- 1Department of Computer Science, University of Central Florida, Orlando, FL, United States
- 2Department of Industrial Engineering and Management Systems, University of Central Florida, Orlando, FL, United States
Artificial intelligence (AI) and extended reality (XR) differ in their origin and primary objectives. However, their combination is emerging as a powerful tool for addressing prominent AI and XR challenges and opportunities for cross-development. To investigate the AI-XR combination, we mapped and analyzed published articles through a multi-stage screening strategy. We identified the main applications of the AI-XR combination, including autonomous cars, robotics, military, medical training, cancer diagnosis, entertainment, and gaming applications, advanced visualization methods, smart homes, affective computing, and driver education and training. In addition, we found that the primary motivation for developing the AI-XR applications include 1) training AI, 2) conferring intelligence on XR, and 3) interpreting XR- generated data. Finally, our results highlight the advancements and future perspectives of the AI-XR combination.
Introduction
Artificial Intelligence (AI) refers to the science and engineering used to produce intelligent machines (Hamet and Tremblay, 2017). AI was developed to automate many human-centric tasks through analyzing a diverse array of data (Wang and Preininger, 2019). AI’s history can be divided into four periods. In the first period (1956–1970), the field of AI began, and terms such as machine learning (ML) and natural language processing (NLP) started to develop (Newell et al., 1958; Samuel, 1959; Warner et al., 1961; Weizenbaum, 1966). In the second period (1970–2012), rule-based approaches received substantial attention, including the development of robust decision rules and the use of expert knowledge (Szolovits, 1988). The third period (2012–2016) began with the advancement of the deep learning (DL) method with the ability to detect cats in pictures (Krizhevsky et al., 2012). The development of DL has since markedly increased (Krizhevsky et al., 2012; Marcus, 2018). Owing to DL advancements, in the fourth period (2016 to the present), the application of AI has achieved notable success as AI has outperformed humans in various tasks (Gulshan et al., 2016; Ehteshami Bejnordi et al., 2017; Esteva et al., 2017; Wang et al., 2017; Yu et al., 2017; Strodthoff and Strodthoff, 2019).
Currently, we are witnessing the rapid growth of virtual reality (VR), augmented reality (AR), and mixed reality (MR) technologies and their applications. VR is a computer-generated simulation of a virtual, interactive, immersive, three-dimensional (3D) environment or image that can be interacted with by using specific equipment (Freina and Ott, 2015). AR is a technology allowing to create a composite view by superimposing digital content (text, images, and sounds) onto a view of the real world (Bower et al., 2014). Mixed reality (MR) is comprising both AR and VR (Kaplan et al., 2020). Extended reality (XR) is an umbrella term including all VR, AR, MR, and virtual interactive environments (Kaplan et al., 2020). In general, XR simulates spatial environments under controlled conditions, thus enabling interaction, modification, and isolation of specific variables, objects, and scenes in a time- and cost-effective manner (Marín-Morales et al., 2018).
XR and AI differ in their focus and applications. XR tries to use computer-generated virtual environments to enhance and extend the human’s capabilities and experiences and enable them to use their capabilities of understanding and discovery in more effective ways. On the other hand, AI attempts to replicate the way humans understand and process information and, combined with the capabilities of a computer, to process vast amounts of data without flaws. Possible applications for both approaches have been found in various application areas, and both are critical and highly active research areas. However, their combination can offer a new range of opportunities. To better understand the potential of the AI-XR combination, we conducted a systematic literature review of published articles by using a multi-stage screening strategy. Our analysis aimed at better understanding the objectives, categorization, applications, limitations, and perspectives of the AI-XR combination.
Methods
We conducted a systematic review of the literature reporting a combination of XR and AI. For this objective, the Preferred Reporting Items for Systematic Reviews and Meta-Analyses (PRISMA) framework was adapted to create a protocol for searching and eligibility (Liberati et al., 2009). The developed protocol was tested and scaled before data gathering. The protocol included two main features of developing research questions on the basis of objectives and determining the search strategy according to research questions.
The following research question was formulated for this review:
RQ. What are the main objectives and applications of the AI-XR combination?
To discover published and gray literature, we used a multi-stage screening strategy including 1) screening scientific and medical publications via bibliographic databases and 2) identifying relevant entities with Google web searches (Jobin et al., 2019). To achieve the best results, we developed multiple sequential search strategies involving an initial keyword-based search of the Google Scholar and ScienceDirect search engines and a subsequent keyword-based search with the Google search engine in private browsing mode outside a personal account. Both steps were performed by using the keywords in Table 1.
In each search step and search keyword, links or articles were followed and screened until the 350th record (Jobin et al., 2019). A total of 321 documents have been identified and included in this step. Total records included 232 articles identified through bibliographic database searching and 89 articles identified through Google searching. The inclusion criteria were articles clearly describing the AI-XR combination and written in the English language. After applying inclusion criteria and removing duplicates, 28 articles were considered from bibliographic database searching and four papers from Google. After combining these two categories and removing duplicates, 28 articles were included in the subsequent analysis. After identification of relevant records, citation-chaining was used to screen and include all relevant references. Seven additional papers were identified and included in this step. To retrieve newly released eligible documents, we continued to search and screen articles until April 3, 2021. One additional article was included on the basis of an extended time frame, as represented in Figure 1. By using three-step searching (web search, search of bibliographic databases of scientific publications, and citation chaining search), we included a total of 36 eligible, non-duplicate articles.
Bias could have occurred in this review through 1) application of the inclusion criteria and 2) extraction of the objectives and applications of the AI-XR combination. To address this bias, two authors separately applied the inclusion criteria and then extracted the objectives and applications of the AI-XR combination. The authors stated the reasons for exclusion, and summarized the main aspects of the AI-XR combination among the included papers. The authors then compared their results with each other, and resolved any disagreements through discussions with the third and fourth authors. In addition, for observational and cross-sectional included articles, we used the National Heart, Lung, and Blood Institute (NHLBI) Quality Assessment Tool for Observational Cohort and Cross-Sectional Studies (National Heart, Lung, and Blood Institute (NHLBI), 2019). We ensured that the quality assessment scores among the included papers were acceptable.
Results
As shown in Table 2, Figures 2, 3, our systematic search identified 36 published records containing AI-XR combinations. A substantial number of developed combinations (n = 13; 36%) have been used for medical and surgical training, as well as autonomous cars and robotics (n = 10; 28%). A review of the affiliations of the authors of the included papers indicated that most AI-XR combination applications were developed in high-ranking research institutes, thus underscoring the importance of the AI-XR research area. The leading high-ranking research institutes cited by the selected articles include Google Brain, Google Health, Intel Labs, different labs in Massachusetts Institute of Technology (MIT), Microsoft Research, Disney Research, Stanford Vision and Learning Laboratory, Ohio Supercomputer Center, Toyota Research Institute, Xerox Research Center, as well as a variety of robotics research laboratories, medical schools, and AI labs.
