- 1Université Paris Cité, UFR de Psychologie, Boulogne-Billancourt, France
- 2Sorbonne Université, CNRS, Institut des Systèmes Intelligents et de Robotique, ISIR, Paris, France
- 3Université Paris-Saclay, CNRS, Laboratoire interdisciplinaire des sciences du numérique, Orsay, France
- 4Université Paris Cité, Maladie d’Alzheimer, Paris, France
- 5Service gériatrie 1&2, Centre Mémoire de Ressources et Recherches Ile de France-Broca, AP-HP, Hôpital Broca, Paris, France
- 6Univ. Lille, ULR 4354 - CIREL - Centre Interuniversitaire de Recherche en Éducation de Lille, Lille, France
Clinician-patient communication is essential to successful care and treatment. However, health training programs do not provide sufficient clinical exposure to practice communication skills that are pivotal when interacting with patients exhibiting mental health or age-related disorders. Recently, virtual reality has been used to develop simulation and training tools, in particular through embodied virtual patients (VP) offering the opportunity to engage in face-to-face human-like interactions. In this article, we overview recent developments in the literature on the use of VP-simulation tools for training communicative skills in psychiatry and geriatrics, fields in which patients have challenging social communication difficulties. We begin by highlighting the importance of verbal and non-verbal communication, arguing that clinical interactions are an interpersonal process where the patient’s and the clinician’s behavior mutually shape each other and are central to the therapeutic alliance. We also highlight the different simulation tools available to train healthcare professionals to interact with patients. Then, after clarifying what communication with a VP is about, we propose an overview of the most significant VP applications to highlight: 1) in what context and for what purpose VP simulation tools are used in psychiatry (e.g. depression, suicide risk, PTSD) and geriatrics (e.g., care needs, dementia), 2) how VP are conceptualized, 3) how trainee behaviors are assessed. We complete this overview with the presentation of VirtuAlz, our tool designed to train health care professionals in the social skills required to interact with patients with dementia. Finally, we propose recommendations, best practices and uses for the design, conduct and evaluation of VP training sessions.
Introduction
“Medicine is an art whose magic and creative ability have long been recognized as residing in the interpersonal aspects of patient-physician relationship” (Hall et al., 1981). Creating and maintaining an effective and trustworthy clinician-patient relationship is fundamental for providing high-quality care (Ha and Longnecker, 2010). More specifically, the professional practice of health providers includes important verbal and non-verbal communication skills, especially during face-to-face interaction in order to promote therapeutic alliance (Zolnierek and DiMatteo, 2009) and patient’s satisfaction (Brown et al., 1999). Communication skills refer to the ability to convey information to another person effectively (Beaulieu et al., 2011). Thus, they refer not only to what is said but how it is said through non-verbal behavior, including tone of voice (prosody), active listening, empathy, gestures, body language, and facial expressions used when interacting with another person. Training in communication techniques for health professionals has become a major issue in health education. However, health training programs do not provide sufficient clinical exposure and supervision to acquire these essential skills (Brown et al., 1999). Recently, virtual reality (VR) has been used to develop simulation and training tools (Pottle, 2019), notably through embodied virtual agents (Lee et al., 2020), in order to respect the fundamental ethical principle of “never the first time on a patient” (Granry and Moll, 2012).
Clinician-Patient Communication: Why and How?
Interactions between a clinician and a patient can vary greatly depending on the clinical context (anesthesia, surgery, nursing, neurology or psychiatry), age (pediatrics, geriatrics) or state of the patient (comprehension capacity, cognitive or psychological profile). In any clinical context, successful interactions are generally associated with better adherence to care or treatment (Catty, 2004), medical outcome (Ruberton et al., 2016), enhanced mutual trust (Khullar, 2019) and patient satisfaction (Williams et al., 1998). Conversely, poorer or negative interactions can lead to medical non-adherence (Piette et al., 2005), patient distrust (Hawley, 2015) or clinician burnout (Chang et al., 2018). Successful interactions are driven by verbal and non-verbal communication. Verbal communication serves to evoke a reality and includes the content of what is said (intonation, choice of words) or even active listening (Kee et al., 2018). Non-verbal communication, whether intentional or unintentional, enables information to be shared without using speech, and includes social signals such as facial expressions, eye contact, head movements, posture, touch, interpersonal distance or tone of voice (for a review see Hall et al., 2019). While verbal communication is undoubtedly important during medical consultations and care (Conigliaro, 2007), non-verbal communication also plays a crucial role as it can reinforce or contradict verbal communication and thereby be instrumental to patient satisfaction or treatment outcome (Ambady et al., 2002; Lorié et al., 2017).
Clinicians should be concerned about their own non-verbal behavior as it influences the success of the consultation (Strasser et al., 2005; Roter et al., 2006). Specifically, maintaining eye contact, smiling, maintaining an adequate distance or direct body orientation with legs and arms uncrossed, and arm symmetry is generally associated with greater patient satisfaction (Beck et al., 2002). In addition, communicating positive messages and signs of empathy to patients can lead to better therapy outcomes, even in patients with serious illnesses (Hojat et al., 2011; Howick et al., 2018). Such elements may also lead the patient to judge the clinician as being warm and competent (Howe et al., 2019). Clinicians should also be skilled at identifying patients’ non-verbal behaviors, typically for diagnostic purposes, such as using facial or vocal cues to assess patient pain (Ruben et al., 2018) or mood changes in severe depression (Ellgring and Scherer, 1996; Douglas and Porter, 2010).
In the psychiatric or geriatric field, clinicians are expected to interpret specific non-verbal cues associated with patients’ psychological and behavioral symptoms, including signs such as emotional blunting, anxiety, apathy or aggression (Lehman et al., 2004; Templier et al., 2015). These behavioral symptoms are known to be difficult for clinicians to address and may even lead them to experience stress and burnout (O’Connor et al., 2018; Isik et al., 2019).
The dynamic non-verbal interaction between clinician and patient, which refers to the way in which the behavior of each interlocutor shapes and influences that of the other, is a crucial element in clinical practice (for a review, see Henry et al., 2012). Most research on non-verbal behavior in clinician-patient communication has been observational, focusing on examining associations with the collaborative relationship between clinician and patient (i.e., the therapeutic alliance) or with treatment outcomes. For instance, during a routine consultation, Lavelle et al. (2015) reported that when the patient displays pro-social behaviors that initiate or maintain interaction (i.e., direct gaze, smiling, nodding, using hand gestures), this in turn leads the clinician to display similar pro-social behavior and results in a better therapeutic relationship. Other studies that analyzed the vocal behavior of clinician-patient dyads from audio recordings have shown that synchronization between clinician and patient in prosody (Imel et al., 2014) or silence (Tomicic et al., 2017) plays a key role in clinical interaction and is associated with a better therapeutic alliance. This behavioral synchrony has also been evidenced with physiological measures, such as levels of electrodermal activity (Marci et al., 2007; Bar-Kalifa et al., 2019) or heart rate (Kodama et al., 2018), suggesting that in clinician-patient dyads, both partners tend to experience concomitant emotional activation. Interestingly, other methods have focused on assessing the relationship between clinicians and patients through automated objective videotape analysis algorithms. Ramseyer and Tschacher (2011, 2014), for instance, reported that in face-to-face psychotherapy, greater coordination of the patient’s and clinician’s body and head movements is associated with more positive therapeutic relationships and greater patient self-efficacy. Hence, the objective characterization of the dynamics of the clinician’s and patient’s movements provides a quantification of the non-verbal synchrony (see Delaherche et al., 2012). More recently, a two-person brain imaging interactive study (fMRI hyperscanning) has identified a potential brain-behavioral mechanism supporting the clinician-patient relationship. It showed that the mirroring of facial expression and brain-to-brain concordance in the temporo-parietal junction (TPJ) was significantly associated with patient analgesia and therapeutic alliance (Ellingsen et al., 2020).
Effective communication skills are an essential component of successful interactions between clinicians and patients. However, patients may exhibit inappropriate behaviors such as aggression, isolating themselves or refusing to respond to those who try to communicate with them, or refusing to take medication or follow treatments. It therefore appears crucial to train clinicians on how to communicate with patients from a person-centered care perspective (Del Piccolo and Goss, 2012), which means respecting and responding to the needs and values of each patient (Lewin et al., 2001; Hardman and Howick, 2019). Yet, this raises the question of whether non-verbal communication skills can be taught. In fact, it is not so much about developing specific non-verbal communication skills but more about using non-verbal communication to develop engagement, reciprocity, and synchronization in order to create a genuine therapeutic alliance that bonds the clinician and the patient (Shattell et al., 2007; McGilton et al., 2009). In any case, training and evaluating non-verbal communication and their links with clinical outcomes is challenging, often intrusive (e.g., real-time observation) or can require extensive resources (e.g., interactions involving actors representing standardized patients; Henry et al. (2012).
Medical Simulation in Healthcare Education
Medical simulation, which is increasingly being promoted by health authorities in many countries (Forbes and Kennedy, 2009; Granry and Moll, 2012; Alinier and Platt, 2014), refers to the use of standardized devices or virtual reality tools to emulate a clinical context to teach or train a health professional in clinical, therapeutic or diagnostic procedures (Ker and Bradley, 2010). The purpose of medical simulation is to reproduce real world clinical scenarios in a standardized, safe and reproducible context, which facilitates the immersion of trainees during their initial or continuing education (Gaba, 2004). While medical simulation cannot substitute for clinical experience, it offers the opportunity to receive feedback and gain confidence without going through the real clinical event or remaining ‘far away from the patient’ the first few times of practice (Okuda et al., 2009; Wolf et al., 2011).
In the field of healthcare, a variety of simulation techniques (see Figure 1) are thus suitable for both novice or expert trainees for psychomotor, cognitive, affective or communicative learning tasks (Munshi et al., 2015). ‘Procedural simulation’ conducted using realistic part- or whole-body manikins is the most traditional (Lapkin et al., 2010) and is mostly used to facilitate the learning of psychomotor skills such as surgery gestures or nursing care (see Rivière et al., 2018). ‘Standardized simulation’ is structured in the form of role-play with well-trained actors to simulate clinical scenarios or to portray a patient with a specific health concern (i.e., human standardized patient) providing in-depth experience of clinical reasoning, decision-making or communication techniques in various situations, including crisis intervention (Brender et al., 2005; Keltner et al., 2011). However, the use of standardised simulation is limited due to the high costs of recruiting and training patient actors, especially when actors need to play an adolescent, an elderly person or a person with mental health issues (Keiser and Turkelson, 2017). In addition, this type of simulation can also lead users to feel uncomfortable or apprehensive about acting in front of their peers (Albright et al., 2016). Finally, ‘virtual reality’ (VR), which has developed extensively over the last 2 decades in the field of healthcare, refers to a computer-screen-based simulation that offers a multisensory and immersive interactive experience in a safe environment (Mantovani et al., 2003; Rizzo et al., 2017; Riva and Serino, 2020). These techniques can be used to implement applications such as ‘serious games’ in which the trainee is confronted with virtual situations drawn from real-life events, allowing them to develop clinical reflexes (e.g., from the discovery of patient files to the administration of medication) (Wang et al., 2016) and that can incorporate the notion of feedback or scoring (Stuckless et al., 2014). Such tools may take the form of virtual world platforms such as Second Life® (see Irwin and Coutts, 2015) or The SimsTM (Arts, 2009), and allow for the creation of virtual hospital units (Aebersold et al., 2012) and interactions with virtual patients. Virtual simulation can also be implemented in the form of conversational agents such as ‘chatbots’ that can interact with users by simulating a human conversation through text or voice via smartphones or computers, and are able to interpret the user’s responses. Chatbots have shown their potential to promote clinician-patient communication (Friedman et al., 1977; Madhu et al., 2017; Jagtap et al., 2021).
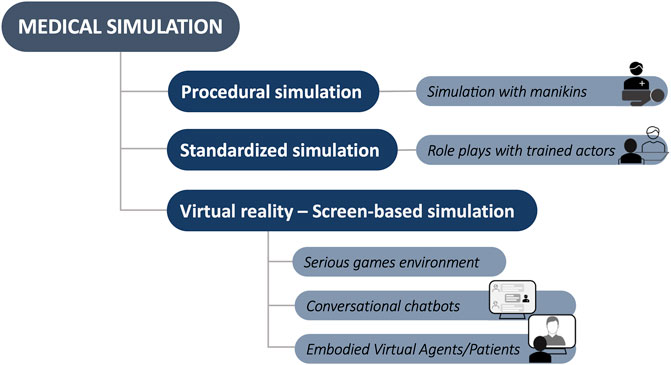
FIGURE 1. Simple classification of healthcare simulations. Procedural simulation is based on manikins that represent a full or partial human body; Standardized simulation is based on role-playing with patients played by actors in real clinical situations or through videos; Virtual reality simulation is based on computer technology to create interactive virtual worlds that the user can interact with (e.g., serious games, conversational chatbots or Embodied Virtual Agents.).
A more recent breakthrough in the field of healthcare is the use of Embodied Conversational Agents or ECAs. One approach, especially in the field of mental health (see Provoost et al., 2017) has been to use ECAs for diagnostic or remediation purposes, either as partners of social interaction (Georgescu et al., 2014; Tanaka et al., 2017b; Grossard et al., 2020), virtual coaches motivating the user (Torres et al., 2018; Ali et al., 2021), or even for virtual clinical interviews with real patients suffering from depression, post-traumatic stress disorder or dementia (Stratou et al., 2015; Philip et al., 2017; Mirheidari et al., 2019). In addition, ECAs have also come to be used as ‘virtual patients’ (VPs), i.e., representing a patient alone or in a virtual environment and offering the trainee (clinician or student) the possibility to engage in a human-like face-to-face interaction (see Combs and Combs, 2019). As such, medical educational strategies are increasingly shifting toward the use of VP simulations, as they are more scalable and reproducible, being available at all times and places, while providing learning outcomes comparable to standardized clinical learning environments (Consorti et al., 2012; Quail et al., 2016), including improved skills when interacting with real patients (Cook and Triola, 2009). It has also been shown that people show empathetic responses (Deladisma et al., 2007) and tend to show more willingness to disclose information when interacting with virtual compared to real humans, possibly because of this “soothing” effect since “after all, it is just a computer” (Lucas et al., 2014).
