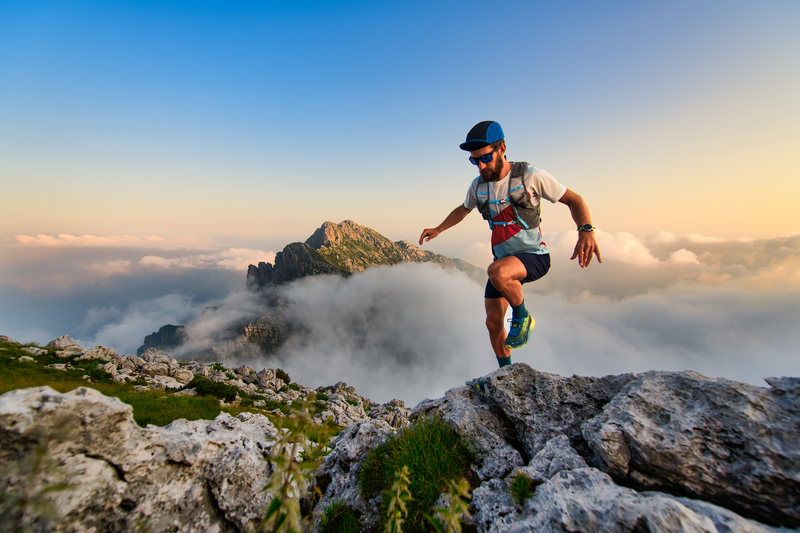
94% of researchers rate our articles as excellent or good
Learn more about the work of our research integrity team to safeguard the quality of each article we publish.
Find out more
METHODS article
Front. Virtual Real. , 10 January 2022
Sec. Virtual Reality in Medicine
Volume 2 - 2021 | https://doi.org/10.3389/frvir.2021.775764
This article is part of the Research Topic Novel Applications of Virtual and Mixed Reality in Pain Research and Treatment View all 6 articles
Chronic Pain (CP) is prevalent in industrialized countries and stands among the top 10 causes of disability. Given the widespread problems of pharmacological treatments such as opioids, a need to find alternative therapeutic approaches has emerged. Virtual Reality (VR) has shown potential as a non-pharmacological alternative for controlling pain over the past 20 years. The effectiveness of VR has been demonstrated in treating CP, and it has been suggested that VR’s analgesic effects may be associated with the Sense of Embodiment (SoE): the sensation of being inside, having and controlling a virtual body in VR. Studies have shown correlations among brain signals, reported pain and a SoE, and correlations have been observed between using an avatar in VR and pain alleviation among CP patients. However, little has been published about the changes in brain physiology associated with having an avatar in VR, and current published studies present methodological issues. Defining a proper methodology to investigate the underlying brain mechanisms of pain, a SoE associated with having an avatar in VR, and its effect on reducing pain in CP patients is key to the emerging field of VR-analgesia. Here, we propose an intervention trial design (test/intervention/test) to evaluate the effects of having a virtual avatar in VR on pain levels and SoE in CP patients using Electroencephalogram (EEG) recordings. Resting-state EEG recordings, perceived pain levels, and SoE scores will be collected before and after the VR intervention. Patients diagnosed with CP will be recruited from local pain clinics and pseudo-randomly assigned to one of two groups—with or without an avatar. Patients will experience a 10-min VR intervention built to treat CP while their EEG signals are recorded. In articulating the study procedure, we propose a framework for future studies that explores the mechanisms of VR-analgesia in patients with chronic pain.
One in five adults in the Western world suffers from pain that persists for more than three months without objective threat to the body, also known as Chronic Pain (CP) (Mills et al., 2019; Scholz et al., 2019). Based on Melzack and Casey’s definition, pain is a subjective experience that emerges from the interaction between sensory, motivational, and cognitive processes (Melzack and Casey, 1968), which is associated with, or resembling that associated with, actual or potential tissue damage (International Association for the Study of Pain, 2021). However, under some circumstances, such as CP, the pain persists regardless of the existence of painful external stimuli, and it can accompany changes in pain perception such as hyperalgesia or allodynia (Scholz et al., 2019). Abnormal neuronal firing in the thalamus and thalamocortical malfunctioning might play a central role in the pathology of CP (Llinás et al., 2005; Ploner et al., 2017) since denervation in this region can cause failure in neurophysiological adjustment to sensory inputs (Ecsy, 2014; Alshelh et al., 2016; Tu et al., 2020). Some studies tracked this thalamocortical dysrhythmia (Llinás et al., 2005) in the changes of the power of brain oscillations in the frequency range of 4–8 Hz (aka. Theta oscillation) over the Prefrontal and Frontal regions of the brain (Pinheiro et al., 2016; Ploner et al., 2017). Additionally, they observed shifts towards lower frequencies in the average power of Peak of Theta-Alpha (4–12 Hz) Frequency (PTAF) in the presence of CP (Boord et al., 2008; Ploner et al., 2017). It is suggested that distorted “body image” might be linked to developing some types of CP conditions, such as in Complex Regional Pain Syndrome (CRPS) (Moseley, 2005; Lotze and Moseley, 2007; Romano and Maravita, 2014; Senkowski and Heinz, 2016).
Body image is a sense and representation (or an image) that one has of their body in terms of its characteristics (Gallagher and Cole, 1995; Longo et al., 2009), giving them a sense of “Embodiment” (Körner et al., 2016). Body image is closely coupled with Body schema: a non-conscious sensorimotor capacity controlling the “performance of the body,” such as updating the position of body parts in space (Gallagher, 2001; Longo et al., 2009; Cuzzolaro, 2018). According to Melzack (Melzack, 1999), this genetically pre-wired body schema is stored in the form of a neural network or matrix in our brain [aka. body matrix (Moseley et al., 2012)]. It is yet unknown how exactly the pain is projected into the body image (Lotze and Moseley, 2007); however, mediums such as Virtual Reality (VR), which can manipulate the body image by providing patients with a virtual body, might be beneficial in understanding this relation.
Immersive Virtual Reality (VR) became available to university researchers in the early 1990s. This novel technology was remarkable in providing users with profoundly new interactions perceptually and sensorially so rich that they reported feeling “immersed” in three-dimensional visual, sonic and haptic computer simulations. One of the first medical applications of VR was using it as a method to control pain. In 2000, Hunter G. Hoffman et al. found that using a game-like virtual environment for severe burn patients reduced their pain levels and the amount of opioids they needed during wound abridgement (Hoffman et al., 2000a; Hoffman et al., 2000b). Since that time, numerous research studies have demonstrated the effectiveness of VR to alleviate or control pain (Li et al., 2011; Gupta et al., 2018), considering it as a viable alternative treatment or a non-pharmacological analgesic (Gupta et al., 2018; Gromala et al., 2011) and a potentially prominent way to avoid using opioids to treat pain (Rosenblum et al., 2008). Nevertheless, few studies have explored the underlying mechanism of VR in pain, especially in CP treatment. Among these few studies, some proposed distraction as the VR mechanism (Hoffman et al., 2006; Gold et al., 2007; Indovina et al., 2018), while others suggested the theory of VR embodiment to explain this phenomenon (Riva et al., 2019; Matamala-Gomez et al., 2019a).
“VR embodiment” can be defined as a new mapping of our body matrix and a virtual avatar when a new body image replaces one’s actual body with a virtual body representation. Some investigations have shown that our body image consists of three subcomponents: Sense of Agency (SoA), Sense of Ownership (SoO) or body image, and Sense of Presence (SoP) or location (David et al., 2008; Braun et al., 2018). It is suggested that in VR, our brain makes a new mental model based on the virtual avatar to gain SoA and SoP and to predict expected inputs from that virtual environment (Riva et al., 2019). Therefore, our body representation changes by adjusting with new sensory signals from the virtual world in VR, changing the distorted body image. As a result, this body matrix modulation affects pain perception, which leads to pain reduction (Matamala-Gomez et al., 2019a). It is believed that the cause of VR embodiment is similar to the Rubber Hand Illusion (RHI), known as Proprioceptive drift (Slater et al., 2009), in which “the perceptual system resolves the sensory conflict between the corresponding visuotactile info and the proprioceptive input” (Matamala-Gomez et al., 2019a). Likewise, in VR, the sensory conflict caused by distorted body image resolves by adjusting to the new virtual body.