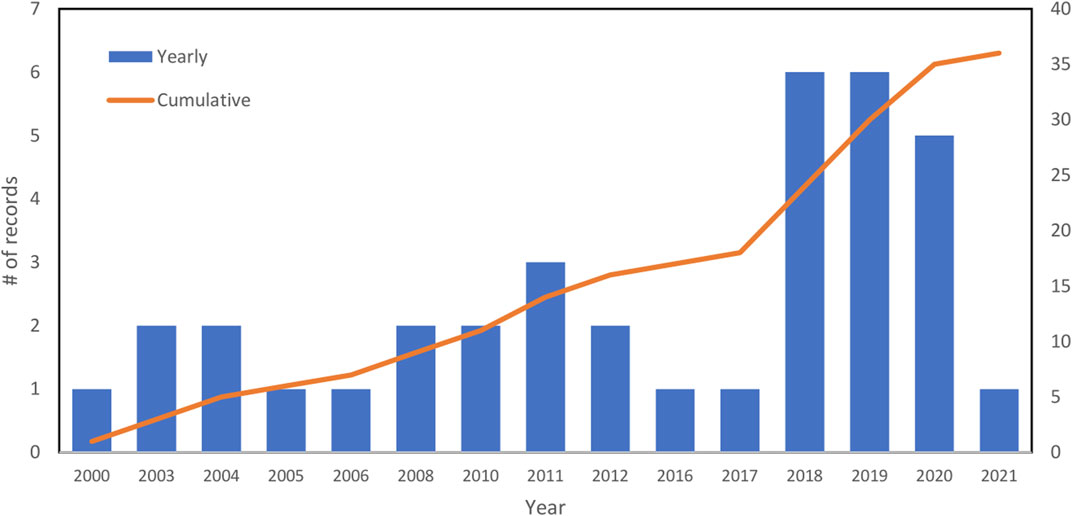
FIGURE 2. Included papers per year (publishing trend). There was a significant increase in the number of included papers published after 2017.
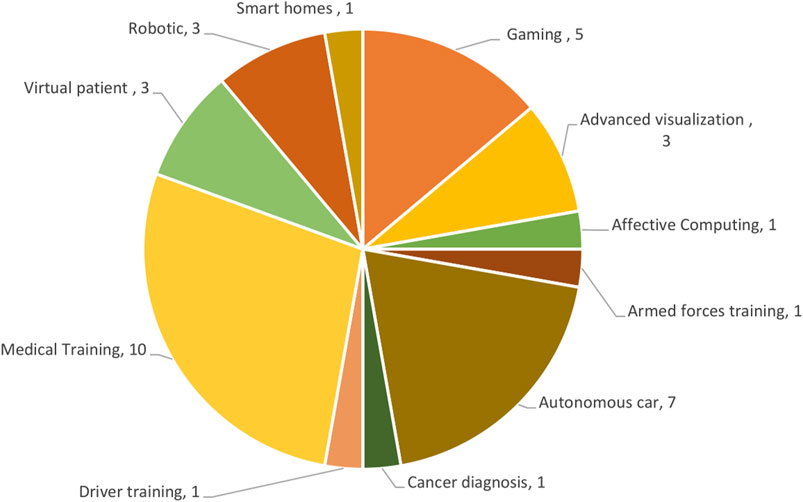
FIGURE 3. Applications of the AI-XR combination among the included records (categorization of included studies). The most popular categories include medical training, autonomous car, and gaming applications.
To explore the included articles, we developed keyword co-occurrence maps of words and terms in the title and abstract. We used VOSviewer software (https://www.vosviewer.com/accessed July 20, 2021) to map the bibliometric data as a network as it is represented in Figure 4. In this figure, nodes are specific terms, their sizes indicate thier frequency, and links represent the co-occurrence of the terms in the title and abstract of the included papers. The most co-occurring terms among selected articles are including virtual reality with artificial intelligence, training, virtual patient, immersive, and visualization.
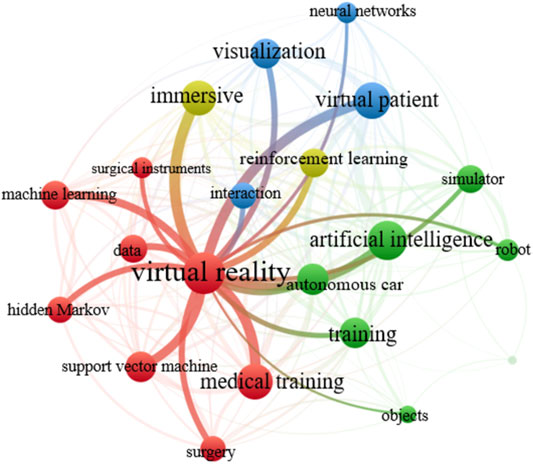
FIGURE 4. The map of the co-occurrence of the words and terms in the title and abstract of included papers.
All included records could be categorized into three groups: 1) interpretation of XR generated data (n = 12; 33%), mainly in medical and surgical training applications, 2) conferring intelligence on XR (n = 10; 28%), mostly in gaming and virtual patient (medical training) applications, and 3) training AI (n = 14; 39%), partly for autonomous cars and robots.
The main applications of the AI-XR combination are medical training, autonomous cars and robotics, gaming, armed forces training, and advanced visualization. Below, we discuss each category.
Medical Training
XR has become one of the most popular technologies in training, and many publications have discussed XR’s advantages in this area (Ershad et al., 2018; Ropelato et al., 2018; Bissonnette et al., 2019). XR provides risk-free, immersive, repeatable environments to improve performance in various tasks, such as surgical and medical tasks. Although XR generates different types of data, interpreting XR generated data and evaluating user skills remain challenging. Combining AI and XR provides an opportunity to better interpret XR dynamics by developing an objective approach for assessing user skill and performance. Through this method, data can be generated from either the XR tool or XR user, after extraction and selection of features from data, and then fed into AI to determine the most relevant skill assessment features. Of 36 included articles, ten (n = 10; 28%) articles focused on medical training. These articles used various AI methods, including Support Vector Machine (two articles), Hidden Markov (two articles), Naive Bayes classifier (two articles), Fuzzy set, and Fuzzy logic (two articles), Neural Networks and Decision trees (two articles). Furthermore, included papers used different XR platforms, including virtual reality surgical (six articles), virtual reality hemilaminectomy (one article), virtual reality laparoscopic (one article), virtual reality mastoidectomy (one article), and EchoComJ (one article). EchoComJ is an AR system that simulates an echocardiographic examination by combining a virtual heart model with real three-dimensional ultrasound data (Weidenbach et al., 2004).
