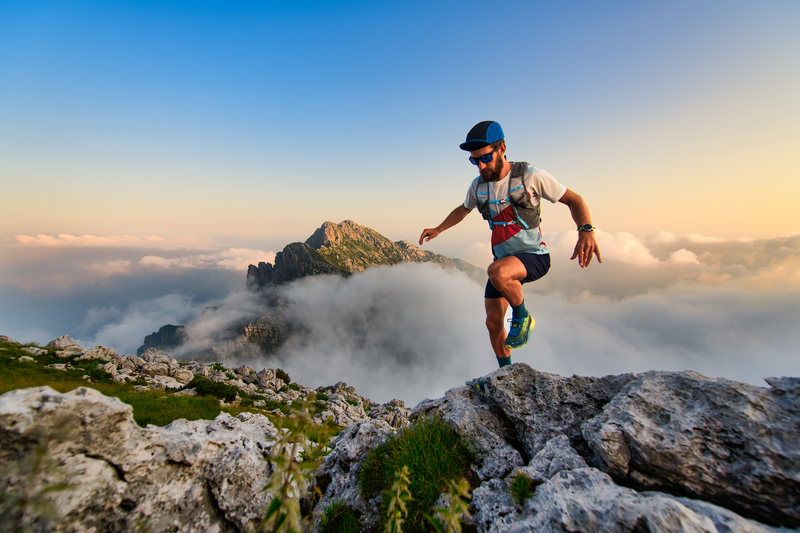
95% of researchers rate our articles as excellent or good
Learn more about the work of our research integrity team to safeguard the quality of each article we publish.
Find out more
ORIGINAL RESEARCH article
Front. Virtual Real. , 24 February 2022
Sec. Technologies for VR
Volume 3 - 2022 | https://doi.org/10.3389/frvir.2022.822189
This article is part of the Research Topic Eye movements in virtual environments View all 5 articles
Head mounted displays provide a good platform for viewing of immersive 360° or hemispheric images. A person can observe an image all around, just by turning his/her head and looking at different directions. The device also provides a highly useful tool for studying the observer’s gaze directions and head turns. We aimed to explore the interplay between participant’s head and gaze directions and collected head and gaze orientation data while participants were asked to view and study hemispheric images. In this exploration paper we show combined visualizations of both the head and gaze orientations and present two preliminary models of the relation between the gaze and the head orientations. We also show results of an analysis of the gaze and head behavior in relation to the given task/question.
Recent development of low cost eye trackers has meant a significant expansion in the research on practical utilization of gaze in various kind of situations. Some practical use cases for eye tracking are, for example, 1) usability studies where the user’s gaze behavior gives valuable information of which features the user is paying and not paying attention to (Jacob and Karn, 2003; Poole and Ball, 2006), 2) market research where gaze behavior is studied to learn what features in a product are noticed (Wedel and Pieters, 2008), and 3) as an input method for human-computer interfaces (Kangas et al., 2016; Morimoto and Mimica, 2005; Rantala et al., 2020). A special application area for gaze tracking has been setting up human-computer input methods for such disabled people who are unable to use other input technologies (Bates et al. (2007).
In interaction between humans the gaze directions are often important cues of interests and the flow of the interaction. Technologies are developed to share the gaze information in co-operation, especially on remote work as there is clear evidence that sharing the gaze improves the common situation understanding (Hanna and Brennan, 2007; Brennan et al., 2008). It has been shown that there are differences in gaze use between experts and novices (Law et al., 2004; Gegenfurtner et al., 2011), and gaze tracking may be beneficial in learning (Wilson et al., 2011). An interesting application for the gaze tracking is to observe indirectly the cognitive processes (Liversedge and Findlay, 2000; Just and Carpenter, 1976), such as in which order the person studies complicated environment or navigates social situations. Eye tracking can also be used for studying possible driver fatigue in automotive applications (Bretzner and Krantz, 2005), cognitive load (Biswas et al., 2016) or cognitive dysfunction, such as autism (Falck-Ytter et al., 2013). Overall, there are plenty of examples of useful applications of gaze tracking.
However, gaze trackers are still a special equipment and not always available. New low-power trackers may change that in future (Angelopoulos et al., 2020; Li et al., 2020). One proposal has been to use the head orientation as a proxy measure of the gaze orientation. There have been some studies (for example, Ohn-Bar et al. (2014) where the observer’s “gaze is inferred using head-pose and eye-state.” In our experiments we wanted to collect data of the gaze and head behaviour to study potential new methods to do the prediction of gaze orientation.
The coordination of head orientation in relation to gaze behavior has been extensively studied and a strong interplay of gaze and head control has often been demonstrated. See, for example, Guitton (1992) for a discussion of the basis of the coordination. In his review article Freedman (2008) reviewed the diversity of the coordination and lists several hypotheses of the neural control.
Many of the early studies used a simple stimulus that the participant was expected to look at, often only a single object appearing on an otherwise empty space. Such an arrangement may lead to somewhat one-sided visual behaviour that might be reflected in results about gaze and head coordination. Biguer et al. (1982) studied a system where a participant was to reach for an object and they showed that while the movements are sequentially ordered (eye, then head and finally arm), the onset of muscles for head and arm movements are synchronous with the eye movement. Goossens and Van Opstal (1997) studied how different stimulus modalities might affect the gaze and head coordination and their main results also supported the strong linkage of gaze and head movements.
In natural tasks the gaze and head coordination is affected by the predictability of the target. Kowler et al. (1992) demonstrated that in reading tasks the head may start returning to the beginning of next line before the gaze have reached the end of previous line. Similarly, Pelz et al. (2001) demonstrated that (in a well known visual environment) head movements showed considerable flexibility and were not tied to the gaze behavior, but were influenced by predictions of the future needs. In their words (Pelz and Canosa, 2001, p. 276): “Thus, the eye-head system is very flexible when the timing and goals of the movements are under the subject’s control.”