Researchers investigated the relationship between VR embodiment, pain alleviation, and Sense of Embodiment by manipulating virtual avatars in VEs. For instance, in some studies, they altered different characteristics of avatars’ virtual hands. As such, they manipulated synchronization (Martini et al., 2014), colour (Slater et al., 2009; Martini, 2016; Matamala-Gomez et al., 2020), size (Romano and Maravita, 2014), relative distance with real hands (Nierula et al., 2017), and transparency (Matamala-Gomez et al., 2019b). For example, a study assessing the RHI in VR by inducing heat pain found significant changes in perceived pain between a synchronous avatar (with higher SoO) and control conditions and non between asynchronous avatar and controls (Nierula et al., 2017). This result could promote the idea that the pain threshold only increases when there is a higher SoO of the avatar; the authors claimed that it is possible to modulate pain perception by increasing the sense of body ownership.
The effect of having an avatar for pain reduction has also been explored using Electroencephalogram (EEG). Another study showed a decrease in EEG signal power over the frequency range of 8–12 Hz (Alpha) among participants during an asynchronous stimulation of a virtual body (visuotactile conflict manipulation) (Lagopoulos et al., 2009). Similarly, Alpha oscillation suppression has also been observed in a study where participants moved in a VR environment using brain imagery. Al-Chalabi and his team found that the subjective feeling of ownership was negatively related to more substantial central, frontal, and centro-parietal alpha oscillation (Alchalabi et al., 2019). However, this study has been conduced on a small sample size group. Therefore, there is a need for a framework exploring study details for larger sample sizes. Similar to the Theta rhythm, it is notable that alpha, which predominantly originates from the occipital lobe during wakeful relaxation with eyes closed (Magosso et al., 2019), can be related to a range of cognitive states. Therefore, tracking EEG oscillations can be additional material to subjective SoE reports exploring how virtual avatars contribute to pain reduction.
Studies investigating the VR-embodiment in pain assessed the effect of virtual body parts modulation on pain and pain threshold. However, these studies have been mostly conducted on healthy subjects and acute (heat/cold-induced) pain, and there are fewer studies on CP patients (Gupta et al., 2018; Matamala-Gomez et al., 2019a). Moreover, given the biopsychosocial nature of pain (Gatchel et al., 2007), questionnaires were the most used assets for evaluating CP and different dimensions of embodiment. However, these methods are subjective and are dependent on a participant’s interpretation of questions, there is a need to combine those methods with neurophysiological measurements (Alchalabi et al., 2019). With its non-invasive nature, high accessibility, high temporal resolution, and low cost, EEG can be considered a good neuroimaging technique to be combined with VR to explore the brain mechanisms among CP patients. However, first, to our knowledge, there is no publication structuring a protocol for conducting VR analgesia studies for CP patients using EEG. Second, given the unique condition of CP patients, such as higher sensitivity to pain and touch, there are special considerations that researchers need to consider designing a VR study for CP patients using EEG.
In this protocol paper, by expounding the details of a study, we will propose a framework for exploring the effect of VR on pain reduction and its neurophysiological correlates. The proposed protocol can be used as a template for future research designs investigating different features of virtual environments (VE) and their role in VR-analgesia by exploring and providing more profound knowledge about neurophysiological changes and the role of an embodied avatar among people with CP condition (Mills et al., 2019).
In the proposed protocol sixty participant (n = 60: control = 30, treatment = 30) with Chronic Pain (CP) condition will be recruited from a pain clinic in Vancouver, BC, Canada. A designed flyer will be distributed among CP patients (diagnosed by their medical doctor specialist based on the International Association for the Study of Pain (IASP) definition of pain) in the clinic. Therefore, patients would voluntarily attend the study and ensure that their attendance would be anonymous to their physicians, and it will not affect their treatment procedure. All participants will be asked to provide their COVID-19 inoculation card before attending the study indicating that they are fully vaccinated.
To mitigate the chance of having unobserved differences between the groups due to confounding variables related to participants’ differences, participants will be assigned to either control or treatment group quasi-randomly (pseudo-randomly) (Moreno et al., 2011). Participants will be assigned to groups by considering their IQ score (using Wechsler Adult Intelligence Scale III (WAIS-III) (Ryan and Lopez, 2001)), age, and sex (Gold and Mahrer, 2018) as possible affecting covariates, and using group matching method (Bang et al., 2020).
Considering the age distribution of patients with CP (Schopflocher et al., 2011), participants older than 18 years old of age, diagnosed with Chronic Pain consistent with IASP pain taxonomy and International Statistical Classification of Diseases and Related Health Problems -11 (ICD-11) (Scholz et al., 2019) criteria will be recruited by medical doctors from aforementioned clinics.
All participants will provide written informed consent before entering the study. They should be able to visit the Pain Studies Lab at SFU. The visit will be limited to the same late morning hours (Bes et al., 2009) to exclude the impact of circadian factors on EEG (Duncan and Northoff, 2013). Participants will be instructed to abstain from caffeinated beverages and food hours before the recording session to avoid the effect and caffeine-induced alpha (Barry et al., 2011; Duncan and Northoff, 2013) and theta reduction in EEG (Dimpfel et al., 1993; Stern et al., 2006). They will also be assessed for caffeine dependence or withdrawal using self-reports. Similarly, they will be asked to abstain from nicotine for 3 h before the study to avoid the effect of nicotine use or withdrawal on EEG signals (Duncan and Northoff, 2013). Participants must be able to read and speak English. They should have a normal or corrected-to-normal eye vision (Tromp et al., 2017) and normal hearing to reach the immersion level and prevent motion sickness using the Head-Mounted Displays (HMDs) in VE. Considering the VE’s characteristics, they should physically be able to sit-stay unmoved for about 20 min and have not been diagnosed with migraines. Finally, all participants should be right-handed due to the difference in neural activity signature and laterality (Cohen et al., 2014). Participants’ handedness will be assessed using the Edinburgh inventory for assessment of handedness before the day of the study (Oldfield, 1971).
We will exclude participants with any history of significant brain injury, medical or surgical illness, neurological disease, drug or alcohol abuse, and any diagnosis of epilepsy or seizure activity (Lagopoulos et al., 2009). Given the target population with CP condition, participants may face severe pain or pain sensitivity if their medication is ceased. Therefore, following previous study protocols on this population (Boord et al., 2008), the use of medications, the usage time and dosage such as opioids that bias trial’s results will be carefully noted initially and be considered as an exclusion criterion if they affect their EEG (Stern et al., 2006). Medication types may include neuroactive medication, benzodiazepines, antiepileptic drugs, tricyclic antidepressants and opioids (Stern et al., 2006). Participants with an IQ score < 80 (using the WAIS-III scale) will be excluded from the study. Finally, possible changes in vigilance will be minuted for the possible slowing of dominant occipital rhythm (Alpha), and EEG files with any sign of drowsiness will be excluded (Stern et al., 2006). Participants will also be asked about their level of tiredness and quality of sleep to have some information about the potential presence of sleep deprivation (Duncan and Northoff, 2013).
The study will occur in a single session. Before the session, participants will be instructed about the use of caffeinated food and beverages, nicotine, and sleep, and checked for their neurological illness history, right-handedness, and cognitive function (ensuring that there will not be any significant group differences in background variables). Figure 1 demonstrates the study procedure. Upon arrival, after a brief introduction to the study, patients will be instructed to read and sign a consent form containing the study process, potential benefits, and participants’ rights. Through a background questionnaire, we will gather participants’ medication (dosage and type) and pain history, to consider them as an exclusion criterion if required.
