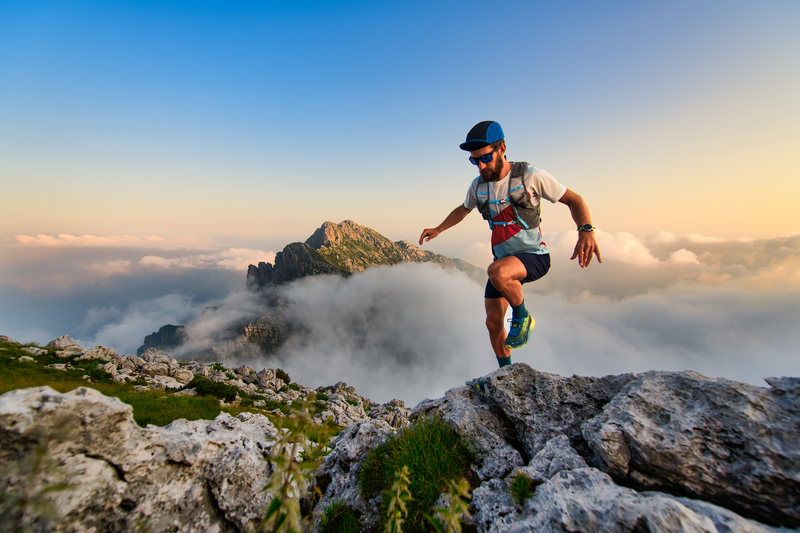
94% of researchers rate our articles as excellent or good
Learn more about the work of our research integrity team to safeguard the quality of each article we publish.
Find out more
ORIGINAL RESEARCH article
Front. Virtual Real. , 16 May 2022
Sec. Virtual Reality in Medicine
Volume 3 - 2022 | https://doi.org/10.3389/frvir.2022.864676
This article is part of the Research Topic Immersive Technologies in Healthcare View all 5 articles
Introduction: Virtual humans have expanded the training opportunities available to healthcare learners. Particularly, virtual humans have allowed simulation to display visual cues that were not previously possible in other forms of healthcare training. However, the effect of virtual human fidelity on the perception of visual cues is unclear. Therefore, we explore the effect of virtual human rendering style on the perceptions of visual cues in a healthcare context.
Methods: To explore the effect of rendering style on visual cues, we created a virtual human interface that allows users to interact with virtual humans that feature different rendering styles. We performed a mixed design user study that had undergraduate healthcare students (n = 107) interact with a virtual patient. The interaction featured a patient experiencing an allergic reaction and required trainees to identify visual cues (patient symptoms). The rendering styles explored include a 3D modeled virtual human and an AI generated photorealistic virtual human. Visual cues were applied using a Snapchat Lens.
Results: When users are given a frame of reference (users could directly compare symptoms on both rendering styles), they rated the realism and severity of the photorealistic virtual human’s symptoms significantly higher than the realism of the 3D virtual human’s symptoms. However, we were unable to find significant differences in symptom realism and severity ratings when users were not given a frame of reference (users only interacted with one style of virtual humans). Additionally, we were unable to find significant differences in user interpersonal communication behaviors between the 3D and photorealistic rendering styles.
Conclusion: Our findings suggest 1) higher fidelity rendering styles may be preferred if the learning objectives of a simulation require observing subtle visual cues on virtual humans and 2) the realism of virtual human rendering style does not necessarily affect participants’ interpersonal communication behaviors (time spent, questions asked).
Virtual humans are an important aspect of virtual reality. Virtual humans are often utilized in virtual reality applications as either a user’s avatar or as characters a user can interact with Fox et al. (2015). Virtual humans have been used in educational virtual environments to train the observational and critical thinking skills of target populations such as those in the military, construction, and healthcare fields (Cendan and Lok, 2012; Hays et al., 2012; White et al., 2015; Eiris et al., 2021). It is critical that when training these populations we provide training observations with levels of fidelity that create appropriate perceptions of realism (Watts et al., 2021). Otherwise, training may be ineffective or even counterproductive (Watts et al., 2021).
Visual cue identification is essential for many forms of training ranging from symptom identification to aviation (Pausch et al., 1992; Huber and Epp, 2021). Cues deliver information to observers by providing some form of verbal or written messaging, or by providing visual artifacts to observers (Xu and Liao, 2020). For example, Kotranza et al. used visual cues such as a sagging face and drifting eyes to help train users to identify cranial nerve symptoms (Kotranza et al., 2010). Observers apply critical thinking to interpret the information provided by cues (Xu and Liao, 2020). Cues can lead to observers applying heuristics that affect decision making (Sundar, 2008). Therefore, understanding how virtual human rendering style may affect user perceptions of visual cues on virtual humans is critical to training appropriate decision making in virtual human simulation (Sundar, 2008; Vilaro et al., 2020).
When exploring virtual human rendering styles, previous research has focused on how realism impacts user perception of virtual humans. This study explores how rendering style fidelity affects the perceived realism and severity of visual cues that will appear on a virtual human in a healthcare context. We also report how rendering style fidelity affects participant interpersonal communication behaviors such as the time spent interacting with a patient and the number of questions they ask. Our approach to examining the effect of virtual human rendering style on visual cues involves using allergic reaction symptoms as visual cues that learners must identify in healthcare training.
To continue building on this previous work, we address the following research questions:
• RQ 1) How does virtual human rendering style affect user perceptions of visual cues on a virtual human?
• RQ 2) How does virtual human rendering style affect interpersonal communication behaviors?
To answer these research questions, we created a virtual human interface that allows users to interact with virtual humans of different rendering styles. We performed a mixed design user study that had undergraduate healthcare students (n = 107) interview a virtual patient. During the patient interview, the virtual patient developed an allergic reaction to the blood transfusion they were receiving. The rendering styles explored include a 3D modeled virtual human and a photorealistic virtual human.