Land (1992) examined the oculomotor behaviour in driving situations, paying attention to situations where large gaze movements were needed, like at road junctions, and noticed that “The results show that the pattern of eye and head movements is highly predictable, given only the sequence of gaze targets.” Doshi and Trivedi (2012) were also studying the driving behaviour and found that there are different coordination strategies depending on situation. For example, they found a pattern of preparatory head motions before the gaze movement in task-oriented attention shifts. Zangemeister and Stark (1982a) and Zangemeister and Stark (1982b) noted that head and gaze coordination is modified by conditions such as “instructions to the subject, frequency and predictability of the target, amplitude of the movement, and development of fatigue.” Also Ron and Berthoz (1991), Guitton and Volle (1987) and Oommen et al. (2004) list situations where the order of gaze and head movements varies. Foulsham et al. (2011) and Hart et al. (2009) demonstrated that the participant’s gaze behavior is highly context dependent by studying the gaze behavior while moving in the world versus seeing the same or similar video views while in a laboratory keeping their head still. The gaze behavior seems to be more concentrated in real world context than in the laboratory. They didn’t study the dynamics between the head and gaze behavior, however.
Sitzmann et al. (2018) collected gaze and head orientation data on participants exploring stereoscopic omni-directional panoramas in virtual reality (VR) setting. The target of the study was mainly to analyze the working of visual saliency predictors, but the data also provided some insights to head and gaze dynamics. We define the visual saliency as the distinct subjective perceptual quality which makes some items in the world stand out from their neighbors and immediately grab our attention (Itti, 2019). Rai et al. (2017) and David et al. (2018) collected two databases of gaze and head orientations where a large number of participants were viewing either 360° images (Rai et al., 2017) or 360° videos (David et al., 2018) using head mounted displays (HMD). Their target was to provide test data and analysis tools to enable the study of the visual saliency in VR environment. Hu et al. studied the natural head moves (Hu et al., 2017) and then the correlations between head and gaze moves (Hu et al., 2018) while studying complex scenes in HMD. They were not recording the head and gaze data during the same session, though, but the correlation was between cumulative data from separate sessions.
Sidenmark and Gellersen (2019b, 2019b) have studied the coordination of head and gaze orientations for enabling fast and convenient gaze interaction mechanisms. In Sidenmark and Gellersen (2019b) the gaze and head were both used to select a location in a view. The dynamics between the head and gaze was then intentional as the user was trying to activate a target. A related method was one option in Kytö et al. (2018) where gaze was used for selection and gaze point error could be corrected by a deliberate head move. The natural behavior or head, gaze and torso directions and timing differences were studied in Sidenmark and Gellersen (2019a) based on measurements on when the respective moves have started and how the fixation directions differ.
Hu et al. (2019) collected gaze and head orientation data from a number of participants viewing 360° virtual worlds using HMD and a gaze tracker. The participants were able to freely navigate in the scenes. The purpose of the study was to develop and evaluate a real-time gaze prediction model based on the head data. The final model by Hu et al. was based on head angular velocities and not on head orientation.
An important limitation in using the HMD devices and studying the gaze behavior is the fact that the HMD field-of-view is naturally limited by the display size. It has been shown that this has a natural effect in the gaze and head behavior (Kollenberg et al., 2010; Pfeil et al., 2018) when studying natural scenes which are larger than the display size. In our study we targeted only for HMD use. The studies on HMD use are important as the near-eye displays will improve and become more common (Koulieris et al., 2019; Konrad et al., 2020; Padmanaban et al., 2017; Kim et al., 2019).
While it has been shown that the coordination of gaze and head is highly dependent on external factors, like the subject’s expectations regarding future directions of gaze, we were interested in understanding the gaze-head behaviour in our special case, the viewing of images on head mounted displays. There have been studies where gaze and head data has been collected using head mounted displays (e.g., Sitzmann et al., 2018; Rai et al., 2017; David et al., 2018) but the main interest has been on analyzing visual saliency, while we were more interested on the general gaze and head dynamics. Also, Hu et al. (2019) were studying gaze-head behaviour when using head mounted displays, but using virtual world scenarios where the participants could freely navigate.
Our main target was to study the possibility of constructing a gaze predictor based on head behaviour. We expected that there would be some similarities to the results by Land (1992) (i.e., more restricted, “simple” gaze-head coordination). If the linkage between gaze and head direction was indeed found rather strong, we could hope for a successful gaze estimation based on the measurements of head coordination.
We decided to collect a database of gaze and head orientations where the participants were looking at a set of hemispherical images (the front half of a spherical image all around you, a 180° view of an image). The preliminary reason to collect the data was to be able to visualize and to analyze the interplay between the head and gaze orientations, i.e., to see if we can create some interesting models of the connection between gaze and head orientations. We expected to find simple relations between these two parameters.
We were also interested in knowing if the context of the image viewing has an effect on the head and gaze interplay. Such an effect has been studied for gaze only (Rayner., 2009; Torralba et al., 2006; Bulling et al., 2009), but to our knowledge nobody has studied the context recognition using the co-operation of the head and gaze directions for image viewing. Separately, we were then interested to make experiments on some measures to see if different tasks/contexts given to the image viewers would noticeably affect their viewing behavior.
Ishimaru et al. (2014) have studied the combination of head motion and eye blinks for activity recognition. Earlier it has also been shown that the gaze path can be used to infer task-based information (see, e.g., Coutrot et al., 2018), as well as the distributions of fixations on salient points to infer the task given to observers (Koehler et al., 2014). Therefore, we decided to do the data collection so that different participants were given different reasons to look at any specific image. For every image viewing we asked the participant a question about the image, or in some cases just asked him/her to look at the image. By analyzing possible differences in viewer behaviors we expected to notice some context effect.
Overall, the main purpose of our experiment was to do an exploratory study and try to find out some promising directions for more extensive future studies.
The studies in this paper are exploratory in nature and we were looking for potential effects and interplay of head and gaze orientations in different viewing tasks. The contribution of this paper is fourfold: 1) We report a data collection effort of both head and gaze orientation of a number of participants when viewing hemispheric images in a fixed virtual reality environment. 2) We show two related new visualizations of the interplay between head and gaze orientations. 3) We report studies of two models of co-ordination between the head orientation and gaze orientation when viewing hemispheric images. 4) We report studies of two measures of gaze and head behaviour to separate between different viewing tasks given to the participants.
We recruited 31 volunteer participants (12 females, 19 males) from university students and personnel. The age distribution was as follows: 3 participants up to 24 years, 15 in the range 25–34, 8 in the range 35–44, 3 in the range 45–54, and 2 older participants. Of the 31 participants 16 had normal vision, 15 had corrected-to-normal vision, 9 of which wore glasses while using the virtual reality head mounted displays.
