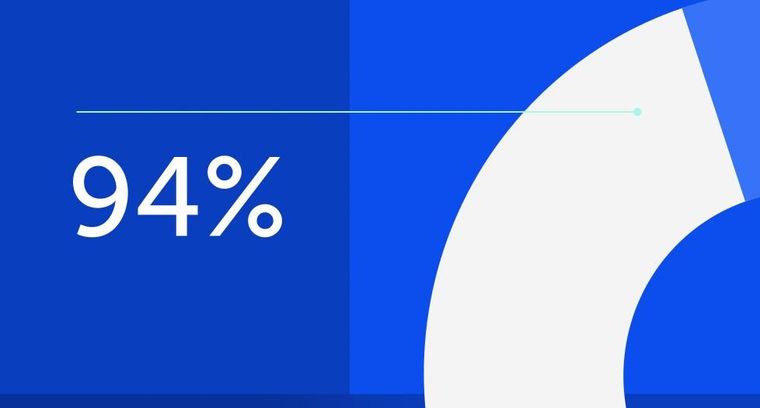
94% of researchers rate our articles as excellent or good
Learn more about the work of our research integrity team to safeguard the quality of each article we publish.
Find out more
ORIGINAL RESEARCH article
Front. Virtual Real., 23 June 2022
Sec. Virtual Reality and Human Behaviour
Volume 3 - 2022 | https://doi.org/10.3389/frvir.2022.773448
This article is part of the Research TopicPresence and Beyond: Evaluating User Experience in AR/MR/VRView all 16 articles
Social presence, the sense of connection with another, is more important than ever as teachers, healthcare providers, and other professionals are using immersive tools to facilitate the social interaction for education, training, therapy and collaboration between geographically distributed humans and surrogates (avatars, agents, or robots). Leading researchers cite the subjective nature of the traditional self-report measures of social presence and the absence of a standardized approach to measuring social presence as a constraint to gaining deeper understanding of user’s experiences of emerging and existing tools. This discourse highlights behavioral indicators of social presence that have been identified over decades across disciplines from psychology, communication, computer science, education, and engineering. The authors explicate the behavioral themes of social presence and describe a classification system grounded in exogenic and endogenic themes of social presence. This article goes on to describe the design of a social presence behavioral coding system (SPBCS) instrument that provides a structure to coding behaviors associated with a users’ experience of social presence. The behavioral coding system described in this paper is the first step in creating a robust standardized approach to quantifying social presence through behavioral, physiological, and subjective indicators that ultimately may replace the current standard subjective approaches to describing the user’s experience in all realities.
The re-emergence of VR as a consumer tool, with the 2013 release of the Oculus Rift, has led to a surge in the discussions about virtual environments, beings, and the tools made available to mediate human communication in virtual spaces (Bailenson et al., 2001, 2005). In addition to the virtual collaboration tools, such as second life, that have been popular for allowing people to communicate with others while representing themselves as avatars on computer screens, new tools, such as Virbela, VRChat, AltSpaceVR, and Rec Room, afford the possibility of communicating with others in a virtual space that may be displayed in an immersive VR headset (Barreda-Ángeles and Hartmann, 2022). While it has not been established which tools are best for different use cases, it is certain that these tools still share the primary goal of allowing people to connect through computers. There are questions about the effectiveness of these computer mediated communication tools and the degree to which any of these tools can provide the experience of the face-to-face social interactions that they are meant to replace (Biocca et al., 2002; Slater, 2004; Hayes, 2015; Oh et al., 2018).
Historically, researchers and practitioners have evaluated social interaction mediated through immersive interfaces by various measures of social presence, or the sense of connection with another (Biocca et al., 2002; Slater, 2004; Hayes, 2015; Oh et al., 2018). Social presence is a sub-construct of presence derived from the constructs: telepresence and co-presence. Initially telepresence expressed the sensation teleoperators had of being at the remote worksite rather than at the operator’s control station (Minsky, 1980). Over time, presence has been simplified to be “the subjective experience of being in one place or environment, even when one is physically situated in another” (Witmer and Singer, 1998, p. 1). Presence is defined as feeling as if one is “there,” while co-presence describes the feeling of “being there with another”. Scientists have used these constructs to evaluate the effectiveness and user experience of teleoperated, remote, and virtual experiences for decades (Chuah et al., 2013; Lu and Fan, 2016). For some experiences, e.g., operating a robot, the sense of presence is central to the effectiveness of an interaction (Lu and Fan, 2016). For other experiences, e.g., teaching a classroom of students, the sense of connection with others is critical. Further, when considering the connection experienced during a computer mediated interaction, the specific notion of presence is not always necessary. One may feel co-present with someone, even without the experience of presence, e.g. talking on the phone with someone; in contrast, one may be quite present in the physical location with another, but still feel socially disengaged, disconnected, or not socially present.
The instruments used to measure these constructs have undergone myriad changes over the years, from design and delivery to evaluation. The majority of these instruments collect subjective self-report from users of the technology. These results are useful, but often lack accuracy and users frequently misrepresent their experiences for various reasons from biases, such as a conformational bias in which the participant tries to please the researcher, to limited self-awareness. Leading researchers have even cited the futility in much of the existing design that focuses this research on subjective measures (Gunawardena and Zittle, 1997; Mennecke et al., 2011; Richardson et al., 2017). However, because of the lack of a simple substitute to the traditional subjective measure, researchers continue to use the tools we have, which are frequently subjective self-report through questionnaires, notwithstanding the acknowledged limitations. The current paper explores the development of a social presence behavioral coding system (SPBCS) meant to provide a simple quantifiable supplement to the way we understand user’s experience of social presence in existing and emerging platforms.
The authors created new technique for measuring social connection experienced with a virtual other, the social presence behavioral coding system (SPBCS) to elucidate and facilitate the process of describing and quantifying the frequency of behaviors that indicate social presence. The design of this social presence behavioral coding system began with a thorough analysis of the literature and approaches to measuring social presence behaviors. The SPBCS provides specific indicators, derived from a literature review of behavioral indicators of social presence. Each such indicator was mapped to the factors of social presence. Qualitative coding is a particularly effective tool for analyzing, summarizing, distilling, and interpreting complex phenomena (Saldana, 2021). Social presence is a complex phenomenon, involving many behavioral indicators, which is why the researchers have chosen this provisional coding approach. The coding system was grounded in the literature about exogenous and endogenous behaviors that identify presence and social presence. The process was refined through the review of unstructured data to identify themes and specific codes for behaviors that indicate social presence.
This coding system is not meant to replace self-report or physiological metrics; instead, this is meant to provide a more robust approach to collecting and representing data about user behavior in regard to social presence. It is the researchers’ intention that an iterative approach to testing this system will deepen and enrich the meaningfulness of the results, while providing a structured process that can be adapted to multiple researchers across contexts and domains.
Similar to physical presence, social presence has been measured through multiple approaches and with varying methodologies including subjective measures, behavioral measures, and physiological measures (Meehan et al., 2002; Biocca et al., 2003; Bailenson et al., 2005; Meehan et al., 2005. In addition to self-report, interviews, and various physiological measures of arousal, researchers have explored using pre-defined behavioral measures that identify a target behavior they measured as a manifestation of a user’s experience of social presence; for instance, verbal and nonverbal self-disclosure has been a metric that researchers assigned as the behavioral metric by which they identified user’s experience of social presence (Bailenson et al., 2005; Moustafa and Steed, 2018)). Further, researchers have explored the relationship between social presence and nonverbal synchrony, presenting the synchrony of turn taking behavior as a potential measure of social presence (Sun et al., 2019).