FIGURE 1. Study Design. This figure shows the process of the study. Patients will be introduced to the study, and their pain will be evaluated. Each patient will be assigned to either the control or treatment group in pseudo-random order. The VR session is divided into four intervals: (a) Pre-Rest—capturing pre-intervention resting-state EEG data with Eyes Closed (EC), (b) VR—Immersion and exploration in VR, (c) EEG-VR—static VR experience (no physical movement) EEG sampling and, (d) Post-Rest—capturing post-intervention resting-state EEG data with Eyes Closed (EC). After the VR session, pain levels and a Sense of Embodiment will be assessed using a Numerical Rating Scale (NRS) and an avatar embodiment questionnaire.
After signing the consent form, all participants will be asked to fill in questionnaires (pre-intervention) for measuring their pain level (Short-Form McGill Pain Questionnaire) (Melzack, 1975). This questionnaire gathers data regarding participant’s general CP level, including a validated Numerical Rating Scale (NRS, 1–10) measuring their pain level right before the intervention. After the pre-intervention, the participants will be comfortably positioned, outfitted with the EEG cap, and get ready to begin the study. Electrodes will then be added to the cap and then patients will be asked to put the HMD on. When the setup completes, patients will experience the VR intervention in a quasi (pseudo)randomized order. It is worth noting that the use of an embodied avatar will not be mentioned in the introductory section to prevent bias amongst participants.
The EEG data sampling and VR intervention will take about 20 min. Participants will be assigned to one of the two conditions: In control conditions, participants experience Virtual Meditative Walk (VMW) without an avatar. While in the treatment condition, they will have a virtual avatar in the VR environment, synchronized with their movement and consistent with their gender, and skin colour (Slater et al., 2009). The procedure will be similar in both conditions: After putting the VR headset on, an eye-closed resting-state EEG sample (Pre-Rest) will be taken for 5 min to provide a baseline before starting the VR intervention. The Pre-Rest EEG will be captured while the participant is wearing the HMD with a black screen. Next, we will initiate the meditative VR application and encourage participants to look around for 1 min to familiarize themselves with the VE (González Franco, 2014). After the VR section, we will ask participants to sit comfortably on the reclining chair providing them with head and neck support, with their head and gaze fixed -still in the VR environment-, to record their EEG signals for the subsequent 9 min (EEG-VR). After recording EEG signals in VR, a second post-test resting state EEG record will be taken for another 5 min (post-Rest) with similar conditions as the Pre-Rest phase. For the post-intervention assessment, we will evaluate participants’ pain levels with a second NRS scale and the Sense of Embodiment (SoE) questionnaires (Gonzalez-Franco and Peck, 2018).
As the primary purpose of this proposed study protocol, we are investigating the effect of adding a virtual avatar on participants’ pain level and sense of embodiment. We are aiming to see if adding a virtual avatar to VMW, as an MBSR-based intervention (See VR), results in pain alleviation and/or increase in SoE amongst CP patients, and if there is any correlation between these two measures. Therefore, the primary evaluation criteria are twofold. The first outcome is Numeric Range Score (NRS) changes for pain five minutes before and after the intervention. Participants’ pain intensity will be evaluated using NRS as a part of the Pre-protocol Questionnaire (Melzack et al., 1987). They will also be asked to specify their pain intensity after the VR session (20 min after the start of the session) on an NRS scale. The difference between the pre-and post- reported NRS values (post-NRS–pre-NRS) would be considered as the Pain Reduction Score (PRS) value. The second outcome will be the Sense of Embodiment (SoE) score, using Avatar Embodiment (AE) questionnaire after taking the VR intervention. This questionnaire will analyze the SoE among participants by emphasizing the key aspects of embodiment: SoO, SoA, and SoP (Gonzalez-Franco and Peck, 2018).
Similarly, we are exploring the effect of the proposed treatment on (pre-Rest—post-Rest) resting-state brain signals and EEG-VR amongst two groups by investigating the effect of adding a virtual avatar on EEG brain oscillations. Based on previous literature, we will study the changes in the power of brain oscillations between two groups. We specifically chose the average of Theta(θ) Power Spectral Density (θ-PSD) which will be recorded at pain-related regions (Boord et al., 2008): frontal, central, and parietal areas, and the average of Alpha (α) Power Spectral Density (α-PSD) recording at the parieto-occipital area, regions in which α oscillation was reported to be negatively related to SoE (Alchalabi et al., 2019). Additionally, the Peak θ-α band Frequency (PTAF), which has been used as an indicator of the presence of CP, will be calculated as the peak power frequency between 4 and 13 Hz, during the 9-min EEG-VR interval over all electrodes (Ploner et al., 2017).
The Virtual Meditative Walk (VMW) (Gromala et al., 2011; Gromala et al., 2015) was developed and customized based on CP patients’ needs in the Pain Studies Lab. This VR system is implemented by the Unity3D game engine (Unity Real-Time Developme Platform, 2020) and runs on Oculus Quest 2 HMD (Oculus Quest: All-in-One VR Headset Oculus, 2020). This lightweight headset does not require a prior room setup and uses an embedded hand tracking system making it suitable for our study purpose. During the intervention, participants will be guided through a mindfulness meditation protocol via a vocal coach as they appear to “walk” or glide forward through a forest. Based on prior studies, the forest was designed to provide a non-disturbing and safe environment (Tong et al., 2015).
Figure 2A depicts a scene of the VMW virtual environment. The forest’s fog density slowly changes based on the patient’s Electrodermal activity (EDA). Although designed to function in a more ambient way than a graph, we nevertheless removed the fog and disabled the EDA capturing feature to eliminate any potential attentional distractors that may violate the validity of the study. The meditation protocol is based on well-studied Mindfulness-Based Stress Reduction (MBSR) techniques (Kabat-Zinn, 2005). MBSR is a scientifically validated form of meditation often used to manage chronic pain (Vehof et al., 2011) because of its long-lasting benefits (Baer, 2003). Considering the hypersensitivities of CP patients, we decided to use the average 10-min duration of previous studies that used VR to reduce or manage chronic and neuropathic pain (Mallari et al., 2019).
FIGURE 2. The Virtual Reality application. (A) Shows Virtual Meditative Walk (VMW) environment: Patients’ will “walk” or float through a forest during their VR intervention. (B) Shows a senior-male model used as an avatar in the VMW. Skin colour will be chosen based on the participant’s skin tone.
During the meditation practice, participants will be asked to lie down on a reclining chair (aka. zero gravity). This sited position will induce a sensation of gliding throughout the forest for the participants. Considering participant’s initial position, for the treatment group, we set the avatar in the same sited position, with a fixed lower body. However, the avatar used in the treatment condition will be synchronized with the participant’s movements using Inverse Kinematic (IK) and animated using Unity’s Animation Rig library (Working with Animation Ri, 2021). Additionally, Oculus Quest 2 provides a built-in hand-tracking feature using inside-out cameras allowing us to get a correct estimation of the participants’ actual hand size and poses (Hand Tracking Privacy Not, 2021). Figure 2B shows one of the models will be used as an avatar in the treatment condition. The age and skin colour of our stylized avatar will be chosen from the closest match to participants’ age and skin colour. For this study, we provided four shades of different skin colours, for male and female avatars and for two levels of age; senior and young adult.
The EEG signals will be captured using an 8-channel Enobio EEG recording system manufactured by Neuroelectrics (Massachusetts, United States) with Geltrode electrodes. Geltrodes are wet EEG electrodes with an Ag/AgCl coated core that require the use of conductive electrode gel; we estimate that this will allow us to set up all electrodes using gel in about 10 min, with a sampling rate of 500 Hz. Therefore, the Enobio system is chosen because of reasons including 1) reasonable signal quality; even using dry electrodes (Collado-Mateo et al., 2015), 2) fast application (Cimtay and Ekmekcioglu, 2020), and 3) previous studies on people with CP (Fibromyalgia) (Villafaina et al., 2019a; Villafaina et al., 2019b), and elderly adults (Abbate et al., 2014). The spatial distribution of the electrodes is based on the standard 10–20 international system configuration with the following electrode distribution: F3, F4, C3, C4, P3, P4, PO3 and PO4, with reference placed on the right earlobe.