Our findings suggest that higher fidelity rendering styles may be preferred if the learning objectives of a simulation require observing subtle visual cues on virtual humans. We also found that the realism of virtual human rendering style does not necessarily affect participants’ interpersonal communication behaviors (time spent, questions asked). This work contributes to the field of virtual human design, by reflecting on the role of fidelity in simulations intended to train the identification of visual cue.
In this section, we discuss how previous advances in virtual human technology have expanded training possibilities, and previous findings on the impact of virtual human rendering style’s on user perceptions of a virtual human.
Virtual humans offer training opportunities that were not previously possible for other forms of healthcare training. These new training opportunities include the depiction of visual cues (e.g., symptoms and other physiological changes) that real actors used for patient interviews (standardized patients) cannot display (Kotranza and Lok, 2008; Deladisma et al., 2009; Kotranza et al., 2009; Kotranza et al., 2010; Cordar et al., 2017; Daher et al., 2018; Daher et al., 2020).
The work of (Deladisma et al., 2009; Kotranza et al., 2010), Cordar et al. (2017), and (Daher et al., 2018; Daher et al., 2020), are examples of how virtual humans offer training opportunities that were not previously possible for other forms of healthcare training. Kotranza used virtual humans to display cranial nerve symptoms. Cranial nerve symptoms (e.g. sagging face, drifting eyes, etc.) are not symptoms that actors can perform in healthcare training interviews (Kotranza et al., 2010). Kotranza and Deladisma et al. used a virtual human, virtual reality, and a physical breast mannequin to allow medical students to practice interactive clinical breast examinations in a mixed reality setting (Kotranza and Lok, 2008; Deladisma et al., 2009). Prior to the use of virtual humans, healthcare learners did not have opportunities to practice handling breast complaints in an interactive training simulation. Cordar used virtual humans to provide team training opportunities using only virtual humans. Prior to this, team training would require the presence of multiple individuals to take place (Cordar et al., 2017). Daher et al. created the Physical-Virtual Patient Bed that uses a virtual human and a mannequin to provide interactive experiences that exhibit physical symptoms (e.g. changes to temperature, changes to skin appearance, etc.) that are not possible for actors to recreate in a healthcare training interview (Daher et al., 2018; Daher et al., 2020).
Unfortunately, these advantages have only been applicable to virtual humans and it is unclear how a virtual human’s rendering style may affect the perception of visual cues that virtual humans display (Lok, 2006; Kotranza and Lok, 2008; Deladisma et al., 2009; Kotranza et al., 2009; Daher et al., 2018; Daher et al., 2020). By exploring the perceived realism and severity of visual cues on virtual humans of different rendering styles, we hope to continue to expand and improve the training possibilities that virtual humans provide.
This study, which investigates how virtual human rendering style affects user perceptions of virtual human and virtual symptoms, is the first step towards understanding if these symptoms can be applied towards standardized patients. Currently, commercial virtual human healthcare training does not use photorealistic rendering styles (Kognito, 2022; Shadow Health, 2022). It is unclear how the rendering style of the virtual human affects user perceptions of realism and severity of physiological changes that occur in virtual humans during virtual human healthcare training. Previous works have explored how virtual human rendering style may affect user perceptions of a virtual human (McDonnell et al., 2012; Zibrek and McDonnell, 2014; Zell et al., 2015; Zibrek et al., 2019). However, these works often do not occur in applied settings. Instead, they use still images or short videos of virtual humans to determine if virtual human rendering style affects factors such as perceived agreeableness, friendliness, and trustworthiness (McDonnell et al., 2012; Zell et al., 2015).
For example, McDonnell et al. developed a virtual human with various rendering styles (McDonnell et al., 2012). McDonnell then had participants view still images and short videos of the differently rendered virtual humans. McDonnell found that cartoon style virtual humans were seen as more appealing and friendly than more realistic virtual humans. Using a similar methodology to McDonnell, Zell et al. found that the facial shape used in rendering styles affects perceptions of realism and expression intensity, that the materials used in rendering style affects the appeal of virtual humans, and that rendering style realism may be a poor predictor of user ratings of appeal, eeriness, and attractiveness (Zell et al., 2015).
Unlike McDonnell and Zell, Volonte et al. used a more applied approach when exploring the effects of rendering style. Volonte et al. investigated the effect of virtual human fidelity on interpersonal communication behaviors in a healthcare context in two previous works (Volonte et al., 2016, 2019). In the first work, Volonte had participants interact with a virtual human created using either a realistic, cartoon, or sketch-like rendering style. Volonte was unable to find significant differences between conditions for either time spent interacting with the virtual patient or the number of questions asked (Volonte et al., 2016). In the second work, Volonte again had participants interact with a virtual human created using either a realistic, cartoon, or sketch-like rendering style. However, in this work Volonte also manipulated the rendering style of the environment separately from the virtual human as well. Volonte found that the number of questions asked was significantly higher for the higher fidelity rendering of the virtual human and higher fidelity rendering of the environment (Volonte et al., 2019)). Further work is needed to determine if virtual human rendering style affects aspects in more applied settings such as interpersonal communication and the appearance of virtual symptoms.
We developed a web application that allows healthcare students to interact with virtual patients (See Figure 1). The web interface consisted of four components: the virtual human, a directions window that displays steps a user should follow during the patient interaction, a Google DialogFlow Messenger integration that enabled conversation with the virtual human, and a button that ends the interaction and returns the user to Qualtrics (see Figure 1) (Qualtrics, 2020; Google, 2021). Previous work has used Unity WebGL to display virtual humans (Zalake et al., 2019), our work uses premade videos of virtual humans. When a response is received from Google DialogFlow, the web application queries a cloud storage service to load the virtual human video that corresponds with the response. Using videos rather than WebGL allowed us to create higher fidelity patients with different rendering styles (see Section 3.3).
FIGURE 1. The interface used to interact with the virtual humans. The interface contains 1) the virtual human, 2) the directions window, 3) the Google DialogFlow Messenger integration that enables conversation with the virtual human, and 4) the button used to end the interaction.