Virtual Patients
In medical training, XR and AI combinations have been used to develop virtual (simulated) patients. In this application, a virtual patient engages with users in a virtual environment. Virtual patients are used for training medical students and trainees to improve specific skills, such as diagnostic interview abilities, and to engage in natural interactions (Gutiérrez-Maldonado et al., 2008). Virtual patients help trainees to easily generalize their knowledge to real-world situations. However, developing appropriate metrics for evaluating user performance during this human-AI interaction in interactive virtual environments remains challenging. Of 36 reviewed papers, three articles focused on the area of virtual patients. Included papers combined NLP methods with virtual environments to develop diagnostic interviews and dialogue skills.
Armed Forces Training
AI can be used as an agent in virtual interactive environments to train armed forces. The main objective of AI is to challenge human participants at a suitable level with respect to their skills. To serve as a credible adversary, the AI agent must determine the human participant’s skill level and then adapt accordingly (Israelsen et al., 2018). For this purpose, the AI agent can be trained in an interactive environment against an intelligent (AI) adversary to learn how to optimize performance. For this AI-AI interaction in an interactive virtual environment, Gaussian process Bayesian optimization techniques have been used to optimize the AI agent’s performance (Israelsen et al., 2018). Only one article focused on armed force training by combining AI decision-maker and Gaussian process Bayesian optimization with virtual environment developed by Orbit Logic simulator.
Gaming Applications
Gaming is another application category of the AI-XR combination. Recently, AI agents have been used to play games such as Starcraft II, Dota 2, the ancient game of Go, and the iconic Atari console games (Kurach et al., 2020). The main objective for developing this application is to provide challenging environments to allow newly developed AI algorithms to be quickly trained and tested. Furthermore, AI agents can be included as non-player characters in video gaming environments to interact with users. However, it is reported that the AI adversary agents used in gaming are highly non-adaptive and scripted (Israelsen et al., 2018). Of 36 included papers, five focused on gaming applications and developed AI-based objects in virtual environments. These articles used various platforms to combine AI-XR such as Reusable Elements for Animation Using Local Integrated Simulation Models (REALISM) software, and Unity.
Robots and Autonomous Cars
Designing a robot with the ability to perform complicated human tasks is very challenging. The main obstacles include the extraction of features from high-dimensional sensor data, modeling the robot’s interaction with the environment, and enabling the robot to adapt to new situations (Hilleli and El-Yaniv, 2018). In reality, resolving these obstacles can be very costly. For autonomous cars, training AI requires capturing vast amounts of data from all possible scenarios, such as near-collision situations, off-orientation positions, and uncommon environmental conditions (Shah et al., 2018). Capturing these data in the real world is not only potentially unsafe or dangerous, but also prohibitively expensive. For both robots and autonomous cars, training and testing AI in virtual environments has emerged as a unique solution. It is reported that both robots and autonomous cars, without any prior knowledge of the task, can be trained entirely in virtual environments and successfully deployed in the real world (Amini et al., 2020). Reinforcement learning (RL) in robots and autonomous cars is commonly trained by using XR. Of 36 papers, ten included articles (28%) focused on robots and autonomous cars. These articles combined DL and RL methods with different virtual environment platforms, including virtual intelligent vehicle urban simulator, CARLA (open source driving simulator with a Python API), AirSim (Microsoft open-source cars and drones simulators built on Unreal Engine), Gazebo (open-source 3D robotics simulator), iGibson (virtual environment providing physics simulation and fast visual rendering based on Bullet simulator), Madras (open-source multi-agent driving simulator), VISTA (a data-driven simulator and training engine), Bullet physics engine simulator, FlightGoggles (photorealistic sensor simulator for perception-driven autonomous cars), and Unity.
Advanced Visualization
XR can create novel displays and improve understanding of complex structures, shapes, and systems. However, automated AI-based imaging algorithms can increase the efficiency of XR by providing automatic visualization of target parts of structures. For example, in patient anatomy applications, the combination of XR and AI can add novelty to the thoracic surgeons’ armamentarium by enabling 3D visualization of the complex anatomy of vascular arborization, pulmonary segmental divisions, and bronchial anatomy (Sadeghi et al., 2021). Finally, XR can be used to visualize the deep learning (DL) structure (Meissler et al., 2019; VanHorn et al., 2019). For example, the XR-based DL development environment can make DL more intuitive and accessible. Three included articles focused on advanced visualization by combining neural networks with different models of XR based on Unity engine, and immersive 3-dimensional-VR platforms.
Discussion
In this section, we describe limitations and perspectives on the AI-XR combination in three categories: interpretation of XR generated data, conferring intelligence on XR, and training AI as it is represented in Figure 5.
Interpretation of XR Generated Data
Recently, much attention has been paid to the use of XR for medical training, particularly in high-risk tasks such as surgery. Extensive data can be collected from users’ technical performance during simulated tasks (Bissonnette et al., 2019). The collected data can be used to extract specific metrics indicating user performance. Because these metrics, in most cases, are incapable of efficiently evaluating users’ level of expertise, they must be extensively validated before implementation and application to real world evaluation (Loukas and Georgiou, 2011). AI, through ML algorithms, can use XR-generated data to validate the metrics of skill evaluation (Ershad et al., 2018).
Data extracted from selected articles are including electroencephalography and electrocardiography results (Marín-Morales et al., 2018); patient eye and pupillary movements (Richstone et al., 2010); volumes of removed tissue, and the position, and angle of the simulated burr and suction instruments (Bissonnette et al., 2019); drilled bones (Kerwin et al., 2012); knot tying and needle driving (Loukas and Georgiou, 2011); and movements of surgical instruments (Megali et al., 2006). Common ML algorithms used to validate skill evaluation metrics include Support Vector Machine (Marín-Morales et al., 2018; Bissonnette et al., 2019), hidden Markov (Megali et al., 2006; Sewell et al., 2008; Loukas and Georgiou, 2011), nonlinear neural networks (Richstone et al., 2010), decision trees (Kerwin et al., 2012), multivariate autoregressive (Loukas and Georgiou, 2011), and Naive Bayes classifier (Sewell et al., 2008).