Throughout the literature, a consensus exists that the drawbacks of each of the approaches may be offset by integration or cross-validation with other approaches. Likewise, while the approach of measuring autonomic responses and collecting data has been effective in gaining insight into the physiological experiences that accompany the senses of presence and social presence (Cui, 2013; Meehan et al., 2002, 2005; Schlögl et al., 2002.), these criteria are hard to directly correlate to social presence as they are also used to indicate other phenomena, such as engagement, attention, and immersion (Slater et al., 2009). The literature has collectively defined several factors as either contributing to, constituting, increasing, detracting, or predicting presence (Gunawardena and Zittle, 1997; Witmer and Singer, 1998; Patel et al., 2008; Thornson et al., 2009; Oh et al., 2018). Table 1 includes those that have been identified in the literature as having a relationship to the experience of social presence. Many studies conducted over the last few decades have identified one or more behavioral indicators of social presence as their operational definition of social presence, depending on the context of the study.
Early researchers outlined two inclusive subdivisions of factors of presence, exogenous or endogenous; exogenous factors are created by the generation of the virtual environment, while endogenous factors are subjective and occur within the user (Slater and Usoh, 1994; Chow, 2012). This distinction preceded a shift in research concerning presence that allowed some research to continue focusing on hardware, fidelity, and display devices, while others began to focus on the analysis of experiences in terms of the affect and cognition of the user (Parsons et al., 2009). Steuer foreshadowed this in the early writing on presence, “In other words, “presence” refers to the natural perception of an environment, and “telepresence” refers to the mediated perception of an environment” (Steuer, 1993, p. 6).
For the study of the specific phenomenon of social presence, the research is informed by the psychological study of attention in which endogenous attention refers to top-down or voluntary attention, whereas exogenous attention is considered bottom-up, or a reorientation of attention to a different stimulus of an attended stimulus (Yantis, 1993; Carretié, 2014). This classification was formerly applied to the construct of presence by early researchers of presence (Slater et al., 1994; Jin and Park, 2009). This distinction between exogenous and endogenous factors facilitates visualization of social presence factors through a structural model in which the elements interact with one another to build a deeper experience of social presence. Likewise, the distinction between exogenous and endogenous factors of social presence serve as a guide for identifying themes, categories, and codes. Table 2 shows these factors of social presence, separated in terms of exogenous or endogenous nature.
Endogenous factors of social presence refer to goal-driven actions directed toward the virtual other in an experience. This presents as the user consciously deciding to engage. Endogenic factors are characterized by conscious and intentional behaviors (Yantis, 1993; Carretié, 2014). Cognitive involvement, flow, self-disclosure, separation anxiety/disorientation, willing suspension of disbelief, and responding to a virtual other as a social actor constitute the endogenous factors of social presence that can be coded by an observer. Flow and novelty can be either top down or bottom up experiences and, as such, are included as both endogenous and exogenous.
Cognitive involvement is said to be an essential component to presence; similarly, it is critical to social presence (Witmer and Singer, 1998; Thornson et al., 2009). “Involvement is a psychological state experienced as a consequence of focusing one’s energy and attention on a coherent set of stimuli or meaningfully related activities and events. Involvement depends on the degree of significance or meaning that the individual attaches to the stimuli, activities, or events” (Witmer and Singer, 1998, p. 227). Cognitive involvement and the state of flow are similar constructs that deal with being absorbed in an experience; and similarly, both can be either active or passive.
Flow is identified as the experience in which an individual experiences the merging of action and awareness, loss of self-consciousness, transformation of time, and enjoyment. (Csikszentmihalyi, 1990). Flow behavior is identified by an individual’s intrinsic motivation to continue, lack of self-consciousness, clarity of goals and feedback, and distorted sense of time (Csikszentmihalyi et al., 2014; Weibel et al., 2008). While flow is characterized by some of the other factors of social presence, the experience of flow can manifest itself in distinct behavioral indicators of social presence. This is related to engagement as it is “characterized by a feeling of energized focus and full involvement in the activity” (Thornson et al., 2009, p. 67). “We define passive cognitive involvement as a cognitive state in which the person is fully engaged in what s/he is doing, characterized by a feeling of energized focus and full involvement in the activity”.
Cognitive involvement and flow often overlap, so, for the behavioral coding, cognitive involvement/flow is operationalized by the lack of attention to surrounding distractions, losing track of time, excitement/joy about the interaction, and expressing a desire to continue the interaction when a user is told “their time is up.” Similarly, this state of cognitive involvement is indicated when a user expresses a desire to maintain the interaction or future interactions with the virtual other.
While self-disclosure has been identified as an indicator of intimacy for decades (Taylor and Binder, 1973; Wheeless and Grotz, 1977), research has also maintained self-disclosure as a measure of the experience of virtual others. While researchers frequently maintain human face to face communication as the “gold standard” of interpersonal communication, some research suggests that hybrid realism possible in virtual representations may maintain high fidelity of interaction without lowering self-disclosure (Bailenson, et al., 2005).
Self-disclosure is operationally defined as an individual speaker sharing information about himself or herself that the audience (students) would not already know (Wood, 2009). Additionally, self-disclosure is a behavior that indicates intimacy and trust (Zimmer et al., 2010; Kang and Gratch, 2014). For the purposes of behavioral coding, this is coded when the participant shares personal information that the virtual other would not otherwise know. Generally, this would be unsolicited self-disclosure, as solicited self-disclosure would be compliance.
The experience of disorientation or anxiety when exiting the virtual environment and returning to the physical world is the indicator of presence Witmer and Singer (1998) called Separation Anxiety/Disorientation. They indicated that the valence of this separation anxiety/disorientation might correlate with the level of presence experienced. This translates to social presence in the endogenous expression of feeling a loss or missing the agents or avatars present in the virtual space after leaving the space.
In terms of behavioral coding, this would be identified in long term interactions or in repeated interactions in which the participant identifies that they “missed” the virtual other or they seek out or request the virtual other or bring up the virtual other’s characteristics as an example.
Understanding the nature of the experiences of individuals with physical presence, co-presence, and social presence is predicated on understanding the intrinsic nature of these phenomena. Suspension of disbelief, immersive tendency, introversion, and empathy are internal experiences that relate to this research into learning within a virtual environment (Thornson et al., 2009). Originating from perceptions around media such as theater, suspension of disbelief is the phenomenon in which a participant in a virtual/synthetic/augmented environment is able to overlook and even forget the fact that the environment is not natural, but constructed and contrived, in order to enhance engagement, presence, and belief of the experience being provided/created (Boellstorff, 2011; Dede, 2009; Jeffries, 2008; Kantor, Waddington, and Osgood, 2000; LeRoy Heinrichs, Youngblood, Harter, and Dev, 2008; Maynes and et al., 1996; Park, Calvert, Brantingham, and Brantingham, 2008; Serby, 2011; Steuer, 1993).
The original concept of suspension of disbelief was actually referred to as willing suspension of disbelief, in which the implication of a conscious action on the part of the participant is central (Steuer, 1993; Serby, 2011). This idea, originating with the poet Samuel Coleridge in the early 1900s, is being challenged by the technology of the day, in which one may willingly suspend disbelief but the technology may also have the power to envelop the user yielding less power than an individual who chooses to pick up a book (Holland, 2008).
Whether it is active or passive suspension of disbelief, the suspension of disbelief is a central element to social presence. There is consistent discussion of suspension of disbelief as a contributing factor or sub-construct that can be used to describe, analyze, and measure presence and social presence (Steuer, 1993; Slater and Wilbur, 1997; Slattery, 2008). These elements of presence have been identified as creating an experience in which the technology mediating the experience “fades” or goes unnoticed or unacknowledged (Mennecke et al., 2011). Suspension of disbelief can be identified by the user ignoring the limits of the technology and behaving in ways that are consistent with believing the experience is real. For instance, if the virtual other is an agent, the user might treat them as if they are real. Likewise, if the interaction is a simulation, the use might engage within the simulation as if the experience were real and meaningful as opposed to laughing and making jokes.