Aim of the Overview
The benefit of VPs for the training of so-called soft or non-technical skills, including communication or decision-making, has been surveyed elsewhere. One of the first reviews to show a benefit of VPs was on clinical reasoning rather than on communication itself (Cook et al., 2010). Another systematic review, that did not cover communication skills, reported that simulation using VPs can enhance clinician empathy among healthcare students (Bearman et al., 2015). An integrative review in the field of nursing demonstrated the value of VPs for developing decision-making, communication, or teamwork skills (Peddle et al., 2016). More recently, a systematic review in the context of pharmacist-patient interactions also showed the benefit of VPs in developing communication or counseling skills (Richardson et al., 2020). In addition, Lee et al. (2020) conducted a systematic review focusing on the design and evaluation characteristics allowing for effective medical communication skills education based on VP simulation. None of these reviews, however, covered studies on training communicative skills with embodied VPs displaying psychiatric or geriatric disorders.
It should be pointed out, however, that communicating with patients with psychiatric disorders (e.g., depression, schizophrenia, personality disorders) or age-related and degenerative disorders (e.g., dementia) is considered as very challenging because of the behavioral, thinking or language disturbances that occur with the illness (Hartley et al., 2020). However, this dimension is not sufficiently taken into account in the training of clinicians, in particular non-verbal communication to which these patients are very sensitive, thanks to their partially preserved capacity to integrate multimodal social signals (Maurage and Campanella, 2013; Giannitelli et al., 2015; Templier et al., 2015; Xavier et al., 2015).
The aim of the present article is to highlight the relevance of technology-assisted education through VPs, in addressing the specific challenges facing the training of clinician-patient communication in psychiatric and geriatric care education. We start by explaining what communicating with a VP is about, and by describing their role in training communication skills in psychiatry and geriatrics by presenting important studies in this field (see Table 1). Our intention is to provide a roadmap for those interested in learning more about the use of VP as a simulation framework for training clinician-patient communication skills, including their strengths and weaknesses, in psychiatric and geriatric care education. To that purpose, the overview is driven by highlighting several key features of VP simulation tools (see Figure 2) related to the VP itself (e.g., predominant competencies, underlying simulation model, tool evaluation) and the user (e.g., target competencies, underlying clinical situation, user evaluation).
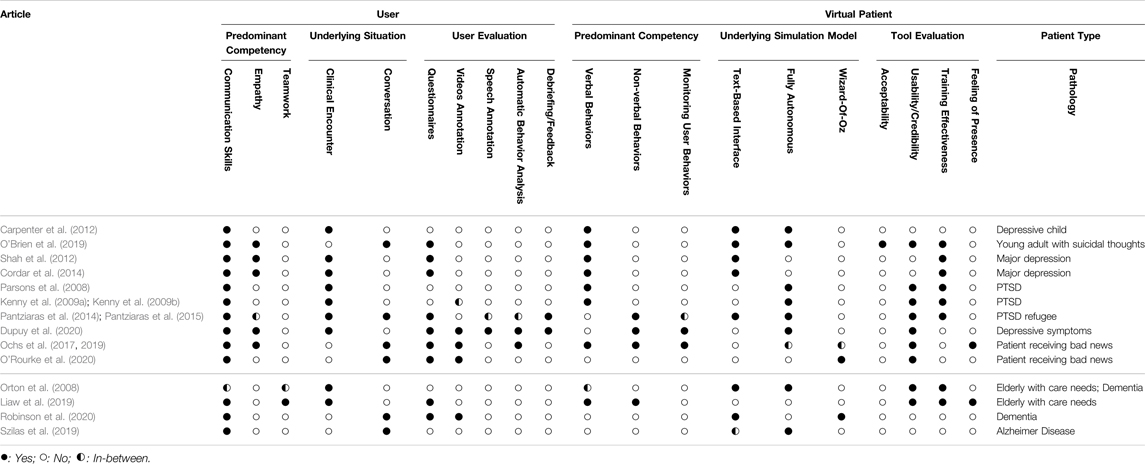
Table 1. Overview of annotated attributes in our selected list of articles presented on Section 2. The annotation procedure captures the user and the VP characteristics in simulation tools for clinician-patient communication in the field of psychiatry and geriatrics.
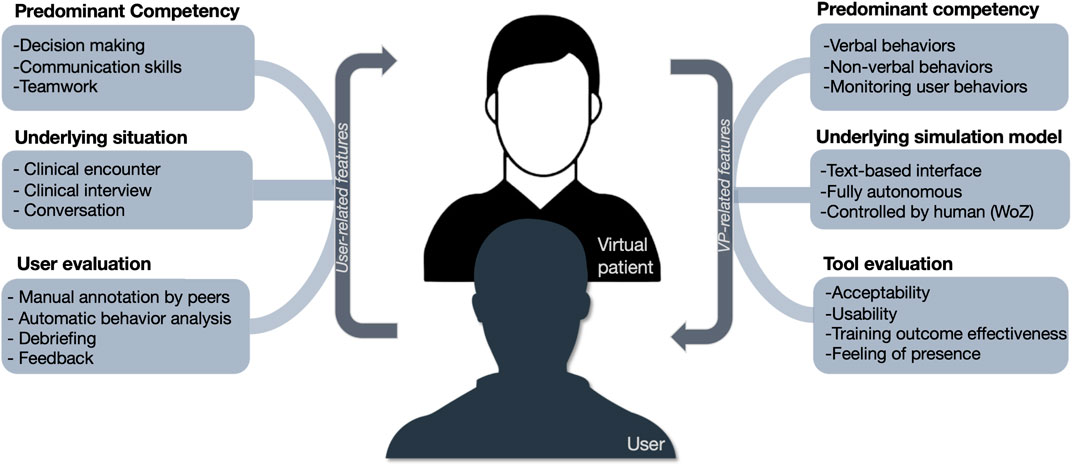
FIGURE 2. Key features of healthcare simulation tools using embodied virtual patients (VPs), applicable to the user and the VP. On the left, those concerning the user (e.g., clinician, student), including the predominant competencies targeted for training, the type of underlying situation simulated, as well as the user evaluation; On the right, those concerning the embodied virtual patient, including the predominant competencies of the VP, the underlying simulation model, and the tool evaluation by the user. WoZ: Wizard-of-Oz.
Role and Interest of Embodied Virtual Patients in Healthcare
Communication With an Embodied Virtual Patient: What Is This About?
Virtual Reality-based technologies are increasingly used in the field of healthcare and scientific research for simulating cognitive and socio-emotional skills (Riva and Serino, 2020) or studying human social interaction (Pan and Hamilton, 2018). Thus, it is now possible to interact and communicate with a virtual partner, such as embodied conversational agents (ECA) (Cassell et al., 2000; Loveys et al., 2020; Pavic et al., 2020), and thanks to the ability of ECAs to simulate and mimic human behavior, users tend to interact with them as with a real person (Gratch et al., 2013) and to assign them mental states (Callejas et al., 2014). In the context of healthcare simulation training, embodied VPs are typically computer-based programs using ECAs and simulating real patients and emulating a clinical encounter (see Cook and Triola, 2009; Su and Chang, 2021). The challenge is then to provide enough realism and reliability to make the learner’s experience sufficiently relevant and useful (see Talbot and Rizzo, 2019). One approach is to offer case-based training whereby the interaction with the VP unfolds as a function of the trainee’s responses (see Staccini and Fournier, 2019; Staccini, 2021). VPs can be fully autonomous and engage in brief interactions with the user, under no human control, or can be controlled by a human operator, through a Wizard-of-Oz (WoZ) procedure, which controls the verbal or non-verbal responses of the virtual patient during the interaction (Fraser and Gilbert, 1991; Riek, 2012). Whether automated or human-controlled, computer systems are generally equipped to record the user’s non-verbal behaviors (e.g., voice, gestures, body movements, gaze, or facial expressions) and use them to modulate the evolution of the interaction, giving a high degree of realism and social presence (Fox et al., 2015). In the context of communication training, the overall value of using VPs in the field of healthcare is that they offer the opportunity to practice communicating with patients in a stress-free context (Elzubeir et al., 2010) where mistakes or bad decisions are inconsequential (e.g., breaking bad news, Carrard et al., 2020). This type of virtual environment also gives the trainee the opportunity of self-observation, allowing for the identification of the most effective communication practices and thereby increasing self-confidence (Baumann-Birkbeck et al., 2017). Such a tool may finally be supplemented by an evaluation or coding of the trainee’s verbal or non-verbal behaviors, via external judges or by computer-based automatic analyses.
VP-Simulation for Clinician-Patient Communication in Psychiatry
Psychiatry is a medical specialty that focuses on the diagnosis, treatment, and prevention of mental, emotional, and behavioral disorders. In recent years, the prevalence of disorders such as anxiety, depression, and stress has increased significantly (see Steel et al., 2014), and was magnified further during the COVID-19 crisis (Castelli et al., 2020; Salari et al., 2020). Other chronic and severe mental disorders, such as schizophrenia, involve social communication difficulties (Burns, 2006) that can be difficult for the clinician to address (McCabe et al., 2013). These difficulties, associated with the stigmatization of patients (Hinshaw and Stier, 2008), as well as stress, burnout, or job dissatisfaction of mental health professionals (Rössler, 2012), has motivated paradigm shifts in medical communication and education approaches. It has been shown, for instance, that ineffective psychiatrist-patient communication is associated with poorer patient outcomes and experiences (Schneider et al., 2004). However, although there are several guides on how to communicate with psychiatric patients (Priebe et al., 2011), studies of non-verbal behaviors in psychiatry are limited (Cruz et al., 2011) and efforts have to be made to improve clinician-patient communication in this field. In this context, training practices based on clinical simulation, including VP-simulation, have the potential to provide health care professionals with safe and controlled tools that can help them develop the necessary skills to care for or communicate with patients experiencing mental disorders (McNaughton et al., 2008; Piot et al., 2020).
One area where VP simulation has proven successful is in training clinicians to interact with people suffering from severe depression or at risk for suicide, as they allow for repetition in practice with challenging scenarios that clinicians may face. One of the first use cases of VPs in the field of psychiatry was designed as a web-based platform to train clinicians to assess suicide risk in youth (Carpenter et al., 2012). The Suicidal Avatars for Mental Health Training (SAMHT) platform allows the user to face a VP who can move, speak and is embodied as a child suffering from depressive symptoms. The user converses with the VP by selecting questions related to suicide risk from a predetermined text-based list, which are repeated by a synthesized voice (as if the user was asking the question) and allows the VP to respond through an intelligent decision tree. One of the major limitations of this study, which appears to be a proof of concept, is that it did not consider the educational value of the tool nor the trainees’ behaviors. More recently, another work (O’Brien et al., 2019) tested the acceptability and benefit of VP simulation, using The PeopleSim® technology, to train mental health practitioners to interact effectively with individuals at risk for suicide. The VP embodies a fictive 20-year-old female patient who states that she has thought about committing suicide. In the scenario, the patient comes to the clinic to be evaluated on her ability to return to work. The trainee’s goal is to encourage the patient to share details of her thoughts to assess the immediate risk of suicide, while empathizing with the patient’s thoughts. The proposed simulation consists of a unidirectional interaction with the VP–via a text-based interface incorporating pre-recorded videos of the patient–where the trainee can select from a list the question to ask the patient who can answer via a chat window. Based on their choices, an on-screen virtual instructor also provides text-based advice or feedback to help trainees improve their skills. After the conversation, the 20 participants who took part in this pilot study found the training experience to be satisfactory (feasibility and acceptability), especially in terms of training communication skills. Finally, the trainees’ knowledge (assessed using pre-post training questionnaires) showed a significant improvement. A limitation of this work is that with the pre-recorded videos of the VP, the interaction lacks naturalness, and the verbal and non-verbal behavior of the trainee is not taken into account.
Using The Sims video game, other studies have focused on the use of VPs to enhance the empathy and interpersonal communication skills of medical students during interactions with a 21-year-old virtual patient (Ms. Cynthia Young) suffering from major depressive episodes (Shah et al., 2012; Cordar et al., 2014). The interaction between the trainee and the VP was bidirectional (i.e., each can ask the other participant questions according to a predefined script) and relied on a text-based interface incorporating images of the patient (Shah et al., 2012) or 90-s cutscenes depicting short moments in the VP’s life to provide a backstory (Cordar et al., 2014). These studies focused mainly on the evaluation of the educational tool by the students via questionnaires. Trainees expressed overall satisfaction of the interaction with the VP, found the tool easy to use, and considered that it could represent a good educational tool. The empathy of 35 trainees was evaluated (Cordar et al., 2014) from text-based transcripts only (with or without VP backstory) and users’ verbal or non-verbal behaviors were not considered. Results suggested that VPs with a backstory, showing how the patient may be affected by their illness, are an effective training tool for interpersonal communication skills, such as empathy. A limitation of these studies is that, in the limited environment of 3D virtual world games, VPs are not able to converse in a realistic manner, nor respond to dialogues with human-like non-verbal behaviors or emotions.
A further area where VP simulation has proven effective is in training to interact with people with post-traumatic stress disorder (PTSD). PTSD is a psychiatric disorder related to trauma caused by exposure to a traumatic or stressful event (e.g., war, natural disasters, terrorist attacks, assaults) usually resulting in persistent feelings of anxiety and psychological distress, and potentially leading to altered social-emotional behaviors or depression (Zoellner et al., 2014). Several studies in this area (e.g., Parsons et al., 2008; Kenny et al., 2009a,b) have focused on improving the interviewing or communication skills of mental health students by having them interacting with a VP embodied in Justina1, a female adolescent suffering from PTSD following an assault. Interactions were designed as a 15-min clinical interview in which 15 novices in mental health (Parsons et al., 2008; Kenny et al., 2009a) or 15 novice and nine expert mental health clinicians (Kenny et al., 2009b) were asked to assess a patient’s initial diagnosis of PTSD. The VP, displayed on a computer screen, spoke in natural language with the user. Interestingly, the user’s speech was recorded and transcribed in real-time so that it could be interpreted by a statistical question-answer system (i.e., based on a real clinical corpus) to generate the VP’s verbal behavior. The transcription of the whole dialogue session was also recorded and annotated in terms of whether clinicians asked the appropriate questions needed to determine whether the patient reported symptoms that met the criteria for PTSD. Overall, results of these studies (see also Rizzo and Shilling, 2017) showed a good evaluation of the credibility of the tool by users, despite encountering some problems with voice recognition. In addition, novices asked the VP more questions about general matters whereas experts were better able to ask the specific questions needed to make a differential diagnosis. While this approach is promising, it focused on natural language, and the fact that non-verbal behaviors such as users’ facial expressions were recorded but not yet exploited in the study somewhat limits its impact.