In the experiment we used a Windows PC, an HTC Vive VR headset with an built-in Tobii gaze tracker, and a custom VR experience player application (Järvenpää et al., 2017) to render the images and to collect the head and gaze data. The application was developed using the Unity platform. The application has a wide cross-platform VR and gaze tracker system support and has been used in various VR experience evaluations. The HTC Vive VR headset had a 110° field of view and had 1440 × 1600 pixels per eye. The Tobii gaze tracker had a reported accuracy of 0.5 – 1.1. The original 180° images had been captured with Canon 6D digital camera with 3600 × 3600 pixels resolution and upscaled to 4096 × 4096 pixels for the player application.
The participant had no control devices other than the “head-gaze” pointer (head pointer) in the headset. The facilitator was using a separate mouse device to control the progress of the experiment software and to confirm the answers selected by the participant. The gaze and head orientation data was collected using a 75 Hz sampling rate. For the head orientation information we captured the direction of the head pointer often available on head mounted display applications for controlling the user interface, even though it was not visible for the participant at the time of viewing (see Section 5.2 for a discussion on the head orientation data).
In the experiment all the participants were sequentially viewing six hemispherical images using a head mounted display device, one image at a time. In the background of Figure 1 one can see an example of the images, in equirectangular projection. For each image viewing the participants were first asked a question or just asked to look at the image. After 40 s of viewing (a predefined fixed length of time) the participants had to answer a question using a multiple choice format, or they were asked to continue to the next image if there was no question. All the questions and choices were shown on the head mounted display.
FIGURE 1. Gaze points (red) and head orientations (blue) collected from one participant while looking at an image for 40 s. The path of the head orientation consists of separate dots (like the more clustered gaze path) but due to the head turning relatively slowly, the adjacent dots are rather close to each other. For viewing this image this participant was not given any specific task.
In the experiment we were giving the participants different motivations for viewing the images. The task of the viewer was either to find an answer to a given question or to just look at the image freely. For analysis purposes we defined four categories, consisting of one free viewing category plus three different question categories. All the questions that we used in the experiment are listed in Table 1.
TABLE 1. All the different questions in the three question categories and the single statement used in the experiment.
For clarifying the research work we formulated the following research questions (RQ1-3) to guide our work:
1. What is the relation between head and gaze orientations in image viewing?
2. Is it possible to estimate the gaze orientation from the head orientation?
3. How does the free viewing category differ in head and gaze orientation data from other categories?
For testing purposes, we also had the following hypotheses (H1-2) that we could try out with the final data concerning our use case:
1. The participants would turn their gaze before their head when looking around.
2. The measurements of head movement can be used to improve the estimate of gaze direction.
Upon arrival, the participant was introduced to the study and to the equipment. The participant was seated on a fixed (non-revolving) chair that would guide the participant to keep facing the same direction throughout the experiment. The images would be pointed so that the exact center of the image would always stay in front of the viewer while sitting straight on the chair.
The participant would next put the head mounted display on and the facilitator would start the data collection program. First the participant was shown one example image that was mainly used to demonstrate how the hemispheric images look like and what is the extent of the image to the right and left, up and down. During that time the participant and the facilitator were also able to observe how well the display fit for the participant. After observing the example image the integrated gaze tracker was calibrated using the tracker manufacturer provided routines. After tracker calibration the participant was asked for some demographic information (age group, gender and vision), which were all answered inside the head-mounted-display. To select an answer, the participant moved the head pointer to the right choice visible at that stage. After all of that had been completed the head pointer was hidden and the real data collection started.
The data collection consisted of six question/image viewing tasks. For each task the participant was displayed one question/statement (see Table 1 for a full list) and after confirmation that s/he had understood the question the hemispherical image was shown. The image was displayed for 40 s, irrespective if the participant was ready with an answer or not. In deciding the length of viewing we wanted to have a fixed duration long enough for all the participants. After the 40 s had passed the image disappeared and the participant was shown the possible answers (multiple choice format) to the question among which s/he then made the selection. For the statement (“Just look … ”) the participant did not answer but proceeded to the next task. All the participants were shown the same six images, but there were several alternative questions/statements for each image and only one was shown to each participant. Each question/statement was used equally often during the experiment.
After going through all the six images, the experiment was over and the participant was instructed to remove the display. The facilitator was available for answering any possible questions about the experiment, but no other data was collected. The whole experiment took less than 30 min per participant. Simulator sickness, which might affect this kind of experiments, was not measured but none of the participants mentioned any such symptoms.
For visual analysis of the collected data we ended up using two different visualization methods. For the first method all the gaze points and head directions were mapped as separate dots on top of the equirectangular image that the participant had been viewing. One example of such a visualization is shown in Figure 1. In the example the participant has been mainly viewing the horizontal street level area, left and right, with some glances up and down.
For the second visualization method the dots of the head orientations were connected to respective gaze points by a thin line to emphasize the timing relation of the head and gaze behavior. One example of that type of visualization is shown in Figure 2. In the image one can observe, for example, how the head is occasionally moving while the gaze remains fixed.
FIGURE 2. Same data as in Figure 1 but here the head points (blue) are connected to their respective gaze points by (green) lines to emphasize the timing of the data points in the two sequences. One can notice that the gaze points on the image sides are generally farther away from the center of the image compared to the respective head points, especially when the head had to be turned more to the sides.
We used a Monte Carlo permutation test (Dugard, 2014; Edgington and Onghena, 2007; Howell, 2007; Nichols and Holmes, 2001) to analyze possible statistically significant differences between different parameter sets. The permutation test is not dependent on as many assumptions on the sample distribution as some other tests such as ANOVA Dugard (2014), especially as the test sample need not be normally distributed. Also, we were using median values as the test statistic, while some other methods can only use the mean. Compared to the mean, median is more tolerant to outliers in the data.
In all tests an observed value of a measurement is compared against a distribution of measurements produced by resampling a large number of sample permutations assuming no difference between the sample sets (null hypothesis). The relevant p-value is then given by the proportion of the distribution values that is more extreme or equal than the observed value. To get the distribution of measurements assuming no difference between the conditions, we pooled the sample set values from both conditions and resampled from that generating 10,000 permutations to be measured.