Due to CP patients’ critical condition and the vulnerability to the high noise-to-signal ratio of EEG data, the whole study should be conducted on highly controlled experimental conditions. Otherwise, we may induce more pain for patients who are sensitive to pain due to allodynia and/or hyperalgesia (Bennett and Bennett, 2010). This induced pain may add noise to EEG signals and causes the loss of data. We will isolate the patients in a dark room shielded against sound and stray electric fields with controlled temperature to address these problems and increase the signal-to-noise ratio (Duncan and Northoff, 2013). Participants will also be instructed to remain still and relax, avoid eye-blinks and movements [even during the resting-state EEG capturing with Eyes Closed (EC)], and relax jaw muscles during recording. The EEG recording will take place in three sessions for each condition: a 5-min pre-Rest, a 9-min subsequent VR-EEG, and a 5-min post-Rest.
Figure 3 shows the EEG data processing pipeline. Data will be preprocessed using the EEGLAB toolbox (Delorme and Makeig, 2004). The recorded EEG signal will be bandpass filtered between 0.01 and 40 Hz, and a notch filter for the 60 Hz frequency (North American electrical noise). Artifacts will be removed using visual inspection (to remove eye movements and muscle artifacts). Bad channels (sensors with signals that are not usable for the process) will also be marked using the EEGLAB plug-in “clean _rawdata,” which will mark data looking for noisy signals, flat lines, and excessively large amplitudes. Data will be detrended, and finally, the signal will be re-referenced to the earlobe signal.
FIGURE 3. EEG data preprocessing pipeline. 1) EEG signal will be bandpass filtered between 0.01 and 40 Hz. 2) Visual inspection will remove eye movements and muscle artifacts. 3) Bad channels (sensors with unusable signals for the process) will be marked. 4) Data will be detrended. 5) and the signal will be re-referenced to the earlobe reference.
After the preprocessing procedure, to compute an estimate of the Power Spectral Density (PSD), EEG spectral analysis will be performed by averaging consecutive Fast Fourier Transform (FFT) of small windows of signal, with overlapping--Welch’s periodogram method with 10s windows and 50% overlapping. The average PSD across these windows will then be calculated, generating the final PSD estimate for each of the electrodes, for each person. These calculations will be applied to data from all three pre-Rest, post-Rest, and EEG-VR sections. We will calculate PSD over 3- (pre-Rest), 7- (EEG-VR), and 3-min (post-Rest) sessions of the study, starting after the first minute of each section, as the noise will gradually decrease in EEG data during this period. After extracting the PSD estimates for each channel, following the work of (Wang et al., 2015), intercepted EEG will be decomposed into two EEG sub-bands of interest: theta (4–7 Hz) and alpha (8–12 Hz) for each participant using band-passed FIR filter. Relative PSD will also be calculated by dividing the PSD for each frequency band by the total PSD of total frequency band over the aforementioned channels. Moreover, the Peak of θ-α Frequency (PTAF) (Boord et al., 2008) will be identified by the frequency at maximal absolute power from the spectral range of 4–13 Hz.
Descriptive and Inferential statistics will evaluate the hypotheses. Due to the non-normal distribution of data, and lack of prior knowledge about the data population under the null hypothesis, we will use non-parametric statistics to compare data. This analysis will be performed using MATLAB.
The null hypotheses for our primary criteria are as follows:
H0_PRS: The probability distribution of participants’ Pain Reduction Score (PRS) will be similar for two conditions.
H0_SOE: The probability distribution of participants’ Sense of Embodiment (SoE) level will be similar for two conditions.
Given the numeric nature of these two criteria, the effect of the treatment can be found using a t-test if data satisfy the normality test. Therefore, data distribution will initially be tested using Normal Probability Plot. Otherwise, if the data does not satisfy the normality test, a non-parametric permutation test will be employed with 5,000 iterations, and α = 0.05 [see (Maris and Oostenveld, 2007; Waterstone et al., 2020; Fu et al., 2020; Cohen, 2014)]. Moreover, in order to see the correlation between PRS and SOE scores, we will run a Pearson correlation test. The linear relation between the variables will also be checked by making a scatterplot with the tested two variables.
Our secondary criteria will be evaluated under the following null hypotheses:
H0_α-PSD: The probability distribution of average alpha-PSD over (PO3, PO4, P3, P4, C3, C4) will be similar for two conditions.
H0_θ-PSD: The probability distribution of average theta-PSD over (F3, F4, C3, C4, P3, P4) will be similar for two conditions.
H0_PTAF: The probability distribution of average PTAF over (F3, F4, C3, C4, P3, P4) will be similar for two conditions.
Descriptive statistics will also be reported. Data normality will be tested using Shapiro–Wilk normality test and Normal Probability Plot. The following procedure will be applied separately for extracted alpha and theta powers, and the analysis of average PTAF values over two conditions. Although, we will only describe the analysis for PTAF values, the procedure for alpha and theta frequency will be the same for both pre- post-Rest and EEG-VR comparisons.
To compare the effect of treatment over channels, for each hypothesis a non-parametric permutation test will be performed to generate an approximate null probability distribution of the test statistics (Cohen, 2014; Waterstone et al., 2020). Following the work of (Waterstone et al., 2020) we will compare the PSD values for each of the frequency bands among two conditions under hypotheses using t-test as our test statistic. The observed t-values then be evaluated in terms of significance and meaningfulness using our non-permutation analysis. Contrary to constraints forced by parametric statistics, non-parametric statistics let us use a right-tailed t-test as our test statistic, despite the normality violation (Maris and Oostenveld, 2007; Waterstone et al., 2020; Fu et al., 2020).
For each electrode, after calculating the t-value for observed data, we will make a pool of PTAF values and permute them in two conditions (i.e., treatment and control) using Mont Carlo sampling (Maris and Oostenveld, 2007), through 5,000 iterations. In each iteration, we will run the test statistics comparing two conditions. Since the data is randomly shuffled (permuted) and distributed over two groups (i.e., control and treatment), it is expected for each iteration to have a near-zero distribution of t-values over all frequencies (Cohen, 2014). After all iterations, histograms of the null distribution will be generated using t-values derived from permutation. From each null-distributions a right-tail permutation p-value (pc) will then be calculated for each electrode to evaluate the significance of observed t-value for electrode e using below formula:
The formula fractions the number of permuted t-values that are greater than our observed t-value over the total number of iterations., where "NHø" is the number of permuting iterations: 5,000. If the calculated p-value is greater than α = 0.05, tobs rejecting the null-hypothesis for each electrode, can be counted as significant, with 95% confidence (see (Maris and Oostenveld, 2007)). Also, to mitigate the multiple comparisons problem in the permutation testing and minimize the type I error, Bonferroni correction will be applied (see (Cohen, 2014; Wang et al., 2015)).
For the proposed study in this protocol paper, our general hypothesis is that VE with an embodied avatar is more effective in pain alleviation and increases the sense of embodiment among CP patients (Martini et al., 2014; Martini, 2016). Consequently, considering the relation of theta waveform to subjective pain intensity over fontal, prefrontal, and central areas, we expect patients who had an embodied avatar in their VR experience will show a lower average of power of theta oscillation range of EEG signal and higher PTAF value. Similarly, since the subjective feeling of ownership can be related to higher central frontal and central parietal Alpha oscillation, a higher sense of ownership stimulated by a virtual avatar can increase the Alpha oscillation power.
This paper aimed to establish a blueprint for neuroimaging studies in VR for CP patients using EEG by presenting a research protocol exploring the changes in EEG oscillation during an embodied VR. During the past two decades, the use of VR has evolved technologically and practically (Gold and Mahrer, 2018), from research labs to houses. This technology has demonstrated potential in various areas, including acute and chronic pain treatment as a non-pharmaceutical alternative (Gromala et al., 2011). Its effectiveness for pain alleviation has been demonstrated through numerous studies (Li et al., 2011; Gupta et al., 2018), yet little is known about VR-analgesia (Gold et al., 2007; Indovina et al., 2018; Riva et al., 2019; Slater et al., 2009; González Franco, 2014), in particular for CP patients. Therefore, more neuroimaging studies on pain alleviation in VR are expected in the future, especially with the development of new lightweight and more accessible VR-EEG HMDs.