Conferring Intelligence on XR
Although the advancement of computer graphic techniques has substantially improved XR tools, including intelligence by adding AI can revolutionize XR. Conferring intelligence on XR is about adding AI to a specific part of XR to improve the XR experience. In this combination, AI can help XR to effectively communicate and interact with users. Conferring intelligence on XR can be useful for different applications, such as cancer detection (Chen et al., 2019), gaming (Turan and Çetin, 2019), advanced visualization (Sadeghi et al., 2021), driver training (Ropelato et al., 2018), and virtual patient and medical training (Gutiérrez-Maldonado et al., 2008). For example, in virtual patient applications, AI has been used to create Artificial Intelligence Dialogue Systems (Talbot et al., 2012). Consequently, virtual patients can engage in natural dialogue with users. In virtual patient, intelligent agents can be visualized in human size with the ability of facial expressions, gazing, and gesturing, and can engage in cooperative tasks and synthetic speech (Kopp et al., 2003). In more advanced virtual patients, intelligent agents can understand human emotional states (Gutiérrez-Maldonado et al., 2008).
The main ML algorithms for this type of combination include neural networks (NNs) and fuzzy logic (FL) algorithms. A combination of NNs and XR is commonly used for developing virtual patients and detecting cancer (Chen et al., 2019). For example, one study has developed an augmented reality microscope, which overlays convolutional neural network-based information onto the real-time view of a sample, and has been used to identify prostate cancer and detect metastatic breast cancer (Chen et al., 2019). NNs have been efficiently applied to XR because of its tolerance of noisy inputs, simplicity in encoding complex learning, and robustness in the presence of missing or damaged inputs (Turan and Çetin, 2019). The FL algorithm can handle imprecise and vague problems. This algorithm has been applied to interactive virtual environments and games to allow for more human-like decisive behavior. Because this algorithm needs only the basics of Boolean logic as prerequisites, it can be added to any XR with little effort (Turan and Çetin, 2019).
Training AI
Training AI by using real-world data can be very difficult. For example, developing urban self-driving cars in the physical world requires considerable infrastructure costs, funds and manpower, and overcoming a variety of logistical difficulties (Dosovitskiy et al., 2017). In this situation, XR, serving as a learning environment for AI, can be used as a substitute for training with experimental data. This technique has received attention because of its many advantages such as safety, cost efficiency, and repeatability of training (Shah et al., 2018; Guerra et al., 2019). The main elements of XR as a learning environment include 3D rendering engine, sensor, physics engine, control algorithms, robot embodiments, and public API layer (Lamotte et al., 2010; Shah et al., 2018). The outcomes of these elements are virtual environments consists of a collection of dynamic, static, intelligent, and non-inteligent objects such as the ground, buildings, robots, and agents.
In the learning environment, a robot can be exposed to a variety of physical phenomena, such as air density, gravity, magnetic fields, and air pressure (Lamotte et al., 2010). In this case, the physics engine can simulate and determine physical phenomena in the environment (Shen et al., 2020). The included papers have used different physics and graphics engines (simulators) including PHYSX, Bullet, Open Dynamics Engine, Unreal Engine, Unity, CARLA, AirSim, Gazebo, iGibson, Madras, VISTA, FlightGoggles for developing virtual worlds. Multiple advancements have also recently enabled the development of a more efficient learning environment for training AI. First, the rapid evolution of 3D graphics rendering engines has allowed for more sophisticated features including advanced illumination, volumetric lighting, real-time reflection, and improved material shaders (Guerra et al., 2019). Second, advanced motion capture facilities, such as laser tracking, infrared cameras, and ultra-wideband radio, have enabled precise tracking of human behavior and robots (Guerra et al., 2019).
In the learning environments, agents expose to variety of generated labeled and unlabeled data and they learn to control physical interactions and motion, navigate according to sensor signals, change the state of the virtual environment toward the desired configuration, or plan complex tasks (Shen et al., 2020). However, for training robust AI, learning environments must address several challenges, including transferring knowledge from XR to the real world; developing complex, stochastic, realistic scenes; and developing fully interactive, multi-AI agent, scalable 3D environments.
Transferring Knowledge
One of the main challenges is transferring the results from learning environments to the real world. Complicated aspects of human behavior, physical phenomena, and robot dynamics can be very challenging to precisely capture and respond to in the real world (Guerra et al., 2019; Amini et al., 2020). In addition, determining (by calculating percentages) whether the results obtained from a learning environment are sufficient for real-world application is difficult (Gaidon et al., 2016). One method to address this challenge is domain adaptation, a process enabling an AI agent trained on a source domain to generalize to a target domain (Bousmalis et al., 2018). For the AI-XR combination, the target domain is the real world, and the source domain is XR. Two types of domain adaptation methods are pixel-level and feature-level adaptation.
Complexity
Another challenge is the lack of complexity in learning environments. A lack of real-world semantic complexity in a learning environment can cause the AI to be trained insufficiently to run in the real world (Kurach et al., 2020). Many learning environments offer simplified modes of interaction, a narrow set of tasks, and small-scale scenes. A lack of complexity, specifically simplified modes of interaction, has been reported to lead to difficulties in applying AI in real-world situations (Dosovitskiy et al., 2017; Shen et al., 2020). For example, training autonomous cars in simple learning environments is not applicable to extensive driving scenarios, varying weather conditions, and exploration of roads. However, newly developed learning environments can add more complexity by using repositories of sparsely sampled trajectories (Amini et al., 2020). For each trajectory, learning environments synthesize many views that enable AI agents to train on acceptable complexity.
One aspect of complexity in learning environments is stochasticity. Many commonly used learning environments are deterministic. Because the real world is stochastic, robots and self-driving cars must be trained in uncertain dynamics. To increase robustness in the real world, newly developed learning environments expose AI (the learning agent) to a variety of random properties of the environment such as varying weather, sun positions, and different visual appearances and objects (e.g., lanes, roads, or buildings) (Gaidon et al., 2016; Amini et al., 2020; Shen et al., 2020). However, in many learning environments, this artificial randomness has been found to be too structured and consequently insufficient to train robust AI (Kurach et al., 2020).
Another aspect of complexity in learning environments is creating realistic scenes. Several learning environments including different annotated elements of the environment with photorealistic materials to create scenes close to real-world scenarios (Shen et al., 2020). These learning environments use scene layouts from different repositories and annotated objects inside the scene. They use various materials (such as marble, wood, or metal) and consider mass and density in the annotation of objects (Shen et al., 2020). However, some included articles have reported the creation of a completely interactive environment de novo without using other repositories.
Interactive or Non-interactive
Some learning environments are created only for navigation and have non-interactive assets. In these environments, the AI agent cannot interact with scenes, because each scene consists of a single fully rigid object. However, other learning environments support interactive navigation, in which agents can interact with the scenes. In newly developed learning environments, objects in scenes can be annotated with the possible actions that they can receive.