While the gaze data directly indicates the direction of interest in the image, the head orientation is not as easy to interpret. First, the head pointing direction is defined relative to the head-mounted-display itself. And while the display rather naturally fits to the wearer’s face, there is no guarantee of the orientation. For example, the display might be slightly tilted because of head geometry, which is difficult to correct. Second, some participants habitually tilt their head slightly forward or backward, which would mean that the head pointing data on average is slightly below or above a “neutral” level defined by collecting and averaging similar data from a large number of participants.
To study the differences in the head and gaze direction distribution for vertical direction we analyzed the collected data of all participants viewing all the images, i.e., 186 viewings (31 participants each viewing 6 images). For each viewing separately we calculated the median values in vertical direction both for gaze directions and head directions, and then collected the histogram of their difference values. For the results see Figure 3 where positive values would mean head point being above the gaze point. The median value for the difference was −6.2°, i.e., the head pointer for most cases stayed slightly lower than their gaze point, on average. In Figure 1 one can observe that on the main horizontal area, at least, the head points (blue) are slightly below the gaze points (red) following the general trend. The vertical difference values for one image (shown in Figure 1) has been collected into Figure 4.
FIGURE 3. A histogram of the differences between the median values in vertical direction for gaze directions and head directions, for all six images and all participants. The median difference value was − 6.2°.
FIGURE 4. A histogram of the difference values between the centers of gaze and head orientations in vertical direction for one image. The displayed image was the same as in Figure 1. For the participant in Figure 1 the difference was − 8.0° while the median value for all participants was − 6.7°.
We separately computed the median vertical difference values for all six images over all participants. The median values varied from − 5.2° to − 6.8° depending on the image, which values are rather tightly clustered around the median value of all the data shown in Figure 3.
For the data analysis part we decided to compensate for the head and gaze directions center difference in vertical direction. We assume that there is such a value for the difference that we can compute from extensive enough material and then use as compensation for aligning (vertically) the head orientation values and the gaze orientation values to avoid that consistent bias. In the following studies we corrected the difference in our data using an image specific median difference value collected over all participants. An alternative might be to use person dependent correction that would be measured using a calibration type system in the beginning, or a global value that would be measured for large number of images and participants once. For example, for the data in Figure 1 we slightly (6.7°, the median value for all participants for that image) raised the head orientation data to align better with the gaze orientations.
Looking at the head and gaze data more closely, one notices that the gaze direction often changes first and the head direction follows a bit later (hinting towards the hypothesis H1). That makes intuitive sense as the gaze is easy to re-orient to different directions while head movements take more time.
Given the clear tendency that the gaze movement will happen slightly before the head movement we used the calculation of an average distance between the gaze direction and the (normalized as described in Section 5.2) head direction as a measure to optimize, trying out different delay values. The basic method of average distance computation with small delays is shown in the Eq. 1 where G is the sequence of gaze points, H is the sequence of the head points, N is the number of samples in the whole sequence, and d ∈ [0, … , M] is the delay value counted in samples.
An example of the result values given by the distance value computation for different delays is shown in Figure 5. The average distance first decreases when the delay is growing, reaches a minimum and then starts increasing again when the delay continues growing. The optimal delay is participant and image dependent but in these experiments most of the measured values were between 100 and 300 milliseconds. We collected all the measured optimal delays into Figure 6 with an overall median value of 211 milliseconds. In Sitzmann et al. (2018) the average delay was measured to be 58 ms, but as noted (Section 2), there is a large variability between the measures depending on the task of viewing among many other factors. Based on these results we can claim that the hypothesis H1 (The participants would turn their gaze before their head when looking around.) is plausible.
FIGURE 5. The average distance between gaze point and head point using different delay values for one example data. The minimum value of the distance can be found to be around 280 ms delay in this specific case.
FIGURE 6. The histogram of the best delay values for each participant and all images. The median value 211.0 is marked by a red vertical line.
If there is no gaze tracker available in a head mounted display to get information of the gazed point, for certain purposes it is possible to substitute the gaze direction with the head direction. We introduced a very simple model to get a slightly better estimate of the true gaze direction from the head direction than by just using the head direction as such. The model (see Eq. 2) was based on the observation that as the participant was turning his/her head the gaze direction usually moved farther away from the center than the head direction. I.e., by assuming that the very center of the viewing area is the center of our coordinate system, the absolute values of both the x and y coordinates grow when the head is turned and the (absolute values of) the gaze coordinates are slightly larger than the head coordinates, on average. The center of the viewing area can be defined for certain very common viewing situations in virtual reality, which are similar to the one used in our studies. For example, in many driving and control room simulations the user is continually facing one fixed direction while s/he is able to look around. The estimate of the gaze position EG = (xG, yG) is computed by applying a fixed multiplier m to both x and y coordinates of the head point H:
We selected a simple linear model for simplicity and we don’t assume that it would be the best choice in all viewing areas.
To measure the effect of the model parameter m in the gaze point estimation, we calculated the total distance between the collected gaze points and the estimated gaze points using a similar equation as above (Eq. 1), replacing the head orientation H by the gaze estimate EG, fixing the delay parameter d to one given value and varying the multiplier m:
where G is the sequence of gaze points, EG is the sequence of the gaze estimates, N is the number of samples in the whole sequence, and d ∈ [0, … , M] is the delay value counted in samples. In the following calculations we used the median delay value do = 211.0 (as defined in previous calculations, see Figure 6).
One example of the distance value computation for different multiplier values m is shown in Figure 7. The optimal multiplier value m in this example is a little less than 1.1. We collected all the measured optimal multipliers into Figure 8 with the overall median multiplier value of 1.175.
FIGURE 7. The average distance between the measured gaze point and the estimated gaze point using the simple multiplicative model using different parameter values (see Eq. 2) for the same example data as in Figure 5. The average distance between gaze and head orientations calculated without any corrections (no delay correction, no position estimation) is shown as a green horizontal line for comparison.
FIGURE 8. The histogram of the best fitting multiplier values for each participant and all images. The median value 1.175 is marked by the red vertical line.