Responding to a virtual agent as if it is a social actor and not just a computer-generated object is considered a demonstration of social presence (Lombard, 2011). Slater et al. (1994) describe the phenomenon of virtual actors responding to subjects as an indicator of presence (Slater et al., 1994). This aligns with observations that participant responses to avatars in the study we present in Section 4 may indicate levels of engagement. Their discussion was driven by Heeter’s (1992) assertion that actors spontaneously reacting to the subject increases presence (Heeter, 1992). Their discussion of subjective factors further supports assumptions that an environment in which one’s own body interacts with a blended physical and virtual environment, yields higher levels of presence than one where the user is being embodied by an avatar.
For behavioral coding, social action and treating the other as a social agent would be identified when participants respond to virtual agents as if they have meaningful experiences and feelings. For example, this can be seen when a user responds with interest or curiosity or even guidance when a virtual character shares a narrative. This can also be seen “when an observer treats a character in a medium as a social actor regardless of whether that actor can respond or is controlled by a human actor (e.g., watching and talking back to a TV anchor)” (Mennecke et al., 2011, p. 414).
Engagement is another widely used and ambiguous term, with many denotative meanings, but the connotations of emotional engagement are essentially consistent. Engagement is generally seen in industry and in psychology as being cognitive, or focused and attentive, emotionally involved, and social, or relating to other people (Cunningham et al., 2006; Skinner et al., 2009). For the purposes of this research, engagement refers to the state of an individual being affectively, behaviorally, and cognitively involved with a virtual other in an experience. This manifests in attention, interest, and motivation to continue.
In terms of behavioral coding, this would be coded when a participant exhibits prolonged gaze/eye contact, body orientation toward the virtual other, smiling, nodding, and minimal encouragement while the virtual other is speaking. Each instance of these behaviors should be coded, for instance if someone if smiling and nodding, both behaviors should be coded.
Exogenous factors of social presence are those generated by the experience in which the user has a “bottom-up” experience due to the interaction with another (Yantis, 1993; Carretié, 2014). Exogenous behaviors are the automatic behaviors that are elicited by the interaction or environment (Slater et al., 1994). The exogenous factors that can be coded through presentation of specific behaviors are social realism, similarity, attributing meaningfulness to an experience, exhibiting similar reactions in face-to-face interactions with another human, intimacy and immediacy as observed by valence of emotions expressed verbally or nonverbally, and physical manipulation.
Scholars note that the Ethopoeia phenomena (Nass and Moon, 2000) reveal that situations or social cues may trigger social action automatically. Similarly, Transformed Social Interaction (TSI) and the Proteus effect are additional theoretical frameworks through which researchers explore the reciprocal relationship between computer-mediated interaction on social behavior (Bailenson et al., 2005; Yee and Bailenson, 2007). Not only do these studies demonstrate the impact of transformed gaze and transformed proximity, they demonstrate automatic reactions, based on random distributions generated by algorithms within systems.
For behavioral coding, social realism is characterized by automatic social responses or automatic feedback responses (e.g., replying to a greeting, turn taking, lack of willingness to walk away from virtual partner when they are talking, or saying goodbye when leaving). Social realism can also be seen in the automatic responses that are characteristic of human interactions, such as turn taking or making eye contact (Ning Shen and Khalifa, 2008).
Similarity, or “homophily” refers to expressing sentiment that the other is similar in terms of attitudes or emotions. Similarity refers to the perception that the other shares attitudes, behaviors, or emotions that create a sense of social attraction.
For behavioral coding, this would be coded when a participant explicitly states their perceived similarity to the other, particularly when used in a positive tone. This may also manifest as the participant suggesting friendship or future interactions with the other. Other behaviors that would constitute similarity could be cross-coded as social realism and active passive social interaction.
Meaningfulness, in relation to virtual objects and environments, refers to realistic perceptual organization (Slattery, 2008). “Meaningfulness pertains to user motivation, task saliency, and previous experience. A more meaningful situation will increase user presence” (Nam and Johnson, 2006, p. 22). While meaningfulness of experience was used in the early discourse about presence, it is appropriate to apply this factor to social presence. In fact, meaningfulness of experience was discussed prior to discussions of “social presence” in virtual experiences. The idea of social presence could easily be substituted for presence in this early analysis, “Presence should increase as the situation presented becomes more meaningful to the person. Meaningfulness is often related to many other factors, such as motivation to learn or perform, task saliency, and previous experience” (Witmer and Singer, 1998, p. 230). This is best illustrated when looking at meaningfulness as immediacy of control, authenticity of the responses and consequences of one’s actions (McGreevy, 1992; Witmer and Singer, 1998).
In terms of behavioral coding, this is identified by self-disclosure of the experience as meaningful, the participant speaking about how the interaction has changed them, or them reflecting on the possibility of future interactions. Meaningfulness of interaction can also be coded when the user’s disclosures demonstrate connection between their lives and the interaction or the virtual other.
Intimacy and immediacy refer to the intensity of emotion expressed (Biocca et al., 2003) and the nonverbal indicators of immediacy, such as proximity, touch, and body orientation (Mehrabian, 1967). Gunawardena (1995) explains that immediacy (and non-immediacy) can be conveyed nonverbally and verbally. Research acknowledges that the intimacy and immediacy are both a cognitive state and a that is typically used to describe behaviors (Biocca et al., 2003), but computer mediated virtual interactions may be measured, controlled or mediated by the design and affordances of interfaces (Palmer 1995; Biocca et al., 2003). Because of this, intimacy and immediacy could be considered both endogenous and exogenous factors, as their manifestations are constrained by the affordances of the environment.
Intimacy and immediacy are operationalized for the behavioral coding system when the user expresses intense emotions by raising his/her voice, crying, laughing, orienting one’s body toward the other, or other body language such as leaning towards the virtual during verbally expressions of intimacy. For example, leaning into the person while engaging in self-disclosure and mirroring of nonverbal behavior indicate rapport (Gratch and Lucas, 2021). Likewise, vocalic indicators, such as voice quality, pitch, rhythm, tempo, resonance, control, and accent, as well as chronemic indicators such as waiting for the other person to speak and talk time can be coded as intimacy/immediacy (Mennecke., et al., 2011). Finally, expressing desire to touch the other (e.g., I want to hug you right now) also indicate intimacy and should be coded as such.
The factor of physical manipulation refers to the effort to physically manipulate objects for another in a virtual space or to ask another to manipulate physical objects, including oneself. Physical touch is associated with social presence and trust in both virtual spaces and the physical world (Chaplain, Phillips, Brown, Clanton, Stein, 2000; Oh et al., 2018).
For behavioral coding, this is operationalized by physically engaging with the virtual other. For example, accepting something that the virtual other hands to the user. Likewise, the use of proximity to engage with the virtual other is a manifestation of physical manipulation. Similarly, changing one’s physical orientation to engage with the virtual other is another approach to physical manipulation, for example, sitting down next to a virtual other or walking with them. Finally, expressing desire to touch the other as a form of manipulating the interaction (e.g., I wish I could walk up behind them).
Another key consideration about social presence throughout the literature is the contrast between active and passive social presence (Lombard et al., 2009). As Slater noted in reference to presence, “it is argued that reality is formed through action, rather than through mental filters” (Slater, 2004). Similarly, researchers distinguish social presence to include verbal or physical action, whereas they refer to perceiving the other as passive social presence (Lombard, 2011). Lombard. (2011) aptly describe active social presence in terms of how often a social actor engaging with an environment makes sounds out-loud, such as laughing or speaking or smiling in response to something that the other social actor does. Inversely, they describe passive social presence as observing the nonverbal behaviors of the other social actor, such as facial expression and tone.