Given the multidisciplinary nature of this growing field of research and criteria needed to be considered for CP patients, there is a need for a general framework indicating the main protocols of EEG-VR study design for CP. In this paper, based on VR-embodiment theory and to explore the brain mechanisms of VR, we proposed a study protocol assessing the effectiveness of using an avatar in VE on patient’s pain, SoE and alpha and theta oscillations. We did so by using questionnaires and EEG as a brain imaging method. The required setup for such studies and following EEG signal and statistical processing was explained and expanded. Contrary to other studies investigating VR-analgesia., we proposed the use of EEG signals in addition to self-reported pain and the sense of embodiment level. This baseline can provide a more profound knowledge for future studies in VR using EEG signals, which can lead to better and more effective VE design for CP patients as an alternative treatment.
Nevertheless, some concerns regarding the proposed study design should be underscored. First, although the proposed sample size is greater than most of the previous studies on acute or chronic pain patients using VR (Mallari et al., 2019), studies with larger sample sizes are suggested to be conducted. A larger sample size not only will increase the power, confidence, and effect size of statistics, but it also will provide the researchers with the freedom to evaluate more measures in their design. Second, with larger sample size, conducting Randomized Controlled Trials (RCTs) will also be possible for future works. We can prevent some of the limitations of the proposed protocol by adding control groups (including healthy controls and outside VR CP controls), running double-blinded experiment sessions, and randomized sampling (using methods such as stratification). These can prevent selection biases amongst participants and provide us with more reliable and generalizable data. Third, given the chronic characteristics of CP, VR evaluation is suggested to happen after a specific dosage of treatment (Gromala et al., 2015; Fu et al., 2020), contrary to the instant effect of treatment on acute pain. Therefore, Longitudinal studies might be preferred to Before-After and Trial studies. This method will also provide the opportunity to evaluate the effect of VR when the potential novelty effect of this medium is decreased. However, it is worth mentioning that concerns regarding the usage setup by participants and dosage in longitudinal studies need to be resolved. Finally, designing studies using different neuroimaging techniques is suggested for future study designs, such as VR HMDs with built-in EEG sensors and MRI-compatible devices.
The original contributions presented in the study are included in the article/Supplementary Material, further inquiries can be directed to the corresponding author.
This study is reviewed and approved by the Harmonized Research Ethics Board of Simon Fraser University Ethics Center and the University of British Columbia (Project ID H2101545). The study will be conducted following the Research Ethics Board REB regulations.
SK, designing the study, drafting and editing the manuscript, and principal investigator in data collecting procedure for the future steps of the project. ZO, revising the study protocol and design, revising and editing the manuscript for content accuracy, and contributing to the data sampling procedure. DG, supervising the project, revising the study protocol and design, revising and editing the manuscript, and providing the project with the VR equipment and system, access to the lab environment for conducting the study, and funding. SM, revising the study protocol and design, revising the manuscript for content accuracy, and supervising the study procedure and data collection in the future. ER, manuscript revision for EEG data processing, and consulting for EEG data acquisition for future steps.
The authors declare that the research was conducted in the absence of any commercial or financial relationships that could be construed as a potential conflict of interest.
All claims expressed in this article are solely those of the authors and do not necessarily represent those of their affiliated organizations, or those of the publisher, the editors, and the reviewers. Any product that may be evaluated in this article, or claim that may be made by its manufacturer, is not guaranteed or endorsed by the publisher.
We would like to thank Pamela Squire and Owen Williamson for offering us access to their clinics and helping us with participant recruitment. We would also like to thank the Computational Health Research Lab at Simon Fraser University for offering us access to their EEG recording systems.
Abbate, S., Avvenuti, M., and Light, J. (2014). Usability Study of a Wireless Monitoring System Among Alzheimer's Disease Elderly Population, [cited 2021 Aug 28]. Int. J. Telemed. Appl., 1–8. doi:10.1155/2014/617495
Alchalabi, B., Faubert, J., and Labbe, D. R. EEG Can Be Used to Measure Embodiment when Controlling a Walking Self-Avatar. In 26th IEEE Conf. Virtual Real 3D User Interfaces, VR 2019 - Proc. 2019;776–783.
Alshelh, Z., di Pietro, F., Youssef, A. M., Reeves, J. M., Macey, P. M., Russell Vickers, E. R., and et al, (2016). Chronic Neuropathic Pain: It’s about the Rhythm, J. Neurosci. 36 (3), [Internet] [cited 2021 Jul 3], 1008–1018. doi:10.1523/jneurosci.2768-15.2016
Baer, R. A. (2003). Mindfulness Training as a Clinical Intervention: A Conceptual and Empirical Review, [Internet] [cited 2021 Jul 14]. Clin. Psychol. Sci. Pract. 10 (2), 125–143. doi:10.1093/clipsy.bpg015
Bang, J. Y., Sharda, M., and Nadig, A. S. (2020). Towards Greater Transparency in Neurodevelopmental Disorders Research: Use of a Proposed Workflow and Propensity Scores to Facilitate Selection of Matched Groups, [Internet]. J. Neurodev. Disord. 12 (1), 1–15. doi:10.1186/s11689-020-09321-6
Bes, F., Jobert, M., and Schulz, H., and (2009). Modeling Napping, post-lunch Dip, and Other Variations in Human Sleep Propensity, [Internet] [cited 2021 Aug 27]. Sleep 32 (3), 392–398. Available from: https://pubmed-ncbi-nlm-nih-gov.proxy.lib.sfu.ca/19294959/.
Boord, P., Siddall, P. J., Tran, Y., Herbert, D., Middleton, J., and Craig, A. (2008). Electroencephalographic Slowing and Reduced Reactivity in Neuropathic Pain Following Spinal Cord Injury, [Internet] [cited 2020 Sep 21]. Spinal Cord 46 (2), 118–123.
Braun, N., Debener, S., Spychala, N., Bongartz, E., Sörös, P., Müller, H. H. O., et al. (2018). The Senses of agency and Ownership: A Review. Front. Psychol. 9, 535. doi:10.3389/fpsyg.2018.00535
Cimtay, Y., and Ekmekcioglu, E. (2020). Investigating the Use of Pretrained Convolutional Neural Network on Cross-Subject and Cross-Dataset EEG Emotion Recognition, [Internet] [cited 2021 Aug 28]. Sensors (Basel) 20 (7). doi:10.3390/s20072034
Cohen, M. X. (2014). Analyzing Neural Time Series Data: Theory and Practice. [Internet]. MIT Press. [cited 2021 Jun 28]. Available from: https://books.google.ca/books?hl=en&lr=&id=rDKkAgAAQBAJ&oi=fnd&pg=PR5&dq=info:T4SzxAVxgeYJ:scholar.google.com&ots=g97iqSX-AV&sig=otWKeHI1zBeGrV_EAwB_atsPeJA&redir_esc=y#v=onepage&q&f=false.
Cohen, O., Koppel, M., Malach, R., and Friedman, D. (2014). Controlling an Avatar by Thought Using Real-Time fMRI, [Internet] [cited 2020 Apr 11]. J. Neural Eng. 11 (3), 35006. Available from: http://medical.nema.org/. doi:10.1088/1741-2560/11/3/035006
Collado-Mateo, D., Adsuar, J. C., Olivares, P. R., Cano-Plasencia, R., and Gusi, N. (2015). Using a Dry Electrode EEG Device during Balance Tasks in Healthy Young-Adult Males: Test-Retest Reliability Analysis, [Internet] [cited 2021 Aug 28]. Somatosensory Mot. Res. 32 (4), 219–226. Available from: http://dx.doi.org.proxy.lib.sfu.ca/103109/0899022020151074566https://www-tandfonline-com.proxy.lib.sfu.ca/doi/abs/10.3109/08990220.2015.1074566. doi:10.3109/08990220.2015.1074566
Cuzzolaro, M. (2018). Body Schema and Body Image: History and Controversies, [Internet] [cited 2021 Jul 15]. Body Image, Eat. Weight, 1–24. Available from: https://link-springer-com.proxy.lib.sfu.ca/chapter/10.1007/978-3-319-90817-5_1.