As seen in the Figure 7 the average distance between the head points and gaze points is clearly shorter (around 2.2° shorter) using the optimal parameter values compared to the average distance computed without any corrections (marked with the green line). We can answer the research question RQ2 (Is it possible to estimate the gaze orientation from the head orientation?) that while it is not possible to get the gaze orientation as such from head orientation we can improve the estimate using our models. In Figure 9 we show the histogram of the improvement values using the model for all images and all participants. As we were using common parameter values that were based on analysis of all the data (not tailored for each sample separately) there were cases where the average distance was growing, sometimes quite a lot. However, in the vast majority of cases the average distance decreased and the median improvement was found to be 2.3°. Therefore, based on these results we claim that the hypothesis H2 (The measurements of head movement can be used to improve the estimate of gaze direction.) is plausible.
FIGURE 9. The histogram of the changes in the median distances between gaze point and the estimated gaze point based on the simple multiplier model for each participant and all images. The median value − 2.3° of the changes is marked by the red vertical line. The median distance between the gaze point and head direction before the model use was 12.6°.
We also performed data analysis to automatically separate the samples by task categories (see Table 1). The method was to compute a measure of the gaze and/or head orientation data and to observe if there were statistically significant differences between the measured values for different categories. In the following we go through the results generated by using two measures developed for this experiment.
To see if the question category would affect the effect of head following the gaze we tried the time delay parameter that was introduced in Section 5.3. We computed the best fitting time delay parameter for each sample separately and divided them to different groups by the task category. To find any statistically significant differences we ran a permutation test (as explained in Section 5.1) for each category pair. As there were six different category pairs we used the Bonferroni corrected p-value limit 0.008. We found only one category pair (between Look and Analyze, p-value 0.003) where the distribution was statistically significantly different, the delay value was longer for category Look than for Analyze.
To see if the question category would affect the relative amounts of gaze and head movements to the sides we tested the distance multiplier that was introduced in Section 5.4. We computed the best fitting multiplier for each sample separately and divided them to different categories. Running the permutation tests we observed that there were no statistically significant differences between the categories.
We can now answer the research question RQ3 (How does the free viewing category differ in head and gaze orientation data from other categories?) by not having clear differences on that data.
The results of these experiments using the head mounted displays and virtual reality material again demonstrate (see, e.g., Freedman, 2008) that there exists an interesting interplay between the head and gaze directions. The presented visualizations (especially Figures 1, 2) and the developed models between head and gaze pointing show that there are structures in the data that can be utilized in making predictions of the use of gaze and in analyzing the context effects.
The correction term of the head orientation vectors (Section 5.2) was based on collecting the difference values from all the available participants for viewing one specific image. The correction term should preferably be determined for each participant separately but in this study we were lacking data. In future studies we need to collect more data from each individual participant, viewing many more images.
The delay term (Section 5.3) was calculated using the whole sample material (40 s of viewing). It is to be expected that if we would take shorter segments of the data at a time the optimal delay values would be different depending on, for example, if the viewer had been studying some object in detail or glancing around for a general view. In earlier studies the delay has varied and even the order of head and gaze movements have changed because of context effects (e.g., Zangemeister and Stark, 1982a; Zangemeister and Stark, 1982b). Hu et al. (2019) found that the delay might be different in vertical and horizontal movements, and computed from their data a 140 ms delay of a head movement for horizontal and a 70 ms delay for vertical directions. In future studies we need to study the delay in more detail, to see if that could be used for context recognition, say.
Our model of calculating the gaze point estimate from head point (Section 5.4) was extremely simple and didn’t consider, for example, the time development of the head point. Taking into account a short history of the head movements would be a natural way of extending the model. Hu et al. (2019) based their model on head velocities only. A combination of head orientation and head velocity would be a natural extension for the model. Also, the current model is the same in all image areas. It is possible that different areas might be better modeled with slightly different functional forms. For example, the extreme sides of the viewing area probably require somewhat different models than the very center. However, we can answer the research question RQ2 (Is it possible to estimate the gaze orientation from the head orientation?) that we can improve the estimate of gaze point using our models.
The measures that we developed for the context recognition (Section 5.5) didn’t separate the different question categories but only in one case, which means that we answer the research question RQ3 (How does the free viewing category differ in head and gaze orientation data from other categories?) by not having differences. One reason for that could be that as the data was collected for so long time some participants spent only a small part of the time answering the question and then moved to “just look at the image”-mode similar to the free viewing category. Then the measures would also look the same between the categories.
The work has been exploratory in nature and the reported results point an interesting direction for promising future studies.
In this paper we have demonstrated interesting interplay between the gaze movements and the head movements. The head movements, on average, happen slightly later than the respective gaze movements. We measured an average delay of 211 milliseconds for our samples. The gaze direction are, on average, slightly farther away from the center of an image (“forward” direction) than the head directions, i.e., the head turns slightly less than the gaze. We measured an optimal multiplier value 1.175 for our material, which means that the gaze is 17.5% more turned from the center of the view than the head, on average. Using measures calculated from the gaze and head data we were showing that there was significant difference between some question categories.
For the research question RQ1 (Section 4.3) our answer is described above. For the research questions RQ2 and RQ3 we found that we can improve the estimate of gaze orientation using our models and head orientation data, and that the free viewing category does not differ from other categories on our data. Both of our hypotheses (H1-2) are plausible.
The raw data supporting the conclusion of this article will be made available by the authors, without undue reservation.
Conceptualization, JK, OŠ, OK, TJ, MS, and RR. Methodology, JK, OK and TJ. Software, OK and TJ. Investigation, JK and OK. Resources, RR. Data curation, JK. Writing—original draft preparation, JK. Writing—review and editing, JK, OŠ, OK, TJ, MS, and RR. Visualization, JK. Supervision, RR. Project administration, RR. Funding acquisition, RR All authors have read and agreed to the published version of the manuscript.
This work has received funding from the Academy of Finland, Augmented Eating Experiences project (Grant 316804).
The authors declare that the research was conducted in the absence of any commercial or financial relationships that could be construed as a potential conflict of interest.
All claims expressed in this article are solely those of the authors and do not necessarily represent those of their affiliated organizations, or those of the publisher, the editors and the reviewers. Any product that may be evaluated in this article, or claim that may be made by its manufacturer, is not guaranteed or endorsed by the publisher.
We would like to thank the members of TAUCHI research unit for their help in preparing the experiment and the paper.
