- Department of Communication, Cornell University, Ithaca, NY, United States
The ability to perceive emotional states is a critical part of social interactions, shaping how people understand and respond to each other. In face-to-face communication, people perceive others’ emotions through observing their appearance and behavior. In virtual reality, how appearance and behavior are rendered must be designed. In this study, we asked whether people conversing in immersive virtual reality (VR) would perceive emotion more accurately depending on whether they and their partner were represented by realistic or abstract avatars. In both cases, participants got similar information about the tracked movement of their partners’ heads and hands, though how this information was expressed varied. We collected participants’ self-reported emotional state ratings of themselves and their ratings of their conversational partners’ emotional states after a conversation in VR. Participants’ ratings of their partners’ emotional states correlated to their partners’ self-reported ratings regardless of which of the avatar conditions they experienced. We then explored how these states were reflected in their nonverbal behavior, using a dyadic measure of nonverbal behavior (proximity between conversational partners) and an individual measure (expansiveness of gesture). We discuss how this relates to measures of social presence and social closeness.
1 Introduction
Perceiving emotional states is a critical part of social interactions, shaping how people understand and respond to each other. In face-to-face communication, people’s perceptions of emotions depend on multiple factors including facial expressions (Montagne et al., 2007; Schirmer and Adolphs, 2017), and verbal (De Gelder and Vroomen, 2000, Doyle and Lindquist, 2017), and nonverbal behavior (Hertenstein et al., 2009; Enea and Iancu, 2016). As immersive technology becomes more prevalent, more interpersonal communication is occurring in social virtual reality platforms (McVeigh-Schultz et al., 2018). One important measure of user experience in social VR is thus the ability to perceive emotional states. In virtual reality, how an avatar appears and what behavior is tracked and rendered must be designed. This allows nonverbal behavior as well as other affective cues to be conveyed in ways that diverge from the usual human appearance. Thus, understanding the relationship between avatar appearance, how people recognize others’ emotions and how they express themselves can help guide the design of expressive avatars and the user experience elicited.
Previous research has found that both the appearance and the behavior of avatars impacts participants’ emotional reactions (Mousas et al., 2018). One study result showed that participants expressed different anxiety levels when giving a speech to virtual audiences depending on whether they presented negative, positive or neutral emotions (Pertaub et al., 2002). In another study, the rapport towards agent-avatars depended on an interaction between appearance and personality, meaning that under some circumstances, realism could be a better choice for avatars (Zibrek et al., 2018). The interaction of avatar realism and avatar appearance influences users’ social perceptions (Garau et al., 2003; Thomas et al., 2017; Nowak and Fox, 2018), including in the social domain (Ehrsson et al., 2005; Bailenson et al., 2006; Lugrin et al., 2015; Latoschik et al., 2017). This paper makes several contributions to understanding emotion perception in avatars. First, we describe two types of avatars, one abstract and one humanoid, both of which convey important qualities of nonverbal behavior. Second, we examine emotion recognition during social interactions in virtual reality, comparing partners’ accuracy with participants’ self-reports. Third, we examine how tracked nonverbal behaviors relates to emotion recognition. Finally, we relate emotion recognition to other measures of user experience, including social presence and social closeness.
Below we review the literature on avatar appearance and behavior, describe our experimental setup and results, and discuss the implications of these findings for avatar design.
1.1 Avatar Appearance and Behavior
To interact with other people in immersive VR platforms, people need to be embodied with virtual avatars. This opens up opportunities for people to create or choose virtual representations that may or may not resemble themselves. Such avatars can have different levels of consistency with the user’s own body, from realistic humanoid avatars that closely resemble the user’s physical body to abstract shapes with no customized features (Bailenson et al., 2006). People can even break traditional norms of embodiment, such as magnifying nonverbal cues (Yee et al., 2008) or remapping the avatars’ movement in a novel way (Won et al., 2015).
Avatar appearances not only reflect people’s virtual identities and their self-perceptions, but also can impact how they behave and interact with others (Garau, 2003; Roth et al., 2016). For example, the Proteus effect demonstrated that embodiment in an avatar could lead the user to behave according to their expectations of that avatar (Yee and Bailenson, 2007). Different avatar appearances also have been found to yield different interaction outcomes (Latoschik et al., 2017). People have responded to anthropomorphic avatars more positively and have been more willing to choose an avatar that reflects their gender (Nowak and Rauh, 2005). Some research suggests that customized avatars significantly improve users’ sense of presence and body ownership in virtual reality (Waltemate et al., 2018). However, due to the limitations of avatar creation and control, some avatar appearances may fall into the “uncanny valley,” such that the representations, though closely resembling humans, are not quite real enough to be acceptable (Mori, 1970). For example, researchers found that avatar realism created “eeriness” that influenced people’s accuracy to judge extroversion and agreeableness as personality traits (Shin et al., 2019). On the other hand, other research has found that avatars with human appearance elicit a slightly lower ‘illusion of virtual body ownership’ compared to machine-like and cartoon-like avatars (Lugrin et al., 2015). To continue these investigations we aimed to explore how avatars of varying realism that gave similar nonverbal information might affect emotion recognition.