Another application of VPs concerns the training of health care professionals in the field of transcultural psychiatry and refers to clinicians’ interactions with patients from diverse ethnic backgrounds. Pantziaras et al. (2014, 2015) developed a VP system called Refugee Trauma Simulation (RT-SIM) portraying a refugee (Mrs K) exhibiting severe symptoms of PTSD. The interaction, based on a predetermined question-answer scenario, was designed as a virtual psychiatric interview (up to 45 min) requiring 32 residents in psychiatry to provide a differential diagnosis and treatment program of a refugee with PTSD. The VP tool aimed at providing knowledge and training on identifying PTSD symptoms, clinical management, and communication skills. The VP displayed on a computer screen was depicted using pre-recorded video clips and used prerecorded sentences that were played according to the questions chosen by trainees on a list. An interesting element of this tool was the inclusion of an automated feedback module covering the VP’s perspective on the consultation, the clinical aspects of PTSD diagnosis/management, and the trainee’s communication skills. This feedback was given in the form of a video presentation of the virtual patient (VP) speaking directly to the trainee (e.g., perceived level of empathy, relevance of questions asked to the problems encountered). Assessments included both tool evaluation (credibility, usability, and effectiveness) by the trainees and trainee evaluation (self-report of emotional reactions, questionnaire-based assessment of PTSD knowledge acquisition, and assessment of communication skills). Overall, the results showed that the tool was positively evaluated by the trainees in terms of ease of use and effectiveness. In addition, the training session had a significant impact on the improvement of knowledge (pre- and post-interaction). Of note, the follow-up evaluation, several weeks after the interaction, showed that the knowledge gain decreased over time, suggesting that a single training session with the VP does not seem to be sufficient to ensure long-term learning. A limitation of the study is that the article did not provide any details about how communication skills were automatically analyzed by the system and evaluated.
More recently, a study focused on improving the empathic communication skills of medical students during realistic psychiatric interviews with a middle-aged VP with affective disorders (Dupuy et al., 2020). Interestingly, the simulation was not exclusively text-based or speech-based as in previous studies, but also addressed the VP’s non-verbal behavior as well as the trainees’ non-verbal and empathic behavior. The interaction, based on a predetermined question-answer scenario, was designed as a 35-min psychiatric interview requiring 35 students (medical, psychiatric) to conduct interviews with a middle-aged VP with major depressive disorder and to extract semiology (i.e., clinical manifestations). The VP was displayed on a large human-sized screen and could interact with the user in natural language, allowing the user’s speech to be interpreted via a speech recognition system. The strength of this study is that it emphasized not only the non-verbal behaviors of the VP (prosody, gestures, movements) but also those of the trainees (recording of facial expressions, with manual annotation of the videos and automatic analysis by an emotion recognition software). The tool was positively evaluated, in terms of usefulness, realism and credibility, during a debriefing (i.e., semi-structured interview) with the trainees. The results also suggest that the trainees maintained a neutral face during the interview, a finding interpreted by the authors as a form of empathy and the ability to maintain a certain emotional distance. Overall, this work highlights the added value of automatic facial expression recognition in psychiatry training.
A last interesting proposal consists in training health professionals to break bad news to patients, which requires fine skills in psychology, non-verbal behaviors, and empathy. Although this work is not strictly in the field of psychiatry, it seemed relevant to all those who wish to learn more about the use of VPs for training clinician-patient communication skills, as breaking bad news is a frequent and challenging task for clinicians in most clinical specialties (Fallowfield and Jenkins, 2004). In psychiatry, this may include conversations about the irreversible cognitive impairment of schizophrenia in a young adult (Cleary et al., 2009), in geriatrics, conversations about death issues (Lenherr et al., 2012). Hence, breaking bad news with empathy and being involved in the struggle that follows can make a significant difference. In one work (Ochs et al., 2017, 2019), the scenario required the physician to explain to a patient that a complication had occurred during her operation, requiring a second operation in a day. The stated objective was to train the physician to verify that their verbal and non-verbal communication had the right impact on the patient. The 22 participants in this study (7 expert physicians, 12 naive students) interacted in natural language with the VP that communicated through verbal (e.g., questions) and non-verbal (e.g., nods, smiles) behaviors depending on the trainee’s behaviors. To overcome the limitations of voice-based tools controlled via speech-to-text modules only (e.g., misunderstandings, frustration due to transcription errors), the authors preferred a Wizard-of-Oz (WoZ) procedure, where a human operator observes the user and updates the VP response accordingly. The strength of this study is that it displayed the VP in different formats, including a computer screen and two previously unexplored immersive virtual reality systems: a Head-Mounted Display (HMD) and a 3D immersive room with wall projection (CAVE). The objective was to analyze the effect of the immersion format on the users’ feeling of presence. The results of the study showed that the CAVE seems to improve the trainee’s experience (feeling of presence, perception of the virtual patient) and the credibility of the tool compared to the HMD and the computer screen. The results also showed that the immersive room (CAVE) is particularly suitable for physicians (i.e., more engagement) compared to naive participants, suggesting the potential effect of the “familiar” context on the interactive experience. Finally, although this approach is promising, especially via the fully immersive approach, the fact that the non-verbal behaviors (gestures, movement, facial expressions) of the users were recorded via sensors (i.e., Kinect) but not yet exploited limits its potential somewhat. In another recent study on the same topic (O’Rourke et al., 2020), 60 medical students were asked to deliver bad news to the spouse of a patient experiencing a medical error. The interest of this study was to compare the interaction in terms of communication skills with an embodied VP or a standardized patient (SP) played by a paid actor. The VP, displayed on a large human-sized screen and controlled by a human operator in a WoZ-like procedure, communicated through verbal and non-verbal behavior (head movements, facial expressions, and gestures). The tool, which was rated by users in terms of credibility, was found to be less authentic with the VP compared to the SP. The novelty of this study was to assess communication performance (e.g., “Used appropriate eye contact”) as well as trainees’ pre-post interaction experience on an emotional level through subjective questionnaires and salivary cortisol concentration. Interestingly, the results of this study suggest that trainees’ task performance and emotional reactions do not differ whether they interact with a SP or a VP.
VP-Simulation for Clinician-Patient Communication in Geriatrics
Geriatrics is a medical specialty devoted to the health of elderly people by preventing and treating diseases and disabilities in the elderly. The population over the age of 65 is gradually increasing and, due to the general decline in their physical or cognitive health (Gill et al., 1996), has substantial needs in terms of health care (Hashimoto and Tabata, 2010). Elderly patients often present with complex pathologies that require extensive explanation by clinicians (see Ambady et al., 2002). In addition, they tend to be relatively passive in their interactions, probably for generational reasons or for apprehension of being perceived as disrespectful (Gorawara-Bhat et al., 2007). Furthermore, as the population ages, an increasing number of people are at risk of developing Alzheimer’s disease or related dementia (Baumgart et al., 2015). Alzheimer’s disease is characterized by a progressive decline in cognitive resources (e.g., memory, language, judgement, attention, etc.) and is associated with behavioral disorders (e.g., aggression, agitation, withdrawal or resistance to care) leading to interpersonal problems and contributing to the patient’s loss of autonomy (Orange, 2001; Chaby and Narme, 2009). This leads to difficulties for the patient in expressing their needs or feelings, even though they may rely on non-verbal cues (prosody, facial expressions, gestures, etc.) over time (Alsawy et al., 2020), which implies several challenges for the clinician to communicate with them adequately (van Manen et al., 2020).
In geriatric practice, although the impact of clinicians’ non-verbal behaviors on elderly patient outcomes is important as well (Ishikawa et al., 2006), methods to study clinicians’ non-verbal behaviors are limited (Collins et al., 2011). A few studies have attempted to use behavioral methods through observation of video recordings to code the non-verbal Dimension in physician-Elderly Patient transactions (NDEPT), including the analysis of the physician’s body language during the interaction such as posture, eye contact, facial expressions, and social touch (Gorawara-Bhat et al., 2007; Gorawara-Bhat and Cook, 2011; Stepanikova et al., 2012; Gorawara-Bhat et al., 2013). Interestingly, these studies found that eye contact was the most frequently used non-verbal behavior by clinicians when communicating with elderly patients.
In this context, it becomes crucial to increase the competence of the entire healthcare workforce to communicate and maintain relationships with this geriatric population. Unfortunately, this dimension is not sufficiently considered in the training of clinicians, although several studies have already shown the positive effects of sensitizing clinicians to the use of non-verbal (Magai et al., 2002; Machiels et al., 2017) and empathetic communication (Brown et al., 2020). While VP simulation in geriatrics education is still in its infancy, it has the potential to address several challenges, including reduced access to real patients and the need to provide safe settings in which trainees can learn or practice their clinical skills (Tan et al., 2010).
To find a way to bridge the gap and improve the relationship between health care professionals and older adults with dementia, a first attempt was to use immersive technologies that virtually expose the clinician to what an older adult with dementia experiences. For instance, the Virtual Dementia Tour® (Beville, 2002; Slater et al., 2019) or myShoes (Adefila et al., 2016) projects allow clinicians to “embody” an elderly patient in a nursing home, to experience the physical and sensory difficulties, as well as the memory loss, feelings, and frustrations associated with dementia-related problems. While not directly involving training through patient interaction, these virtual immersion techniques that allow clinicians to put themselves in the patient’s shoes have shown to improve clinicians’ empathy and non-verbal behaviors toward elderly patients in real clinical practice (see Campbell et al., 2021).
One of the first use cases for VPs in geriatrics was designed as a web-based platform to offer clinicians the opportunity of interacting with elderly patients in clinical care encounters (Orton and Mulhausen, 2008). The GeriaSims platform allows the user to interact with a VP embodied as an elderly person and displayed as an image or multimedia clip. The interaction can last one to 2 hours, including questions about history, physical examination, or choices about treatment. Several scenario topics (i.e., modules) are proposed, including cognitive and behavioral disorders in dementia, medication management, primary care, palliative care, or falls (e.g., Ruiz and Leipzig, 2008). Prior to the interaction, the trainee has access to the patient’s backstory and the objectives of the encounter–which can be diagnostic or therapeutic–are indicated. The user converses with the VP by selecting questions from a predetermined text list. A virtual mentor is also available for assistance or guidance. At the end of the interaction, the tool was evaluated with questionnaires in terms of usability and effectiveness of learning. The authors report that the tool was rated by 287 trainees as easy to use and an effective way to achieve the targeted objective. An advantage of such a web-based tool is its accessibility at any time and place and thus the flexibility in planning training, which can be a drawback when using other VP simulation tools. One of the limitations, however, is that the VP is not able to converse in a realistic manner, nor respond to dialogues with non-verbal behaviors or human-like emotions. In addition, the trainee’s verbal and non-verbal behaviors are not assessed.
Another particularly innovative aspect of VP simulation in geriatrics is the training of communication skills between members of geriatric care teams. These interprofessional approaches are known to improve care efficiency and patient health outcomes (Curran et al., 2007). In this regard, a recent study proposed an interprofessional virtual visit scenario with multiple healthcare professionals at the bedside of Mr. Jin, a 80-year-old man with pain and fever after surgery (Liaw et al., 2019). To provide a backstory, the patient’s medical file is displayed on the screen before the interaction begins. Here, the various clinicians (physician, nurse, physical therapist, social worker, etc.) are integrated into the virtual environment via an avatar representing them and displayed on the screen and at the same time as the VP. The VP can communicate through verbal (synthesized voice) and non-verbal behavior (e.g., facial expressions, body movements, breathing noise, moaning) in response to the behavior of the clinicians, who can also communicate with each other. The tool was positively evaluated by the 29 trainees participating in the study in terms of usability and effectiveness, with a moderate evaluation of the feeling of presence. The interprofessional attitude of the trainees was also assessed by judges using a questionnaire. Overall, the results of this study show the feasibility of using a 3D environment simulation including a VP to foster social interactions and collaborative practices between multiple healthcare professionals to facilitate the sharing of information about the elderly patient. Note, however, that the non-verbal behavior of the participants was not recorded and or analyzed. Interestingly, this work was complemented by a study on the transferability –5 months after training–of virtual simulation learning to clinical practice (Liaw et al., 2020). Although the assessment of transferability to clinical practice was based on students’ subjective perceptions via focus groups, the results indicated transferability effects through clinical practice and how working together with different healthcare professions could ensure a more holistic care of a patient.
Interestingly, a study by Robinson et al. (2020) focused specifically on training communication skills of 82 speech pathology students in realistic conversations with an elderly VP with behavioral symptoms of dementia and resident of a nursing home (for a full description of the tool, see Quail et al., 2016). The 15-min interaction was based on a predetermined scenario of verbal (e.g., comprehension difficulties, word search, confusion) and non-verbal (e.g., crying, shrugging, chuckling) responses that were representative of dementia. Trainees were instructed to have a conversation with the VP to identify any problems he might be experiencing. Following this, a 15-min feedback with a clinical educator was offered to the trainees, aimed at encouraging the trainee to engage in self-reflection, followed by a second 15-min interaction with the elderly patient. The VP was displayed on a large human-sized screen and could interact with the user in natural language, with the user’s speech interpreted via a WoZ operator. The strength of this study is that it emphasized, for each clinical encounter, on the analysis of the trainee’s verbal and non-verbal behavior based on speech transcription (e.g., “demonstrates awareness of how his/her responses are affecting the communication partner”) and video annotation (e.g., “maintains appropriate eye contact”). This was coupled with a self-rating by the trainees of their communication skills. Findings revealed an improvement in students’ communication skills in the second interaction, confirmed by the improved self-ratings. However, it is not possible here to distinguish the benefit of the simulation on the verbal vs non-verbal level. Although not directly focused on clinician-patient communication training, another related study deserves to be mentioned (Szilas et al., 2019) as it reports the preliminary implementation of an embodied VP simulation tool to support interactions between family caregivers of patients with Alzheimer’s disease and the patient himself (i.e., a 65-year-old apathetic woman suffering from an early-stage Alzheimer Disease).
VirtuAlz–A VP Tool for Training Clinician to Communicate With People With Alzheimer’s Disease
As mentioned above, the development of simulation tools for training clinicians to communicate with people with dementia is still very limited. Here, we present a virtual VP tool called VirtuAlz that was designed for geriatric health professionals to sensitize them to the basic communication skills needed to interact with elderly patients with Alzheimer’s disease. The tool, including the behavior of the Alzheimer’s VP, is based on real clinical cases (e.g., medication administration, patient’s wandering) derived from a field observation conducted in a geriatric service and an analysis of communication training needs in this field (Becerril-Ortega et al., 2022).