Angelopoulos, A. N., Martel, J. N. P., Kohli, A. P., Conradt, J., and Wetzstein, G. (2021). Event-Based Near-Eye Gaze Tracking beyond 10,000 Hz. IEEE Trans. Vis. Comput. Graphics 27, 2577–2586. arXiv preprint arXiv:2004.03577. doi:10.1109/TVCG.2021.3067784
Bates, R., Donegan, M., Istance, H. O., Hansen, J. P., and Räihä, K.-J. (2007). Introducing Cogain: Communication by Gaze Interaction. Univ. Access Inf. Soc. 6, 159–166. doi:10.1007/s10209-007-0077-9
Biguer, B., Jeannerod, M., and Prablanc, C. (1982). The Coordination of Eye, Head, and Arm Movements during Reaching at a Single Visual Target. Exp. Brain Res. 46, 301–304. doi:10.1007/bf00237188
Biswas, P., Dutt, V., and Langdon, P. (2016). Comparing Ocular Parameters for Cognitive Load Measurement in Eye-Gaze-Controlled Interfaces for Automotive and Desktop Computing Environments. Int. J. Human-Computer Interaction 32, 23–38. doi:10.1080/10447318.2015.1084112
Brennan, S. E., Chen, X., Dickinson, C. A., Neider, M. B., and Zelinsky, G. J. (2008). Coordinating Cognition: The Costs and Benefits of Shared Gaze during Collaborative Search. Cognition 106, 1465–1477. doi:10.1016/j.cognition.2007.05.012
Bretzner, L., and Krantz, M. (2005). “Towards Low-Cost Systems for Measuring Visual Cues of Driver Fatigue and Inattention in Automotive Applications,” in IEEE International Conference on Vehicular Electronics and Safety, Shaanxi, China, October 2005, 161–164. doi:10.1109/ICVES.2005.1563634
Bulling, A., Ward, J. A., Gellersen, H., and Tröster, G. (2009). “Eye Movement Analysis for Activity Recognition,” in Proceedings of the 11th International Conference on Ubiquitous Computing, Orlando Florida USA, 30 September-3 October 2009 (New York, NY, USA: ACM), 41–50. UbiComp ’09. doi:10.1145/1620545.1620552
Coutrot, A., Hsiao, J. H., and Chan, A. B. (2018). Scanpath Modeling and Classification with Hidden Markov Models. Behav. Res. 50, 362–379. doi:10.3758/s13428-017-0876-8
David, E. J., Gutiérrez, J., Coutrot, A., Da Silva, M. P., and Callet, P. L. (2018). “A Dataset of Head and Eye Movements for 360° Videos,” in Proceedings of the 9th ACM Multimedia Systems Conference, Amsterdam Netherlands, June 2018 (New York, NY, USA: ACM), 432–437.
Doshi, A., and Trivedi, M. M. (2012). Head and Eye Gaze Dynamics during Visual Attention Shifts in Complex Environments. J. Vis. 12, 9. doi:10.1167/12.2.9
Dugard, P. (2014). Randomization Tests: a New Gold Standard? J. Contextual Behav. Sci. 3, 65–68. doi:10.1016/j.jcbs.2013.10.001
Falck-Ytter, T., Bölte, S., and Gredebäck, G. (2013). Eye Tracking in Early Autism Research. J. Neurodevelop Disord. 5, 28. doi:10.1186/1866-1955-5-28
Foulsham, T., Walker, E., and Kingstone, A. (2011). The where, what and when of Gaze Allocation in the Lab and the Natural Environment. Vis. Res. 51, 1920–1931. doi:10.1016/j.visres.2011.07.002
Freedman, E. G. (2008). Coordination of the Eyes and Head during Visual Orienting. Exp. Brain Res. 190, 369–387. doi:10.1007/s00221-008-1504-8
Gegenfurtner, A., Lehtinen, E., and Säljö, R. (2011). Expertise Differences in the Comprehension of Visualizations: A Meta-Analysis of Eye-Tracking Research in Professional Domains. Educ. Psychol. Rev. 23, 523–552. doi:10.1007/s10648-011-9174-7
Goossens, H. H. L. M., and Opstal, A. J. V. (1997). Human Eye-Head Coordination in Two Dimensions under Different Sensorimotor Conditions. Exp. Brain Res. 114, 542–560. doi:10.1007/pl00005663
Guitton, D. (1992). Control of Eye-Head Coordination during Orienting Gaze Shifts. Trends Neurosciences 15, 174–179. doi:10.1016/0166-2236(92)90169-9
Guitton, D., and Volle, M. (1987). Gaze Control in Humans: Eye-Head Coordination during Orienting Movements to Targets within and beyond the Oculomotor Range. J. Neurophysiol. 58, 427–459. doi:10.1152/jn.1987.58.3.427
Hanna, J. E., and Brennan, S. E. (2007). Speakers' Eye Gaze Disambiguates Referring Expressions Early during Face-To-Face Conversation. J. Mem. Lang. 57, 596–615. doi:10.1016/j.jml.2007.01.008
Hart, B. M., Vockeroth, J., Schumann, F., Bartl, K., Schneider, E., König, P., et al. (2009). Gaze Allocation in Natural Stimuli: Comparing Free Exploration to Head-Fixed Viewing Conditions. Vis. Cogn. 17, 1132–1158. doi:10.1080/13506280902812304
Hu, B., Johnson-Bey, I., Sharma, M., and Niebur, E. (2018). “Head Movements Are Correlated with Other Measures of Visual Attention at Smaller Spatial Scales,” in Proceedings of the 2018 52nd Annual Conference on Information Sciences and Systems (CISS), Princeton, NJ, USA, May 2018, 1–6. doi:10.1109/CISS.2018.8362264
Hu, B., Johnson-Bey, I., Sharma, M., and Niebur, E. (2017). “Head Movements during Visual Exploration of Natural Images in Virtual Reality,” in Proceedings of the 2017 51st Annual Conference on Information Sciences and Systems (CISS), Baltimore, MD, USA, March 2017, 1–6. doi:10.1109/ciss.2017.7926138
Hu, Z., Zhang, C., Li, S., Wang, G., and Manocha, D. (2019). Sgaze: A Data-Driven Eye-Head Coordination Model for Realtime Gaze Prediction. IEEE Trans. Vis. Comput. Graphics 25, 2002–2010. doi:10.1109/TVCG.2019.2899187
Ishimaru, S., Kunze, K., Kise, K., Weppner, J., Dengel, A., Lukowicz, P., et al. (2014). “In the Blink of an Eye: Combining Head Motion and Eye Blink Frequency for Activity Recognition with Google Glass,” in Proceedings of the 5th augmented human international conference, Kobe Japan, March, 2014 (New York, NY, United States: ACM), 15.