For the social presence behavioral coding system (SPBCS), active social interaction is operationalized as the apparently automatic sounds out-loud, such as laughing or speaking or smiling in response to something that the other social actor does as well as facial expressions and tone not coded elsewhere. Likewise, passive social interaction is operationalized as automatic responses, such as frowning, grimacing, or rolling one’s eyes at the virtual other, not recorded elsewhere. This could also extend to mirroring behaviors in which the participant mirrors the behaviors of the other (Ning Shen and Khalifa, 2008).
Another effective measure of social presence was derived by Schultze in which presence is contrasted with “absence” as an occurrence in which “an individual retreats from the shared world of the here and now into a private, internal and imagined world of the mind” (Schultze and Orlikowski, 2010, p.436). The distinction between presence and absence, drawn by (Waterworth et al., 2010), refers to the attention or inattention to internal or external stimulus. Specifically, they identify absence as “psychological focus on conceptual processing,” whereas, for them, presence is the psychological focus on direct perceptual processing (pg1).
For the purposes of this coding system, absence is indeed the inverse of presence, as when one becomes more present in the virtual world the loss is to presence in the physical world (absence from the physical world), and vice versa. For behavioral coding, absence would be identified by not noticing stimuli in the physical environment, due to engagement with the virtual other. Since the construct of absence is operationally defined as absence from the physical world, the coding is specifically about indicators that the user is absent from the physical world and inversely present in the “other” world. The measure of absence is the exogenic factor that relates to the endogenic factor of flow and is behaviorally indistinguishable from flow.
It is difficult to extricate the impact of the novelty of experiencing virtual environments from the variables of motivation and engagement. This is particularly true of the subjective perspective of users who may experience fun but not be able to identify or pinpoint the stimulus that generates the effect of pleasure (Taylor and Binder, 1973; Slater et al., 2006; Gibson et al., 2012). “Technological novelty is the quality of perceiving digital platforms as unfamiliar, interesting, and unlike those presently used or understood” (Tokunaga, 2013, p. 3). The effect of novelty experienced can be positive or negative on learning, transfer or sense of engagement (Taylor and Binder, 1973; Jacko and Sears, 2008; Tokunaga, 2013). Four dimensions of novelty relevant to virtual interactions are thrill, change from routine, boredom alleviation, and surprise. This must be taken into consideration when evaluating both the user experience and the effectiveness of a virtual environment or experience.
For behavioral coding, novelty is operationalized by the individual explicitly makes statements of awe or wonder during the interaction (e.g. “Wow, this is so cool”). Similarly, expressions of curiosity about the system and surprise about the technology are coded as novelty.
Researchers have asserted the reciprocal nature of the relationship between interactivity and social presence (Gunawardena, 1995; Tu and McIsaac, 2002; Mykota, 2018). Heeter (1992) described interaction in a shared space with shared objects as interactivity. Tu and McIsaac (2002) discuss interactivity in terms of synchronous and asynchronous behavior, but this coding system is focusing on synchronous communication. In some instances, an individual may be eliciting interaction with the virtual other, while in other’s they may be automatically responding to the virtual other. Because of this reciprocal relationship, interactivity can be either endogenous or exogenous. For the social presence behavioral coding system, interactivity refers to interplay between the user and the virtual other.
For the purposes of behavioral coding, interactivity indicators should be coded when the participant responds to the virtual other in a synchronous exchange in a way that is not coded in one of the other constructs. For this reason, this construct of interactivity should be coded to indicate interplay between interactants, such as the number of times the speaker changes (e.g., participant changing from speaker to listener every 15 s or every 3 min) (Dawson and Lignugaris/Kraft, 2017; Hayes, 2015; Li et al., 2021; Miller et al., 2021). Ideally, long term work can be done to accurately capture interplay between conversant that can be represented as a ratio of talk time and the number of times the speaker switched in an interaction.
A new technique for measuring social connection that a person is experiencing with a virtual other, the social presence behavioral coding system (SPBCS) was applied to users of a mixed reality classroom. The social presence factors in the literature was used to collect frequency counts of the behaviors listed that indicate each factor represented. Each of these factors of social presence was from the literature reported in Section 2. The coder was instructed to place a mark in the box for each occurrence of the noted behavior during the time frame. In this case, the time frame was 8 min. There was a box available for notes to be taken for future consideration, including duration and intensity of the behavior.
The participants for this study were 22 active college instructors (10 women and 12 men) of undergraduate and graduate students at a large Southeastern university. Participants elected to complete a professional development activity to improve their teaching. These participants were screened to include only individuals who had not been exposed to the testbed, so as to eliminate familiarity with the technology as a possible confound.
The pilot test for the social presence behavioral coding system (SPBCS) was conducted in the test-bed of TeachLivE, a Mixed Reality classroom simulator in which participants interact with a classroom of five virtual students displayed on a large screen, as shown in Figure 1. The virtual students in the TeachLivE environment are controlled by one human actor in the loop who is trained to simulate student behavior with higher levels of behavioral fidelity than artificial intelligence is currently capable. The user interfaced with the virtual students by delivering their lesson to the virtual kids presented on a large monitor through video conference tool. The interaction was recorded and archived in the after-action review system for review.
FIGURE 1. The teacher practices teaching human in the loop virtual students in the mixed reality classroom simulation training system. Teacher is observed by virtual students through a web camera and voice over internet protocol software.
The social presence instrument included in this research is the Bailenson Social Presence Instrument from a 2006 study on embodied agents designed to measure social presence. The concise nature of the instrument works well to minimize participant fatigue. Also, the items have been used in the past for a similar research approach of comparing the data from more than one method (Bailenson et al., 2005). The questions on this instrument are delivered with a Likert scale (Appendix C).
The social presence instrument included in this research is the Bailenson Social Presence Instrument from a 2006 study on embodied agents designed to measure social presence. The concise nature of the instrument works well to minimize participant fatigue. Also, the items have been used in the past for a similar research approach of comparing the data from more than one method (Bailenson et al., 2004). The questions on this instrument are delivered with a Likert scale (Appendix C).
This tool and training for this tool guided the rating of user behavior by two raters. Coders using the instrument were trained, using the coding sheet and review of videos, to gain a shared understanding of potential manifestations of each of the themes and coded. To save space, the coding system shown in Table 3 has eliminated the columns for tally marks and comments that are on the actual coding system.
Each session was recorded using the After-Action Review (AAR) Coding system that was created to allow users a period of directed reflection that follows the training experience in the simulation. The AAR sessions record the interaction by recording the view of the simulation and the user. While the system is frequently used to reinforce desired behaviors and extinguish undesired behaviors, this pilot did not review the video with the participants. The videos were used for coding behaviors that indicate social presence.
The dependent variables in this study are Presence and Subjective Social Presence and Observed Social Presence. Presence was measured by the modified presence questionnaire; self-reported social presence was measured with the social presence instrument and qualitative responses about the experience. Finally, behavioral social presence was measured by the behavioral social presence score from the social presence behavioral coding system (SPBCS). Consistent with the literature, the objective measure added to the study is physiological data, by way of participant heart rate (Meehan et al., 2002; Meehan et al., 2005).
Participants were asked to engage with the students for 8 minutes, while being observed by the researcher and human in loop controlling the virtual students through the video over internet protocol software. The participants delivered their lessons while interacting with the virtual students on the 72” HD monitor, and while being observed by the researcher and recorded by the After-Action Review coding system. These videos were later reviewed for the researchers to code the participant behaviors. During the interaction, participants (faculty at a southeastern university) were told that they were part of a career day and to talk to the kids briefly about the importance of higher education. They were encouraged to participate in this experience, getting exposure to a virtual classroom and to report their perspectives. Participants were told, “keep in mind that the virtual students are able to see and hear you, but not to physically interact with you, as they are represented by avatars on a large screen but are not physically in the room.” While the virtual students are all controlled by one human in the loop interactor that works to make the students display realistic behaviors of middle school students, the participants were not told if the students were agents or avatars.