A first 3D prototype including a hospital setting and an 89-year-old virtual patient were modeled and displayed on a large human-size screen (Figure 3). To provide a backstory, a patient file is displayed on the screen before the interaction starts. It includes medical information about the patient (i.e., name, age, diagnosis and medical history) and a description of the context before the interaction (e.g., restless night, refusal to eat) and the trainee’s objective for the current scenario (e.g., stimulate the patient and ensure that the medication is taken).
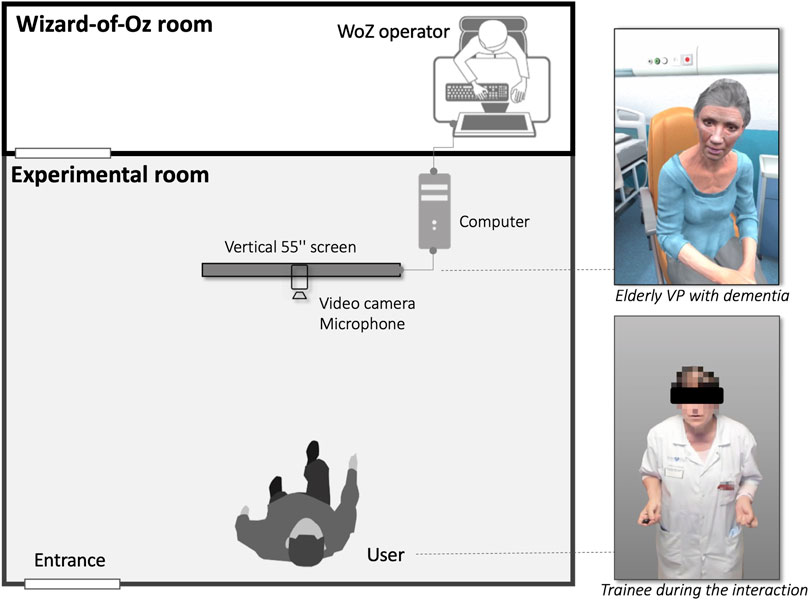
FIGURE 3. Overall architecture of the VirtuAlz platform. Here a user (i.e., clinician) is instructed to interact in a face-to-face clinical encounter with Ms. Dupont, an elderly virtual patient (VP) with Alzheimer’s disease symptoms. The 10-min interaction concerns taking medication and the VP exhibits behaviors such as agitation, aggression, apathy, or refusal of medication, and that the clinician must address (e.g., stimulate or pacify the patient) in order to persuade them to take their medication. The interface with the VP, including the hospital room, is displayed on a large screen (55′). The VP is controlled by a Wizard-of-Oz (WoZ) operator and equipped to record the user’s non-verbal behaviors (i.e., through a video camera and microphone) to modulate the evolution of the interaction.
Particular attention was paid to generating the predominant competencies of the VP, the implementation of the underlying technology through a WoZ simulation, the evaluation of the tool by trainees, and the automatic monitoring of the users’ behavior (see Figure 2). Concretely, the VP could produce verbal (synthesized voice) and non-verbal (body and head movements, gaze direction, facial expressions) behaviors that mimicked an elderly patient with signs of Alzheimer’s disease (apathy, memory loss, agitation, aggression, or refusal of care). The trainee could interact in natural language with the VP. In our simulation approach (Figure 3), the WoZ operator (a geriatric expert) selects the verbal and non-verbal behaviors (facial expressions, posture, etc.) to be generated in real time on the VP, based on the verbal and non-verbal behavior of the trainee who was expected to act as in a role-play with a real patient (Benamara et al., 2020). Each action performed by the WoZ to control the VP behavior is recorded and logged. After each session, the educational tool is assessed with questionnaires in terms of system usability, acceptability, VP realism and effectiveness of the educational tool.
In addition, a key aspect of the VirtuAlz platform relates to the automatic evaluation of the trainee’s non-verbal behaviors, that are captured by a front-facing camera during the interaction with the VP that lasted on average 6 min. The corpus collected was composed of 29 videos of clinician-VP interactions (each video involved a different clinician, exercising at the geriatric hospital as a physician, psychologist, nurse, or health care provider). We focus our analysis on non-verbal features of the trainee using automated feature extraction. Several non-verbal cues have been shown to capture relevant socio-affective states in similar settings with children (e.g., Delaherche et al., 2013; Avril et al., 2014; Anzalone et al., 2019) or adults (e.g., Aigrain et al., 2016). The main difference with this line of research is that VirtuAlz focuses on the training of clinician to communicate with elderly patient with signs of Alzheimer’s disease. Based on the literature mentioned in Section 2.3, we decided to focus on the analysis of the clinician’s non-verbal behaviors by considering the following non-verbal cues: body posture (i.e., body openness, computed as the distance between wrists and shoulder of the clinician), proxemics (i.e., physical proximity with the VP), facial expressions based on facial Action Units analysis (i.e., smile with mouth corners pull, AU14—mouth corners depress, AU15—frowning with eyebrows lowered and drawn together, AU4—eyebrow/eyes raising AU1/AU5) or self-touching on the head (i.e., hand position in the zone of the head, see Aigrain et al. (2016). The facial Action Units (AUs) are automatically extracted by OpenFace (Baltrusaitis et al., 2018) while the features related to self-touching on the head, proxemics and body openness are computed from the Body and Hand pose estimation using OpenPose (Cao et al., 2021). This set of non-verbal cues is then transformed into symbols and evaluated throughout the interaction (for more details see Zagdoun et al., 2021) to obtain explainable measures that could be indicators of openness to others, warmth, or empathy (e.g., duration of the clinician’s smiles, physical proximity or body opening during the interaction, see Mast and Hall (2017)) or discomfort or stress exhibited by the clinician (e.g., number of times the clinician touches their head, see Harrigan (1985). For the feedback, the symbols are contextualized by considering the behaviors of the virtual patient in order to assess the consistency of the clinician’s behaviors (e.g., smiling when approaching the patient, do not appear nervous with over-gesturing or excessive self-touching). Although the potential of the VirtuAlz tool has not yet been fully realized, it offers the opportunity for trainees to work on their non-verbal behavior in safe environments. Although the potential of the VirtuAlz tool has not yet been fully realized, it offers the opportunity for trainees to work on their non-verbal behavior in safe environments. Results from the automatic analysis of non-verbal behavior should also allow, during interviews with a clinical educator, to provide finer-grained feedback aimed at encouraging the trainee to engage in self-reflection before interacting with a real patient.
Discussion
As mentioned above, the value of VP-based simulation is that it provides tools that allow clinical teams and researchers to examine and model the verbal and non-verbal behaviors of the clinician/student while manipulating either the social-emotional or cognitive behavior of the VP and the visual appearance of the graphic environments. With good experimental control, these features make VPs powerful, useful, and reliable tools for studying the social communication that is central to clinician-patient interactions. However, in the field of psychiatry and geriatrics (Table 1), research has mainly focused on modeling the VP to simulate specific clinical encounters or on the evaluation of the simulation tool itself (e.g., feasibility, credibility, usability). Among the 14 tools (17 articles) using VP simulation presented in this overview, five used simulation models (e.g., prerecorded videos associated with text-based interfaces) that do not allow for realistic human-like conversations (Carpenter et al., 2012; O’Brien et al., 2019; Shah et al., 2012; Cordar et al., 2014; Pantziaras et al., 2014, 2015). By contrast, four tools offer immersive experiences using VPs displayed on human-sized screens and interacting in natural language (Ochs et al., 2017, 2019; Dupuy et al., 2020; O’Rourke et al., 2020; Robinson et al., 2020). Note that while tools based on web interfaces or pre-recorded videos may lack realism and fluidity, tools based on natural language and speech recognition, are rarely fully autonomous and currently require the intervention of a human WoZ operator. In addition, our overview reveals a lack of interest in evaluating the learner. Note that in most of the studies, the learner evaluation in terms of knowledge is considered in post-session (except in, Carpenter et al., 2012; O’Brien et al., 2019; Orton and Mulhausen, 2008; Szilas et al., 2019). Interestingly, a few studies propose a follow-up of learners’ knowledge several weeks after the interaction (Pantziaras et al., 2015) or an assessment of the transferability of the training to clinical practice (Liaw et al., 2020). By contrast, it was pointed out that an aspect seldom assessed was the learner’s verbal (except, Parsons et al., 2008; Kenny et al., 2009a,b; Pantziaras et al., 2014, 2015; Dupuy et al., 2020) and non-verbal (except, Dupuy et al., 2020; O’Rourke et al., 2020; Liaw et al., 2020; Robinson et al., 2020) behaviors. Non-verbal behaviors play a crucial role in clinician-patient relationships (Henry et al., 2012). Eye contact, physical proximity, clinician’s posture leaning toward the patient, or synchronization of the movements of this dyad may be associated with the length of the visit, the patient’s perceived empathy, feeling of trust, or patient self-disclosure (Lorié et al., 2017; Goldstein et al., 2020). One possible reason for this poor consideration of non-verbal behaviors is that the tools used rely primarily on human coding (speech transcript, video annotation), which is time-consuming and labor-intensive (D’Agostino and Bylund, 2011) and highly subjective due to biases of human annotation (Mast and Cousin, 2013). A precursor to developing adequate simulation tools in clinical settings is being able to capture and analyze the user’s non-verbal behaviors automatically so that they can be linked in real time to the patient’s behavior or for ulterior feedback (e.g., focus group, exchange with a tutor). Such automatic methods (without requiring intensive human coding or specialized training) have already been validated for analyzing the clinician-patient relationship (e.g., Hart et al., 2016; Tan et al., 2020). More generally, we suggest that automated analysis of clinician-patient interaction could offer a high temporal resolution and fine-grained analysis–sometimes invisible to the clinician’s or tutor’s eye–to provide feedback to clinicians or students on key aspects of their communication. The link between such fine-grained analysis of interaction and learning gain of clinicians has to be investigated. A promising direction is to consider the concept of productive engagement as the level of engagement that maximizes learning Nasir et al. (2021). Lastly, these educational tools should consider the ethical issues surrounding virtual reality research (e.g., risks related to information overload, intensification of arousal with virtual environments and re-entry into the real world, Behr et al., 2005) or human-computer interaction (usefulness in the light of the purpose, Grinbaum et al., 2017; Wullenkord and Eyssel, 2020), and also protection of the user’s data and privacy (e.g., audio/video recording of the learner, see Parsons, 2021).
Toward Best Practices and Uses
Importantly, when developing a VP simulation tool, the pedagogical context has a strong impact on the choice of technologies needed to develop the modules for simulating the VP’s verbal and non-verbal behaviors. Hence, a simulation tool that focuses on training nonverbal communication skills requires technologies to detect and interpret the trainee’s nonverbal behavior (Hoque et al., 2013).
It should also be considered that one of the most challenging parts of any VP application is the development of modules for simulating verbal and nonverbal behaviors. According to the context, they may only require a small subset of non-verbal behaviors such as posture and gestures, without necessarily focusing on facial expressions or gaze (Ochs et al., 2018). However, if the prime pedagogical objective is rather directed to the verbal content of communication or decision-making, a chatbot or a text-based interface may be suitable (Tanaka et al., 2017a).
At the same time, the choices regarding the technologies to be used are dependent on the resources and constraints of the project. Computer screen simulation can provide a multisensory and immersive interactive experience, with the possibility to design a VP that communicates verbally and nonverbally in real time. Alternatively, if the simulation needs to run on smartphones or tablets, technical resources are limited, and heavy computations cannot always be performed (e.g. real-time detection of non-verbal behavior). In such cases, the simulation will rely instead on text and graphical menu interfaces and use predefined animations to control the VP’s behavior (Philip et al., 2020).
It also has to be mentioned that several different technologies are available for simulating verbal and nonverbal VP behaviors. In most simulation systems in medical training, a decision tree is used to guide the interaction and trigger the VP’s reactions depending on the trainee’s choices and/or behavior. The possible VP reactions depends on the specific needs of the simulation, and can display specific characteristics of an agent, such as emotional states or pathological symptoms (Rizzo and Shilling, 2017). These reactions can be triggered from a predefined set of combinations of verbal and non-verbal behaviors, following the unfolding of the scenario script. VP’s reactions can also be selected in real-time by a human experimenter using a wizard-of-oz setup according to the specific goals of the training. Finally, VP reactions can be generated automatically using computational models that take into account a set of inputs provided by the user (for a review, see Wang and Ruiz, 2021). From a technical point of view, this latter technique is the most challenging to design. A first category of simulation is based on theories of cognitive psychology and allows emulating emotional states or a personality appropriate for a given context (Jones and Sabouret, 2013; DeVault et al., 2014), or even pathological symptoms (Benamara et al., 2022). A second category of simulation focuses on the verbal content of the interaction and allows interaction with the VP through natural language. In this context, speech-based facial animation can be synthesized from keywords or automatically with machine learning methods. Note that most systems are hybrid, incorporating both emotional models and natural language-based techniques (Vougioukas et al., 2020). In the future, additional non-verbal behaviors could be explored to improve the simulation of social interaction in medical environments. For instance, in medical care, the use of touch plays a crucial role in communicating with patients (Kim and Buschmann, 1999). Thus, the development of haptic interfaces should provide new ways to train social skills related to social touch (for a review, see Pelachaud et al., 2021).
In conclusion, the survey of the literature enables us to propose concrete recommendations for each step of the VP simulation process, including the development of the VP, conduct and evaluation of the training sessions. Designers will be expected to include healthcare professionals in all stages of the VP design in order to develop and implement a VP tailored to their needs and to define ways to improve the tool.
Virtual patient (VP) development.
• Use cases–Determine clinical cases that are challenging for clinician/student and patient interactions, and identify learner populations that might be interested in or benefit from training.
• Needs–Examine the needs of learners, particularly in difficult situations, by reviewing the literature and conducting field observations in clinical units, interviews and questionnaires.
• VP–Design a VP adapted to the needs and constraints of the project. On smartphones/tablets, the VP should be based on predefined animations. On computer screen the VP should be able to communicate autonomously verbally (e.g., question-answer) and non-verbally (e.g., facial expressions, gaze, body movements, gestures, prosody), to promote immersion (taking into account screen size) and human-like interactions.