Jacob, R. J. K., and Karn, K. S. (2003). “Eye Tracking in Human-Computer Interaction and Usability Research,” in The Mind’s Eye. Editor O. Sacks (Amsterdam, Netherlands: Elsevier), 573–605. doi:10.1016/b978-044451020-4/50031-1
Järvenpää, T., Eskolin, P., and Salmimaa, M. (2017). “Vr Experience Player for Subjective Evaluations of Visual Vr Content,” in Proceedings of the IDW International Display Workshop, Sendai, Japan, December 2017, 1060–1063.
Just, M. A., and Carpenter, P. A. (1976). Eye Fixations and Cognitive Processes. Cogn. Psychol. 8, 441–480. doi:10.1016/0010-0285(76)90015-3
Kangas, J., Špakov, O., Isokoski, P., Akkil, D., Rantala, J., and Raisamo, R. (2016). “Feedback for Smooth Pursuit Gaze Tracking Based Control,” in Proceedings of the 7th Augmented Human International Conference 2016, Geneva Switzerland, February, 2016, 1–8. doi:10.1145/2875194.2875209
Kim, J., Jeong, Y., Stengel, M., Akşit, K., Albert, R., Boudaoud, B., et al. (2019). Foveated Ar: Dynamically-Foveated Augmented Reality Display. ACM Trans. Graphics (Tog) 38, 1–15. doi:10.1145/3306346.3322987
Koehler, K., Guo, F., Zhang, S., and Eckstein, M. P. (2014). What Do Saliency Models Predict? J. Vis. 14, 14. doi:10.1167/14.3.14
Kollenberg, T., Neumann, A., Schneider, D., Tews, T.-K., Hermann, T., Ritter, H., et al. (2010). “Visual Search in the (Un)real World: How Head-Mounted Displays Affect Eye Movements, Head Movements and Target Detection,” in Proceedings of the 2010 Symposium on Eye-Tracking Research & Applications, Austin Texas, March, 2010 (New York, NY, USA: Association for Computing Machinery), 121–124. ETRA ’10. doi:10.1145/1743666.1743696
Konrad, R., Angelopoulos, A., and Wetzstein, G. (2020). Gaze-contingent Ocular Parallax Rendering for Virtual Reality. ACM Trans. Graphics (Tog) 39, 1–12. doi:10.1145/3361330
Koulieris, G. A., Akşit, K., Stengel, M., Mantiuk, R. K., Mania, K., and Richardt, C. (2019). “Near-eye Display and Tracking Technologies for Virtual and Augmented Reality,” in Computer Graphics Forum. Editors H. Hauser, and P. Alliez (Hoboken, New Jersey, United States: Wiley Online Library), 38, 493–519. doi:10.1111/cgf.13654
Kowler, E., Pizlo, Z., Zhu, G.-L., Erkelens, C. J., Steinman, R. M., and Collewijn, H. (1992). “Coordination of Head and Eyes during the Performance of Natural (And Unnatural) Visual Tasks,” in The Head-Neck Sensory Motor System. Editors A. Berthoz, W. Graf, and P. P. Vidal (Oxford, United Kingdom: Oxford University Press), 419–426. doi:10.1093/acprof:oso/9780195068207.003.0065
Kytö, M., Ens, B., Piumsomboon, T., Lee, G. A., and Billinghurst, M. (2018). “Pinpointing: Precise Head- and Eye-Based Target Selection for Augmented Reality,” in Proceedings of the 2018 CHI Conference on Human Factors in Computing Systems, Montreal, QC, Canada, April, 2018 (New York, NY, USA: Association for Computing Machinery), 1–14. CHI ’18. doi:10.1145/3173574.3173655
Land, M. F. (1992). Predictable Eye-Head Coordination during Driving. Nature 359, 318. doi:10.1038/359318a0
Law, B., Atkins, M. S., Kirkpatrick, A. E., and Lomax, A. J. (2004). “Eye Gaze Patterns Differentiate Novice and Experts in a Virtual Laparoscopic Surgery Training Environment,” in Proceedings of the 2004 Symposium on Eye Tracking Research & Applications, San Antonio Texas, March, 2004 (New York, NY, USA: ACM), 41–48. ETRA ’04. doi:10.1145/968363.968370
Li, R., Whitmire, E., Stengel, M., Boudaoud, B., Kautz, J., Luebke, D., et al. (2020). “Optical Gaze Tracking with Spatially-Sparse Single-Pixel Detectors,” in Proceedings of the 2020 IEEE International Symposium on Mixed and Augmented Reality (ISMAR) (IEEE), Porto de Galinhas, Brazil, November, 2020, 117–126. doi:10.1109/ismar50242.2020.00033
Liversedge, S. P., and Findlay, J. M. (2000). Saccadic Eye Movements and Cognition. Trends Cognitive Sciences 4, 6–14. doi:10.1016/s1364-6613(99)01418-7
Morimoto, C. H., and Mimica, M. R. (2005). Eye Gaze Tracking Techniques for Interactive Applications. Computer Vis. image understanding 98, 4–24. doi:10.1016/j.cviu.2004.07.010
Nichols, T. E., and Holmes, A. P. (2001). Nonparametric Permutation Tests for Functional Neuroimaging: a Primer with Examples. Hum. Brain Mapp. 15, 1–25. doi:10.1002/hbm.1058
Ohn-Bar, E., Martin, S., Tawari, A., and Trivedi, M. M. (2014). “Head, Eye, and Hand Patterns for Driver Activity Recognition,” in Proceedings of the Pattern Recognition (ICPR), 2014 22nd International Conference on (IEEE), Stockholm, Sweden, August 2014, 660–665. doi:10.1109/icpr.2014.124
Oommen, B. S., Smith, R. M., and Stahl, J. S. (2004). The Influence of Future Gaze Orientation upon Eye-Head Coupling during Saccades. Exp. Brain Res. 155, 9–18. doi:10.1007/s00221-003-1694-z
Padmanaban, N., Konrad, R., Stramer, T., Cooper, E. A., and Wetzstein, G. (2017). Optimizing Virtual Reality for All Users through Gaze-Contingent and Adaptive Focus Displays. Proc. Natl. Acad. Sci. 114, 2183–2188. doi:10.1073/pnas.1617251114
Pelz, J. B., and Canosa, R. (2001). Oculomotor Behavior and Perceptual Strategies in Complex Tasks. Vis. Res. 41, 3587–3596. doi:10.1016/S0042-6989(01)00245-0