David, N., Newen, A., and Vogeley, K. (2008). The “Sense of agency” and its Underlying Cognitive and Neural Mechanisms, [Internet]. Conscious. Cogn. 17 (2), 523–534. [cited 2020 Jun 25]; Available from: https://pubmed.ncbi.nlm.nih.gov/18424080/.
Delorme, A., and Makeig, S. (2004). EEGLAB: An Open Source Toolbox for Analysis of Single-Trial EEG Dynamics Including Independent Component Analysis, [Internet][cited 2021 Mar 4]. J. Neurosci. Methods 134 (1), 9–21. doi:10.1016/j.jneumeth.2003.10.009
Dimpfel, W., Schober, F., and Spüler, M. (1993). The Influence of Caffeine on Human EEG under Resting Condition and during Mental Loads, [Internet][cited 2021 Mar 30]. Clin. Investig. 71 (3), 197–207. doi:10.1007/bf00180102
Duncan, N. W., and Northoff, G. (2013). Overview of Potential Procedural and Participant-Related Confounds for Neuroimaging of the Resting State, [Internet] [cited 2021 Aug 25]. J. Psychiatry Neurosci. 38 (2), 84. doi:10.1503/jpn.120059
Ecsy, K. (2014). Analgesic Effects of EEG Alpha-Wave Entrainment on Acute and Chronic Pain. [Internet]. Manchester, England: University of Manchester. [cited 2020 Jun 20]. Available from: https://books.google.ca/books?id=Pl0yvwEACAAJ.
Fu, H., Garrett, B., Tao, G., Cordingley, E., Ofoghi, Z., Taverner, T., et al. (2020). Virtual Reality Guided Meditation for Chronic Pain in Cancer Patients: An Exploratory Analysis of EEG Activity (Preprint). [Internet] [cited 2021 Jun 27]. JMIR Biomed. Eng. 6 (2), e26332. Available from: https://biomedeng.jmir.org/2021/2/e26332.
Gallagher, S., and Cole, J. (1995). Body Image and Body Schema in a Deafferented Subject, [Internet]. J. Mind Behav. 16 (4), 369–389. Available from: http://www.jstor.org/stable/43853796.
Gallagher, S. (2001). Dimensions of Embodiment: Body Image and Body Schema in Medical Contexts. [cited 2020 Dec 18]. Dordrecht: Springer, 147–175. Available from: https://link.springer.com/chapter/10.1007/978-94-010-0536-4_8.
Gatchel, R. J., Peng, Y. B., Peters, M. L., Fuchs, P. N., and Turk, D. C. (2007). The Biopsychosocial Approach to Chronic Pain: Scientific Advances and Future Directions, [Internet] [cited 2020 Sep 29]. Psychol. Bull 133 (4), 581–624. Available from:. doi:10.1037/0033-2909.133.4.581
Gold, J. I., Belmont, K. A., and Thomas, D. A. (2007). The Neurobiology of Virtual Reality Pain Attenuation, [Internet] [cited 2021 Aug 11]. Cyberpsychology Behav. 10 (4). Available from: www.liebertpub.com.
Gold, J. I., and Mahrer, N. E. (2018). Is Virtual Reality Ready for Prime Time in the Medical Space? A Randomized Control Trial of Pediatric Virtual Reality for Acute Procedural Pain Management. J. Pediatr. Psychol. 43 (3), 266–275. doi:10.1093/jpepsy/jsx129
González Franco, M. (2014). Neurophysiological Signatures of the Body Representation in the Brain Using Immersive Virtual Reality, [Internet]. TDX (Tesis Dr en Xarxa), 1–193. Available from: http://www.tdx.cat/handle/10803/359383%5Cnhttp://www.tdx.cat/bitstream/10803/359383/1/MGF_PhD_THESIS.pdf.
Gonzalez-Franco, M., and Peck, T. C. (2018). Avatar Embodiment. Towards a Standardized Questionnaire, [Internet][cited 2020 Jun 24]. Front. Robot AI 5 . Available from: https://www.frontiersin.org/article/10.3389/frobt.2018.00074/full.
Gromala, D., Barnes, S. J., Song, M., Nazemi, M., Yim, J. D., Shaw, C., et al. (2011). A Non-pharmacological Analgesic for Chronic Pain. Conference on Human Factors in Computing Systems - Proceedings. [Internet] [cited 2020 May 28]. New York, New York, USA: ACM Press, 1171–1176. Available from: http://portal.acm.org/citation.cfm?doid=1979742.1979704.
Gromala, D., Tong, X., Choo, A., Karamnejad, M., and Shaw, C. D. (2015). “The Virtual Meditative Walk: Virtual Reality Therapy for Chronic Pain Management,”. [cited 2020 May 28] In Conference on Human Factors in Computing Systems - Proceedings [Internet] (New York, New York, USA: Association for Computing Machinery), 521–524. Available from: http://dl.acm.org/citation.cfm?doid=2702123.2702344.
Gupta, A., Scott, K., and Dukewich, M. (2018). Innovative Technology Using Virtual Reality in the Treatment of Pain: Does it Reduce Pain via Distraction, or Is There More to it, (United States) [Internet] [cited 2020 Apr 13]. Pain Med. 19 (1), 151–159. Available from: http://ovidsp.ovid.com/ovidweb.cgi?T=JS&PAGE=reference&D=emexa&NEWS=N&AN=620509197.
Hand Tracking Privacy Notice 2021, [Internet]. [cited 2021 Nov 16]. Available from: https://support.oculus.com/articles/accounts/privacy-information-and-settings/hand-tracking-privacy-notice.
Hoffman, H. G., Doctor, J. N., Patterson, D. R., Carrougher, G. J., and Furness, T. A. (2000). Virtual Reality as an Adjunctive Pain Control during Burn Wound Care in Adolescent Patients. Pain 85 (1–2), 305–309. doi:10.1016/s0304-3959(99)00275-4
Hoffman, H. G., Patterson, D. R., and Carrougher, G. J. (2000). Use of Virtual Reality for Adjunctive Treatment of Adult Burn Pain during Physical Therapy: A Controlled Study, [Internet] [cited 2020 Jul 5]. Clin. J. Pain 16 (3), 244–250. Available from: https://pubmed.ncbi.nlm.nih.gov/11014398/.
Hoffman, H. G., Richards, T. L., Bills, A. R., Van Oostrom, T., Magula, J., Seibel, E. J. E. J., and et al, (2006). Using fMRI to Study the Neural Correlates of Virtual Reality Analgesia. CNS Spectr. 11 (1), 45–51. doi:10.1017/s1092852900024202
Indovina, P., Barone, D., Gallo, L., Chirico, A., De Pietro, G., and Giordano, A. (2018). Virtual Reality as a Distraction Intervention to Relieve Pain and Distress during Medical Procedures, [Internet] [cited 2020 Apr 11]. Clin. J. Pain 34 (9), 858–877. doi:10.1097/ajp.0000000000000599
Kabat-Zinn, J. 2005, Full Catastrophe Living : Using the Wisdom of Your Body and Mind to Face Stress, Pain, and Illness, 650.
Körner, A., Topolinski, S., and Strack, F. (2016). Routes to Embodiment, [Internet] [cited 2020 Jul 6]. Front. Psychol. 6, 1–10. Available from: www.frontiersin.org.
Lagopoulos, J., Xu, J., Rasmussen, I., Vik, A., Malhi, G. S., Eliassen, C. F. C. F., and et al, (2009). Increased Theta and Alpha EEG Activity during Nondirective Meditation. J. Altern. Complement. Med. 15 (11), 1187–1192. doi:10.1089/acm.2009.0113
Li, A., Montaño, Z., Chen, V. J., and Gold, J. I. (2011). Virtual Reality and Pain Management: Current Trends and Future Directions, [Internet] [cited 2020 Apr 13]. Pain Manag. 1 (2), 147–157. Available from: https://www.futuremedicine.com/doi/abs/10.2217/pmt.10.15.