Single or Multiple Agents
In many learning environments, only one agent can be trained and tested. However, one way to train robust AI involves implementing several collaborative or compete AI agents (Kurach et al., 2020). In multi-agent learning environments, attention must be paid to communication and interactions between agents. In this complex interaction, optimizing the behavior of the learning AI agent is highly challenging. Furthermore, the appearance and behavior of adversary agents in learning environments must be considered. Implementing kinematic parameters and advanced controllers to govern these agents has been reported in several studies (Dosovitskiy et al., 2017).
Sensorimotor
Sensorimotor control in a 3D virtual world is another challenging aspect of learning environments. For example, challenging states for sensorimotor control in autonomous cars include exploring densely populated virtual environments, tracking multi-agent dynamics at urban intersections, recognizing prescriptive traffic rules, and rapidly reconciling conflicting objectives (Dosovitskiy et al., 2017).
Open-Source Licenses
To test new research ideas, learning environments must be open-source licenses so that researchers can modify the environments. However, many advanced environments and physics simulators offer restricted licenses (Kurach et al., 2020).
Scalable
Finally, developing scalable learning environments is another concern of researchers. Creating learning environments that do not require operating and storing virtual 3D data for entire cities or environments is important (Amini et al., 2020).
In conclusion, some advanced features in learning environments include:
• Fully interactive realistic 3D scenes
• Ability to import additional scenes and objects
• Ability to operate without storing enormous amounts of data
• Flexible specification of sensor suites
• Realistic virtual sensor signals such as virtual LiDAR signals
• High-quality images from a physics-based renderer
• Flexible specification of cameras and their type and position
• Provision of a variety of camera parameters such as 3D location and 3D orientation
• Pseudo-sensors enabling ground-truth depth and semantic segmentation
• Ability to have endless variation in scenes
• Human-environment interface for humans (fully physical interactions with the scenes)
• Provision of open digital assets (urban layouts, buildings, or vehicles)
• Provision of a simple API for adding different objects such as agents, actuators, sensors, or arbitrary objects
Guidelines
In general, the combination of AI-XR can be used for two main objectives, i.e.: 1) AI serving and assisting XR and 2) XR serving and assisting AI as it is represented in Figure 6.
AI Serving XR
AI consists of different subdomains, including the following: 1) ML that teaches a machine to make a decision based on identifying patterns and analyzing past data; 2) DL that processes input data through different layers to identify and predict the outcome; 3) Neural Networks (NNs) that process the input data in a way that the human brain does; 4) NLP which is about understanding, reading, and interpreting a human language by a machine; and 5) Computer Vision (CV) that tries to understand, recognize and classify images and videos (Great Learning, 2020). Among included papers, AI served XR in order to 1) detect patterns of XR generated data by using ML methods and 2) improving XR experience by using NNs and NLP methods.
Using AI to detect patterns of XR-generated data is very common among included papers. This type of AI-XR combination can be divided into two main categories 1) non-interactive: simply feeding the results of XR into AI and detecting the patterns, and 2) interactive: feeding XR generated data into AI, identifying the pattern, and returning results to XR. While non-interactive method can help to extract specific metrics indicating XR’s user performance, the interactive category can be used for the optimization of process parameters. In this category, AI can analyze different modes of XR and return feedback to XR.
Several articles focused on improving the XR experience by adding NLP and DL. In this objective, AI can be added to a specific part of XR and provide various advantages to the XR experience. For example, in virtual patients, adding NLP can bring an ability to understand human speech and hold a dialogue. Furthermore, in gaming applications, implementing AI-based objects can increase the randomness of a game.
XR Serving AI
Data availability is one of the main concerns of AI developers (Davahli et al., 2021). Despite significant efforts in collecting and releasing datasets, most data might have different deficiencies, such as, missing data in datasets, lack of coverage of rare and novel cases, high-dimensionality with small sample sizes, lack of appropriately labeled data, and data contamination with artifacts (Davahli et al., 2021). Furthermore, most data are generally collected for operations but not specifically for AI research and training. In this situation, XR can be used as an additional source for generating high-quality AI-ready data. These data can be rich, cover rare and novel cases, and extend beyond available datasets.
In general, XR can be used for various applications such as medical education, improving patient experiences, helping individuals to understand their emotions, handling dangerous materials, remote training, visualizing complex products and compounds, building effective collaborations, training workers in safe environments, virtual exhibitions, and entertainment, and considering as a computational platform (Forbes Technology Council, 2020). However, in the “XR serving AI” objective, XR can have a new function and generate AI-ready data. By using XR, AI can learn and become well-trained before implementing in the physical world. The main advantages of using XR for training AI systems are including 1) the entire training can occur in XR without collecting data from the physical world, 2) learning in XR can be fast, and 3) XR allows AI developers to simulate novel cases for training AI systems.
Even though the reviewed articles describe applications of XR for training AI systems in different areas, including autonomous cars, robots, gaming applications, and armed force training; surprisingly, no study focused on the healthcare area. In healthcare, data privacy plays an important role in developing and implementing AI. Because of the complexity of protecting data in healthcare, data privacy has had significant impact on increasing data availability. In this situation, AI can be trained in virtual environments containing patient demographics, disease states, and health conditions.
Although majority of included papers used XR to train AI, XR can be used to improve performance of AI by 1) validating the results and verifying hypotheses generated by AI systems, and 2) offering advanced visualization of AI structure. For example, in drug and antibiotic discovery where AI systems propose new compounds, XR can be used to compute the different properties of discovered drugs.
Limitations
We realize that not all potentially relevant papers to this review may have been included. First, even though we used the most related set of keywords, some relevant articles might not have been identified due to the limited number of keywords. Second, for each search keyword, we only screened links or articles until the 350th record. Third, we used only one round of citation-chaining to screen references. More citation-chaining could have identified additional papers relevant to AI and XR synergy. Finally, we used a limited number of bibliographic databases for records discovery.
Conclusion
This article comprehensively reviewed the published literature relevant to the intersection of themes related to the combination of AI and XR domains. By following PRISMA guidelines and using targeted keywords, we identified 36 articles that met the specific inclusion criteria. The examined papers were categorized into three main groups: 1) the interrelations of AI and XR dynamics, 2) the influence of AI on making XR applications more useful and valuable in a variety of domains, and 3) common AI and XR training issues. We also identified the main applications of the AI-XR combination technologies, including advanced visualization methods, autonomous cars, robotics, military, and medical training, cancer diagnosis, entertainment and gaming applications, smart homes, affective computing, and driver education, and training. The study results point to the growing importance of the interrelationships between AI and XR technology developments and the future prospects for their extensive applications in business, industry, government, and education.
Data Availability Statement
The original contributions presented in the study are included in the article/Supplementary Material, further inquiries can be directed to the corresponding author.