We created 123 videos for each virtual human rendering style both with and without symptoms displayed. These videos each contained phrases that the virtual human could use to respond, as well as an idle video. When a virtual human was asked a question, the video would change to the correct phrase video and then upon its completion, the video player would switch back to playing the idle video. In addition to these videos, we also created a pre-brief video and a set of intermission videos. The pre-brief video informed students of the scenario they were to participate in and explained how to use the interface to interact with the virtual human. The intermission videos had the users answer multiple choice questions regarding blood transfusions (questions not used in this work). This intermission was used to act as a time buffer to simulate symptom development over time.
We created the virtual human with two different rendering styles: a 3D virtual human rendering style and a photorealistic rendering style, as shown in Figure 2. Users interacted with only one of the rendering styles during the study.
The AI generated photorealistic virtual human was created using Synthesia. io, a platform that uses AI driven systems to create videos with photorealistic virtual humans (Synthesia.io, 2021). The 3D virtual human was then created based on this photorealistic character.
To create the 3D virtual human condition, an image of the Synthesia character was used in a program called FaceBuilder (KeenTools, 2021). FaceBuilder is a Blender plug-in that generates 3D virtual human head objects and face textures based on uploaded images (Blender, Online Community, 2021). These generated heads were applied to bodies created using Adobe Fuse (Adobe, 2020). Outfits were chosen to best match the photorealistic characters outfits based on what was available in Adobe Fuse.
The voices were identical across rendering style conditions. Both virtual humans used the voice created using Synthesia’s text-to-speech software. To increase user perceptions of plausibility (Hofer et al., 2020), the background used for the virtual environment resembles a clinical environment. It should be noted that because of the different methods used to generate these virtual humans, the head movement animations used while speaking did differ. However, we did not find significant differences in the rated realism of the virtual human movement (See Section 4.3.2).
The virtual character designed represented a 50 year old white male. At this time, one race was chosen for participants to interact with. This is because allergic reaction may differ between individuals of different age, race, or gender (Brown, 2004; Robinson, 2007). Thus, one age, race, and gender was chosen to limit potential differences. However, we do recommend that future works explore if perception differences exist when manipulating age, race, and gender.
This study had healthcare students experience a virtual human having an allergic reaction to a blood transfusion. To apply this reaction to the virtual humans, an augmented reality filter was made using Lens Studio (Snap Inc, 2021). This filter was reviewed by nursing collaborators for accuracy. This filter was then applied to the videos of the photorealistic virtual human. As for the 3D character, a new face texture was created using the FaceBuilder software using images from the photorealistic virtual human with the filter applied. Then, we created the 3D character videos with the new face texture containing the symptoms (See Figure 3).
The conversation users had with the virtual human was designed in collaboration with nursing educators. A case study that was used in previous semesters of the health assessment course was adapted for this interaction. The conversation was open-ended and allowed users to ask the patient any question at any time. However, the interface also provided participants directions that included typical nursing protocols they needed to complete to progress in the interview. This included having the participant introduce themselves to the patient, identify the patient, ask the patient why they are in the clinic, and then asking the patient if they have any questions. The patient was designed to ask questions regarding why they needed a blood transfusion and the safety of receiving a blood transfusion. Participants are expected to be able to answer by providing the virtual human with the safety protocols used to keep blood secure for blood transfusions. Once, the patient begins to have a reaction to the blood transfusion, the participant must respond to the virtual human’s concerns and then provide the patient with a course of action that they will take (e.g. the participant says they will stop the transfusion, or the participant says they will provide medication). Once a course of action has been given, participants were free to ask about other symptoms the virtual human may be having or to end the interaction.
Using a mixed design user study, we explored the effect of virtual human rendering style on user perceptions of visual cues. The user study had participants interact with a virtual patient. The interaction featured a patient experiencing an allergic reaction and required trainees to identify symptoms’ visual cues. In the following sections we will describe the participants, study procedure, and measures used.
Nursing students (n = 107) were recruited from a nursing course taught at the University of Florida. 79 students were between 18 and 24 years old, 20 were 25–34, six were 35–44, 1 was 45–54 years old, and 1 was 55–64 years old. Students self-reported genders were: 14 Male, 90 Female, and 3 chose not to identify. All students completed the interaction in a classroom setting. Students used their own laptops and headphones to complete the interaction.
Participants completed the virtual human interaction as a part of an ungraded course lab exercise (See Figure 4 to view procedure). Participants were split into two groups that interacted with different rendering style conditions: 53 interacted with the photorealistic virtual human and 54 interacted with the 3D virtual human. The study was distributed via a link in the Canvas learning management system used by the course. The link led to a Qualtrics survey to begin the study (Qualtrics, 2020). Users began the study by completing the informed consent and a pre-survey. The users were then asked to watch a pre-brief video. The pre-brief video informed students of the scenario they were to participate in and explained how to use the interface to interact with the virtual human. Following the pre-brief video, the nursing students interacted with a virtual patient that is scheduled to receive a blood transfusion. The interaction is divided into two parts. In the first part, students were asked to educate the virtual patient by answering the patient’s questions regarding their visit. In the second part, the virtual patient begins to exhibit symptoms and students must respond to the virtual human’s adverse reaction to the blood transfusion. The two parts of the interaction are separated by the approximately 5 min intermission. Students interacted with the virtual human by typing their questions into a text box provided by the Google DialogFlow Messenger integration. Once the participants completed the interaction with the virtual human, they clicked on the “End Interview” button which returned them to the Qualtrics survey. The study concluded with a post survey (See Section 3.9).
The measures used in this study focus on users’ past experience with virtual human simulations, user confidence, virtual human realism, virtual human symptom realism, and virtual human symptom severity. As previous work (Wang et al., 2019) described, we use visual analogue scales (VAS) (Flynn et al., 2004) to measure qualities of the user and the virtual human (See Table 1). All questions measured using VAS use ratio/scale data from 0–100 with one significant digit; open-ended questions are analyzed qualitatively.