1.2 Emotion Perception From Movement
Though facial expressions and voice are important channels for emotion recognition (Banse and Scherer, 1996), bodily movements and posture also convey critical emotional cues (Dael et al., 2012). Gesture is an integral part of nonverbal communication that conveys emotion (Dael et al., 2013). Furthermore, body movement could also be used to predict people’s emotional ratings of others (De Meijer, 1989). By combining gestures and facial expressions, people were able to be more accurate in emotion recognition (Gunes and Piccardi, 2007), as people infer each other’s emotions through channels that process implicit messages (Cowie et al., 2001). Emotions can even be identified from minimal information about body movements, as in the phenomenon “point light display” (Atkinson et al., 2004; Clarke et al., 2005; Lorey et al., 2012). First introduced by Johnansson (Johansson, 1973), the phenomenon has been confirmed and evolved with new findings (Kozlowski and Cutting, 1977; Missana et al., 2015). For example, when body movements were exaggerated, recognition became more accurate and led to higher emotional ratings (Atkinson et al., 2004).
Researchers have found that Big Five personality traits are related to verbal and nonverbal behavior in VR (Yee et al., 2011). Biological motion also correlated to personality traits. For example, with minimal movement information that people relied on to make the first impression, people were able to predict others’ perceived personality (Koppensteiner, 2013). Even when the personality traits were inferred from a thin slice of movement, there is a significant correlation with the information provided by knowledgeable informants (Borkenau et al., 2004). Understanding others’ personality traits is important because it influenced how people felt about their interactions with others, and how they evaluated themselves and others in virtual reality (Astrid et al., 2010). Some personality traits that exhibited specific movement patterns were predictive of people’s evaluations (Astrid et al., 2010). In terms of social interactions with a virtual character, a study showed that certain personality traits were found to be more easily inferred from the avatars and those avatars also revealed accurate information about specific personality traits of people who created them (Fong and Mar 2015). How much people like the virtual characters depended on an interaction between the virtual avatars’ appearances and personalities (Zibrek et al., 2018). Thus, in our study, we asked participants to report on both emotional state and personality trait for themselves and their partners.
Researchers have proposed various ways to analyze behavior in virtual reality using the movement data provided by trackers in virtual reality headsets and hand controllers (Kobylinski et al., 2019). Using movement data, researchers found that people who performed a communication task better in virtual reality had more movement using their gestures as an aid to communicate (Dodds et al., 2010). Additionally, when people interacted with humans or virtual agents with open gestures in a mixed reality platform, they were more willing to interact and they reported being more engaged in the experience (Li et al., 2018). Mimicry behavior, known as the “chameleon effect,” showed that when people interacted with a virtual agent that mimicked them with a time lag, these agents received more positive ratings on their traits (Bailenson and Yee, 2005; Tarr et al., 2018). We thus ask how emotion can be perceived through participants’ movements when they are rendered through an avatar, and whether a humanoid or abstract avatar appearance makes a difference if the movement data conveyed is equivalent.
1.3 Current Study
This paper describes the second part of a pre-registered study on nonverbal behavior and collaboration in immersive virtual reality (Sun et al., 2019). In the first paper from this study, we followed our pre-registered hypotheses and research questions to examine whether nonverbal synchrony would emerge naturally during conversations in virtual reality; if it would differ depending on the types of avatars participants used, and if nonverbal synchrony would be linked to task success. Stronger positive and negative correlations between real pairs compared to an artificial “pseudosynchrony” pairs were found supporting the hypothesis that nonverbal synchrony occurred naturally in dyadic conversations in virtual reality. Though there was no significant correlation between the task success and nonverbal synchrony, we found a positive significant correlation between social closeness and nonverbal synchrony. In this second paper, we address the remaining research question and add exploratory analyses to address the relationship between emotion recognition, individual and dyadic measures of nonverbal behavior (specifically, proximity and openness of gesture) and avatar appearance. We defined open gestures as the expansiveness of participants’ hand movements, and operationalized this measure as the distance between the participants’ hands. We asked participants to self-report their own emotional states, as well as to estimate their partners’ emotional states. These state measures were not analyzed elsewhere, and we analyze them now for the first time to answer the final research question of that pre-registration:
RQ4: Will there be an effect of appearance on emotion perception, such that a conversational partner perceives emotion differently depending on whether participants are represented by a cube or a realistic-looking avatar?
In our study, we designed two conditions with different avatar appearances to convey approximately the same information about users’ posture and gestures. One avatar was humanoid, and the other cube-shaped. Because we used consumer headsets, we were limited to tracking data derived from the position of the headset, and the position of the two hand controllers. In both the humanoid and cube avatars, the position of the avatar was linked to the head tracker. Similarly, we aimed to provide equivalent information about the position of the hands. The humanoid avatar hands followed the position of the participants’ hand controllers. In the cube avatar, the sides of the cube scaled depending on where the participant held the hand tracker. Thus, we explored two measures of nonverbal behavior that were clearly related to this positional or postural information. One behavior was measured on the pair level: proximity, or the distance between the two participants in a pair (Won et al., 2018). The other measure was on the individual level: expansiveness of gesture, or how far apart an individual participant held his or her hands (Li et al., 2018).