• Scenario–Develop a narrative scenario, which may include problem-solving and allow multiple training sessions. A non-linear navigation structure (in which the learner’s decisions shape the VP’s behavior) will ensure flexibility and learner interactivity with the VP.
• Feedback–Provide feedback with messages, scores, visual representation of the VP’s emotional state. Gamification with leaderboards could help make the learning experience fun and engaging.
• Choices and tradeoffs–Review the available technology solutions for VP simulation and choose the one that seems to best meet the needs of the learners in terms of accessibility, usability, training needs, cost, data security and privacy, technical assistance requirements and sustainability. Authoring tools, if available, could help design, prototype, and deploy VPs in a variety of use cases.
• Feasibility/Usability testing–Test the feasibility of the system with learners, conduct usability testing, and define necessary adaptations of the tool.
Conduct and Assessment of training sessions.
• Training plan–Establish a training program (where and when to use VP simulation) that is tailored to the needs and abilities of the clinicians or students who will be involved in the learning.
• Tutorial–Offer specific training on the use of the tool for learners interested in participating in the learning experience. The availability of educational material adapted to this objective (e.g., tutorials) can help to enhance understanding of the tool’s use.
• Debriefing–Conduct VP learning sessions followed by debriefing with a tutor at the end of the session to allow learners to discuss the use case and ask questions.
• Training effectiveness–Evaluate the learning gain pre- and post-session with questionnaires.
• Non-verbal communication–Provide tools to measure and interpret non-verbal characteristics of clinician-patient communication. An automated or semi-automated tool (commercial software) for measuring and analyzing non-verbal communication can provide additional value over manual annotation.
• Follow-up–Define a way to follow-up on the VP learning at the individual and institutional level (university, hospital) to identify necessary modifications.
Author Contributions
LC and MC contributed to the conception and planning of the overview LC identified articles relevant to the topic and wrote the first draft of the manuscript. All authors contributed to manuscript revision, read and approved the submitted version.
Funding
This work has been supported by the French National Agency (ANR) (VirtuAlz, project ANR-17-CE19-0028)
Conflict of Interest
The authors declare that the research was conducted in the absence of any commercial or financial relationships that could be construed as a potential conflict of interest.
The handling editor AO declared a past co-authorship with the author LC.
Publisher’s Note
All claims expressed in this article are solely those of the authors and do not necessarily represent those of their affiliated organizations, or those of the publisher, the editors and the reviewers. Any product that may be evaluated in this article, or claim that may be made by its manufacturer, is not guaranteed or endorsed by the publisher.
Footnotes
1https://www.youtube.com/watch?v=jy1NKDz47aQ&t=14.
References
Adefila, A., Graham, S., Clouder, L., Bluteau, P., and Ball, S. (2016). Myshoes–The Future of Experiential Dementia Training? J. Ment. Health Train. Educ. Pract. 11, e12243. doi:10.1108/jmhtep-10-2015-0048
Aebersold, M., Tschannen, D., Stephens, M., Anderson, P., and Lei, X. (2012). Second Life: A New Strategy in Educating Nursing Students. Clin. Simul. Nurs. 8, e469–e475. doi:10.1016/j.ecns.2011.05.002
Aigrain, J., Spodenkiewicz, M., Dubuisson, S., Detyniecki, M., Cohen, D., and Chetouani, M. (2016). Multimodal Stress Detection from Multiple Assessments. IEEE Trans. Affect. Comput. 9, 491–506.
Albright, G., Adam, C., Serri, D., Bleeker, S., and Goldman, R. (2016). Harnessing the Power of Conversations with Virtual Humans to Change Health Behaviors. Mhealth 2, 44. doi:10.21037/mhealth.2016.11.02
Ali, R., Hoque, E., Duberstein, P., Schubert, L., Razavi, S. Z., Kane, B., et al. (2021). Aging and Engaging: A Pilot Randomized Controlled Trial of an Online Conversational Skills Coach for Older Adults. Am. J. Geriatric Psychiatry 29, 804–815. doi:10.1016/j.jagp.2020.11.004
Alinier, G., and Platt, A. (2014). International Overview of High-Level Simulation Education Initiatives in Relation to Critical Care. Nurs. Crit. Care 19, 42–49. doi:10.1111/nicc.12030
Alsawy, S., Tai, S., McEvoy, P., and Mansell, W. (2020). 'It's Nice to Think Somebody's Listening to Me Instead of Saying "oh Shut up"'. People with Dementia Reflect on what Makes Communication Good and Meaningful. J. Psychiatr. Ment. Health Nurs. 27, 151–161. doi:10.1111/jpm.12559
Ambady, N., Koo, J., Rosenthal, R., and Winograd, C. H. (2002). Physical Therapists' Nonverbal Communication Predicts Geriatric Patients' Health Outcomes. Psychol. aging 17, 443–452. doi:10.1037/0882-7974.17.3.443
Anzalone, S. M., Xavier, J., Boucenna, S., Billeci, L., Narzisi, A., Muratori, F., et al. (2019). Quantifying Patterns of Joint Attention during Human-Robot Interactions: An Application for Autism Spectrum Disorder Assessment. Pattern Recognit. Lett. 118, 42–50. doi:10.1016/j.patrec.2018.03.007
Avril, M., Leclère, C., Viaux, S., Michelet, S. p., Achard, C., Missonnier, S., et al. (2014). Social Signal Processing for Studying Parentâ€"infant Interaction. Front. Psychol. 5, 1437. doi:10.3389/fpsyg.2014.01437
Baltrusaitis, T., Zadeh, A., Lim, Y. C., and Morency, L.-P. (2018). “Openface 2.0: Facial Behavior Analysis Toolkit,” in 2018 13th IEEE International Conference on Automatic Face Gesture Recognition (FG 2018), 59–66. doi:10.1109/FG.2018.00019
Bar-Kalifa, E., Prinz, J. N., Atzil-Slonim, D., Rubel, J. A., Lutz, W., and Rafaeli, E. (2019). Physiological Synchrony and Therapeutic Alliance in an Imagery-Based Treatment. J. Couns. Psychol. 66, 508–517. doi:10.1037/cou0000358
Baumann-Birkbeck, L., Florentina, F., Karatas, O., Sun, J., Tang, T., Thaung, V., et al. (2017). Appraising the Role of the Virtual Patient for Therapeutics Health Education. Curr. Pharm. Teach. Learn. 9, 934–944. doi:10.1016/j.cptl.2017.05.012
Baumgart, M., Snyder, H. M., Carrillo, M. C., Fazio, S., Kim, H., and Johns, H. (2015). Summary of the Evidence on Modifiable Risk Factors for Cognitive Decline and Dementia: A Population‐based Perspective. Alzheimer's & Dement. 11, 718–726. doi:10.1016/j.jalz.2015.05.016
Bearman, M., Palermo, C., Allen, L. M., and Williams, B. (2015). Learning Empathy through Simulation. Simul. Healthc. 10, 308–319. doi:10.1097/sih.0000000000000113
Beaulieu, M.-D., Haggerty, J., Beaulieu, C., Bouharaoui, F., Lévesque, J.-F., Pineault, R., et al. (2011). Interpersonal Communication from the Patient Perspective: Comparison of Primary Healthcare Evaluation Instruments. hcpol 7, 108–123. doi:10.12927/hcpol.2011.22636
Becerril-Ortega, R., Vanderstichel, H., Petit, L., Urbiolagallegos, M. J., Schoch, J., Dacunha, S., et al. (2022). “Design Process for a Virtual Simulation Environment for Training Healthcare Professionals in Geriatrics,” in Simulation Training through the Lens of Experience and Activity Analysis, Ed. Springer (Berlin: Springer), 101–127. chap. 5. doi:10.1007/978-3-030-89567-9_6
Beck, R. S., Daughtridge, R., and Sloane, P. D. (2002). Physician-patient Communication in the Primary Care Office: a Systematic Review. J. Am. Board Fam. Pract. 15, 25–38.
Behr, K.-M., Nosper, A., Klimmt, C., and Hartmann, T. (2005). Some Practical Considerations of Ethical Issues in Vr Research. Presence Teleoperators Virtual Environ. 14, 668–676. doi:10.1162/105474605775196535
Benamara, A., Martin, J.-C., Prigent, E., Chaby, L., Chetouani, M., Zagdoun, J., et al. (2022). “Copalz: A Computational Model of Pathological Appraisal Biases for an Interactive Virtual Alzheimer Patient,” in Proceedings of the 21th International Conference on Autonomous Agents and Multi-Agent Systems (AMAS 2022) (AMAAS), 1–14.
Benamara, A., Ravenet, B., Prigent, E., Chaby, L., and Martin, J.-C. (2020). “Patient virtuel alzheimer: vers une modélisation informatique des pathologies émotionnelles et expressives,” in Workshop sur les Affects, Compagnons artificiels et Interactions.
Beville, P. K. (2002). Virtual Dementia Tour Helps Senditiz Health Care Providers. Am. J. Alzheimers Dis. Other Demen 17, 183–190. doi:10.1177/153331750201700301
Brender, E., Burke, A., and Glass, R. M. (2005). Standardized Patients. JAMA 294, 1172. doi:10.1001/jama.294.9.1172
Brown, E. L., Agronin, M. E., and Stein, J. R. (2020). Interventions to Enhance Empathy and Person-Centered Care for Individuals with Dementia: a Systematic Review. Res. gerontological Nurs. 13, 158–168. doi:10.3928/19404921-20191028-01
Brown, J. B., Boles, M., Mullooly, J. P., and Levinson, W. (1999). Effect of Clinician Communication Skills Training on Patient Satisfaction. Ann. Intern Med. 131, 822–829. doi:10.7326/0003-4819-131-11-199912070-00004
Callejas, Z., Griol, D., and López-Cózar, R. (2014). A Framework for the Assessment of Synthetic Personalities According to User Perception. Int. J. Human-Computer Stud. 72, 567–583. doi:10.1016/j.ijhcs.2014.02.002
Campbell, D., Lugger, S., Sigler, G. S., and Turkelson, C. (2021). Increasing Awareness, Sensitivity, and Empathy for Alzheimer's Dementia Patients Using Simulation. Nurse Educ. Today 98, 104764. doi:10.1016/j.nedt.2021.104764
Cao, Z., Hidalgo, G., Simon, T., Wei, S.-E., and Sheikh, Y. (2021). Openpose: Realtime Multi-Person 2d Pose Estimation Using Part Affinity Fields. IEEE Trans. Pattern Anal. Mach. Intell. 43, 172–186. doi:10.1109/tpami.2019.2929257
Carpenter, C., Osterberg, L., and Sutcliffe, G. (2012). “Samht-suicidal Avatars for Mental Health Training,” in 25th International Florida Artificial Intelligence Research Society Conference, FLAIRS-25, 484–487.
Carrard, V., Bourquin, C., Orsini, S., Schmid Mast, M., and Berney, A. (2020). Virtual Patient Simulation in Breaking Bad News Training for Medical Students. Patient Educ. Couns. 103, 1435–1438. doi:10.1016/j.pec.2020.01.019
Cassell, J., Sullivan, J., Churchill, E., and Prevost, S. (2000). Embodied Conversational Agents. Cambridge, MA, USA: MIT Press.
Castelli, L., Di Tella, M., Benfante, A., and Romeo, A. (2020). The Spread of Covid-19 in the Italian Population: Anxiety, Depression, and Post-traumatic Stress Symptoms. Can. J. Psychiatry 65, 731–732. doi:10.1177/0706743720938598
Catty, J. (2004). 'The Vehicle of Success': Theoretical and Empirical Perspectives on the Therapeutic Alliance in Psychotherapy and Psychiatry. Psychol. Psychotherapy Theory, Res. Pract. 77, 255–272. doi:10.1348/147608304323112528
Chaby, L., and Narme, P. (2009). Processing Facial Identity and Emotional Expression in Normal Aging and Neurodegenerative Diseases. Psychol. Neuropsychiatr. Vieil. 7, 31–42. doi:10.1684/pnv.2008.0154
Chang, B. P., Carter, E., Ng, N., Flynn, C., and Tan, T. (2018). Association of Clinician Burnout and Perceived Clinician-Patient Communication. Am. J. Emerg. Med. 36, 156–158. doi:10.1016/j.ajem.2017.07.031
Cleary, M., Hunt, G. E., and Horsfall, J. (2009). Delivering Difficult News in Psychiatric Settings. Harv. Rev. Psychiatry 17, 315–321. doi:10.3109/10673220903271780
Collins, L. G., Schrimmer, A., Diamond, J., and Burke, J. (2011). Evaluating Verbal and Non-verbal Communication Skills, in an Ethnogeriatric Osce. Patient Educ. Couns. 83, 158–162. doi:10.1016/j.pec.2010.05.012
Combs, C. D., and Combs, P. F. (2019). Emerging Roles of Virtual Patients in the Age of Ai. AMA J. Ethics 21, E153–E159. doi:10.1001/amajethics.2019.153
Conigliaro, R. L. (2007). Doctors Talking with Patients/patients Talking with Doctors: Improving Communication in Medical Visits. JAMA 297, 748–752. doi:10.1001/jama.297.7.750
Consorti, F., Mancuso, R., Nocioni, M., and Piccolo, A. (2012). Efficacy of Virtual Patients in Medical Education: a Meta-Analysis of Randomized Studies. Comput. Educ. 59, 1001–1008. doi:10.1016/j.compedu.2012.04.017
Cook, D. A., Erwin, P. J., and Triola, M. M. (2010). Computerized Virtual Patients in Health Professions Education: a Systematic Review and Meta-Analysis. Acad. Med. 85, 1589–1602. doi:10.1097/acm.0b013e3181edfe13
Cook, D. A., and Triola, M. M. (2009). Virtual Patients: a Critical Literature Review and Proposed Next Steps. Med. Educ. 43, 303–311. doi:10.1111/j.1365-2923.2008.03286.x
Cordar, A., Borish, M., Foster, A., and Lok, B. (2014). “Building Virtual Humans with Back Stories: Training Interpersonal Communication Skills in Medical Students,” in International Conference on Intelligent Virtual Agents (Berlin: Springer), 144–153. doi:10.1007/978-3-319-09767-1_17
Cruz, M., Roter, D., Cruz, R. F., Wieland, M., Cooper, L. A., Larson, S., et al. (2011). Psychiatrist-patient Verbal and Nonverbal Communications during Split-Treatment Appointments. Ps 62, 1361–1368. doi:10.1176/ps.62.11.pss6211_1361
Curran, V. R., Sharpe, D., and Forristall, J. (2007). Attitudes of Health Sciences Faculty Members towards Interprofessional Teamwork and Education. Med. Educ. 41, 892–896. doi:10.1111/j.1365-2923.2007.02823.x
D’Agostino, T. A., and Bylund, C. L. (2011). The Nonverbal Accommodation Analysis System (Naas): Initial Application and Evaluation. Patient Educ. Couns. 85, 33–39.