A pre-study demographics survey gathered data on user age, gender, past experience with virtual human simulations, and user confidence in managing a blood transfusion. Questions 1 and 2 were asked in the pre-survey in addition to demographics questions (See Table 1).
During the interaction, we recorded the time a participant spent interacting with the virtual human and the participant’s conversation transcript with the virtual human was recorded.
The post-study survey included measures regarding the participants’ perceptions of the virtual human and its symptoms. The post-survey was divided into two parts. The first part of the post-survey focused on user perceptions directly after the interaction when they had only seen one virtual human rendering style. The second part of the post-survey focused on user perceptions when they could directly compare symptoms on both rendering styles. We refer to the first part of the post-survey as user perceptions with no frame of reference, and we refer to the second part of the post-survey as user perceptions with a frame of reference.
This method was used to investigate the effect of rendering style on the perception of visual cues because it was known that the participants had previous knowledge of virtual humans training simulations (based on previous curriculum). Based on previous work, we believed that participants might respond to the initial survey (Part 1) based on their expectations and knowledge of past virtual human experiences, rather than on their true perceptions of the current virtual human Rademacher (2003). Therefore, we provided an opportunity for participants to directly compare virtual human rendering styles and provide feedback on how the rendering style affected their perceptions in part 2 of the post survey.
Part 1: Users were asked to respond to questions three to nine directly after interacting with the virtual human. This allowed us to gather user perceptions when they have no frame of reference outside of past experiences to compare virtual human rendering styles (See Table 1).
Part 2: After completing part 1 of the post-survey, users were provided two images side by side. One image depicted the virtual human experiencing symptoms in the rendering style they interacted with, and the other image depicted the virtual human experiencing symptoms in the rendering style they did not interact with (See Figure 3). Users were then asked to respond to questions 10–15 (See Table 1). By providing static images, we were able to gather user perceptions when they have a frame of reference to compare virtual human render styles. Static images were chosen over the use of videos to help the authors to control for differences in animation style of the rendering styles. By using static images, animations/movements that users experienced during the interaction could be assumed to be similar for both virtual humans.
Participant average rated experience with training simulation experiences was 66.51/100 with a standard deviation of 20.09 (0 being no experience and 100 being extensive experience). This higher experience level is expected as virtual human training simulations were a part of the students’ course curriculum. Additionally, for Question 1, we tested to see if there was a difference in experience ratings between groups. Normality and variance checks were conducted. The data distribution in both the conditions was normally distributed, and a Levene’s test indicated equal variances (p = 0.079). As expected, we could not find significant differences in experience levels between groups. Since we could not find differences between group experience levels, we continued with the rest of the data analysis.
For each rendering style, we used a Wilcoxon’s signed-rank test to compare users’ confidence in managing a blood transfusion reaction before and after the interaction. Confidence increased significantly for both the photorealistic condition (Mdn1 = 34.90, Mdn2 = 51.00, W = 118, p
In this section, the goal of this analysis was to understand if users perceived differences in virtual human realism when only one virtual human rendering style has been seen. It is important to explore whether there are differences when only seeing one virtual human because users may not have the frame of reference or virtual human experience to apply accurate ratings of realism.
For Question 3, variance and normality checks were conducted. A Levene’s test indicated equal variances (p = 0.059). The distribution of the data in the 3D condition was normally distributed. However, the data for the photorealistic condition was not normally distributed. Therefore, we used a Mann-Whitney U test to compare the two groups. The Mann-Whitney U test showed that there was a significant difference (U = 926.5, p = 0.002) between the photorealistic condition compared to 3D condition. The realism of the photorealistic rendering style was rated significantly higher than the realism of the 3D rendering style. The median appearance realism rating was 78.3 for the photorealistic group compared to 63.8 for those in the 3D condition suggesting that the appearance of the photorealistic virtual human is seen as more realistic by participants.
For Question 4, variance and normality checks were conducted. A Levene’s test indicated equal variances (p = 0.071). The distribution of the data in both the conditions was normally distributed. Therefore, we used an independent sample T-test to compare the two groups. There was no significant effect for rendering style on the rated realism of the virtual human movement, t (105) = −1.17, p = 0.241, despite the photorealistic condition (M = 61.39, SD = 20.34) attaining higher scores than the 3D condition (M = 56.93, SD = 18.73).
83 students provided a response to Q6. 36 (3D = 20, Photorealistic = 16) stated nothing was distracting. 15 (3D = 4, Photorealistic = 11) noted the virtual human movement was distracting with the most mentioned feature being the looping of the movements (3D = 2, Photorealistic = 11). 11 (3D = 8, Photorealistic = 13) students noted the virtual human either ignored their questions or had strange responses. 6 (3D = 3, Photorealistic = 3) students noted that the loading delay in the virtual human responses was distracting. 6 (3D = 3, Photorealistic = 3) noted the virtual humans voice or tone was distracting. 4 (3D = 2, Photorealistic = 2) students reported they saw glitchy/unusual visuals. Five students reported other miscellaneous responses.
The goal of this analysis was to understand if users perceived differences in virtual symptom realism when only viewing one virtual human rendering style. Similar to the above analysis, it is important to explore whether there are differences when only seeing one virtual human rendering style because users may not have the frame of reference or virtual human experience to apply accurate ratings of realism. In this between subjects analysis our variable was 3D vs. AI-generated and the dependent measures were users’ self-reported perceptions of symptom realism (See Questions six and 7).
For Question 7, variance and normality checks were conducted. A Levene’s test indicated equal variances (p = 0.103). The distribution of the data in both the conditions was normally distributed. Therefore, we used an independent sample T-test to compare the two groups. There was no significant effect for rendering style on the rated severity of the virtual human’s symptoms, t (105) = 0.69, p = 0.492, despite the photorealistic condition (M = 53.15, SD = 20.73) attaining lower scores than the 3D condition (M = 55.69, SD = 17.13).