In addition to answering this question, we conducted exploratory analyses on participants’ tracked movements and related these to participants’ self-reported emotional states.
Finally, we also collected participants’ self-reported personality traits and their ratings of their partners as part of a larger on-going study. While we did not pre-register research questions for these data, we did duplicate the analyses for emotional states with these data. We present those results in the supplementary materials in Appendix B.
The complete data set can be found here (https://doi.org/10.6077/xvcp-p578) and is available on submission of an approved IRB protocol to the archive.
2 Methods and Materials
2.1 Participants
This study was approved by the Institutional Review Board and all participants gave informed consent. We excluded one pair of participants due to motion sickness and one pair who reported being close friends or relatives. After removing participants with missing movement data, due to sensor issues, there were 76 pairs of participants left for the analyses.
Participants (n = 152) were 49 males, 102 females and one participant who preferred not to reveal their gender. Participants were randomly assigned to pairs, resulting in 33 male-female pairs, eight male-male pairs, and 34 female-female pairs, and one pair whose gender composition was not revealed. When describing their race/ethnicity, 12 participants described themselves as African Americans, 68 as Asian or Pacific Islanders, 69 as Caucasians as multi-racial, 7 people selected more than one race/ethnicity, eight people described themselves as “other” and three people chose “I prefer not to answer this question.” Participants received course credits or cash compensation for the experiment. 41 pairs of participants were assigned to the humanoid avatar condition, and 35 pairs of participants to the cube avatar condition.
2.2 Apparatus
Participants wore Oculus Rifts and held Oculus Touch hand controllers. Movement data from these components were stored on a database on a local server. The experimental environment was created using the game engine Unity 3D.
2.3 Data Collection Procedure
A tracking platform saved the movement data from both participants at 30 times per second. Data timestamping was done on the movement tracking platform to avoid discrepancies arising from using each client’s individual system clock. The setup for the tracking platform, its architecture, and the procedures used to minimize noise due to latency are described in detail in (Shaikh et al., 2018).
2.4 Experiment Flow
Each participant visited the lab twice. First, they visited the lab to be photographed. The photographs were taken in a passport style with no glasses and in neutral lighting.
Participants were then randomly assigned to one of two avatar appearance conditions; humanoid avatar and cube avatar. If participants were assigned to the humanoid avatar conditions, research assistants then created their avatars based on their photos, using the procedure described in Shaikh et al. (2018). In the humanoid avatar condition, participants could see their hands and bodies from the first person perspective. In the abstract cube condition, participants were represented by generic white cubes with no personalized features.
2.4.1 Second Visit: The Experiment
At their second visit, participants were instructed to go to two different lab rooms on different sides of the building so that they did not see each other in person before the experiment. A research assistant in each room assisted participants with the head-mounted display and the hand controllers. Participants completed the remainder of the task in a minimal networked virtual environment consisting of a plain white platform with directional lighting.
Participants first experienced their avatar body in a solitary mirror scene. They performed three exercises: raising up their arms, holding their arms wide and then folding them, and stepping toward and back from the mirror. These exercises helped the participants to gain a sense of embodiment in the assigned avatars.
In the humanoid avatar condition, the avatar’s head and hands followed the movement of the head and hand trackers, while the rest of the avatar body was animated by inverse kinematics (Tolani et al., 2000). Participants could see avatars customized with their own faces in the first, mirror scene, but during the interaction, which occurred in a separate scene without a mirror, could only see avatar hands and the rest of their avatar bodies from the first person perspective.
In the abstract cube condition, the volume of the cube avatar shrank or grew as participants moved their hands. In other words, each cube got bigger or smaller as participants moved the hand controllers closer together or farther apart. In addition, the angle and position of the cube followed the angle and position of the participant’s head. Participants could see their own shadows as well as the avatars and shadows of conversational partners.
After the mirror scene, participants were then connected to their conversational partners in a mirror-less environment. Figures 1, 2 show the humanoid avatar pairs and the abstract cube pairs. Participants then completed a brainstorming task, either competing or collaborating with their conversational partners. While participants’ ideas were scored to answer earlier hypotheses, they are not further discussed in this paper. Collaborative and competitive conditions differed only in small variations in the verbal instructions to participants. For the purposes of this analysis, we collapse across conditions.