Del Piccolo, L., and Goss, C. (2012). People-centred Care: New Research Needs and Methods in Doctor-Patient Communication. Challenges in Mental Health. Epidemiol. Psychiatr. Sci. 21, 145–149. doi:10.1017/s2045796012000091
Deladisma, A. M., Cohen, M., Stevens, A., Wagner, P., Lok, B., Bernard, T., et al. (2007). Do medical Students Respond Empathetically to a Virtual Patient? Am. J. Surg. 193, 756–760. doi:10.1016/j.amjsurg.2007.01.021
Delaherche, E., Boucenna, S., Chetouani, M., and Cohen, D. (2013). “How Social Signal Processing (Ssp) Can Help Assessment of Bonding Phenomena in Developmental Psychology?,” in Neural Nets and Surroundings (Berlin: Springer), 345–356. doi:10.1007/978-3-642-35467-0_34
Delaherche, E., Chetouani, M., Mahdhaoui, A., Saint-Georges, C., Viaux, S., and Cohen, D. (2012). Interpersonal Synchrony: A Survey of Evaluation Methods across Disciplines. IEEE Trans. Affect. Comput. 3, 349–365. doi:10.1109/t-affc.2012.12
DeVault, D., Artstein, R., Benn, G., Dey, T., Fast, E., Gainer, A., et al. (2014). “Simsensei Kiosk: A Virtual Human Interviewer for Healthcare Decision Support,” in Proceedings of the 2014 international conference on Autonomous agents and multi-agent systems, 1061–1068.
Douglas, K. M., and Porter, R. J. (2010). Recognition of Disgusted Facial Expressions in Severe Depression. Br. J. Psychiatry 197, 156–157. doi:10.1192/bjp.bp.110.078113
Dupuy, L., Micoulaud-Franchi, J.-A., Cassoudesalle, H., Ballot, O., Dehail, P., Aouizerate, B., et al. (2020). Evaluation of a Virtual Agent to Train Medical Students Conducting Psychiatric Interviews for Diagnosing Major Depressive Disorders. J. Affect. Disord. 263, 1–8. doi:10.1016/j.jad.2019.11.117
Ellgring, H., and Scherer, K. R. (1996). Vocal Indicators of Mood Change in Depression. J. Nonverbal Behav. 20, 83–110. doi:10.1007/bf02253071
Ellingsen, D. M., Isenburg, K., Jung, C., Lee, J., Gerber, J., Mawla, I., et al. (2020). Dynamic Brain-To-Brain Concordance and Behavioral Mirroring as a Mechanism of the Patient-Clinician Interaction. Sci. Adv. 6, eabc1304. doi:10.1126/sciadv.abc1304
Elzubeir, M. A., Elzubeir, K. E., and Magzoub, M. E. (2010). Stress and Coping Strategies Among Arab Medical Students: towards a Research Agenda. Educ. Health (Abingdon) 23, 355.
Fallowfield, L., and Jenkins, V. (2004). Communicating Sad, Bad, and Difficult News in Medicine. Lancet 363, 312–319. doi:10.1016/s0140-6736(03)15392-5
Forbes, R., and Kennedy, P. (2009). The Enhancing Simulation (Safety in Medicine Utilizing Leading Advanced Simulation Technologies to Improve Outcomes Now) Act of 2009. Washington, DC: Committee on Energy and Commerce.
Fox, J., Ahn, S. J., Janssen, J. H., Yeykelis, L., Segovia, K. Y., and Bailenson, J. N. (2015). Avatars versus Agents: a Meta-Analysis Quantifying the Effect of Agency on Social Influence. Human-Computer Interact. 30, 401–432. doi:10.1080/07370024.2014.921494
Fraser, N. M., and Gilbert, G. N. (1991). Simulating Speech Systems. Comput. Speech & Lang. 5, 81–99. doi:10.1016/0885-2308(91)90019-m
Friedman, R. B., Newsom, R. S., Entine, S. M., Cheung, S., and Schultz, J. V. (1977). A Simulated Patient-Physician Encounter Using a Talking Computer. JAMA 238, 1927–1929. doi:10.1001/jama.238.18.1927
Gaba, D. M. (2004). The Future Vision of Simulation in Health Care. Qual. Saf. Health Care 13, i2–i10. doi:10.1136/qshc.2004.009878
Georgescu, A. L., Kuzmanovic, B., Roth, D., Bente, G., and Vogeley, K. (2014). The Use of Virtual Characters to Assess and Train Non-verbal Communication in High-Functioning Autism. Front. Hum. Neurosci. 8, 807. doi:10.3389/fnhum.2014.00807
Giannitelli, M., Xavier, J., François, A., Bodeau, N., Laurent, C., Cohen, D., et al. (2015). Facial, Vocal and Cross-Modal Emotion Processing in Early-Onset Schizophrenia Spectrum Disorders. Schizophrenia Res. 168, 252–259. doi:10.1016/j.schres.2015.07.039
Gill, T. M., Williams, C. S., Richardson, E. D., and Tinetti, M. E. (1996). Impairments in Physical Performance and Cognitive Status as Predisposing Factors for Functional Dependence Among Nondisabled Older Persons. Journals Gerontology Ser. A Biol. Sci. Med. Sci. 51A, M283–M288. doi:10.1093/gerona/51a.6.m283
Goldstein, P., Losin, E. A. R., Anderson, S. R., Schelkun, V. R., and Wager, T. D. (2020). Clinician-patient Movement Synchrony Mediates Social Group Effects on Interpersonal Trust and Perceived Pain. J. Pain 21, 1160–1174. doi:10.1016/j.jpain.2020.03.001
Gorawara-Bhat, R., and Cook, M. A. (2011). Eye Contact in Patient-Centered Communication. Patient Educ. Couns. 82, 442–447. doi:10.1016/j.pec.2010.12.002
Gorawara-Bhat, R., Cook, M. A., and Sachs, G. A. (2007). Nonverbal Communication in Doctor-Elderly Patient Transactions (NDEPT): Development of a Tool. Patient Educ. Couns. 66, 223–234. doi:10.1016/j.pec.2006.12.005
Gorawara-Bhat, R., Dethmers, D. L., and Cook, M. A. (2013). Physician Eye Contact and Elder Patient Perceptions of Understanding and Adherence. Patient Educ. Couns. 92, 375–380. doi:10.1016/j.pec.2013.03.002
Granry, J., and Moll, M. (2012). “Rapport de mission: État de l’art (national et international) en matière de pratiques de simulation dans le domaine de la santé,” in Guide de bonnes pratiques en matière de simulation en santé. Available at: www.has-sante.fr.
Gratch, J., Hartholt, A., Dehghani, M., and Marsella, S. (2013). Virtual Humans: a New Toolkit for Cognitive Science Research. Proc. Annu. Meet. Cognitive Sci. Soc. 35, 42–43.
Grinbaum, A., Chatila, R., Devillers, L., Ganascia, J.-G., Tessier, C., and Dauchet, M. (2017). Ethics in Robotics Research: Cerna Mission and Context. IEEE Robot. Autom. Mag. 24, 139–145. doi:10.1109/mra.2016.2611586
Grossard, C., Dapogny, A., Cohen, D., Bernheim, S., Juillet, E., Hamel, F., et al. (2020). Children with Autism Spectrum Disorder Produce More Ambiguous and Less Socially Meaningful Facial Expressions: an Experimental Study Using Random Forest Classifiers. Mol. Autism 11, 5–14. doi:10.1186/s13229-020-0312-2
Hall, J. A., Horgan, T. G., and Murphy, N. A. (2019). Nonverbal Communication. Annu. Rev. Psychol. 70, 271–294. doi:10.1146/annurev-psych-010418-103145
Hall, J. A., Roter, D. L., and Rand, C. S. (1981). Communication of Affect between Patient and Physician. J. Health Soc. Behav. 22, 18–30. doi:10.2307/2136365
Hardman, D., and Howick, J. (2019). The Friendly Relationship between Therapeutic Empathy and Person-Centred Care. Eur. J. Person Centered Healthc. 7, 351–357.
Harrigan, J. A. (1985). Self-touching as an Indicator of Underlying Affect and Language Processes. Soc. Sci. Med. 20, 1161–1168. doi:10.1016/0277-9536(85)90193-5
Hart, Y., Czerniak, E., Karnieli-Miller, O., Mayo, A. E., Ziv, A., Biegon, A., et al. (2016). Automated Video Analysis of Non-verbal Communication in a Medical Setting. Front. Psychol. 7, 1130. doi:10.3389/fpsyg.2016.01130
Hartley, S., Raphael, J., Lovell, K., and Berry, K. (2020). Effective Nurse-Patient Relationships in Mental Health Care: A Systematic Review of Interventions to Improve the Therapeutic Alliance. Int. J. Nurs. Stud. 102, 103490. doi:10.1016/j.ijnurstu.2019.103490
Hashimoto, K.-i., and Tabata, K. (2010). Population Aging, Health Care, and Growth. J. Popul. Econ. 23, 571–593. doi:10.1007/s00148-008-0216-5
Hawley, K. (2015). Trust and Distrust between Patient and Doctor. J. Eval. Clin. Pract. 21, 798–801. doi:10.1111/jep.12374
Henry, S. G., Fuhrel-Forbis, A., Rogers, M. A. M., and Eggly, S. (2012). Association between Nonverbal Communication during Clinical Interactions and Outcomes: a Systematic Review and Meta-Analysis. Patient Educ. Couns. 86, 297–315. doi:10.1016/j.pec.2011.07.006
Hinshaw, S. P., and Stier, A. (2008). Stigma as Related to Mental Disorders. Annu. Rev. Clin. Psychol. 4, 367–393. doi:10.1146/annurev.clinpsy.4.022007.141245
Hojat, M., Louis, D. Z., Markham, F. W., Wender, R., Rabinowitz, C., and Gonnella, J. S. (2011). Physiciansʼ Empathy and Clinical Outcomes for Diabetic Patients. Acad. Med. 86, 359–364. doi:10.1097/acm.0b013e3182086fe1
Hoque, M., Courgeon, M., Martin, J.-C., Mutlu, B., and Picard, R. W. (2013). “Mach: My Automated Conversation Coach,” in Proceedings of the 2013 ACM international joint conference on Pervasive and ubiquitous computing, 697–706.
Howe, L. C., Leibowitz, K. A., and Crum, A. J. (2019). When Your Doctor "Gets it" and "Gets You": The Critical Role of Competence and Warmth in the Patient-Provider Interaction. Front. Psychiatry 10, 475. doi:10.3389/fpsyt.2019.00475
Howick, J., Moscrop, A., Mebius, A., Fanshawe, T. R., Lewith, G., Bishop, F. L., et al. (2018). Effects of Empathic and Positive Communication in Healthcare Consultations: a Systematic Review and Meta-Analysis. J. R. Soc. Med. 111, 240–252. doi:10.1177/0141076818769477
Imel, Z. E., Barco, J. S., Brown, H. J., Baucom, B. R., Baer, J. S., Kircher, J. C., et al. (2014). The Association of Therapist Empathy and Synchrony in Vocally Encoded Arousal. J. Couns. Psychol. 61, 146–153. doi:10.1037/a0034943
Irwin, P., and Coutts, R. (2015). A Systematic Review of the Experience of Using Second Life in the Education of Undergraduate Nurses. J. Nurs. Educ. 54, 572–577. doi:10.3928/01484834-20150916-05
Ishikawa, H., Hashimoto, H., Kinoshita, M., Fujimori, S., Shimizu, T., and Yano, E. (2006). Evaluating Medical Students' Non-verbal Communication during the Objective Structured Clinical Examination. Med. Educ. 40, 1180–1187. doi:10.1111/j.1365-2929.2006.02628.x
Isik, A. T., Soysal, P., Solmi, M., and Veronese, N. (2019). Bidirectional Relationship between Caregiver Burden and Neuropsychiatric Symptoms in Patients with Alzheimer's Disease: A Narrative Review. Int. J. Geriatr. Psychiatry 34, 1326–1334. doi:10.1002/gps.4965
Jagtap, R., Phulare, K., Kurhade, M., and Gawande, K. S. (2021). “Healthcare Conversational Chatbot for Medical Diagnosis,” in Handbook of research on engineering, business, and healthcare applications of data science and analytics (Pennsylvania, USA: IGI Global), 401–415. doi:10.4018/978-1-7998-3053-5.ch020
Jones, H., and Sabouret, N. (2013). “Tardis-a Simulation Platform with an Affective Virtual Recruiter for Job Interviews,” in IDGEI (Intelligent Digital Games for Empowerment and Inclusion).
Kee, J. W. Y., Khoo, H. S., Lim, I., and Koh, M. Y. H. (2018). Communication Skills in Patient-Doctor Interactions: Learning from Patient Complaints. Health Prof. Educ. 4, 97–106. doi:10.1016/j.hpe.2017.03.006
Keiser, M. M., and Turkelson, C. (2017). Using Students as Standardized Patients: Development, Implementation, and Evaluation of a Standardized Patient Training Program. Clin. Simul. Nurs. 13, 321–330. doi:10.1016/j.ecns.2017.05.008
Keltner, N. L., Grant, J. S., and McLernon, D. (2011). Use of Actors as Standardized Psychiatric Patients. J. Psychosoc. Nurs. Ment. Health Serv. 49, 34–40. doi:10.3928/02793695-20110329-02
Kenny, P. G., Parsons, T. D., Gratch, J., and Rizzo, A. A. (2009a). “Evaluation of Novice and Expert Interpersonal Interaction Skills with a Virtual Patient,” in International Workshop on Intelligent Virtual Agents (Berlin: Springer), 511–512. doi:10.1007/978-3-642-04380-2_67
Kenny, P. G., Parsons, T. D., and Rizzo, A. (2009b). A Comparative Analysis between Experts and Novices Interacting with a Virtual Patient with Ptsd. Annu. Rev. Cybertherapy Telemedicine 144, 122–124.