Llinás, R., Urbano, F. J., Leznik, E., Ramírez, R. R., and van Marle, H. J. F. (2005). Rhythmic and Dysrhythmic Thalamocortical Dynamics: GABA Systems and the Edge Effect, [Internet] [cited 2021 Aug 18]. Trends Neurosci. 28 (6), 325–333. doi:10.1016/j.tins.2005.04.006
Longo, M. R., Schüür, F., Kammers, M. P. M., Tsakiris, M., and Haggard, P. (2009). Self Awareness and the Body Image. Acta Psychol. (Amst) 132 (2), 166–172. doi:10.1016/j.actpsy.2009.02.003
Lotze, M., and Moseley, G. L. (2007). Role of Distorted Body Image in Pain, [Internet] Springer; [cited 2021 Jul 3]. p. Curr. Rheumatol. Rep. 9 (6), 488–496. Available from: https://link.springer.com/article/10.1007/s11926-007-0079-x.
Magosso, E., De Crescenzio, F., Ricci, G., Piastra, S., and Ursino, M. (2019). EEG Alpha Power Is Modulated by Attentional Changes during Cognitive Tasks and Virtual Reality Immersion. Comput. Intell. Neurosci. 2019, 7051079. doi:10.1155/2019/7051079
Mallari, B., Spaeth, E. K., Goh, H., and Boyd, B. S. (2019). Virtual Reality as an Analgesic for Acute and Chronic Pain in Adults: A Systematic Review and Meta-Analysis, [Internet] Dove Medical Press Ltd.; [cited 2020 Jun 4]. J. Pain Res. 12, 2053–2085. Available from: /pmc/articles/PMC6613199/?report=abstract.
Maris, E., and Oostenveld, R. (2007). Nonparametric Statistical Testing of EEG- and MEG-Data. J. Neurosci. Methods 164 (1), 177–190. doi:10.1016/j.jneumeth.2007.03.024
Martini, M., Perez-Marcos, D., and Sanchez-Vives, M. V. V. (2014). Modulation of Pain Threshold by Virtual Body Ownership, [Internet] [cited 2020 Sep 16]. Eur. J. Pain 18 (7), 1040–1048. doi:10.1002/j.1532-2149.2014.00451.x
Martini, M. (2016). Real, Rubber or Virtual: The Vision of “one's Own” Body as a Means for Pain Modulation. A. Narrative Review. Conscious. Cogn. 43, 143–151. [cited 2020 Nov 27]; Available from. doi:10.1016/j.concog.2016.06.005
Matamala-Gomez, M., Gonzalez, A. M. D., Slater, M., and Sanchez-Vives, M. V. (2019). Decreasing Pain Ratings in Chronic Arm Pain through Changing a Virtual Body: Different Strategies for Different Pain Types, [Internet]. J. Pain 20 (6), 685–697. [cited 2021 Jul 15]. Available from: http://www.jpain.org/article/S1526590018309945/fulltext. doi:10.1016/j.jpain.2018.12.001
Matamala-Gomez, M., Nierula, B., Donegan, T., Slater, M., and Sanchez-Vives, M. V. (2020). Manipulating the Perceived Shape and Color of a Virtual Limb Can Modulate Pain Responses, [Internet]. [cited 2021 Feb 8]. J. Clin. Med., 291. Available from: www.mdpi.com/journal/jcm.
Matamala-Gomez, M., Donegan, T., Bottiroli, S., Sandrini, G., Sanchez-Vives, M. V., and Tassorelli, C. (2019). Immersive Virtual Reality and Virtual Embodiment for Pain Relief, Frontiers Media S.A. Front. Hum. Neurosci. Vol 13, 279. doi:10.3389/fnhum.2019.00279
Melzack, R., and Casey, K. L. (1968). Sensory, Motivational, and central Control Determinants of Pain: A New Conceptual Model, 423–443.
Melzack, R. (1999). From the Gate to the Neuromatrix, [Internet] [cited 2020 Jun 25]. Pain 82 (Suppl. 1), S121–S126. Available from: https://pubmed.ncbi.nlm.nih.gov/10491980/.
Melzack, R. (1975). The McGill Pain Questionnaire: Major Properties and Scoring Methods. [Internet] [cited 2020 Jul 6]. Pain 1 (3), 277–299. Available from: https://pubmed.ncbi.nlm.nih.gov/1235985/.
Melzack, R. (1987). The short-form McGill Pain Questionnaire. Pain 30 (2), 191–197. doi:10.1016/0304-3959(87)91074-8
Mills, S. E. E., Nicolson, K. P., and Smith, B. H. (2019). Chronic Pain: a Review of its Epidemiology and Associated Factors in Population-Based Studies, [Internet][cited 2020 Jul 2]. Br. J. Anaesth. 123 (2), e273–83. Available from:/pmc/articles/PMC6676152/?report=abstract. doi:10.1016/j.bja.2019.03.023
Moreno, S., Bialystok, E., Barac, R., Schellenberg, E. G., Cepeda, N. J., and Chau, T. (2011). Short-Term Music Training Enhances Verbal Intelligence and Executive Function, [Internet] [cited 2021 Aug 12]. Psychol. Sci. 22 (11), 1425–1433. Available from: https://doi-org.proxy.lib.sfu.ca/101177/0956797611416999https://journals-sagepub-com.proxy.lib.sfu.ca/doi/full/10.1177/0956797611416999.
Moseley, G. L. (2005). Distorted Body Image in Complex Regional Pain Syndrome, [Internet]. Neurology 65 (5), 773. [cited 2021 Jul 3]. Available from: https://n.neurology.org/content/65/5/773. doi:10.1212/01.wnl.0000174515.07205.11
Moseley, G. L., Gallace, A., and Spence, C. (2012). Bodily Illusions in Health and Disease: Physiological and Clinical Perspectives and the Concept of a Cortical ‘body Matrix’, Pergamon;. Neurosci. Biobehavioral Rev. 36 (1), 34–46. doi:10.1016/j.neubiorev.2011.03.013
Nierula, B., Martini, M., Matamala-Gomez, M., Slater, M., and Sanchez-Vives, M. V. (2017). Seeing an Embodied Virtual Hand Is Analgesic Contingent on Colocation, [Internet] [cited 2021 Jul 15]. J. Pain 18 (6), 645–655. Available from: http://www.jpain.org/article/S1526590017300172/fulltext.
Oculus Quest: All-In-One VR Headset Oculus 2020, [Internet]. [cited 2020 Jul 6]. Available from: https://www.oculus.com/quest/?locale=en_US.
Oldfield, R. C. (1971). The Assessment And Analysis Of Handedness: The Edinburgh Inventory. Neuropsychologia 9, 97–113. doi:10.1016/0028-3932(71)90067-4
Pinheiro, E. S. d. S., De Queirós, F. C., Montoya, P., Santos, C. L., do Nascimento, M. A., Ito, C. H., et al. (2016). Electroencephalographic Patterns in Chronic Pain: A Systematic Review of the Literature, [Internet] [cited 2020 May 20]. PLoS One, 11 (2). Available from: http://www.fapesb.ba.gov.br/.
Ploner, M., Sorg, C., and Gross, J. (2017). Brain Rhythms of Pain [Internet], Elsevier Ltd; [cited 2020 May 25]. Trends Cogn. Sci. 21 (2), 100–110. Available from. doi:10.1016/j.tics.2016.12.001
Riva, G., Wiederhold, B. K., and Mantovani, F. (2019). Neuroscience of Virtual Reality: From Virtual Exposure to Embodied Medicine. Cyberpsychology, Behav. Soc. Networking 22 (1), 82–96. doi:10.1089/cyber.2017.29099.gri
Barry, R. J, Clarke, A. R, and Johnstone, S. J (2011). Caffeine and Opening the Eyes Have Additive Effects on Resting Arousal Measures, [Internet] [cited 2021 Aug 27]. Clin. Neurophysiol. 122 (10), 2010–2015. Available from: https://pubmed-ncbi-nlm-nih-gov.proxy.lib.sfu.ca/21489866/.