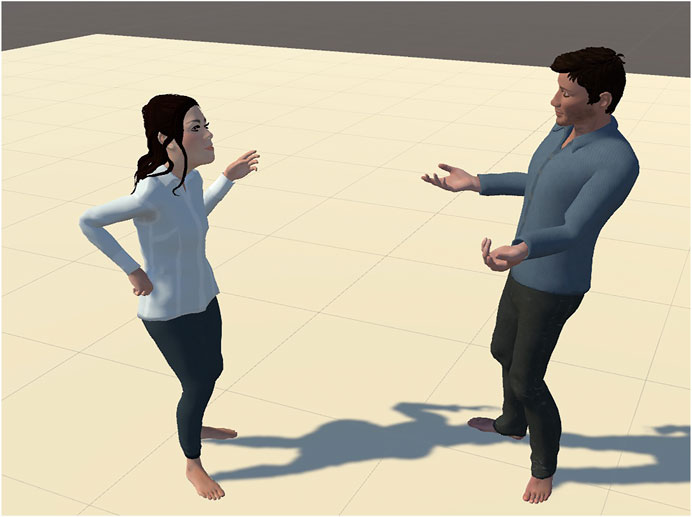
FIGURE 1. The figure shows the third person view of two customized humanoid avatars in the brainstorming task.
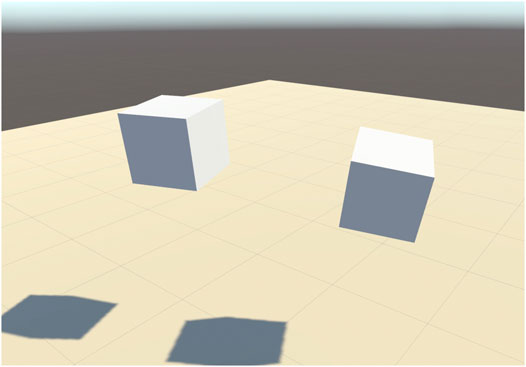
FIGURE 2. The figure shows the third person view of two abstract cube avatars in the brainstorming task.
After the 5-min brainstorming task in VR, participants were directed to another laptop to complete a post-test survey.
2.5 Measures
Below, we list the measures of emotional accuracy. In response to this issue’s call to reanalyze previous research from a new perspective, we also have reanalyzed the measures of presence found in Sun et al. (2019) as well as the Witmer and Singer immersion measures which were not previously analyzed.
2.5.1 Social Closeness
In order to have a proxy for rapport, we used a measure of social closeness that was previously collected but had not previously been used in emotion recognition analysis. Following previous work on social closeness, we asked 10 questions on liking, affiliation, and connectedness (Won et al., 2018) (alpha = 0.92). We averaged 10 questions for each individual, and averaged these with their partners’ scores to create a pair-level social closeness measure (M = 3.429, SD = 0.586). Unlike the other measures discussed, this measure was previously correlated with nonverbal synchrony in the previous paper.
2.5.2 Emotional State
In the post-experiment questionnaire, participants were asked to rate to what extent the 18 emotional adjectives represent their current feelings and states. Then they were asked to rate how they guessed the same adjectives would represent their conversational partners’ feelings and states. The rating was on a 4-point scale (1 = disagree strongly to 4 = agree strongly).
This questionnaire was adapted from the UWIST mood checklist (Matthews et al., 1990). The 18 adjectives were grouped into three categories: hedonic tone items, tense arousal items, and energetic arousal items. For each item there were three positive and three negative adjectives respectively. To process the data, we first reverse coded the ratings for the negative adjectives. Then we categorized the adjectives into three groups for each participant and took the average of the adjective ratings in that group to create three new variables: hedonic tone, tense arousal, and energetic arousal. For each individual, we thus had their self-rating of their emotional state, and their other-rating of their partner’s emotional state. For each group, we created the group self ratings on hedonic (M = 2.439, SD = 0.587), tense (M = 1.823, SD = 0.548) and energetic (M = 2.965, SD = 0.595) states as well as the group partner ratings hedonic (M = 2.253, SD = 0.553), tense (M = 1.837, SD = 0.462) and energetic (M = 3.034, SD = 0.536) states by averaging both participants’ self ratings and their ratings on their partners respectively.
To create the emotional consensus measure, we calculated the correlation between two participants in a pair’s emotional ratings. First we calculated the correlation between Participant A’s eighteen self emotional ratings and Participant B’s emotional ratings to A. Then vice versa to get Participant B’s eighteen self-ratings. The emotional consensus score for the pair is the average of these two ratings (M = 0.298, SD = 0.251).
There is at least one other way to score participants’ accuracy on ratings of emotional state. This could be done by calculating the difference between the two participants’ ratings, as follows. First we calculated the difference between Participant A’s self emotional ratings and Participant B’s emotional ratings of Participant A. Then vice versa to get Participant B’s self-ratings and Participant A’s ratings of Participant B. The pairwise emotional recognition score is thus the average of these two ratings, or how close each person’s rating of their partner was to the “ground truth” of their partner’s self-rating (M = 0.827, SD = 0.230). This method produces very similar results to the measure of emotional accuracy described above, so for brevity, we describe those outcome measures in Appendix A.