Ker, J., and Bradley, P. (2010). Simulation in Medical Education. Underst. Med. Educ. Evid. theory Pract. 1, 164–180. doi:10.1002/9781444320282.ch12
Khullar, D. (2019). Building Trust in Health Care-Why, where, and How. Jama 322, 507–509. doi:10.1001/jama.2019.4892
Kim, E. J., and Buschmann, M. T. (1999). The Effect of Expressive Physical Touch on Patients with Dementia. Int. J. Nurs. Stud. 36, 235–243. doi:10.1016/s0020-7489(99)00019-x
Kodama, K., Tanaka, S., Shimizu, D., Hori, K., and Matsui, H. (2018). Heart Rate Synchrony in Psychological Counseling: a Case Study. Psych 09, 1858–1874. doi:10.4236/psych.2018.97108
Lapkin, S., Levett-Jones, T., Bellchambers, H., and Fernandez, R. (2010). Effectiveness of Patient Simulation Manikins in Teaching Clinical Reasoning Skills to Undergraduate Nursing Students: A Systematic Review. Clin. Simul. Nurs. 6, e207–e222. doi:10.1016/j.ecns.2010.05.005
Lavelle, M., Dimic, S., Wildgrube, C., McCabe, R., and Priebe, S. (2015). Non-verbal Communication in Meetings of Psychiatrists and Patients with Schizophrenia. Acta Psychiatr. Scand. 131, 197–205. doi:10.1111/acps.12319
Lee, J., Kim, H., Kim, K. H., Jung, D., Jowsey, T., and Webster, C. S. (2020). Effective Virtual Patient Simulators for Medical Communication Training: a Systematic Review. Med. Educ. 54, 786–795. doi:10.1111/medu.14152
Lehman, A. F., Lieberman, J. A., Dixon, L. B., McGlashan, T. H., Miller, A. L., Perkins, D. O., et al. (2004). Practice Guideline for the Treatment of Patients with Schizophrenia, Second Edition. Am. J. Psychiatry 161, 1–56.
Lenherr, G., Meyer-Zehnder, B., Kressig, R. W., and Reiter-Theil, S. (2012). To Speak, or Not to Speak-- Do Clinicians Speak about Dying and Death with Geriatric Patients at the End of Life? Swiss Med. Wkly. 142, w13563. doi:10.4414/smw.2012.13563
Lewin, S., Skea, Z., Entwistle, V. A., Zwarenstein, M., and Dick, J. (2001). Interventions for Providers to Promote a Patient-Centred Approach in Clinical Consultations. Cochrane database Syst. Rev. 12, CD003267. doi:10.1002/14651858.cd003267
Liaw, S. Y., Soh, S. L.-H., Tan, K. K., Wu, L. T., Yap, J., Chow, Y. L., et al. (2019). Design and Evaluation of a 3d Virtual Environment for Collaborative Learning in Interprofessional Team Care Delivery. Nurse Educ. today 81, 64–71. doi:10.1016/j.nedt.2019.06.012
Liaw, S. Y., Wu, L. T., Soh, S. L. H., Ringsted, C., Lau, T. C., and Lim, W. S. (2020). Virtual Reality Simulation in Interprofessional Round Training for Health Care Students: A Qualitative Evaluation Study. Clin. Simul. Nurs. 45, 42–46. doi:10.1016/j.ecns.2020.03.013
Lorié, Á., Reinero, D. A., Phillips, M., Zhang, L., and Riess, H. (2017). Culture and Nonverbal Expressions of Empathy in Clinical Settings: A Systematic Review. Patient Educ. Couns. 100, 411–424. doi:10.1016/j.pec.2016.09.018
Loveys, K., Sebaratnam, G., Sagar, M., and Broadbent, E. (2020). The Effect of Design Features on Relationship Quality with Embodied Conversational Agents: a Systematic Review. Int. J. Soc. Robotics 12, 1293–1312. doi:10.1007/s12369-020-00680-7
Lucas, G. M., Gratch, J., King, A., and Morency, L.-P. (2014). It's Only a Computer: Virtual Humans Increase Willingness to Disclose. Comput. Hum. Behav. 37, 94–100. doi:10.1016/j.chb.2014.04.043
Machiels, M., Metzelthin, S. F., Hamers, J. P. H., and Zwakhalen, S. M. G. (2017). Interventions to Improve Communication between People with Dementia and Nursing Staff during Daily Nursing Care: A Systematic Review. Int. J. Nurs. Stud. 66, 37–46. doi:10.1016/j.ijnurstu.2016.11.017
Madhu, D., Jain, C. N., Sebastain, E., Shaji, S., and Ajayakumar, A. (2017). “A Novel Approach for Medical Assistance Using Trained Chatbot,” in 2017 international conference on inventive communication and computational technologies (ICICCT) (Coimbatore, India: IEEE), 243–246. doi:10.1109/icicct.2017.7975195
Magai, C., Cohen, C. I., and Gomberg, D. (2002). Impact of Training Dementia Caregivers in Sensitivity to Nonverbal Emotion Signals. Int. Psychogeriatr. 14, 25–38. doi:10.1017/s1041610202008256
Mantovani, F., Castelnuovo, G., Gaggioli, A., and Riva, G. (2003). Virtual Reality Training for Health-Care Professionals. CyberPsychology Behav. 6, 389–395. doi:10.1089/109493103322278772
Marci, C. D., Ham, J., Moran, E., and Orr, S. P. (2007). Physiologic Correlates of Perceived Therapist Empathy and Social-Emotional Process during Psychotherapy. J. Nerv. Ment. Dis. 195, 103–111. doi:10.1097/01.nmd.0000253731.71025.fc
Mast, M. S., and Cousin, G. (2013). “The Role of Nonverbal Communication in Medical Interactions: Empirical Results, Theoretical Bases, and Methodological Issues,” in The Oxford Handbook of Health Communication, Behavior Change and Treatment Adherence (Oxford, UK: Oxford University Press), 38–53.
Maurage, P., and Campanella, S. (2013). Experimental and Clinical Usefulness of Crossmodal Paradigms in Psychiatry: an Illustration from Emotional Processing in Alcohol-Dependence. Front. Hum. Neurosci. 7, 394. doi:10.3389/fnhum.2013.00394
McCabe, R., Healey, P. G. T., Priebe, S., Lavelle, M., Dodwell, D., Laugharne, R., et al. (2013). Shared Understanding in Psychiatrist-Patient Communication: Association with Treatment Adherence in Schizophrenia. Patient Educ. Couns. 93, 73–79. doi:10.1016/j.pec.2013.05.015
McGilton, K. S., Boscart, V., Fox, M., Sidani, S., Rochon, E., and Sorin-Peters, R. (2009). A Systematic Review of the Effectiveness of Communication Interventions for Health Care Providers Caring for Patients in Residential Care Settings. Worldviews Evidence-Based Nurs. 6, 149–159. doi:10.1111/j.1741-6787.2009.00155.x
McNaughton, N., Ravitz, P., Wadell, A., and Hodges, B. D. (2008). Psychiatric Education and Simulation: a Review of the Literature. Can. J. Psychiatry 53, 85–93. doi:10.1177/070674370805300203
Mirheidari, B., Blackburn, D., Walker, T., Reuber, M., and Christensen, H. (2019). Dementia Detection Using Automatic Analysis of Conversations. Comput. Speech & Lang. 53, 65–79. doi:10.1016/j.csl.2018.07.006
Munshi, F., Lababidi, H., and Alyousef, S. (2015). Low- versus High-Fidelity Simulations in Teaching and Assessing Clinical Skills. J. Taibah Univ. Med. Sci. 10, 12–15. doi:10.1016/j.jtumed.2015.01.008
Nasir, J., Bruno, B., Chetouani, M., and Dillenbourg, P. (2021). What if Social Robots Look for Productive Engagement? Int J Soc Robotics 14, 55–71. doi:10.1007/s12369-021-00766-w
O'Brien, K. H. M., Fuxman, S., Humm, L., Tirone, N., Pires, W. J., Cole, A., et al. (2019). Suicide Risk Assessment Training Using an Online Virtual Patient Simulation. Mhealth 5, 31. doi:10.21037/mhealth.2019.08.03
O'Rourke, S. R., Branford, K. R., Brooks, T. L., Ives, L. T., Nagendran, A., and Compton, S. N. (2020). The Emotional and Behavioral Impact of Delivering Bad News to Virtual versus Real Standardized Patients: a Pilot Study. Teach. Learn Med. 32, 139–149. doi:10.1080/10401334.2019.1652180
Ochs, M., de Montcheuil, G., Pergandi, J.-M., Saubesty, J., Pelachaud, C., Mestre, D., et al. (2017). “An Architecture of Virtual Patient Simulation Platform to Train Doctors to Break Bad News,” in Conference on computer animation and social agents (casa).
Ochs, M., Jain, S., and Blache, P. (2018). “Toward an Automatic Prediction of the Sense of Presence in Virtual Reality Environment,” in Proceedings of the 6th International Conference on Human-Agent Interaction, 161–166.
Ochs, M., Mestre, D., De Montcheuil, G., Pergandi, J.-M., Saubesty, J., Lombardo, E., et al. (2019). Training Doctors' Social Skills to Break Bad News: Evaluation of the Impact of Virtual Environment Displays on the Sense of Presence. J. Multimodal User Interfaces 13, 41–51. doi:10.1007/s12193-018-0289-8
O’Connor, K., Neff, D. M., and Pitman, S. (2018). Burnout in Mental Health Professionals: A Systematic Review and Meta-Analysis of Prevalence and Determinants. Eur. Psychiatry 53, 74–99.
Okuda, Y., Bryson, E. O., DeMaria, S., Jacobson, L., Quinones, J., Shen, B., et al. (2009). The Utility of Simulation in Medical Education: what Is the Evidence? Mt. Sinai J. Med. 76, 330–343. doi:10.1002/msj.20127
Orange, J. (2001). “Family Caregivers, Communication, and Alzheimer’s Disease,” in Aging, Communication, and Health : Linking Research and Practice for Successful Aging (Mahwah, NJ: Lawrence Erlbaum), 225–248.
Orton, E., and Mulhausen, P. (2008). E-learning Virtual Patients for Geriatric Education. Gerontology geriatrics Educ. 28, 73–88. doi:10.1300/j021v28n03_06
Pan, X., and Hamilton, A. F. d. C. (2018). Why and How to Use Virtual Reality to Study Human Social Interaction: The Challenges of Exploring a New Research Landscape. Br. J. Psychol. 109, 395–417. doi:10.1111/bjop.12290
Pantziaras, I., Fors, U., and Ekblad, S. (2015). Training with Virtual Patients in Transcultural Psychiatry: Do the Learners Actually Learn? J. Med. Internet Res. 17, e46. doi:10.2196/jmir.3497
Pantziaras, I., Fors, U., and Ekblad, S. (2014). Virtual Mrs K: The Learners and #8217; Expectations and Attitudes towards a Virtual Patient System in Transcultural Psychiatry. J. Contemp. Med. Edu 2, 109–115. doi:10.5455/jcme.20140627042240
Parsons, K., Parsons, T., Pataki, C., Paton, M., St George, C., Sugar, J., et al. (2008). Virtual Justina: A Ptsd Virtual Patient for Clinical Classroom Training. Annu. Rev. CyberTherapy Telemedicine 6.
Parsons, T. D. (2021). Ethical Challenges of Using Virtual Environments in the Assessment and Treatment of Psychopathological Disorders. J. Clin. Med. 10, 378. doi:10.3390/jcm10030378
Pavic, K., Oker, A., Chetouani, M., and Chaby, L. (2020). EXPRESS: Age-Related Changes in Gaze Behavior during Social Interaction: an Eye-Tracking Study with an Embodied Conversational Agent. Q. J. Exp. Psychol. (Hove) 74, 1747021820982165–1139. doi:10.1177/1747021820982165
Peddle, M., Bearman, M., and Nestel, D. (2016). Virtual Patients and Nontechnical Skills in Undergraduate Health Professional Education: an Integrative Review. Clin. Simul. Nurs. 12, 400–410. doi:10.1016/j.ecns.2016.04.004
Pelachaud, C., Busso, C., and Heylen, D. (2021). “Multimodal Behavior Modeling for Socially Interactive Agents,” in The Handbook on Socially Interactive Agents: 20 Years of Research on Embodied Conversational Agents, Intelligent Virtual Agents, and Social Robotics Volume 1: Methods, Behavior, Cognition, 259–310. doi:10.1145/3477322.3477331
Philip, P., Micoulaud-Franchi, J. A., Sagaspe, P., Sevin, E., Olive, J., Bioulac, S., et al. (2017). Virtual Human as a New Diagnostic Tool, a Proof of Concept Study in the Field of Major Depressive Disorders. Sci. Rep. 7, 42656–7. doi:10.1038/srep42656
Philip, P., Dupuy, L., Morin, C. M., de Sevin, E., Bioulac, S., Taillard, J., et al. (2020). Smartphone-based Virtual Agents to Help Individuals with Sleep Concerns during Covid-19 Confinement: Feasibility Study. J. Med. Internet Res. 22, e24268. doi:10.2196/24268
Piette, J. D., Heisler, M., Krein, S., and Kerr, E. A. (2005). The Role of Patient-Physician Trust in Moderating Medication Nonadherence Due to Cost Pressures. Arch. Intern Med. 165, 1749–1755. doi:10.1001/archinte.165.15.1749
Piot, M. A., Dechartres, A., Attoe, C., Jollant, F., Lemogne, C., Layat Burn, C., et al. (2020). Simulation in Psychiatry for Medical Doctors: A Systematic Review and Meta‐analysis. Med. Educ. 54, 696–708. doi:10.1111/medu.14166
Pottle, J. (2019). Virtual Reality and the Transformation of Medical Education. Future Healthc. J. 6, 181–185. doi:10.7861/fhj.2019-0036
Priebe, S., Dimic, S., Wildgrube, C., Jankovic, J., Cushing, A., and McCabe, R. (2011). Good Communication in Psychiatry - a Conceptual Review. Eur. Psychiatr. 26, 403–407. doi:10.1016/j.eurpsy.2010.07.010
Provoost, S., Lau, H. M., Ruwaard, J., and Riper, H. (2017). Embodied Conversational Agents in Clinical Psychology: a Scoping Review. J. Med. Internet Res. 19, e151. doi:10.2196/jmir.6553
Quail, M., Brundage, S. B., Spitalnick, J., Allen, P. J., and Beilby, J. (2016). Student Self-Reported Communication Skills, Knowledge and Confidence across Standardised Patient, Virtual and Traditional Clinical Learning Environments. BMC Med. Educ. 16, 73–12. doi:10.1186/s12909-016-0577-5
Ramseyer, F., and Tschacher, W. (2011). Nonverbal Synchrony in Psychotherapy: Coordinated Body Movement Reflects Relationship Quality and Outcome. J. Consult. Clin. Psychol. 79, 284–295. doi:10.1037/a0023419
Ramseyer, F., and Tschacher, W. (2014). Nonverbal Synchrony of Head- and Body-Movement in Psychotherapy: Different Signals Have Different Associations with Outcome. Front. Psychol. 5, 979. doi:10.3389/fpsyg.2014.00979
Richardson, C. L., White, S., and Chapman, S. (2020). Virtual Patient Technology to Educate Pharmacists and Pharmacy Students on Patient Communication: a Systematic Review. BMJ Simul. Technol. Enhanc. Learn 6, 332–338. doi:10.1136/bmjstel-2019-000514
Riek, L. (2012). Wizard of Oz Studies in Hri: a Systematic Review and New Reporting Guidelines. Jhri 1, 119–136. doi:10.5898/jhri.1.1.riek
Riva, G., and Serino, S. (2020). Virtual Reality in the Assessment, Understanding and Treatment of Mental Health Disorders. J. Clin. Med. 9, 3434. doi:10.3390/jcm9113434
Rivière, E., Saucier, D., Lafleur, A., Lacasse, M., and Chiniara, G. (2018). Twelve Tips for Efficient Procedural Simulation. Med. Teach. 40, 743–751. doi:10.1080/0142159X.2017.1391375
Rizzo, A. S., and Koenig, S. T. (2017). Is Clinical Virtual Reality Ready for Primetime? Neuropsychology 31, 877–899. doi:10.1037/neu0000405
Rizzo, A. S., and Shilling, R. (2017). Clinical Virtual Reality Tools to Advance the Prevention, Assessment, and Treatment of Ptsd. Eur. J. Psychotraumatology 8, 1414560. doi:10.1080/20008198.2017.1414560
Robinson, K. E., Allen, P. J., Quail, M., and Beilby, J. (2020). Virtual Patient Clinical Placements Improve Student Communication Competence. Interact. Learn. Environ. 28, 795–805. doi:10.1080/10494820.2018.1552869
Rössler, W. (2012). Stress, Burnout, and Job Dissatisfaction in Mental Health Workers. Eur. archives psychiatry Clin. Neurosci. 262, 65–69.