For Question 8, variance and normality checks were conducted. A Levene’s test indicated equal variances (p = 0.085). The distribution of the data in both the conditions was normally distributed. Therefore, we used an independent sample T-test to compare the two groups. There was no significant effect for rendering style on the rated realism of the virtual human’s symptoms, t (105) = 0.50, p = 0.617, despite the photorealistic condition (M = 61.85, SD = 20.22) attaining lower scores than the 3D condition (M = 63.57, SD = 15.15).
For question 9, users were asked if the rendering style positively or negatively affected their ability to identify the virtual human’s symptoms. Variance and normality checks were conducted. A Levene’s test indicated equal variances (p = 0.112). The distribution of the data in both the conditions was normally distributed. Therefore, we used an independent sample T-test to compare the two groups. There was no significant effect for rendering style on participants’ abilities to identify the virtual human’s symptoms, t (105) = −1.14, p = 0.258, despite the photorealistic condition (M = 64.17, SD = 21.17) attaining higher scores than the 3D condition (M = 60.00, SD = 16.49).
82 students provided a follow-up response to this rating in Q10. Without having a frame of reference, many students seemed to struggle to answer how the rendering style affected their ability to identify the virtual human’s symptoms. 39 students (3D = 24, Photorealistic = 15) responded with answers that simply acknowledge that they could see the symptoms with no reference to rendering style and 8 (3D = 5, Photorealistic = 3) students stated they did not notice the symptoms. However, 13 (3D = 7, Photorealistic = 6) students stated the rendering style made no difference. Seven students in the photorealistic group noted that the realism helped them identify the symptoms at this point in the study. 15 (3D = 7, Photorealistic = 8) students provided miscellaneous comments.
To improve the depiction of virtual human symptoms in future simulations, users can directly compare the symptoms applied to different rendering styles. By allowing users to compare different rendering styles, we would be able to elicit user perceptions of depicted symptoms. This approach would allow us to understand which rendering style is better suited for virtual human medical simulations that use visual cues for depicting symptoms. Therefore, after completing the interaction and all other survey questions, users were asked to directly compare the symptoms of the two rendering styles.
In a within subjects comparison, the realism of the photorealistic virtual human’s symptoms were rated significantly higher than the realism of the 3D virtual human’s symptoms. A Wilcoxon’s signed-rank test showed that the photorealistic condition was rated significantly higher (Mdn = 92.40) compared to the 3D condition (Mdn = 35.10), W = 5515.50, p
In a within subjects test, the severity of the photorealistic virtual human’s symptoms was rated significantly higher than the severity of the 3D virtual human’s symptoms. A Wilcoxon’s signed-rank test showed that the photorealistic condition was rated significantly higher (Mdn = 64.40) compared to the 3D condition (Mdn = 51.30), W = 4682.00, p
66 students provided a follow-up response for Q15.33 of these students noted the rendering style affected their symptom identification. 26 students (3D = 13, Photorealistic = 13) responded with answers that stated the more realistic rendering style helped in identifying symptoms, 6 (3D = 1, Photorealistic = 5) students noted that less realistic rendering styles made it more difficult to identify symptoms, and 1 student in the photorealistic group stated it affected their identification but did not elaborate (See Table 2 for examples).
TABLE 2. Example User Responses to question 15, “How the virtual human’s rendering style (i.e. visual appearance) affected your perception of the realism and severity of the virtual human’s symptoms.”
The distribution of the data was normal but Levene’s test indicated unequal variances (p
The distribution of the data was not normally distributed and Levene’s test indicated unequal variances (p
When providing participants with a frame of reference, we found that the realism and severity of the photorealistic virtual human’s symptoms were rated significantly higher than the 3D virtual human’s symptoms. Additionally, several open-ended responses suggest that the photorealistic rendering style allowed participants to assess the virtual human and its symptoms with lowered difficulty. The main reason participants cited for the lowered difficulty was that the visual cues used to display the symptoms on the virtual humans are more easily identified as intentional when shown on the photorealistic virtual humans than 3D virtual humans (See Table 2).
These results provide evidence that increasing the fidelity of a virtual human may aid participants in identifying symptoms in visual cue training. However, further research is necessary as these results could be domain specific. Previous work has found that medical illustrations, which opt for more cartoonistic style representations, have been found to aid in understanding while limiting the introduction of distracting details (Houts et al., 2006; Krasnoryadtseva et al., 2020). Therefore, increasing fidelity may not be the only option for improving visual cue identification. Ring et al. stated that the optimal rendering style used for virtual humans varies based on task domain and user characteristics, indicating it may also be helpful to consider lowering the fidelity of a virtual human to be more similar to medical illustrations Ring et al. (2014). Using a medical illustration rendering style may allow educators to highlight relevant visual cues when initially teaching about them, and once the information is well-learned, then using a scaffolded approach, cues could be made more realistically subtle using the higher fidelity virtual humans used in this work. We suggest future work explores how the effects of placing filters differ when used on more traditional medical illustrations or manikins, as compared to real humans or photorealistic virtual humans.
Additionally, we do not wish to ignore that we could not find significant differences in rated realism or severity when participants rated visual realism and severity of the virtual human symptoms without a frame of reference. It is paramount to train users with realistic training opportunities and this may include being able to display subtle visual changes (redness, slight yellowing of the eyes, etc.) (Watts et al., 2021). However, trainees interacting in simulations will follow a fiction contract (Nanji et al., 2013). A fiction contract is an agreement between the trainee and those conducting the simulation regarding how the trainee should interact (Dieckmann et al., 2007). In the case of virtual humans with symptoms, a compelling fiction contract will lead trainees to see symptoms regardless of whether the symptoms are the most realistic or not. Therefore, comparisons where only 1 condition is shown to each group may not be the best option for understanding differences in user visual cue perception in simulation. Thus, we suggest, future works involving the perceptions of visual cues in simulation should be performed using within subjects designs to provide users with more context of how a visual cue would be depicted in the real world.