The researchers compiled each of the empirical measures for social presence identified in the literature into a coding system for use while observing an interaction. The coding system instructs observers to record the frequency of the targeted behaviors as shown in Table 3: Cognitive Involvement/Flow, Emotional Engagement, (laughing, sweating, raising voice, raising hands), Self-Disclosure, Valence Emotion (intensity) Suspension of Disbelief Social Realism, Social Action (response to agent as if they are a social actor), Physical Manipulation, Similarity, Meaningfulness of Experience/Similarity to Real World (manifested by constructing a narrative for the student and caring about them), and Novelty (expressing amazement at the technology).
In this pilot study, two raters reviewed the video and coded each of the social presence factors exhibited by the participant (the teacher) in the virtual rehearsal. When the raters did not agree, they discussed the discrepancy until they agreed upon an answer.
While there were no disagreements as to whether a behavior constituted a behavioral marker of social presence, there were some discussions as to where to code a behavior. For instance, when a participant enthusiastically told the virtual students about their experience in college while laughing and smiling, one coder considered that suspension of disbelief, social realism, valance, and intimacy; while the other coder only noticed it as disclosure. This happened most frequently when deciding whether to code a behavior in the category of social actor or active social interaction or similarity. The coders had between four to seven discussions on how to code behaviors like these per participant. The approach taken in this study was to code user behaviors in as many social presence behavioral factors as they fit, which did mean that some behaviors were coded in multiple categories. Future iterations of this research will follow a more traditional approach to interrater coding to allow for double blind evaluation of interrater reliability. Likewise, future uses of this coding system will integrate some of the factors.
No new codes or coding categories emerged, instead; the coding process led the researchers to combine some categories of factors into a single category. For the coding process, the construct of emotional engagement needed to be clarified with greater specificity, as there was a great deal of overlap with the rather broad construct with other, more specifically defined constructs (e.g., active social interaction, valance, and intimacy). We decided to code actions that could be either social engagement or more specifically defined constructs as the other construct, due to the very broad nature of social engagement, and only to code social engagement if the behavior did not fit into another, more specific category. Similarly, the construct, suspension of disbelief has a great deal of overlap with social realism and social action/social actor. Because of the overlap, the other variables were collapsed into the broader construct of suspension of disbelief. This also facilitated the coding process.
While it is important to remember the importance of utterances in the coding process, the authors have included some examples of how different physical movements should be coded, according to this first iteration of the SPBCS. Individual utterances may enhance or detract from the detection of social presence behaviors, which is to be explored in greater depth in a training video. Figure 2 demonstrates examples of some of the physical behaviors that align with the coding standards. For Figure 2A., a coder should code based solely on participant utterances, as they are facing away from their conversant(s), therefore the coder can only code what the speaker says and perhaps what they write on the board. For Figure 2B., a coder should code emotional engagement (the teacher is smiling) and immediacy (the gaze toward the conversant), in addition to participant’s utterances. A coder should code Figure 2C with codes of emotional engagement (smiling and hand gestures). For Figure 2D., a coder should code the laughter as emotional engagement and the participant’s action of throwing back her head can be used as an indicator of the intensity/valence of the behavior. In Figure 2E., the participant is pointing to a student to call on them. This would be coded as active social interaction (pointing at the student) and passive social interaction (gaze directed toward student). The coder should code Figure 2F with codes of similarity and physical manipulation, due to the teacher’s use of proximity to the student and approaching the student. Figure 2G should be coded with codes of Suspension of disbelief/Social Realism to reflect the teacher’s waving goodbye to the students.
FIGURE 2. Sample behaviors to guide SPBCS coding include (A) facing away from the interactant, (B) smiling, gaze, (C) gestures, (D) laughing, (E) calling on students, (F) using proximity, (G) and waving hello or goodbye. Coding behaviors and gestures should accompany coding of the participants utterances, as they frequently inform interpretation.
The researchers began with qualitative coding using the SPBCS grounded in the literature and social presence and moved to analysis of unstructured data to identify the themes. During the user study, we collected both qualitative subjective data and quantitative data to test and validate the Behavioral Coding System. The results are presented in the following section. Data for one participant was not included in this analysis, due to corruption of the video capture. Data for another two participants was not included, due to problems with the heart rate tracking.
Each 8-min classroom simulation took approximately 1 hour to review, code, discuss and finalize the analysis. In order to come to agreements about the frequency of behaviors, the coders assigned each construct a ranking of Very High, High, Moderate, Low, or null rather than counting each instance of the behaviors aligned with each factor of social presence. This approach aligned the ordinal data from the Likert scale social presence questionnaire data.
The researchers removed valance and indications of absence or presence as behavioral indicators of social presence for this study, as there was a great deal of disagreement between raters on when to tag a behavior as presence or absence. Specifically, Presence/Absence was removed because the mixed reality nature of the test bed relies on cues in the virtual content that lead individuals to interact with the real and vice versa, so that can lead to an incorrect coding of absence. Finally, the researchers removed th,e ratings of novelty, as novelty of the technology could serve to distract from the user’s actual sense of connection with the virtual others.
The remaining factors included: Cognitive Involvement/Flow, Emotional Engagement, Self-Disclosure, Intimacy/Immediacy, Suspension of Disbelief/Social Realism, Physical Manipulation, Similarity, Meaningfulness of Experience, Interactivity, Active and Passive Social Interaction were all included.
When we classify the comprehensive Behavioral Coding System Frequencies and the Bailenson Social Presence instrument responses as Low, Very Low, Moderate, High, or Very High; all of which was in the 8-min session. Nine of the 19 participants ranked the same in the coding system as they did in the self-report, only one participant showed two levels of difference between the self-report and the Behavioral Coding System, as shown in Figure 4. This participant self-report indicated a moderate level of social presence, while the participant’s behaviors demonstrated high social presence. Upon review of the qualitative interview responses, the participant’s reflection also suggested high levels of social presence, including remembering the names of the virtual students, expressions of amazement with the technology, and the statement, “I didn’t even consider that they are virtual kids (laughs) I play too many video games”. Similarly, when comparing the discrepancies between the Behavioral Coding System and Social presence Questionnaire with the participant’s interview reflections, the behavioral coding system ranking was more closely aligned with the reflections. Likewise, this participant was aware of the human-in the loop design of the system, which may have influenced the responses to the social presence questionnaire.
Initial analysis comparing the Social Presence Scores and the totals of the Social Presence Behavioral Coding System, using an ANOVA test, did not show any relationship between the self-report and our coding system. This led us to rethink our analysis of the data and look at the Behavioral Coding System as Ordinal Data. For this analysis, we included Cognitive Involvement/Flow, Emotional Engagement, Self-Disclosure, Intimacy/Immediacy, Suspension of Disbelief/Social Realism, Physical Manipulation, Similarity, and Meaningfulness of Experience. Interactivity proved to be another problematic construct for the strict coding, so we removed it from our analysis as well.
The scores for the Social Presence Instrument and the Behavioral Coding System rankings were analyzed using Kendall’s Tau (Puka, 2011), which measures the strength of relationships between ordinal variables. Based on the results of the study, those with higher self-report of experiencing social presence were also more likely to demonstrate higher frequencies of behavioral indicators of social presence, the Kendall’s Tau Phi coefficient rt = 0.44, p <0 .05. Similarly, when a multiple regression analysis was used to test if the Behavioral Coding System could be used to predict the subjective report of the presence, the results of the regression indicated that the Behavioral Coding System significantly predicted Social Presence Composite scores (R2 = 0.74, F (1,17) = 47.16, p <0 .01), as shown in Figures 3, 4.
FIGURE 3. Social Presence Coding vs Self-Report Scores demonstrate the trend of social presence coding scores largely correlating with the self report scores of social presence. This ordinal ranking.