Roter, D. L., Frankel, R. M., Hall, J. A., and Sluyter, D. (2006). The Expression of Emotion through Nonverbal Behavior in Medical Visits. Mechanisms and Outcomes. J. Gen. Intern Med. 21 (Suppl. 1), S28–34. doi:10.1111/j.1525-1497.2006.00306.x
Ruben, M. A., Blanch-Hartigan, D., and Shipherd, J. C. (2018). To Know Another's Pain: A Meta-Analysis of Caregivers' and Healthcare Providers' Pain Assessment Accuracy. Ann. Behav. Med. 52, 662–685. doi:10.1093/abm/kax036
Ruberton, P. M., Huynh, H. P., Miller, T. A., Kruse, E., Chancellor, J., and Lyubomirsky, S. (2016). The Relationship between Physician Humility, Physician-Patient Communication, and Patient Health. Patient Educ. Couns. 99, 1138–1145. doi:10.1016/j.pec.2016.01.012
Ruiz, J. G., and Leipzig, R. M. (2008). Geriasims: Falls Module. J. Am. Geriatrics Soc. 56, 130–131. doi:10.1111/j.1532-5415.2007.01480.x
Salari, N., Hosseinian-Far, A., Jalali, R., Vaisi-Raygani, A., Rasoulpoor, S., Mohammadi, M., et al. (2020). Prevalence of Stress, Anxiety, Depression Among the General Population during the Covid-19 Pandemic: a Systematic Review and Meta-Analysis. Glob. Health 16, 57–11. doi:10.1186/s12992-020-00589-w
Schneider, B., Scissons, H., Arney, L., Benson, G., Derry, J., Lucas, K., et al. (2004). Communication between People with Schizophrenia and Their Medical Professionals: a Participatory Research Project. Qual. Health Res. 14, 562–577. doi:10.1177/1049732303262423
Shah, H., Rossen, B., Lok, B., Londino, D., Lind, S. D., and Foster, A. (2012). Interactive Virtual-Patient Scenarios: an Evolving Tool in Psychiatric Education. Acad. Psychiatry 36, 146–50. doi:10.1176/appi.ap.10030049
Shattell, M. M., Starr, S. S., and Thomas, S. P. (2007). ?Take My Hand, Help Me Out?: Mental Health Service Recipients' Experience of the Therapeutic Relationship. Int. J. Ment. Health Nurs. 16, 274–284. doi:10.1111/j.1447-0349.2007.00477.x
Slater, P., Hasson, F., Gillen, P., Gallen, A., and Parlour, R. (2019). Virtual Simulation Training: Imaged Experience of Dementia. Int. J. Older People Nurs. 14, e12243. doi:10.1111/opn.12243
Staccini, P. M., and Fournier, J.-P. (2019). “Virtual Patients and Serious Games,” in Clinical Simulation (Amsterdam, Netherlands: Elsevier), 41–51. doi:10.1016/b978-0-12-815657-5.00004-8
Staccini, P. (2021). “Serious Games, Simulations, and Virtual Patients,” in Digital Innovations in Healthcare Education and Training (Amsterdam, Netherlands: Elsevier), 17–27. doi:10.1016/b978-0-12-813144-2.00002-7
Steel, Z., Marnane, C., Iranpour, C., Chey, T., Jackson, J. W., Patel, V., et al. (2014). The Global Prevalence of Common Mental Disorders: a Systematic Review and Meta-Analysis 1980-2013. Int. J. Epidemiol. 43, 476–493. doi:10.1093/ije/dyu038
Stepanikova, I., Zhang, Q., Wieland, D., Eleazer, G. P., and Stewart, T. (2012). Non-verbal Communication between Primary Care Physicians and Older Patients: How Does Race Matter? J. Gen. Intern Med. 27, 576–581. doi:10.1007/s11606-011-1934-z
Strasser, F., Palmer, J. L., Willey, J., Shen, L., Shin, K., Sivesind, D., et al. (2005). Impact of Physician Sitting versus Standing during Inpatient Oncology Consultations: Patients' Preference and Perception of Compassion and Duration. A Randomized Controlled Trial. J. pain symptom Manag. 29, 489–497. doi:10.1016/j.jpainsymman.2004.08.011
Stratou, G., Morency, L.-P., DeVault, D., Hartholt, A., Fast, E., Lhommet, M., et al. (2015). “A Demonstration of the Perception System in Simsensei, a Virtual Human Application for Healthcare Interviews,” in 2015 international conference on affective computing and intelligent interaction (ACII) (Xi'an, China: IEEE), 787–789. doi:10.1109/acii.2015.7344661
Stuckless, P., Hogan, M., and Kapralos, B. (2014). “Virtual Simulations and Serious Games in Community Health Nursing Education: a Review of the Literature,” in Virtual, Augmented Reality and Serious Games for Healthcare 1 (Berlin: Springer), 145–158. doi:10.1007/978-3-642-54816-1_8
Su, W.-S., and Chang, C.-Y. (2021). Virtual Patient in Interactive Learning Environments: a Review of 1989-2020 Publications in Selected SSCI Journals. Interact. Learn. Environ. 2021, 1–14. doi:10.1080/10494820.2021.1879873
Szilas, N., Chauveau, L., Andkjaer, K., Luiu, A. L., Bétrancourt, M., and Ehrler, F. (2019). “Virtual Patient Interaction via Communicative Acts,” in Proceedings of the 19th ACM International Conference on Intelligent Virtual Agents, 91–93. doi:10.1145/3308532.3329457
Talbot, T. B., and Rizzo, A. S. (2019). “Virtual Standardized Patients for Interactive Conversational Training,” in Exploring the Cognitive, Social, Cultural, and Psychological Aspects of Gaming and Simulations (Pennsylvania, USA: IGI Global), 62–86. doi:10.4018/978-1-5225-7461-3.ch003
Tan, T., Montague, E., Furst, J., and Raicu, D. (2020). “Robust Physician Gaze Prediction Using a Deep Learning Approach,” in 2020 IEEE 20th International Conference on Bioinformatics and Bioengineering (BIBE) (Cincinnati, OH, USA: IEEE), 993–998. doi:10.1109/bibe50027.2020.00168
Tan, Z. S., Mulhausen, P. L., Smith, S. R., and Ruiz, J. G. (2010). Virtual Patients in Geriatric Education. Gerontology geriatrics Educ. 31, 163–173. doi:10.1080/02701961003795813
Tanaka, H., Adachi, H., Ukita, N., Ikeda, M., Kazui, H., Kudo, T., et al. (2017a). Detecting Dementia through Interactive Computer Avatars. IEEE J. Transl. Eng. Health Med. 5, 1–11. doi:10.1109/jtehm.2017.2752152
Tanaka, H., Negoro, H., Iwasaka, H., and Nakamura, S. (2017b). Embodied Conversational Agents for Multimodal Automated Social Skills Training in People with Autism Spectrum Disorders. PloS one 12, e0182151. doi:10.1371/journal.pone.0182151
Templier, L., Chetouani, M., Plaza, M., Belot, Z., Bocquet, P., and Chaby, L. (2015). Altered Identification with Relative Preservation of Emotional Prosody Production in Patients with Alzheimer's Disease. Gériatrie Psychol. Neuropsychiatrie du Vieillissement 13, 106–115. doi:10.1684/pnv.2015.0524
Tomicic, A., Pérez, J. C., Martínez, C., and Rodríguez, E. (2017). Vocalization-Silence Dynamic Patterns: A System for Measuring Coordination in Psychotherapeutic Dyadic Conversations. Rev. Latinoam. Psicol. 49, 48–60. doi:10.1016/j.rlp.2016.09.004
Torres, M. I., Chollet, G., Montenegro, C., Tenorio-Laranga, J., Gordeeva, O., Esposito, A., et al. (2018). “Empathic, Expressive, Advanced Virtual Coach to Improve Independent Healthy-Life-Years of the Elderdy,” in IberSPEECH, 172–173.
van Manen, A. S., Aarts, S., Metzelthin, S. F., Verbeek, H., Hamers, J. P. H., and Zwakhalen, S. M. G. (2020). A Communication Model for Nursing Staff Working in Dementia Care: Results of a Scoping Review. Int. J. Nurs. Stud. 113, 103776. doi:10.1016/j.ijnurstu.2020.103776
Vougioukas, K., Petridis, S., and Pantic, M. (2020). Realistic Speech-Driven Facial Animation with Gans. Int. J. Comput. Vis. 128, 1398–1413. doi:10.1007/s11263-019-01251-8
Wang, I., and Ruiz, J. (2021). Examining the Use of Nonverbal Communication in Virtual Agents. Int. J. Human-Computer Interact. 37, 1648–1673. doi:10.1080/10447318.2021.1898851
Wang, R., DeMaria, S., Goldberg, A., and Katz, D. (2016). A Systematic Review of Serious Games in Training Health Care Professionals. Simul. Healthc. 11, 41–51. doi:10.1097/sih.0000000000000118
Williams, S., Weinman, J., and Dale, J. (1998). Doctor-patient Communication and Patient Satisfaction: a Review. Fam. Pract. 15, 480–492. doi:10.1093/fampra/15.5.480
Wolf, L., Dion, K., Lamoureaux, E., Kenny, C., Curnin, M., Hogan, M. A., et al. (2011). Using Simulated Clinical Scenarios to Evaluate Student Performance. Nurse Educ. 36, 128–134. doi:10.1097/nne.0b013e318216120b
Wullenkord, R., and Eyssel, F. (2020). Societal and Ethical Issues in Hri. Curr. Robot. Rep. 1, 85–96. doi:10.1007/s43154-020-00010-9
Xavier, J., Vignaud, V., Ruggiero, R., Bodeau, N., Cohen, D., and Chaby, L. (2015). A Multidimensional Approach to the Study of Emotion Recognition in Autism Spectrum Disorders. Front. Psychol. 6, 1954. doi:10.3389/fpsyg.2015.01954
Zagdoun, J., Chaby, L., Benamara, A., Urbiolla Gallegos, M.-J., and Chetouani, M. (2021). “Non-verbal Behaviors Analysis of Healthcare Professionals Engaged with a Virtual-Patient,” in Socially-Informed AI for Healthcare, ICMI’21, Montreal, Canada, October 18–22. doi:10.1145/3461615.3485442
Zoellner, L. A., Pruitt, L. D., Farach, F. J., and Jun, J. J. (2014). Understanding Heterogeneity in Ptsd: Fear, Dysphoria, and Distress. Depress Anxiety 31, 97–106. doi:10.1002/da.22133
Keywords: virtual and e-learning, virtual patient, geriatrics and gerontology, psychiatry, clinician-patient communication/relationship, non-verbal communication (NVC), embodied conversational agent (ECA), human-agent interaction (HAI)
Citation: Chaby L, Benamara A, Pino M, Prigent E, Ravenet B, Martin J-C, Vanderstichel H, Becerril-Ortega R, Rigaud A-S and Chetouani M (2022) Embodied Virtual Patients as a Simulation-Based Framework for Training Clinician-Patient Communication Skills: An Overview of Their Use in Psychiatric and Geriatric Care. Front. Virtual Real. 3:827312. doi: 10.3389/frvir.2022.827312
Received: 01 December 2021; Accepted: 25 May 2022;
Published: 29 June 2022.
Edited by:
Ali Oker, Université de Reims Champagne-Ardenne, FranceReviewed by:
Mariano Alcañiz, Universitat Politècnica de València, SpainJordi Vallverdu, Universitat Autònoma de Barcelona, Spain
Copyright © 2022 Chaby, Benamara, Pino, Prigent, Ravenet, Martin, Vanderstichel, Becerril-Ortega, Rigaud and Chetouani. This is an open-access article distributed under the terms of the Creative Commons Attribution License (CC BY). The use, distribution or reproduction in other forums is permitted, provided the original author(s) and the copyright owner(s) are credited and that the original publication in this journal is cited, in accordance with accepted academic practice. No use, distribution or reproduction is permitted which does not comply with these terms.
*Correspondence: Laurence Chaby, laurence.chaby@u-paris.fr