2.5.3 Personality Traits
In the post experiment questionnaire, the participants were also asked to choose the extent to which they agree or disagree with ten personality traits that might or might not apply to them. Participants were also asked to rate the same ten personality traits that may or may not apply to their conversational partners. As with the emotional state measures, these ratings were then calculated to create both individual and pair measures. The ratings were on a 7-point scale (1 = disagree strongly to 7 = agree strongly). The questionnaire was adapted from Ten Item Personality Measure (TIPI) (Gosling et al., 2003). For each individual, we thus had their self-rating of their personality traits, and their other-rating of their partner’s personality traits.
To create the trait consensus measure, we calculated the correlation between two participants in a pair’s personality traits ratings. First we calculated the correlation between Participant A’s self-rating of personality traits and Participant B’s rating of A’s personality traits. Then, we repeated that calculation in reverse to get Participant B’s ratings. The personality traits score for the pair is the average of these two ratings (M = 0.394, SD = 0.265).
2.5.4 User Experience/Presence
In the post-experiment questionnaire, the participants were asked 10 questions about their user experience and sense of presence in the virtual environment (Witmer and Singer, 1998). Based on the categorizations of those questions described in Witmer et al. (2005), we broke these measures into five categories: adaptation/immersion, involvement, visual quality, audio quality and distraction. The detailed survey questions are listed in Table 1.
Adaptation/immersion
Due to the low internal consistency score for the three questions above (alpha = 0.51). The question “How much delay did you experience between your actions and expected outcomes?” was dropped for analysis, which increased the alpha to 0.69. For every participant in the pair, the adaptation/immersion score is the average of the two questions (M = 3.645, SD = 0.608).
Involvement
The questions about how “natural or compelling” the experience was are categorized based on Witmer et al. (2005). The internal consistency was low (alpha = 0.66). After dropping the question “How well could you concentrate on the assigned tasks or required activities rather than on the mechanisms used to perform those tasks or activities?”, the alpha was increased to 0.72, and this subset was then used for the rest of the analysis. For every participant in the pair, the involvement score was the average of the three questions (M = 2.414, SD = 0.859).
Visual quality
For every participant in the pair, the visual quality score is the average of this question (M = 2.151, SD = 1.031).
Audio quality
For every participant in the pair, the audio quality score is the average of this question (M = 3.217, SD = 1.150).
Distraction
For every participant in the pair, the distraction score is the average of this question (M = 2.795, SD = 1.133).
We compared these measures to two other measures of presence that were discussed in Sun et al. (2019), self-presence (alpha = 0.84) and social presence (alpha = 0.82).
Then, the self-presence scores and the social presence scores from two participants in a pair were averaged respectively to get the group’s self-presence scores (M = 2.277, SD = 0.656) and the group’s social presence scores (M = 3.086, SD = 0.594).
3 Results
Below, we report all analyses conducted on these measures. Some presence measures were examined on both the pair level (social closeness and social presence) while other measures were examined only on the individual level (self-presence and the Witmer and Singer immersion questions).
3.1 Emotion Recognition Accuracy and Avatar Appearance
We first sought to answer our final research question in our previous pre-registration link here: RQ4: Will there be an effect of appearance on emotion perception, such that a conversational partner perceives emotion differently depending on whether participants are represented by a cube or a realistic-looking avatar?
First, we explored whether there is a difference in how emotional states were perceived by participants depending on the appearance of the avatar used during their interaction. In other words, did participants who saw their partners represented by cubes rate their partner’s emotional state as more tense, more energetic, or more hedonic than did participants who saw their partners represented by humanoid avatars?
We used a linear mixed-effect model in R’s lme4 package, including the pair ID as a random effect to account for the non-independence of the two partner’s ratings. We tested whether the appearance of the partners’ avatar (both members of a pair always had the same avatar condition) predicted participants’ ratings of their conversational partners’ emotional states. We found no difference between participants’ ratings of their conversational partners’ hedonic (F = 0.129, p = 0.721), tense (F = 0.009, p = 0.926) or energetic (F = 0.207, p = 0.650) emotional states regardless of the avatar appearances of their conversational partners. In other words, avatar appearance did not impact participants’ perceptions of their partners’ emotional states.
Secondly, we explored whether participants who saw their partner in a humanoid avatar could perceive their partner’s emotional states more accurately than those who saw their partner represented by an animated cube. To do this, we used the emotional consensus scores.
The emotional consensus score, which was generated by averaging the correlation between participants’ ratings of their partners and their partner’s rating of themselves, indicated that participants were able to recognize each other’s emotional states at a rate significantly different than chance across both conditions (M = 0.298, SD = 0.251) using a one sample t-test comparing the result with 0 (t (71) = 10.077, p < 0.001).
Since the emotional consensus score is normally distributed (W = 0.984, p = 0.520) using a Shapiro-Wilk normality test, we used a Welch Two Sample t-test. And we found that there was no significant difference in the emotion consensus scores (t (66.093) = 0.155, p = 0.878) whether participants were represented by humanoid avatars or cubes. In other words, we did not see a difference between conditions in participants’ ability to recognize their partners’ emotional state.