Author Contributions
DR and MD: Methodology and writing—original draft and revisions; WK, CC-N: Conceptualization, writing—review and revisions, editing, and supervision. All authors equally contributed to this research.
Conflict of Interest
The authors declare that the research was conducted in the absence of any commercial or financial relationships that could be construed as a potential conflict of interest.
Publisher’s Note
All claims expressed in this article are solely those of the authors and do not necessarily represent those of their affiliated organizations, or those of the publisher, the editors and the reviewers. Any product that may be evaluated in this article, or claim that may be made by its manufacturer, is not guaranteed or endorsed by the publisher.
References
Amini, A., Gilitschenski, I., Phillips, J., Moseyko, J., Banerjee, R., Karaman, S., et al. (2020). Learning Robust Control Policies for End-To-End Autonomous Driving from Data-Driven Simulation. IEEE Robot. Autom. Lett. 5, 1143–1150. doi:10.1109/LRA.2020.2966414
Bicakci, S., and Gunes, H. (2020). Hybrid Simulation System for Testing Artificial Intelligence Algorithms Used in Smart Homes. Simul. Model. Pract. Theor. 102, 101993. doi:10.1016/j.simpat.2019.101993
Bissonnette, V., Mirchi, N., Ledwos, N., Alsidieri, G., Winkler-Schwartz, A., and Del Maestro, R. F. (2019). Artificial Intelligence Distinguishes Surgical Training Levels in a Virtual Reality Spinal Task. J. Bone Jt. Surg. 101, e127. doi:10.2106/JBJS.18.01197
Bousmalis, K., Irpan, A., Wohlhart, P., Bai, Y., Kelcey, M., Kalakrishnan, M., et al. (2018). “Using Simulation and Domain Adaptation to Improve Efficiency of Deep Robotic Grasping,” in 2018 IEEE international conference on robotics and automation (ICRA), Brisbane, Australia, May 20–25, 2018 (IEEE), 4243–4250. doi:10.1109/ICRA.2018.8460875
Bower, M., Howe, C., McCredie, N., Robinson, A., and Grover, D. (2014). Augmented Reality in Education - Cases, Places and Potentials. Educ. Media Int. 51, 1–15. doi:10.1080/09523987.2014.889400
Caudell, T. P., Summers, K. L., Holten, J., Hakamata, T., Mowafi, M., Jacobs, J., et al. (2003). Virtual Patient Simulator for Distributed Collaborative Medical Education. Anat. Rec. 270B, 23–29. doi:10.1002/ar.b.10007
Cavazza, M., and Palmer, I. (2000). High-level Interpretation in Virtual Environments. Appl. Artif. Intelligence 14, 125–144. doi:10.1080/088395100117188
Chen, P.-H. C., Gadepalli, K., MacDonald, R., Liu, Y., Kadowaki, S., Nagpal, K., et al. (2019). An Augmented Reality Microscope with Real-Time Artificial Intelligence Integration for Cancer Diagnosis. Nat. Med. 25, 1453–1457. doi:10.1038/s41591-019-0539-7
Davahli, M. R., Karwowski, W., Fiok, K., Wan, T., and Parsaei, H. R. (2021). Controlling Safety of Artificial Intelligence-Based Systems in Healthcare. Symmetry 13 (1), 102. doi:10.3390/sym13010102
Dosovitskiy, A., Ros, G., Codevilla, F., Lopez, A., and Koltun, V. (2017). “CARLA: An Open Urban Driving Simulator,” in Conference on robot learning (PMLR), Mountain View, CA, November 13–15, 2017, 1–16. Available at: http://proceedings.mlr.press/v78/dosovitskiy17a.html.
Ehteshami Bejnordi, B., Veta, M., Johannes van Diest, P., van Ginneken, B., Karssemeijer, N., Litjens, G., et al. (2017). Diagnostic Assessment of Deep Learning Algorithms for Detection of Lymph Node Metastases in Women with Breast Cancer. JAMA 318, 2199. doi:10.1001/jama.2017.14585
Ershad, M., Rege, R., and Fey, A. M. (2018). Meaningful Assessment of Robotic Surgical Style Using the Wisdom of Crowds. Int. J. CARS 13, 1037–1048. doi:10.1007/s11548-018-1738-2
Esteva, A., Kuprel, B., Novoa, R. A., Ko, J., Swetter, S. M., Blau, H. M., et al. (2017). Dermatologist-level Classification of Skin Cancer with Deep Neural Networks. nature 542, 115–118. doi:10.1038/nature21056
Forbes Technology Council (2020). Council Post: 15 Effective Uses of Virtual Reality for Businesses and Consumers. Forbes. Available at: https://www.forbes.com/sites/forbestechcouncil/2020/02/12/15-effective-uses-of-virtual-reality-for-businesses-and-consumers/ (Accessed May 7, 2021).
Freina, L., and Ott, M. (2015). “A Literature Review on Immersive Virtual Reality in Education: State of the Art and Perspectives,” in The international scientific conference elearning and software for education, Bucharest, Romania, November, 2015, 10–1007. Available at: http://citeseerx.ist.psu.edu/viewdoc/summary?doi=10.1.1.725.5493.
Gaidon, A., Wang, Q., Cabon, Y., and Vig, E. (2016). “VirtualWorlds as Proxy for Multi-Object Tracking Analysis,” in Proceedings of the IEEE conference on computer vision and pattern recognition, Las Vegas, NV, June 26–July 1, 2016, 4340–4349. doi:10.1109/CVPR.2016.470
Great Learning (2020). Medium. What Is Artificial Intelligence? How Does AI Work and Future of it. Available at: https://medium.com/@mygreatlearning/what-is-artificial-intelligence-how-does-ai-work-and-future-of-it-d6b113fce9be (Accessed March 7, 2021).
Guerra, W., Tal, E., Murali, V., Ryou, G., and Karaman, S. (2019). Flightgoggles: Photorealistic Sensor Simulation for Perception-Driven Robotics Using Photogrammetry and Virtual Reality. ArXiv Prepr. ArXiv190511377. doi:10.1109/IROS40897.2019.8968116
Gulshan, V., Peng, L., Coram, M., Stumpe, M. C., Wu, D., Narayanaswamy, A., et al. (2016). Development and Validation of a Deep Learning Algorithm for Detection of Diabetic Retinopathy in Retinal Fundus Photographs. Jama 316, 2402–2410. doi:10.1001/jama.2016.17216
Gutiérrez-Maldonado, J., Alsina-Jurnet, I., Rangel-Gómez, M. V., Aguilar-Alonso, A., Jarne-Esparcia, A. J., Andrés-Pueyo, A., et al. (2008). “Virtual Intelligent Agents to Train Abilities of Diagnosis in Psychology and Psychiatry,” in New Directions in Intelligent Interactive Multimedia (Piraeus, Greece: Springer), 497–505. Available at:. doi:10.1007/978-3-540-68127-4_51
Hamet, P., and Tremblay, J. (2017). Artificial Intelligence in Medicine. Metabolism 69, S36–S40. doi:10.1016/j.metabol.2017.01.011
Hilleli, B., and El-Yaniv, R. (2018). “Toward Deep Reinforcement Learning without a Simulator: An Autonomous Steering Example,” in Proceedings of the AAAI Conference on Artificial Intelligence, New Orleans, LA, February 2–7, 2018. Available at: https://ojs.aaai.org/index.php/AAAI/article/view/11490.