Pelz, J., Hayhoe, M., and Loeber, R. (2001). The Coordination of Eye, Head, and Hand Movements in a Natural Task. Exp. Brain Res. 139, 266–277. doi:10.1007/s002210100745
Pfeil, K., Taranta, E. M., Kulshreshth, A., Wisniewski, P., and LaViola, J. J. (2018). “A Comparison of Eye-Head Coordination between Virtual and Physical Realities,” in Proceedings of the 15th ACM Symposium on Applied Perception, Vancouver British Columbia Canada, August, 2018 (New York, NY, USA: Association for Computing Machinery). SAP ’18. doi:10.1145/3225153.3225157
Poole, A., and Ball, L. J. (2006). “Eye Tracking in Hci and Usability Research,” in Encyclopedia of Human Computer Interaction. Editor C. Ghaoui (Hershey, Pennsylvania: IGI Global), 211–219. doi:10.4018/978-1-59140-562-7.ch034
Rai, Y., Gutiérrez, J., and Le Callet, P. (2017). “A Dataset of Head and Eye Movements for 360 Degree Images,” in Proceedings of the 8th ACM on Multimedia Systems Conference, Taipei Taiwan, June, 2017 (New York, NY, USA: ACM), 205–210. doi:10.1145/3083187.3083218
Rantala, J., Majaranta, P., Kangas, J., Isokoski, P., Akkil, D., Špakov, O., et al. (2020). Gaze Interaction with Vibrotactile Feedback: Review and Design Guidelines. Human-Computer Interaction 35, 1–39. doi:10.1080/07370024.2017.1306444
Rayner, K. (2009). Eye Movements and Attention in reading, Scene Perception, and Visual Search. Q. J. Exp. Psychol. 62, 1457–1506. doi:10.1080/17470210902816461
Ron, S., and Berthoz, A. (1991). Eye and Head Coupled and Dissociated Movements during Orientation to a Double Step Visual Target Displacement. Exp. Brain Res. 85, 196–207. doi:10.1007/BF00230001
Sidenmark, L., and Gellersen, H. (2019b). “Eye&head: Synergetic Eye and Head Movement for Gaze Pointing and Selection,” in Proceedings of the 32nd Annual ACM Symposium on User Interface Software and Technology, New Orleans LA USA, October, 2019 (New York, NY, USA: Association for Computing Machinery), 1161–1174. UIST ’19. doi:10.1145/3332165.3347921
Sidenmark, L., and Gellersen, H. (2019a). Eye, Head and Torso Coordination during Gaze Shifts in Virtual Reality. ACM Trans. Comput.-Hum. Interact. 27, 1–40. doi:10.1145/3361218
Sitzmann, V., Serrano, A., Pavel, A., Agrawala, M., Gutierrez, D., Masia, B., et al. (2018). Saliency in Vr: How Do People Explore Virtual Environments? IEEE Trans. visualization Comput. graphics 24, 1633–1642. doi:10.1109/tvcg.2018.2793599
Torralba, A., Oliva, A., Castelhano, M. S., and Henderson, J. M. (2006). Contextual Guidance of Eye Movements and Attention in Real-World Scenes: the Role of Global Features in Object Search. Psychol. Rev. 113, 766. doi:10.1037/0033-295x.113.4.766
Wedel, M., and Pieters, R. (2008). “A Review of Eye-Tracking Research in Marketing,” in Review of Marketing Research. Editor N. K. Malhotra (Bingley, United Kingdom: Emerald Group Publishing Limited), 123–147. doi:10.1108/s1548-6435(2008)0000004009
Wilson, M. R., Vine, S. J., Bright, E., Masters, R. S., Defriend, D., and McGrath, J. S. (2011). Gaze Training Enhances Laparoscopic Technical Skill Acquisition and Multi-Tasking Performance: a Randomized, Controlled Study. Surg. Endosc. 25, 3731–3739. doi:10.1007/s00464-011-1802-2
Zangemeister, W. H., and Stark, L. (1982b). Gaze Latency: Variable Interactions of Head and Eye Latency. Exp. Neurol. 75, 389–406. doi:10.1016/0014-4886(82)90169-8
Keywords: virtual reality, head pointing, gaze orientation, head orientation, image viewing in VR
Citation: Kangas J, Špakov O, Raisamo R, Koskinen O, Järvenpää T and Salmimaa M (2022) Head and Gaze Orientation in Hemispheric Image Viewing. Front. Virtual Real. 3:822189. doi: 10.3389/frvir.2022.822189
Received: 25 November 2021; Accepted: 03 February 2022;
Published: 24 February 2022.
Edited by:
Vsevolod Peysakhovich, Institut Supérieur de l’Aéronautique et de l’Espace (ISAE-SUPAERO), FranceReviewed by:
Mark Billinghurst, University of South Australia, AustraliaCopyright © 2022 Kangas, Špakov, Raisamo, Koskinen, Järvenpää and Salmimaa. This is an open-access article distributed under the terms of the Creative Commons Attribution License (CC BY). The use, distribution or reproduction in other forums is permitted, provided the original author(s) and the copyright owner(s) are credited and that the original publication in this journal is cited, in accordance with accepted academic practice. No use, distribution or reproduction is permitted which does not comply with these terms.
*Correspondence: Jari Kangas, amFyaS5hLmthbmdhc0B0dW5pLmZp
Disclaimer: All claims expressed in this article are solely those of the authors and do not necessarily represent those of their affiliated organizations, or those of the publisher, the editors and the reviewers. Any product that may be evaluated in this article or claim that may be made by its manufacturer is not guaranteed or endorsed by the publisher.
Research integrity at Frontiers
Learn more about the work of our research integrity team to safeguard the quality of each article we publish.