We also examined the relationship between recognizing partners’ self-reported personality traits and avatar appearance. The results were highly similar to those for emotion recognition. However, as with emotional state ratings, these ratings did not differ by condition (all p’s larger than 0.100) and there was no difference in trait consensus by condition. For detailed results, please see Appendix B.
We summarize these findings as follows. If we assume that self-report is a reasonable “ground truth” for emotional states, then participants were able to identify each other’s emotional states at a rate higher than chance. This ability was not significantly affected by the appearance of the avatars in which participants were embodied.
3.2 Emotional Recognition Accuracy and User Experience/Presence
A linear mixed-effect model was used to test whether adaptation/immersion, involvement, visual quality, audio quality and distraction predict how well participants are able to tell their partners’ emotional states. The pair ID is included as a random effect to account for the non-independence of the two partner’s ratings.
We found no significant effect of adaptation/immersion (F = 1.130, p = 0.290) or distraction (F = 0.166, p = 0.684), involvement (F = 0.251, p = 0.617), visual quality (F = 0.095, p = 0.758), audio quality (F = 0.134, p = 0.715). This result indicates that the participants’ experience of a virtual environment does not impact how they interpret their conversational partners’ emotional states.
Additionally, we checked whether there was a significant effect of participants’ self-presence measures to predict how their conversational partners predict their emotional states. There was no significant effect of self-presence on their partners’ interpretation of their emotional states (F = 0.566, p = 0.453).
3.3 Social Closeness and Social Presence
We found a marginally significant effect of social closeness on how well the participants predicting their conversational partners’ emotional states (F = 3.438, p = 0.066). On the pair level, there was a significant positive correlation between social closeness and how well participants predict others emotional states (S = 39,882, p = 0.00197, rho = 0.359).
There was no significant effect of social presence on participants’ prediction of their conversational partners’ emotional states (F = 0.264, p = 0.609). On the pair level, there was not a significant correlation between social closeness and their prediction of their conversational partners’ emotional states (S = 52,246, p = 0.180, rho = 0.160).
3.4 Movement Measures
The lack of significant differences between avatar conditions in participants’ perception of each other’s emotional states implies that the mere appearance of the avatars did not have an effect on emotion recognition. There are several potential explanations for this. Participants may have focused on the voice, or words, of their partners to get information about their emotional state and other affective information, rather than taking cues from their partner’s non-verbal behavior as they would do during face to face interactions. This aligns with previous work in which participants reported using tone of voice as a primary cue for emotional state (Sun et al., 2019), and also with the finding that audio quality predicted emotional recognition accuracy. While we find this explanation plausible, it is also true that both the cube avatar and humanoid avatar conditions were designed to convey similar information about participants’ gestures and postures, since in both, participant’s head position and hand positions were rendered in the avatars. So, participants could have been using nonverbal behavior similarly in both conditions. This hypothesis is supported by the idea that visual quality also predicted emotion recognition. In fact, other research has found that even when participants report attending primarily to voice they are still influenced by nonverbal behavior (Garau et al., 2003). Thus, we also wanted to explore whether nonverbal cues that would be observable in these avatar conditions could be related to participants’ self-reported states of mind, or their partners’ estimations of their states of mind.
In order to do this, we selected two nonverbal behaviors to explore. One behavior was on the pair level: proximity, or the distance between the two participants in a pair. The second behavior was on the individual level: expansiveness of gesture. These were the only measures that we generated from movement data in this exploratory analysis.
3.4.1 Proximity
We selected the measure of proximity as a between-pairs measure of rapport, following Tickle-Degnen and Rosenthal (1987). To create the proximity measure, for each pair of participants, we calculated the Euclidean distance between two participants’ tracked distance between their head-mounted displays using the X and Z positions. We excluded the y position from our measure because they position shows participants’ height, which could introduce noise in the measure due to individual differences in height. We then took the average of the distance between their heads over the entire interaction for each pair.
3.4.2 Expansive Gesture
We selected the measure of expansiveness as an individual-level measure that has been used in the literature as an indicator of extraversion in humans (Campbell and Rushton, 1978; Gallaher, 1992; Argyle, 2013) as well as in agent-avatars to express extraversion (André et al., 2000; Pelachaud, 2009). We operationalized expansiveness of gesture by how far apart an individual participant held their hands. To create the expansive gesture measure, for each participant, we calculated the Euclidean distance between their left and right hands using the X, Y, and Z position. Then the distance of the hand movement over the entire interaction was averaged for each participant to create an open gesture measure for each individual. As described in Sun et al. (2019), we filtered out eleven pairs of participants due to either left hand or right hand data missing due to the technical tracking issue before calculating the measure of expansiveness.
If either of these movement measures could be linked to participants’ self-reported states of mind, or their estimations of their partners’ states of mind, then this would support the possibility that participants were still using nonverbal behavior as information. In order to limit our analyses, these were the only two nonverbal behaviors we explored.