Israelsen, B., Ahmed, N., Center, K., Green, R., and Bennett, W. (2018). Adaptive Simulation-Based Training of Artificial-Intelligence Decision Makers Using Bayesian Optimization. J. Aerospace Inf. Syst. 15, 38–56. doi:10.2514/1.I010553
Jobin, A., Ienca, M., and Vayena, E. (2019). The Global Landscape of AI Ethics Guidelines. Nat. Mach. Intell. 1, 389–399. doi:10.1038/s42256-019-0088-2
Jog, A., Itkowitz, B., May Liu, M., DiMaio, S., Hager, G., Curet, M., and Kumar, R. (2011). “Towards Integrating Task Information in Skills Assessment for Dexterous Tasks in Surgery and Simulation,” in 2011 IEEE International Conference on Robotics and Automation, Shanghai, China, May 9–13, 2011 (IEEE), 5273–5278. doi:10.1109/ICRA.2011.5979967
Kaplan, A. D., Cruit, J., Endsley, M., Beers, S. M., Sawyer, B. D., and Hancock, P. A. (2020). The Effects of Virtual Reality, Augmented Reality, and Mixed Reality as Training Enhancement Methods: a Meta-Analysis. Hum. Factors 63, 706–726. doi:10.1177/0018720820904229
Kerwin, T., Wiet, G., Stredney, D., and Shen, H.-W. (2012). Automatic Scoring of Virtual Mastoidectomies Using Expert Examples. Int. J. CARS 7, 1–11. doi:10.1007/s11548-011-0566-4
Koenig, N., and Howard, A. (2004). “Design and Use Paradigms for Gazebo, an Open-Source Multi-Robot Simulator,” in 2004 IEEE/RSJ International Conference on Intelligent Robots and Systems (IROS)(IEEE Cat. No. 04CH37566), Sendai, Japan, September 28–October 2, 2004 (IEEE), 2149–2154. doi:10.1109/IROS.2004.1389727
Kopp, S., Jung, B., Lessmann, N., and Wachsmuth, I. (2003). Max-a Multimodal Assistant in Virtual Reality Construction. KI 17, 11.
Krizhevsky, A., Sutskever, I., and Hinton, G. E. (2017). Imagenet Classification with Deep Convolutional Neural Networks. Commun. ACM 60, 84–90. doi:10.1145/3065386
Kurach, K., Raichuk, A., Stańczyk, P., Zając, M., Bachem, O., Espeholt, L., Riquelme, C., Vincent, D., Michalski, M., Bousquet, O., and Gelly, S. (2020). “Google Research Football: A Novel Reinforcement Learning Environment,” in Proceedings of the AAAI Conference on Artificial Intelligence, New York, NY, February 7–12, 2020, 4501–4510. doi:10.1609/aaai.v34i04.5878
Lamotte, O., Galland, S., Contet, J.-M., and Gechter, F. (2010). “Submicroscopic and Physics Simulation of Autonomous and Intelligent Vehicles in Virtual Reality,” in 2010 Second International Conference on Advances in System Simulation, Nice, France, August 22–27, 2010 (IEEE), 28–33. doi:10.1109/SIMUL.2010.19
Latoschik, M. E., Biermann, P., and Wachsmuth, I. (2005). “Knowledge in the Loop: Semantics Representation for Multimodal Simulative Environments,” in International Symposium on Smart Graphics (Frauenwörth, Germany: Springer), 25–39. doi:10.1007/11536482_3
Liang, H., and Shi, M. Y. (2011). “Surgical Skill Evaluation Model for Virtual Surgical Training,” in Applied Mechanics and Materials (Trans Tech Publ), 812–819. doi:10.4028/www.scientific.net/AMM.40-41.812
Liberati, A., Altman, D. G., Tetzlaff, J., Mulrow, C., Gøtzsche, P. C., Ioannidis, J. P. A., et al. (2009). The PRISMA Statement for Reporting Systematic Reviews and Meta-Analyses of Studies that Evaluate Health Care Interventions: Explanation and Elaboration. PLOS Med. 6, e1000100. doi:10.1371/journal.pmed.1000100
Loukas, C., and Georgiou, E. (2011). Multivariate Autoregressive Modeling of Hand Kinematics for Laparoscopic Skills Assessment of Surgical Trainees. IEEE Trans. Biomed. Eng. 58, 3289–3297. doi:10.1109/TBME.2011.2167324
Marcus, G. (2018). Deep Learning: A Critical Appraisal. ArXiv Prepr. ArXiv180100631. Available at: https://arxiv.org/abs/1801.00631 (Accessed April 8, 2021)
Marín-Morales, J., Higuera-Trujillo, J. L., Greco, A., Guixeres, J., Llinares, C., Scilingo, E. P., et al. (2018). Affective Computing in Virtual Reality: Emotion Recognition from Brain and Heartbeat Dynamics Using Wearable Sensors. Sci. Rep. 8, 13657. doi:10.1038/s41598-018-32063-4
Megali, G., Sinigaglia, S., Tonet, O., and Dario, P. (2006). Modelling and Evaluation of Surgical Performance Using Hidden Markov Models. IEEE Trans. Biomed. Eng. 53, 1911–1919. doi:10.1109/TBME.2006.881784
Meissler, N., Wohlan, A., Hochgeschwender, N., and Schreiber, A. (2019). “Using Visualization of Convolutional Neural Networks in Virtual Reality for Machine Learning Newcomers,” in 2019 IEEE International Conference on Artificial Intelligence and Virtual Reality (AIVR), San Diego Bay, CA, December 9–11, 2019 (IEEE), 152–1526. doi:10.1109/AIVR46125.2019.00031
National Heart, LungBlood Institute (NHLBI) (2019). Quality Assessment Tool for Observational Cohort and Cross-Sectional Studies. Bethesda, MD: National Heart, Lung, and Blood Institute. Available at: https://www.nhlbi.nih.gov/health-topics/study-quality-assessment-tools (Accessed May 24, 2019).