We found that without a frame of reference, participants rated the realism of the photorealistic rendering style significantly higher than the realism of the 3D rendering style. However, we were unable to find significant differences between the 3D rendering style and the photorealistic rendering style for the number of questions asked to the virtual human or the time spent with the virtual human. Additionally, there was no difference in the increase in participant confidence in managing a blood transfusion between the two conditions.
Overall, we believe that continuing to increase fidelity to photorealistic levels will not increase interpersonal communication behaviors such as time spent interacting or the number of questions asked. When comparing our work to that of Volonte et al. (See Section 2.2), our low fidelity character is comparable to the high fidelity character used in both works (Volonte et al., 2016; Volonte et al., 2019). If comparing our findings to Volonte in 2016, our work would provide further evidence that the realism of virtual human rendering style does not necessarily affect participants’ interpersonal communication behaviors such as time spent interacting and the number of questions asked. However, if compared to (Volonte, 2019) work, our work may indicate that increasing fidelity may lead to diminishing return over time or that there is a ceiling effect on the correlation between increasing rendering style and increasing the number of questions asked. Unfortunately, these scenarios are not directly comparable as they involve different scenarios, participant demographics, and levels of virtual human fidelity. Nonetheless, our findings in combination with previous works highlight an opportunity for future work to further explore the effect of rendering style on interpersonal communication behaviors.
To prevent ineffective or counterproductive visual cue training, previous research in simulation-based medical education has recommended that simulation designers should provide training observations with levels of fidelity that create appropriate perceptions of realism (Watts et al., 2021). Thus, this work explored the effect of virtual human rendering style on the perceptions of visual cues (patient symptoms) in a healthcare context.
In summary, we present our conclusions below in reference to the questions we raised in Section 1:
• RQ 1) How does virtual human rendering style affect user perceptions of visual cues on a virtual human?
Our results provide evidence that, when provided with a frame of reference, users view symptoms as more realistic and severe on photorealistic virtual humans compared to 3D virtual humans. From these findings, we suggest that simulation designers opt to use higher fidelity virtual human rendering styles when they are required to apply subtle visual cues to virtual humans. Based on user feedback, we believe this will help users to recognize subtle visual cues as intentionally occurring in a simulation.
• RQ 2) How does virtual human rendering style affect interpersonal communication behaviors?
Interpersonal communication behaviors (time spent interacting, number of questions asked) were not significantly different between rendering style conditions. Based on previous research in a similar context, there may be multiple reasons for this finding (see Section 5.2). Future work will be required to understand the effect of rendering style on interpersonal communication behaviors.
Ring et al. stated that the optimal rendering style used for virtual humans varies based on task domain and user characteristics Ring et al. (2014). This work highlights that the benefits provided by increased rendering style fidelity can be domain and task specific. In the task of identifying visual cues, this work shows that higher fidelity can provide users with noticeable benefits. However, this work also indicates that in the task of interacting with the patient, the users do not seem to change their behavior much in this domain. These findings may apply to virtual reality applications in the same domain, but researchers should consider how in virtual reality the virtual environment rendering style may also affect user interactions Volonte et al. (2020). Overall, this work encourages VR community members to research the optimal fidelity needed for specific tasks and domains. By doing so, the VR community may be able to better understand which fidelity levels should be used in new scenarios.
As previously mentioned, there are a few limitations to this work. First, the animation styles were not able to be controlled to be the same due to the different methods used to generate the virtual humans. We do not believe this affected user perceptions of symptoms as we were unable to find significant differences in virtual human movement realism. However, this finding could have been due to users basing their expectations on knowledge from past virtual human experiences such as previous course simulation exercises or other outside activities (see Section 4.1). Second, we only investigated two rendering styles. It is possible that other rendering styles not meant to simulate real life (e.g. cartoon style) may affect interpersonal communication behaviors and the perception of symptoms differently. Therefore, we suggest future work should further explore the effect of different rendering styles on these aspects. Third, students used their own laptops to complete the interaction. Differences in screen size, resolution, or brightness may have affected users’ ability to identify the virtual patient’s visual cues. This approach is more ”in the wild.” Since these variations occurred for both groups, we do not see this impacting our results. However, the variation should be noted, and future work may benefit by investigating the differences in perceptions using a more controlled screen environment. Fourth, the semi-guided approach may have affected the number of questions users asked. Users that strictly followed the interview directions may have prevented rendering style effects on the conversation from arising. Future work focusing on conversational effects of rendering style may wish to explore this area using an unguided approach. Finally, we did not explore how perceptions of virtual symptoms may change due to differences in virtual human race or gender. This was done because allergic reactions may differ between individuals of different age, race, or gender (Brown, 2004; Robinson, 2007). Thus, we limited the scope for this work. However, we do recommend that future works explore if perception differences exist when manipulating age, race, and gender.
The raw data supporting the conclusions of this article will be made available by the authors, without undue reservation.
The studies involving human participants were reviewed and approved by University of Florida Internal Review Board (IRB). The patients/participants provided their written informed consent to participate in this study.
JS conducted the user study, carried out the data analysis, and wrote the manuscript in consultation with all others. AS was the instructor for the courses in which virtual patient interviews were integrated. All authors contributed to the study design, virtual human design, visual cue design, and conceptualization of the paper.
This work was funded by the National Science Foundation award numbers 1800961 and 1800947.
The authors declare that the research was conducted in the absence of any commercial or financial relationships that could be construed as a potential conflict of interest.
All claims expressed in this article are solely those of the authors and do not necessarily represent those of their affiliated organizations, or those of the publisher, the editors and the reviewers. Any product that may be evaluated in this article, or claim that may be made by its manufacturer, is not guaranteed or endorsed by the publisher.
We would like to thank the members of the Virtual Experiences Research Group in their help in providing feedback.
