021. doi:10.1037/xlm0000760
Osborne, J. W., and Costello, A. (2008). Best Practices in Exploratory Factor Analysis BT - Best Practices in Quantitative Methods. Best Pract. Quant. Methods: Thousand Oaks, CA.
Shine, J. P., Valdés-Herrera, J. P., Tempelmann, C., and Wolbers, T. (2019). Evidence for Allocentric Boundary and Goal Direction Information in the Human Entorhinal Cortex and Subiculum. Nat. Commun. 10, 4004. doi:10.1038/s41467-019-11802-9
Stewart, S., Jeewajee, A., Wills, T. J., Burgess, N., and Lever, C. (2014). Boundary Coding in the Rat Subiculum. Philos. Trans. R. Soc. B Biol. Sci. 369, 20120514. doi:10.1098/rstb.2012.0514
Twyman, A. D., and Newcombe, N. S. (2010). Five Reasons to Doubt the Existence of a Geometric Module. Cogn. Sci. 34, 1315–1356. doi:10.1111/j.1551-6709.2009.01081.x
Uttal, D. H., Meadow, N. G., Tipton, E., Hand, L. L., Alden, A. R., Warren, C., et al. (2013). The Malleability of Spatial Skills: A Meta-Analysis of Training Studies. Psychol. Bull. 139, 352–402. doi:10.1037/a0028446
Voyer, D., Nolan, C., and Voyer, S. (2000). The Relation between Experience and Spatial Performance in Men and Women. Sex. Roles 43, 891–915. doi:10.1023/A:1011041006679
Wang, C.-H., Monaco, J. D., and Knierim, J. J. (2020). Hippocampal Place Cells Encode Local Surface-Texture Boundaries. Curr. Biol. 30, 1397–1409.e7. doi:10.1016/j.cub.2020.01.083
Weissker, T., Kunert, A., Fröhlich, B., and Kulik, A. (2018). “Spatial Updating and Simulator Sickness during Steering and Jumping in Immersive Virtual Environments,” in Proc. IEEE Conference on Virtual Reality and 3d User Interfaces (VR) (Washington, D.C.: IEEE), 97–104. doi:10.1109/VR.2018.8446620
Keywords: locomotion interfaces, teleporting, virtual reality, spatial updating, navigation, boundaries, virtual environments
Citation: Kelly JW, Doty TA, Cherep LA and Gilbert SB (2022) Boundaries Reduce Disorientation in Virtual Reality. Front. Virtual Real. 3:882526. doi: 10.3389/frvir.2022.882526
Received: 23 February 2022; Accepted: 27 May 2022;
Published: 16 June 2022.
Edited by:
Regis Kopper, University of North Carolina at Greensboro, United StatesReviewed by:
Hiroshi Ashida, Kyoto University, JapanCopyright © 2022 Kelly, Doty, Cherep and Gilbert. This is an open-access article distributed under the terms of the Creative Commons Attribution License (CC BY). The use, distribution or reproduction in other forums is permitted, provided the original author(s) and the copyright owner(s) are credited and that the original publication in this journal is cited, in accordance with accepted academic practice. No use, distribution or reproduction is permitted which does not comply with these terms.
*Correspondence: Jonathan W. Kelly, am9ua2VsbHlAaWFzdGF0ZS5lZHU=
Disclaimer: All claims expressed in this article are solely those of the authors and do not necessarily represent those of their affiliated organizations, or those of the publisher, the editors and the reviewers. Any product that may be evaluated in this article or claim that may be made by its manufacturer is not guaranteed or endorsed by the publisher.
Research integrity at Frontiers
Learn more about the work of our research integrity team to safeguard the quality of each article we publish.