The social presence behavioral coding system (SPBCS) described in this article is the first step in refining a deductive approach to analyzing, codifying, summarizing, and interpreting human behaviors that indicate social presence within certain contexts. Qualitative coding systems are, by nature, subject to continuous evolution, as researchers refine their understanding of phenomena and contexts and subjects are also in regular flux (Weston et al., 2001; Saldana, 2021). The initial codes that were established and refined in this study can inform the refinement of endogenic and exogenic indicators of social presence. The most promising outcome of this process has been the refinement of the codes through the collapsing of codes that overlapped. The original coding system, which included 13 codes, was refined to ten codes. This also led to a more specific and granular understanding of each code, that could only be done through trial.
The work to create a social presence behavioral coding system (SPBCS) tested in this pilot validation project provides critical information on how to simplify the behavioral coding system. Utilizing this coding system revealed some redundant variables and others that lacked clarity. Not only does this demonstrate a need to reduce the categories on the coding system, this also demonstrated a need for clear training for the researcher before using the social presence behavioral coding system.
The fact that the initial approach to analysis that included the numeric values of the behaviors that indicate social presence, is rather informative. Looking at these values as ordinal, categorical data aligns best with the approach of classification of behaviors. Rather than looking at a specific number of (e.g. made eye contact four times or seven times) we are looking at ordered categories (e.g. made eye contact rarely or frequently).
We maintained interactivity in the coding system, as it is an important measure of social presence, but did not include it in the data analysis, as this was high for all interactions, by the nature of the simulation. This construct would be measured best as the number of exchanges in the speaker and listener in an interaction or the percentage of time there was interactivity in an interaction. Also, this would include the percentage of time each participant (human, agent, or avatar) speaks.
The fact that the regression and Kendall’s’ Tau demonstrated that the Social Presence Coding system could predict the subjective self-report of social presence also provides support for the application of this tool. However, the tool does provide information that is not available in the self-report. Likewise, when the self-report and the SPBCS don’t align, it may be used as an indicator that more analysis of the interaction is needed.
The Social Presence Behavior Coding System discussed in this article is the first iteration of a system grounded in literature that addresses the need for a more standardized way to objectively measure social presence. The process of testing this system, detailed in this discourse, has led to a more streamlined version of the coding system that collapses some codes found to be redundant in their description of individual human behaviors that indicate social presence (e.g. flow and absence). Likewise, as human behavior changes with context, the coding system can be adapted to accurately predict social presence within specific contexts.
Additional work will be done to continue to refine and validate the SPBCS. This will include a Delphi study plus additional iterations with more in-depth training for coders and measurement of interrater reliability. The current study relied on the agreement of coders at the time of coding, and the lack of a measure of interrater reliability is a significant limitation to the generalizability of the social presence behavioral coding system. This study revealed the importance of a standardized training system to accompany the coding system. This training will be critical and future iterations will test the system with various levels of training among coders to determine the amount of training needed to standardize this approach.
The process of coding of user’s social presence behaviors on the social presence behavioral coding system took more time and analysis than originally expected. While the a priori codes and categories based in the literature were clear, overlap between codes emerged when they were applied to actually coding participant behavior. Changes that were identified during this pilot validation process ranged from oversights, such as duplicates, to classification problems in which an activity classified as more than one construct.
Because the researchers discussed the coding system and came to an agreement before the final coding, there was no opportunity to evaluate interrater reliability. Interrater reliability will be critical to validate this coding system. While that was helpful in this first cycle of coding, testing interrater reliability of the codes is the next step. The raters should have rated separately and interrater reliability should have been calculated. The next iteration of this study will be conducted with this approach.
Future applications of the SPBCS should also be mindful in the inclusion of codes, as they are appropriate to the research context, some of the codes are specific to the context of the specific virtual classroom experience that was used for this research. For instance, Physical Manipulation in this instrument is dependent on the affordances of the virtual experience. TeachLivE afforded movement, so this would be measured more easily than it would be in an environment in which physical manipulation is not an option. This would not occur as frequently in environments that do not afford movement. What this means is that accurate choice of the changes in affordance has the potential to change the score. The researchers would also suggest establishing a baseline of expected or target behaviors for each study or context. For example, the expectations for a peer to peer interaction could be measured by the SPBCS, but the baseline expectation would differ greatly from the baseline of a teacher to classroom interaction.
Future applications of this preliminary SPBCS will implement a training job aid to be reviewed by the research team before using the social presence behavioral coding sheet. This will address some of the ambiguity with the coding system. Future uses will integrate training that includes a plan to avoid classification problems in which an activity classified as more than one construct. Future training should also include the distinction of whether the team intends to code certain events as one or multiple factors. To standardize this training process, the research team will use videos of interactions from multiple contexts and code them as the training set for other researchers who want to use the instrument. All videos will be coded independently by coders from the same cultural background, the partner’s cultural background, and a third, unrelated cultural background.
The next steps in the iteration on the social presence behavioral coding system will be a Delphi Study. After the necessary changes, revealed by this pilot have been addressed, the researchers will retain a panel of experts to dive into the strengths and limitations of the social presence behavioral coding system (SPBCS) to arrive at a consensus over the representation of the factors of social presence on the coding system. The most up to date version of the SPBCS can be seen in Table 4.
Future studies to build and validate the Behavioral Coding System should include an additional physiological metric, in order to add clarity to the ambiguity in the interpretation of heart rate data. Heart rate data, while correlated with experience of presence, can also indicate many other phenomena in the user (e.g., stress to movement). For example, spikes in heart rate when students asked about the participant could indicate that the user experienced social presence or engagement, but it could also reflect stress related to the user’s personality type (introversion vs. extroversion).
Upon completion of the Delphi study and validation study, the next steps for this research will be to automate the capture of behaviors that indicate social presence. Face tracking, body tracking, and natural language processing can be used to automate and refine the process described for the Social Presence Behavioral Coding System.
Social presence is a complex human experience. Not only can this SPBCS contribute to the measurement of the degree of social presence that an individual experiences, it can also provide a deeper description of how an individual is experiencing social presence. While the researchers intend to continue to iterate on the Social Presence Behavioral Coding System, this work is a starting point for this team and other researchers to begin streamlined approaches to objectively measure and describe users’ behaviors that indicate social presence. Not only will this improve the meaningfulness of research outcomes, it can guide future design. This work can also lead to a more unified theory of what to look for when measuring human connection in physical, hybrid, or virtual spaces. The work is also useful as we move to build agents and avatars to address human needs for learning, training, socialization, and interaction. The work that comes from the applications of this social presence behavioral coding system (SPBCS) can inform the development, iteration, and evaluation of agents and systems.
Raw data were generated at University of Central Florida. Derived data supporting the findings of this study are available from the corresponding author ATH on request.
The studies involving human participants were reviewed and approved by University of Central Florida Institutional Review Board. The patients/participants provided their written informed consent to participate in this study.
All authors listed have made a substantial, direct, and intellectual contribution to the work and approved it for publication.
The authors declare that the research was conducted in the absence of any commercial or financial relationships that could be construed as a potential conflict of interest.
All claims expressed in this article are solely those of the authors and do not necessarily represent those of their affiliated organizations, or those of the publisher, the editors and the reviewers. Any product that may be evaluated in this article, or claim that may be made by its manufacturer, is not guaranteed or endorsed by the publisher.
We would like to acknowledge the principal investigators (PIs) of TLE TeachLivE, Lisa Dieker, and Michael Hynes who were not among the authors of this paper. We would like to acknowledge the Bill & Melinda Gates Foundation, whose gift in part supported the TeachLivE team and the execution of this study, and the National Science Foundation (CNS1051067) whose support contributed to the technical infrastructure.