The Supplementary Material for this article can be found online at: https://www.frontiersin.org/articles/10.3389/frvir.2022.864676/full#supplementary-material
Brown, S. G. A. (2004). Clinical Features and Severity Grading of Anaphylaxis. J. Allergy Clin. Immunol. 114, 371–376. doi:10.1016/j.jaci.2004.04.029
Cendan, J., and Lok, B. (2012). The Use of Virtual Patients in Medical School Curricula. Adv. Physiology Educ. 36, 48–53. doi:10.1152/advan.00054.2011
Cordar, A., Wendling, A., White, C., Lampotang, S., and Lok, B. (2017). “Repeat after Me: Using Mixed Reality Humans to Influence Best Communication Practices,” in 2017 IEEE Virtual Reality (VR) (IEEE), 148–156. doi:10.1109/VR.2017.7892242
Daher, S., Hochreiter, J., Norouzi, N., Gonzalez, L., Bruder, G., and Welch, G. (2018). “Physical-Virtual Agents for Healthcare Simulation,” in Proceedings of the 18th International Conference on Intelligent Virtual Agents. doi:10.1145/3267851.3267876
Daher, S., Hochreiter, J., Schubert, R., Gonzalez, L., Cendan, J., Anderson, M., et al. (2020). The Physical-Virtual Patient Simulator. Sim Healthc. 15, 115–121. doi:10.1097/SIH.0000000000000409
Deladisma, A. M., Gupta, M., Kotranza, A., Bittner, J. G., Imam, T., Swinson, D., et al. (2009). A Pilot Study to Integrate an Immersive Virtual Patient with a Breast Complaint and Breast Examination Simulator into a Surgery Clerkship. Am. J. Surg. 197, 102–106. doi:10.1016/j.amjsurg.2008.08.012
Dieckmann, P., Gaba, D., and Rall, M. (2007). Deepening the Theoretical Foundations of Patient Simulation as Social Practice. Simul. Healthc. J. Soc. Simul. Healthc. 2, 183–193. doi:10.1097/SIH.0b013e3180f637f5
Eiris, R., Wen, J., and Gheisari, M. (2021). iVisit - Practicing Problem-Solving in 360-degree Panoramic Site Visits Led by Virtual Humans. Automation Constr. 128, 103754. doi:10.1016/j.autcon.2021.103754
Flynn, D., van Schaik, P., and van Wersch, A. (2004). A Comparison of Multi-Item Likert and Visual Analogue Scales for the Assessment of Transactionally Defined Coping Function1. Eur. J. Psychol. Assess. 20, 49–58. doi:10.1027/1015-5759.20.1.49
Fox, J., Ahn, S. J., Janssen, J. H., Yeykelis, L., Segovia, K. Y., and Bailenson, J. N. (2015). Avatars versus Agents: A Meta-Analysis Quantifying the Effect of Agency on Social Influence. Human-Computer Interact. 30, 401–432. doi:10.1080/07370024.2014.921494
Hays, M. J., Campbell, J. C., Trimmer, M. A., Poore, J. C., Webb, A. K., and King, T. K. (2012). Can Role-Play with Virtual Humans Teach Interpersonal Skillsin Interservice/Industry Training, Simulation and Education Conference (I/ITSEC). Orlando, FL: University Of Southern California Los Angeles Inst For Creative Technologies.
Hofer, M., Hartmann, T., Eden, A., Ratan, R., and Hahn, L. (2020). The Role of Plausibility in the Experience of Spatial Presence in Virtual Environments. Front. virtual real. 1, 2. doi:10.3389/frvir.2020.00002
Houts, P. S., Doak, C. C., Doak, L. G., and Loscalzo, M. J. (2006). The Role of Pictures in Improving Health Communication: A Review of Research on Attention, Comprehension, Recall, and Adherence. Patient Educ. Couns. 61, 173–190. doi:10.1016/j.pec.2005.05.004
Huber, B. J., and Epp, S. M. (2022). Teaching & Learning Focused Physical Assessments: An Innovative Clinical Support Tool. Nurse Educ. Pract. 59, 103131. doi:10.1016/j.nepr.2021.103131
Kotranza, A., Cendan, J., and Lok, B. (2010). Simulation of a Virtual Patient with Cranial Nerve Injury Augments Physician-Learner Concern for Patient Safety. Bio Algorithms Med. Syst. 6, 25–34. doi:10.2478/bams-2012-0001
Kotranza, A., Lok, B., Deladisma, A., Pugh, C. M., and Lind, D. S. (2009). Mixed Reality Humans: Evaluating Behavior, Usability, and Acceptability. IEEE Trans. Vis. Comput. Graph. 15, 369–382. doi:10.1109/TVCG.2008.195
Kotranza, A., and Lok, B. (2008). “Virtual Human + Tangible Interface = Mixed Reality Human an Initial Exploration with a Virtual Breast Exam Patient,” in Proceedings - IEEE Virtual Reality. doi:10.1109/VR.2008.4480757
Krasnoryadtseva, A., Dalbeth, N., and Petrie, K. J. (2020). The Effect of Different Styles of Medical Illustration on Information Comprehension, the Perception of Educational Material and Illness Beliefs. Patient Educ. Couns. 103, 556–562. doi:10.1016/j.pec.2019.09.026
Lok, B. (2006). Teaching Communication Skills with Virtual Humans. IEEE Comput. Grap. Appl. 26, 10–13. doi:10.1109/MCG.2006.68
McDonnell, R., Breidt, M., and Bülthoff, H. H. (2012). Render Me Real? ACM Trans. Graph. 31, 1–11. doi:10.1145/2185520.2185587
Nanji, K. C., Baca, K., and Raemer, D. B. (2013). The Effect of an Olfactory and Visual Cue on Realism and Engagement in a Health Care Simulation Experience. Simul. Healthc. J. Soc. Simul. Healthc. 8, 143–147. doi:10.1097/SIH.0b013e31827d27f9
Pausch, R., Crea, T., and Conway, M. (1992). A Literature Survey for Virtual Environments: Military Flight Simulator Visual Systems and Simulator Sickness. Presence Teleoperators Virtual Environ. 1, 344–363. doi:10.1162/pres.1992.1.3.344
Rademacher, P. M. (2003). Measuring the Perceived Visual Realism of Images. Ann Arbor, MI: ProQuest, The University of North Carolina at Chapel Hill.