Romano, D., and Maravita, A. (2014). The Visual Size of One’s Own Hand Modulates Pain Anticipation and Perception. Neuropsychologia 57 (1), 93–100. Available at: /pmc/articles/PMC2711509/?report=abstract. doi:10.1016/j.neuropsychologia.2014.03.002
Rosenblum, A., Marsch, L. A., Joseph, H., and Portenoy, R. K. (2008). Opioids and the Treatment of Chronic Pain: Controversies, Current Status, and Future Directions, [Internet] [cited 2020 Jul 2]. Exp. Clin. Psychopharmacol. 16 (5), 405–416. Available from:. doi:10.1037/a0013628
Ryan, J. J., and Lopez, S. J. (2001). Wechsler Adult Intelligence Scale-III, [Internet][cited 2021 Aug 26]. Underst Psychol. Assess., 19–42. Available from: https://link-springer-com.proxy.lib.sfu.ca/chapter/10.1007/978-1-4615-1185-4_2.
Scholz, J., Finnerup, N. B., Attal, N., Aziz, Q., Baron, R., Bennett, M. I., et al. (2019). The IASP Classification of Chronic Pain for ICD-11: Chronic Neuropathic Pain, [Internet] Lippincott Williams and Wilkins, [cited 2021 Mar 12]. Pain 160 (1), 53–59. Available from: /pmc/articles/PMC6310153/. doi:10.1097/j.pain.0000000000001365
Schopflocher, D., Taenzer, P., and Jovey, R. (2011). The Prevalence of Chronic Pain in Canada, [Internet] [cited 2020 Aug 11]. Pain Res. Manag. 16 (6), 445–450. Available from: /pmc/articles/PMC3298051/?report=abstract.
Senkowski, D., and Heinz, A. (2016). Chronic Pain and Distorted Body Image: Implications for Multisensory Feedback Interventions, [Internet] [cited 2020 May 1]. Neurosci. Biobehav. Rev. 69, 252–259. Available from. doi:10.1016/j.neubiorev.2016.08.009
Slater, M., Pérez Marcos, D., Ehrsson, H., and Sanchez-Vives, M. V. (2009). Inducing Illusory Ownership of a Virtual Body, [Internet] [cited 2021 Jul 14]. Front. Neurosci. 3 (SEP), 29. Available from: www.frontiersin.org. doi:10.3389/neuro.01.029.2009
Stern, J., Jeanmonod, D., and Sarnthein, J. (2006). Persistent EEG Overactivation in the Cortical Pain Matrix of Neurogenic Pain Patients. [cited 2020 Sep 21]; Available from: http://www.sccn.ucsd.edu/eeglab/.doi:10.1016/j.neuroimage.2005.12.042
International Association for the Study of Pain (2021). Association for the Study of Pain, [Internet]. [cited 2021 Aug 17]. Available from: https://www.iasp-pain.org/resources/terminology/#pain.
Tong, X., Gromala, D., Choo, A., Amin, A., and Shaw, C. (2015). “The Virtual Meditative Walk: An Immersive Virtual Environment for Pain Self-Modulation through Mindfulness-Based Stress Reduction Meditation,” in Lecture Notes in Computer Science (Including Subseries Lecture Notes in Artificial Intelligence and Lecture Notes in Bioinformatics) (Springer-Verlag), 388–397. doi:10.1007/978-3-319-21067-4_40
Tromp, J., Peeters, D., Meyer, A. S., and Hagoort, P. (2017). The Combined Use of Virtual Reality and EEG to Study Language Processing in Naturalistic Environments.
Tu, Y., Fu, Z., Mao, C., Falahpour, M., Gollub, R. L., Park, J., et al. (2020). Distinct Thalamocortical Network Dynamics Are Associated with the Pathophysiology of Chronic Low Back Pain, [Internet] [cited 2021 Jul 3]. Nat. Commun. 11 (1), 1–12. doi:10.1038/s41467-020-17788-z
Unity Real-Time Development Platform, 2020 | 3D, 2D VR & AR Visualizations [Internet]. [cited 2020 Jul 6]. Available from: https://unity.com/.
Veehof, M. M., Oskam, M., Schreurs, M. G. K., and Bohlmeijer, E. T. (2011). Acceptance-based Interventions for the Treatment of Chronic Pain: a Systematic Review and Meta-Analysis. Pain [Internet] [cited 2021 Jul 14]; 152 (3), 533–542. Available from: https://pubmed-ncbi-nlm-nih-gov.proxy.lib.sfu.ca/21251756/.
Villafaina, S., Collado-Mateo, D., Fuentes, J. P., Rohlfs-Domínguez, P., and Gusi, N. (2019). Effects of Exergames on Brain Dynamics in Women with Fibromyalgia: A Randomized Controlled Trial, [Internet]. 2019 Jul 11 [cited 2021 Aug 28]. Jcm 8 (7), 1015. doi:10.3390/jcm8071015
Villafaina, S., Collado-Mateo, D., Fuentes-García, J. P., Domínguez-Muñoz, F. J., and Gusi, N. (2019). Duration of the Symptoms and Brain Aging in Women with Fibromyalgia: A Cross-Sectional Study. Appl. Sci. 9 (10), 2106, [Internet]. 2019 May 23 [cited 2021 Aug 28]. doi:10.3390/app9102106
Wang, R., Wang, J., Yu, H., Wei, X., Yang, C., and Deng, B. (2015). Power Spectral Density and Coherence Analysis of Alzheimer’s EEG, [Internet][cited 2021 Aug 29]. Cogn. Neurodyn. 9 (3), 291, Available from: /pmc/articles/PMC4427585/.
Waterstone, T. S., Niazi, I. K., Navid, M. S., Amjad, I., Shafique, M., Holt, K., et al. (2020). Functional Connectivity Analysis on Resting-State Electroencephalography Signals Following Chiropractic Spinal Manipulation in Stroke Patients, [Internet] [cited 2021 Jun 27]. Brain Sci. 10 (9), 1–18. Available from: www.mdpi.com/journal/brainsci. doi:10.3390/brainsci10090644
Working with Animation Rigging - Unity Learn, 2021, [Internet]. [cited 2021 Nov 16]. Available from: https://learn.unity.com/tutorial/working-with-animation-rigging#6014ac53edbc2a0eea5c1d92.
Keywords: virtual reality, chronic pain, EEG, VR analgesia, embodiment, avatar, alpha wave, theta wave
Citation: Kiaei Ziabari SP, Ofoghi Z, Rodrigues EA, Gromala D and Moreno S (2022) Investigating the Role of Having an Avatar in Virtual Reality on Pain Alleviation and Embodiment in Patients With Pain Using Electroencephalogram: A Neuroimaging Protocol. Front. Virtual Real. 2:775764. doi: 10.3389/frvir.2021.775764
Received: 14 September 2021; Accepted: 03 December 2021;
Published: 10 January 2022.
Edited by:
Daniel Simon Harvie, Griffith University, AustraliaReviewed by:
Tomasz Kupka, Polish Dental Association, PolandCopyright © 2022 Kiaei Ziabari, Ofoghi, Rodrigues, Gromala and Moreno. This is an open-access article distributed under the terms of the Creative Commons Attribution License (CC BY). The use, distribution or reproduction in other forums is permitted, provided the original author(s) and the copyright owner(s) are credited and that the original publication in this journal is cited, in accordance with accepted academic practice. No use, distribution or reproduction is permitted which does not comply with these terms.
*Correspondence: Seyedeh Pegah Kiaei Ziabari, c2thaWVpemlAc2Z1LmNh
Disclaimer: All claims expressed in this article are solely those of the authors and do not necessarily represent those of their affiliated organizations, or those of the publisher, the editors and the reviewers. Any product that may be evaluated in this article or claim that may be made by its manufacturer is not guaranteed or endorsed by the publisher.
Research integrity at Frontiers
Learn more about the work of our research integrity team to safeguard the quality of each article we publish.