3.4.3 Emotional States and Proximity
We first explored proximity, measured by the distance between participants’ heads. Using a t-test, we found no significant difference (t (65.324) = 0.491, p = 0.625) in proximity between pairs in the humanoid avatar condition (M = 2.516, SD = 0.643) and the cube condition (M = 2.433, SD = 0.794).
First, we tested whether the distance between pair members correlated to pairs’ average ratings of their own emotional states. Using a Shapiro-Wilk normality test, we found that the proximity measure was normally distributed (W = 0.970, p = 0.065). Because proximity is a measure taken at the pair level of analysis, we combined participants’ emotional state self-reports to get a joint measure of emotional state. We used a Pearson’s r correlation test and found that there was a negative significant relationship between participants’ joint ratings of how tense they felt and the proximity between their two heads (r (73) = −0.344, p = 0.003). In other words, the closer participants were standing to each other, the more tense emotions they reported experiencing. We also found a positive significant correlation between participants’ energetic emotion and proximity (r (74) = 0.262, p = 0.022). The more participants felt energetic about their own emotional states, the larger their interpersonal distance. However, there was not a significant correlation between hedonic emotion and proximity (r (74) = − 0.148, p = 0.203). Figure 3 shows the correlation between proximity and group self-ratings on emotional states.
3.4.4 Proximity and Social Closeness
We next used a Pearson’s r correlation test to check whether proximity is related to social closeness. We found that there was a marginally significant positive correlation between proximity and social closeness (r (74) = 0.202, p = 0.080). Surprisingly, the higher distance between the two participants, the more social closeness on average that they reported.
In order to further explore this result, we used a linear model examining the interaction between avatar appearance and proximity. In this model, there was no significant interaction, nor any significant main effect of these two variables on social closeness (all p’s larger than 0.200). In other words, proximity predicted social closeness ratings no matter what kind of avatar participants used.
3.4.5 Extraversion, Emotional States and Expansive Gesture
Second, we investigated the relationship between individuals’ gestures; their self-reported emotional states, and their partners’ ratings. To do so we used the measure of expansiveness of gesture reflected by how far apart participants held their hands. In the case of the humanoid avatar, this would have been reflected by the distance between the avatar’s hands; in the case of the cube avatar, this would have been reflected by the width of the cube which would have grown wider as participants moved their hands apart. Because some participants’ left or right hand data were missing, we dropped 11 participant pairs from our analysis, leaving 65 pairs of participants.
We used the lme4 package in R to test linear mixed effect models. In order to control for differences in participant size, which could also have influenced the distance between hands, we used participant height (operationalized by the mean of the Y-axis position of the head) as a fixed effect in all models. We used pair ID as a random effect to account for the non-independence of the two partner’s self-ratings.
We next examined whether participants’ self-ratings of extraversion were related to the expansiveness of their gestures. We again included height as a fixed effect and pair ID as a random effect. We found a non-significant difference between expansiveness of gesture and participant’s self-reported extraversion ratings, such that participants with higher ratings of extraversion had a slightly greater distance between their hands on average (F = 1.663, p = 0.200). As the literature would predict, we did not find a significant difference in the rest of the participants’ self-rating of personality traits when they had different open gestures (all p’s larger than 0.150).
Finally, we examined whether there was an interaction between avatar appearance and expansiveness of gesture predicting self-ratings of extraversion using a linear mixed-effects model with the lmer function from the lme4 package in R. Appearance and extraversion were used as fixed effects, and pair ID as a random effect in the model. We found a significant interaction between appearance and expansiveness of gesture, such that participants in the cube condition who self-rated themselves as being more extraverted also had more expansive gestures (F = 6.013, p = 0.016) (see Figure 4). We also found a main effect of appearance, such that participants in the cube condition had more expansive gestures overall. This aligns with our observations of participants in the cube condition; when participants first saw their new appearance in the mirror scene, many were intrigued by their ability to make the cube grow and shrink by moving their arms and spent some time playing with this ability, which may have made this gesture more salient.
4 Discussion
In this paper, we explored whether differences in avatar appearance led to differences in participants’ perception of their conversational partners’ personality or emotional states. Participants’ ratings of their partners’ emotional states agreed with the partners’ self-ratings at a rate significantly higher than chance across both conditions. However, we did not find any significant differences in emotion perception between avatar appearance conditions. We propose two possible explanations for this. First, the gestural and postural information participants received in both conditions might have been equivalently informative. Alternatively, because some important parts of interpersonal communication were missing from virtual reality, participants may not have relied on their partner’s movements at all. For example, there was no eye contact, lip sync or facial expressions rendered for participants’ avatars in either condition, which are all important information streams that could aid in emotion recognition. In this case, in both conditions, participants might rely primarily on the voice and words of their conversational partner.
To further investigate this, we looked at nonverbal cues that could be communicated through these avatars: proximity and expansiveness of gesture, and whether these clues could be linked to participants’ self report of their own or their partner’s states of mind. Participants’ joint self ratings on energetic emotion were positively correlated with their proximity with each other; participants who reported higher levels of energetic emotion stood further apart. Tense emotion was negatively correlated with proximity; participants who reported higher joint levels of tension stood closer together. Surprisingly, social closeness also correlated positively with proximity: participant pairs who expressed higher levels of social closeness stood far apart.