Ring, L., Utami, D., and Bickmore, T. (2014). The Right Agent for the Job? Intelligent Virtual Agents,Lecture Notes Comput. Sci. 8637, 374–384. doi:10.1007/978-3-319-09767-1_49
Robinson, M. K. (2007). Population Differences in Skin Structure and Physiology and the Susceptibility to Irritant and Allergic Contact Dermatitis: Implications for Skin Safety Testing and Risk Assessment. Contact Dermat. 41, 65–79. doi:10.1111/j.1600-0536.1999.tb06229.x
Sundar, S. (2008). The MAIN Model : A Heuristic Approach to Understanding Technology Effects on Credibility. Cambridge, MA: Massachusetts Institute of Technology, Digital Media, Youth, and Credibility.
Vilaro, M. J., Wilson‐Howard, D. S., Griffin, L. N., Tavassoli, F., Zalake, M. S., Lok, B. C., et al. (2020). Tailoring Virtual Human‐delivered Interventions: A Digital Intervention Promoting Colorectal Cancer Screening for Black Women. Psycho‐Oncology 29, 2048–2056. doi:10.1002/pon.5538
Volonte, M., Anaraky, R. G., Venkatakrishnan, R., Venkatakrishnan, R., Knijnenburg, B. P., Duchowski, A. T., et al. (2020). Empirical Evaluation and Pathway Modeling of Visual Attention to Virtual Humans in an Appearance Fidelity Continuum. J. Multimodal User Interfaces 15, 109–119. doi:10.1007/s12193-020-00341-z
Volonte, M., Babu, S. V., Chaturvedi, H., Newsome, N., Ebrahimi, E., Roy, T., et al. (2016). Effects of Virtual Human Appearance Fidelity on Emotion Contagion in Affective Inter-personal Simulations. IEEE Trans. Vis. Comput. Graph. 22, 1326–1335. doi:10.1109/TVCG.2016.2518158
Volonte, M., Duchowski, A. T., and Babu, S. V. (2019). “Effects of a Virtual Human Appearance Fidelity Continuum on Visual Attention in Virtual Reality,” in IVA 2019 - Proceedings of the 19th ACM International Conference on Intelligent Virtual Agents. doi:10.1145/3308532.3329461
Wang, I., Smith, J., and Ruiz, J. (2019). “Exploring Virtual Agents for Augmented Reality,” in Proceedings of the 2019 CHI Conference on Human Factors in Computing Systems (New York, NY, USA: ACM). doi:10.1145/3290605.3300511
Watts, P. I., McDermott, D. S., Alinier, G., Charnetski, M., Ludlow, J., Horsley, E., et al. (2021). Healthcare Simulation Standards of Best PracticeTM Simulation Design. Clin. Simul. Nurs. 58, 14–21. doi:10.1016/j.ecns.2021.08.009
White, C., Chuah, J., Robb, A., Lok, B., Lampotang, S., Lizdas, D., et al. (2015). Using a Critical Incident Scenario with Virtual Humans to Assess Educational Needs of Nurses in a Postanesthesia Care Unit. J. Continuing Educ. Health Prof. 35, 158–165. doi:10.1002/chp.21302
Xu, K., and Liao, T. (2020). Explicating Cues: A Typology for Understanding Emerging Media Technologies. J. Computer-Mediated Commun. 25, 32–43. doi:10.1093/jcmc/zmz023
Zalake, M., Tavassoli, F., Griffin, L., Krieger, J., and Lok, B. (2019). “Internet-Based Tailored Virtual Human Health Intervention to Promote Colorectal Cancer Screening,” in Proceedings of the 19th ACM International Conference on Intelligent Virtual Agents (New York, NY, USA: Association for Computing Machinery), 73–80. doi:10.1145/3308532.3329471
Zell, E., Aliaga, C., Jarabo, A., Zibrek, K., Gutierrez, D., McDonnell, R., et al. (2015). To Stylize or Not to Stylize? ACM Trans. Graph. 34, 1–12. doi:10.1145/2816795.2818126
Zibrek, K., Martin, S., and McDonnell, R. (2019). Is Photorealism Important for Perception of Expressive Virtual Humans in Virtual Reality? ACM Trans. Appl. Percept. 16, 1–19. doi:10.1145/3349609
Keywords: virtual human, rendering style, visual cue, augmented reality, photorealistic, conversational agent
Citation: Stuart J, Aul K, Stephen A, Bumbach MD and Lok B (2022) The Effect of Virtual Human Rendering Style on User Perceptions of Visual Cues. Front. Virtual Real. 3:864676. doi: 10.3389/frvir.2022.864676
Received: 28 January 2022; Accepted: 25 April 2022;
Published: 16 May 2022.
Edited by:
Roghayeh Barmaki, University of Delaware, United StatesReviewed by:
Mark Billinghurst, University of South Australia, AustraliaCopyright © 2022 Stuart, Aul, Stephen, Bumbach and Lok. This is an open-access article distributed under the terms of the Creative Commons Attribution License (CC BY). The use, distribution or reproduction in other forums is permitted, provided the original author(s) and the copyright owner(s) are credited and that the original publication in this journal is cited, in accordance with accepted academic practice. No use, distribution or reproduction is permitted which does not comply with these terms.
*Correspondence: Jacob Stuart, amFjb2JzdHVhcnRAdWZsLmVkdQ==
Disclaimer: All claims expressed in this article are solely those of the authors and do not necessarily represent those of their affiliated organizations, or those of the publisher, the editors and the reviewers. Any product that may be evaluated in this article or claim that may be made by its manufacturer is not guaranteed or endorsed by the publisher.
Research integrity at Frontiers
Learn more about the work of our research integrity team to safeguard the quality of each article we publish.