Bailenson, J. N., Beall, A. C., Loomis, J., Blascovich, J., and Turk, M. (2005). Transformed Social Interaction, Augmented Gaze, and Social Influence in Immersive Virtual Environments. Hum. Comm. Res. 31 (4), 511–537. doi:10.1111/j.1468-2958.2005.tb00881.x
Bailenson, J. N., Blascovich, J., Beall, A. C., and Loomis, J. M. (2001). Equilibrium Theory Revisited: Mutual Gaze and Personal Space in Virtual Environments. Presence Teleoperators Virtual Environ. 10 (6), 583–598. doi:10.1162/105474601753272844
Barreda-Ángeles, M., and Hartmann, T. (2022). Psychological Benefits of Using Social Virtual Reality Platforms during the Covid-19 Pandemic: The Role of Social and Spatial Presence. Comput. Hum. Behav. 127, 107047. doi:10.1016/j.chb.2021.107047
Biocca, F., Harms, C., and Burgoon, J. K. (2003). Toward a More Robust Theory and Measure of Social Presence: Review and Suggested Criteria. Presence Teleoperators Virtual Environ. 12 (5), 456–480. doi:10.1162/105474603322761270
Biocca, F., Harms, C., and Burgoon, J. K. (2002). Criteria for a theory and measure of social presence. Paper under review for Presence.
Carretié, L. (2014). Exogenous (Automatic) Attention to Emotional Stimuli: A Review. Cogn. Affect Behav. Neurosci. 14 (4), 1228–1258. doi:10.3758/s13415-014-0270-2
Chow, M. C. M. (2012). “Factors Influencing Presence in Virtual Worlds,” in NI 2012 : 11th International Congress on Nursing Informatics (Montreal, Canada), 083. June 23-27, 2012.
Chuah, J. H., Robb, A., White, C., Wendling, A., Lampotang, S., Kopper, R., et al. (2013). Exploring Agent Physicality and Social Presence for Medical Team Training. Presence Teleoperators Virtual Environ. 22 (2), 141–170. doi:10.1162/PRES_a_00145
Cui, G. (2013). Evaluating Online Social Presence: An Overview of Social Presence Assessment. Jetde 6 (1). doi:10.18785/jetde.0601.02
Cunningham, T., Hall, A. S., and Young, C. (2006). The Advertising Magnifier Effect: An MTV Study. Jar 46 (4), 369–380. doi:10.2501/S0021849906060454
Dawson, M. R., and Lignugaris/Kraft, B. (2017). Meaningful Practice. Teach. Educ. Special Educ. 40 (1), 26–50. doi:10.1177/0888406416664184
Gibson, D., Osterweil, S., Zintgraff, C., Fisser, P., Wood, T., Knezek, G., et al. (2012). “Next Generation Learning Challenges EDUCAUSE WInners,” in Proceedings of SITE 2012—Society for Information Technology and Teacher Education International Conference. Editor P. Resta (Austin, Texas, USA: Association for the Advancement of Computing in Education (AACE)).
Gunawardena, C. N. (1995). Social Presence Theory and Implications for Interaction and Collaborative Learning in Computer Conferences. Int. J. Educ. Telecommun. 1 (2), 147
Gunawardena, C. N., and Zittle, F. J. (1997). Social Presence as a Predictor of Satisfaction within a Computer‐mediated Conferencing Environment. Am. J. Distance Educ. 11 (3), 8–26. doi:10.1080/08923649709526970
Hayes, A. (2015). The Experience of Physical and Social Presence in a Virtual Learning Environment as Impacted by the Affordance of Movement Enabled by Motion Tracking. USA: University of Central Florida. Doctoral dissertation. https://stars.library.ucf.edu/etd/5014.
Heeter, C. (1992). Being There: The Subjective Experience of Presence. Presence Teleoperators Virtual Environ. 1, 262–271. doi:10.1162/pres.1992.1.2.262
Holland, N. N. (2008). Spider-Man? Sure! the Neuroscience of Suspending Disbelief. Interdiscip. Sci. Rev. 33 (4), 312–320. doi:10.1179/174327908X392870
Jacko, J. A., and Sears, A. (2008). The Human-Computer Interaction Handbook: Fundamentals, Evolving Technologies, and Emerging Applications. nlebk: Lawrence Erlbaum Associates. Available at: http://ezproxy.lib.ucf.edu/login?URL=http://search.ebscohost.com/login.aspx?direct=true&db=nlebk&AN=211061&site=ehost-live.
Jin, S.-A. A., and Park, N. (2009). Parasocial Interaction with My Avatar: Effects of Interdependent Self-Construal and the Mediating Role of Self-Presence in an Avatar-Based Console Game, Wii. CyberPsychology Behav. 12 (6), 723–727. doi:10.1089/cpb.2008.0289
Kang, S.-H., and Gratch, J. (2014). Exploring Users' Social Responses to Computer Counseling Interviewers' Behavior. Comput. Hum. Behav. 34, 120–130. doi:10.1016/j.chb.2014.01.006
Li, J. V., Fernandes, S., McVeigh-Schultz, J., and Isbister, K. (2021). “Challenges in Running Social VR Studies A Tale of a Covid Procedural Pivot,” in Conference on Human Factors in Computing Systems At: Yokohama, Japan. (ResearchGate).
Lombard, M., Ditton, T. B., and Weinstein, L. (2009). Measuring Presence: The Temple Presence Inventory.” in Proceedings of the 12th Annual International Workshop on Presence
Lombard, M. (2011). “Why Telepresence Matters: A Personal Answer To the “So what?”,” in Presented at the 2011 Annual Conference of the International Society for Presence Research (ISPR), Edinburgh, Scotland, 2011, October.
Lu, B., Fan, W., and Zhou, M. (2016). Social Presence, Trust, and Social Commerce Purchase Intention: An Empirical Research. Comput. Hum. Behav. 56, 225–237. doi:10.1016/j.chb.2015.11.057
McGreevy, M. W. (1992). The Presence of Field Geologists in Mars-Like Terrain. Presence Teleoperators Virtual Environ. 1 (4), 375–403. doi:10.1162/pres.1992.1.4.375
Meehan, M., Insko, B., Whitton, M., and Brooks, F. P. (2002). Physiological Measures of Presence in Stressful Virtual Environments. ACM Trans. Graph. 21 (3), 645–652. doi:10.1145/566654.566630
Meehan, M., Razzaque, S., Insko, B., Whitton, M., and Brooks, F. P. (2005). Review of Four Studies on the Use of Physiological Reaction as a Measure of Presence in StressfulVirtual Environments. Appl. Psychophysiol. Biofeedback 30 (3), 239–258. doi:10.1007/s10484-005-6381-3
Mehbabian, A. (1967). Orientation Behaviors and Nonverbal Attitude Communication1. J. Commun. 17 (4), 324–332. doi:10.1111/j.1460-2466.1967.tb01190.x
Mennecke, B. E., Triplett, J. L., Hassall, L. M., Conde, Z. J., and Heer, R. (2011). An Examination of a Theory of Embodied Social Presence in Virtual Worlds*. Decis. Sci. 42 (2), 413–450. doi:10.1111/j.1540-5915.2011.00317.x
Miller, M. R., Sonalkar, N., Mabogunje, A., Leifer, L., and Bailenson, J. (2021). Synchrony within Triads Using Virtual Reality. Proc. ACM Hum.-Comput. Interact. 5 (CSCW2), 4001–40027. doi:10.1145/3479544
Moustafa, F., and Steed, A. (2018). A Longitudinal Study of Small Group Interaction in Social Virtual Reality.” in Proceedings of the 24th ACM Symposium on Virtual Reality Softw.are and Technology, 1–10. doi:10.1145/3281505.3281527
Mykota, D. (2018). The Effective Affect: A Scoping Review of Social Presence. Int. J. E-Learning Distance Educ. 33 (2), 1–30.