Newell, A., Shaw, J. C., and Simon, H. A. (1958). Elements of a Theory of Human Problem Solving. Psychol. Rev. 65, 151–166. doi:10.1037/h0048495
Richstone, L., Schwartz, M. J., Seideman, C., Cadeddu, J., Marshall, S., and Kavoussi, L. R. (2010). Eye Metrics as an Objective Assessment of Surgical Skill. Ann. Surg. 252, 177–182. doi:10.1097/SLA.0b013e3181e464fb
Ropelato, S., Zünd, F., Magnenat, S., Menozzi, M., and Sumner, R. (2018). Adaptive Tutoring on a Virtual Reality Driving Simulator. Int. Ser. Inf. Syst. Manag. Creat. Emedia Cremedia 2017, 12–17. doi:10.3929/ethz-b-000195951
Sadeghi, F., Toshev, A., Jang, E., and Levine, S. (2018). “Sim2real Viewpoint Invariant Visual Servoing by Recurrent Control,” in Proceedings of the IEEE Conference on Computer Vision and Pattern Recognition, Salt Lake City, UT, June 18–22, 2018, 4691–4699. doi:10.1109/CVPR.2018.00493
Sadeghi, A. H., Maat, A. P. W. M., Taverne, Y. J. H. J., Cornelissen, R., Dingemans, A.-M. C., Bogers, A. J. J. C., et al. (2021). Virtual Reality and Artificial Intelligence for 3-dimensional Planning of Lung Segmentectomies. JTCVS Tech. 7, 309–321. doi:10.1016/j.xjtc.2021.03.016
Samuel, A. L. (1959). Some Studies in Machine Learning Using the Game of Checkers. IBM J. Res. Dev. 3, 210–229. doi:10.1147/rd.33.0210
Santara, A., Rudra, S., Buridi, S. A., Kaushik, M., Naik, A., Kaul, B., et al. (2020). MADRaS: Multi Agent Driving Simulator. ArXiv Prepr. ArXiv201000993.
Sewell, C., Morris, D., Blevins, N., Dutta, S., Agrawal, S., Barbagli, F., et al. (2008). Providing Metrics and Performance Feedback in a Surgical Simulator. Comp. Aided Surg. 13, 63–81. doi:10.3109/1092908080195771210.1080/10929080801957712
Shah, S., Dey, D., Lovett, C., and Kapoor, A. (2018). “Airsim: High-Fidelity Visual and Physical Simulation for Autonomous Vehicles,” in Field and Service Robotics (Springer), 621–635. doi:10.1007/978-3-319-67361-5_40 (Accessed February 15, 2021)
Shen, B., Xia, F., Li, C., Martín-Martín, R., Fan, L., Wang, G., et al. (2020). iGibson, a Simulation Environment for Interactive Tasks in Large RealisticScenes. ArXiv Prepr. ArXiv201202924. Available at: https://arxiv.org/abs/2012.02924.
Strodthoff, N., and Strodthoff, C. (2019). Detecting and Interpreting Myocardial Infarction Using Fully Convolutional Neural Networks. Physiol. Meas. 40, 015001. doi:10.1088/1361-6579/aaf34d
Szolovits, P. (1988). Artificial Intelligence in Medical Diagnosis. Ann. Intern. Med. 108, 80. doi:10.7326/0003-4819-108-1-80
Talbot, T. B., Sagae, K., John, B., and Rizzo, A. A. (2012). Sorting Out the Virtual Patient. Int. J. Gaming Comput.-Mediat. Simul. IJGCMS 4, 1–19. doi:10.4018/jgcms.2012070101
Turan, E., and Çetin, G. (2019). Using Artificial Intelligence for Modeling of the Realistic Animal Behaviors in a Virtual Island. Comput. Stand. Inter. 66, 103361. doi:10.1016/j.csi.2019.103361
VanHorn, K. C., Zinn, M., and Cobanoglu, M. C. (2019). Deep Learning Development Environment in Virtual Reality. ArXiv Prepr. ArXiv190605925. Available at: https://arxiv.org/abs/1906.05925 (Accessed February 4, 2021).
Wang, F., and Preininger, A. (2019). AI in Health: State of the Art, Challenges, and Future Directions. Yearb. Med. Inform. 28, 016–026. doi:10.1055/s-0039-1677908
Wang, X., Peng, Y., Lu, L., Lu, Z., Bagheri, M., and Summers, R. M. (2017). “Chestx-ray8: Hospital-Scale Chest X-ray Database and Benchmarks on Weakly-Supervised Classification and Localization of Common Thorax Diseases,” in Proceedings of the IEEE conference on computer vision and pattern recognition, Honolulu, HI, July 21–26, 2017, 2097–2106. doi:10.1109/CVPR.2017.369
Warner, H. R., Toronto, A. F., Veasey, L. G., and Stephenson, R. (1961). A Mathematical Approach to Medical Diagnosis. JAMA 177, 177–183. doi:10.1001/jama.1961.03040290005002
Weidenbach, M., Trochim, S., Kreutter, S., Richter, C., Berlage, T., and Grunst, G. (2004). Intelligent Training System Integrated in an Echocardiography Simulator. Comput. Biol. Med. 34, 407–425. doi:10.1016/S0010-4825(03)00084-2
Weizenbaum, J. (1966). ELIZA-a Computer Program for the Study of Natural Language Communication between Man and Machine. Commun. ACM 9, 36–45. doi:10.1145/365153.365168
Keywords: artificial intelligence, extended reality, learning environment, autonomous cars, robotics
Citation: Reiners D, Davahli MR, Karwowski W and Cruz-Neira C (2021) The Combination of Artificial Intelligence and Extended Reality: A Systematic Review. Front. Virtual Real. 2:721933. doi: 10.3389/frvir.2021.721933
Received: 07 June 2021; Accepted: 23 August 2021;
Published: 07 September 2021.
Edited by:
David J. Kasik, Boeing, United StatesReviewed by:
Vladimir Karakusevic, Boeing, United StatesMary C. Whitton, University of North Carolina at Chapel Hill, United States
Alberto Raposo, Pontifical Catholic University of Rio de Janeiro, Brazil
Copyright © 2021 Reiners, Davahli, Karwowski and Cruz-Neira. This is an open-access article distributed under the terms of the Creative Commons Attribution License (CC BY). The use, distribution or reproduction in other forums is permitted, provided the original author(s) and the copyright owner(s) are credited and that the original publication in this journal is cited, in accordance with accepted academic practice. No use, distribution or reproduction is permitted which does not comply with these terms.
*Correspondence: Mohammad Reza Davahli, mohammadreza.davahli@ucf.edu
†These authors have contributed equally to this work and share first authorship