Nam, C., and Johnson, S. (2006). Presence in Technologically Mediated Environments: A Research Framework.
Nass, C., and Moon, Y. (2000). Machines and Mindlessness: Social Responses to Computers. J. Soc. Isssues 56 (1), 81–103. doi:10.1111/0022-4537.00153
Ning Shen, K., and Khalifa, M. (2008). Exploring Multidimensional Conceptualization of Social Presence in the Context of Online Communities. Int. J. Human-Computer Interact. 24 (7), 722–748. doi:10.1080/10447310802335789
Oh, C. S., Bailenson, J. N., and Welch, G. F. (2018). A Systematic Review of Social Presence: Definition, Antecedents, and Implications. Front. Robot. AI 5, 5. doi:10.3389/frobt.2018.00114
Parsons, T. D., Kenny, P., Cosand, L., Iyer, A., Courtney, C., and Rizzo, A. A. (2009). A Virtual Human Agent for Assessing Bias in Novice Therapists. Stud. health tech. Info. 142, 253–258. doi:10.3233/978-1-58603-964-6-253
Patel, K., Bailenson, J., Hack-Jung, S., Diankov, R., and Bajcsy, R. (2008). R.: The Effects of Fully Immersive Virtual Reality on the Learning of Physical Tasks.
Richardson, J. C., Maeda, Y., Lv, J., and Caskurlu, S. (2017). Social Presence in Relation to Students' Satisfaction and Learning in the Online Environment: A Meta-Analysis. Comput. Hum. Behav. 71, 402–417. doi:10.1016/j.chb.2017.02.001
Saldana, J. (2021). Analyzing and Interpreting Qualitative Research: After the Interview. SAGE Publications.
Schultze, U., and Orlikowski, W. J. (2010). Research Commentary-Virtual Worlds: A Performative Perspective on Globally Distributed, Immersive Work. Inf. Syst. Res. 21 (4), 810–821. doi:10.1287/isre.1100.0321
Serby, T. (2011). Willing Suspension of Disbelief: A Study in Online Learning through Simulation, and its Potential for Deeper Learning in Higher Education. Liverp. Law Rev. 32 (2), 181–195. doi:10.1007/s10991-011-9095-z
Skinner, E. A., Kindermann, T. A., and Furrer, C. J. (2009). A Motivational Perspective on Engagement and Disaffection Conceptualization and Assessment of Children's Behavioral and Emotional Participation in Academic Activities in the Classroom. Educ. Psychol. Meas. 69 (3), 493–525. doi:10.1177/0013164408323233
Slater, M., Guger, C., Edlinger, G., Leeb, R., Pfurtscheller, G., Antley, A., et al. (2006). Analysis of Physiological Responses to a Social Situation in an Immersive Virtual Environment. Presence Teleoperators Virtual Environ. 15 (5), 553–569. doi:10.1162/pres.15.5.553
Slater, M. (2004). How Colorful Was Your Day? Why Questionnaires Cannot Assess Presence in Virtual Environments. Presence Teleoperators Virtual Environ. 13 (4), 484–493. doi:10.1162/1054746041944849
Slater, M., Lotto, B., Arnold, M. M., and Sánchez-Vives, M. V. (2009). How We Experience Immersive Virtual Environments: The Concept of Presence and its Measurement. Available at: http://diposit.ub.edu/dspace/handle/2445/49643.
Slater, M., Usoh, M., and Steed, A. (1994). Depth of Presence in Virtual Environments. Presence Teleoperators Virtual Environ. 3, 130–144. doi:10.1162/pres.1994.3.2.130
Slater, M., and Wilbur, S. (1997). A Framework for Immersive Virtual Environments (FIVE): Speculations on the Role of Presence in Virtual Environments. Presence Teleoperators Virtual Environ. 6, 603–616. doi:10.1162/pres.1997.6.6.603
Slattery, D. R. (2008). VR and Hallucination: A Technoetic Perspective. Technoetic arts 6 (1), 3–18. doi:10.1386/tear.6.1.3_1
Sun, Y., Shaikh, O., and Won, A. S. (2019). Nonverbal Synchrony in Virtual Reality. PLOS ONE 14 (9), e0221803. doi:10.1371/journal.pone.0221803
Taylor, D. A., and Binder, A. (1973). Degree of Training and the Novelty Transfer Effect. Mem. Cognition 1 (1), 61–63. doi:10.3758/BF03198069
Thornson, C. A., Goldiez, B. F., and Le, H. (2009). Predicting Presence: Constructing the Tendency Toward Presence Inventory. Int. J. Human-Computer Stud. 67, 62–78. doi:10.1016/j.ijhcs.2008.08.006
Tokunaga, R. S. (2013). Engagement with Novel Virtual Environments: The Role of Perceived Novelty and Flow in the Development of the Deficient Self-Regulation of Internet Use and Media Habits. Hum. Commun. Res. 39 (3), 365–393. doi:10.1111/hcre.12008
Tu, C.-H., and McIsaac, M. (2002). The Relationship of Social Presence and Interaction in Online Classes. Am. J. Distance Educ. 16 (3), 131–150. doi:10.1207/S15389286AJDE1603_2
Waterworth, J., Waterworth, E., Mantovani, F., and Riva, G. (2010). On Feeling (The) Present:An Evolutionary Account of the Sense of Presence in Physical and Electronically-Mediated Environments. J. Conscious. Stud. 17, 1–19.
Weston, C., Gandell, T., Beauchamp, J., McAlpine, L., Wiseman, C., and Beauchamp, C. (2001). Analyzing Interview Data: The Development and Evolution of a Coding System. Qual. Sociol. 24 (3), 381–400. doi:10.1023/A:1010690908200
Witmer, B. G., and Singer, M. J. (1998). Measuring Presence in Virtual Environments: A Presence Questionnaire. Presence 7 (3), 225–240. doi:10.1162/105474698565686
Yantis, S. (1993). Stimulus-driven Attentional Capture and Attentional Control Settings. J. Exp. Psychol. Hum. Percept. Perform. 19 (3), 676–681. doi:10.1037/0096-1523.19.3.676
Yee, N., and Bailenson, J. (2007). The Proteus Effect: The Effect of Transformed Self-Representation on Behavior. Hum. Comm. Res. 33 (3), 271–290. doi:10.1111/j.1468-2958.2007.00299.x
Keywords: mixed reality, human computer interaction, user experience evaluation, research methodology, social presence
Citation: Hayes AT, Hughes CE and Bailenson J (2022) Identifying and Coding Behavioral Indicators of Social Presence With a Social Presence Behavioral Coding System. Front. Virtual Real. 3:773448. doi: 10.3389/frvir.2022.773448
Received: 09 September 2021; Accepted: 03 May 2022;
Published: 23 June 2022.
Edited by:
Mary C. Whitton, University of North Carolina at Chapel Hill, United StatesReviewed by:
Ali Haskins Lisle, Virginia Tech, United StatesCopyright © 2022 Hayes, Hughes and Bailenson. This is an open-access article distributed under the terms of the Creative Commons Attribution License (CC BY). The use, distribution or reproduction in other forums is permitted, provided the original author(s) and the copyright owner(s) are credited and that the original publication in this journal is cited, in accordance with accepted academic practice. No use, distribution or reproduction is permitted which does not comply with these terms.
*Correspondence: Aleshia Taylor Hayes, YWxlc2hpYS5wcm9mQGdtYWlsLmNvbQ==; Charles E. Hughes, Y2VoQHVjZi5lZHU=
Disclaimer: All claims expressed in this article are solely those of the authors and do not necessarily represent those of their affiliated organizations, or those of the publisher, the editors and the reviewers. Any product that may be evaluated in this article or claim that may be made by its manufacturer is not guaranteed or endorsed by the publisher.
Research integrity at Frontiers
Learn more about the work of our research integrity team to safeguard the quality of each article we publish.