In order to better understand whether this last finding might be a false positive, we explored whether there was an interaction effect of the avatar appearance and open gesture on proximity. Because open gestures caused the cubes to grow in all three dimensions, this may have made their avatar appear closer, participants who were making open gestures may have increased the distance between themselves and their partners to maintain an appropriate interpersonal space. However, we did not find a significant interaction effect: the interaction between avatar appearance no S and the open gestures did not impact the proximity in a statistically significant way. Thus, we are left without a good explanation for the unexpected positive relationship between the distance between participants and social closeness ratings. However, this result does point to the idea that changes in avatar embodiment may have unexpected effects both on emergent nonverbal behavior, and also how people perceive and interpret newly emergent nonverbal behaviors.
Expansiveness of gesture was predictive of self-ratings of extraversion, but only in the cube condition. Participants did not appear to change their ratings of their partner’s extraversion according to expansiveness in either condition.
We interpret these exploratory findings as supporting the possibility that even though participants may have primarily used audio channels to determine their partners’ emotional states, emergent nonverbal behavior, which may or may not have been informative to their partners, was reflected in their avatar gestures, and this visual information may have also aided emotion recognition. Interestingly, some behavior, like expansiveness of gesture, may have been more salient in the abstract conditions. Further work is necessary to confirm these exploratory findings, and also to determine whether such visually apparent nonverbal behavior is eventually used by conversational partners to aid in the interpretation of states of mind. Even if this is possible, it may take time for participants to learn to extrapolate from their own avatar gestures to interpret those of others.
Notably, there were few relationships between conventional measures of presence and emotion recognition. For this reason, we argue that measures of emotion recognition may be an important and overlooked indicator of usability in social virtual environments, especially in those where the avatar may not closely resemble a human form.
4.1 Limitations
There were several limitations that could be improved in future studies. First, while we have limited our exploratory analyses, and we report all of the analyses we did run, further confirmatory experiments are necessary to build on these findings.
When considering the variable of proximity or interpersonal distance, there are some potential confounds. For example, in the cube condition, if people expand their arms, the cubes will enlarge in all directions. Although the expansion of the cube was meant to resemble the way humanoid avatars would take up more space when their arms were extended, this was not a perfect match because humans would generally extend their arms more in the X axis. When the cube avatars expanded, they expanded in X axis but also in the Y and the Z axes. The Z axis in particular could also give the appearance of increased proximity. Thus, future work that specifically examines proximity should use more precisely designed comparison conditions.
In theory, emotion could be perceived even with the minimal three points available through tracked avatar movements. However, our exploratory work probably does not show the full picture of people’s emotional states and personality traits. As tracking improves in consumer systems, future work can examine movement data more granularly.
4.2 Next Steps
In this study we used participants’ self-reported emotional states as the “ground truth” of emotional scores. Future work could cross-validate participants’ self-reported emotional state and psychological trait scores; for example, by running prosodic analysis on participants’ voice recordings as the “ground truth”.
Future work could also seek other ways to create virtual humanoid avatars to include more nonverbal features. Some current virtual environments render gaze or mouth movements in social interactions. In some social VR platforms such as Facebook spaces (Facebook, 2019), users can create different emotions by using their hand controllers. All of these nonverbal features could be represented abstractly, to further examine whether this transformed nonverbal behavior is being used to inform emotional state and psychological trait perception.
Finally, our movement measures were intentionally kept simple, and there are many other interesting ways to explore movement trends over time. For example, we could use time series (McCleary et al., 1980, Wei, 2006) to understand how people’s proximity and gestures change over time and further explore whether these movement are predictive of people’s emotional states and personality traits.
5 Conclusion
This study examined our final pre-registered research question on the relationship between emotion recognition, individual and dyadic measures of the proximity and openness of gesture, in the context of humanoid and abstract (cube) avatar appearance. We found no difference in emotional state and personality trait recognition between two different avatar appearances. However, recognition was significantly higher than chance in both instances—people were able to perceive each other’s emotional states and personality traits, even when inhabiting an abstract cube-shaped avatar. To help elucidate this result, we explored how emotion correlated to proximity and expansiveness of gesture, and found significant correlations between proximity and emotional states as well as certain personality traits.
Whether emotional and psychological perception was aided by the nonverbal behavior available in both avatar conditions, or was purely dependent on other cues, remains unknown. Further investigation is needed to understand what information streams people perceived from their partners’ avatar representations, and how those perceptions influenced emotion recognition in virtual reality.
Data Availability Statement
We have packaged our data for sharing on a permanent server through Cornell Center for Social Sciences. Because movement data can provide sensitive information about individuals (Bailenson, 2018), we will require an IRB before releasing movement data. Our movement data has been packaged with our processing code so that researchers can exactly replicate our findings or run their own analyses.
Ethics Statement
The studies involving human participants were reviewed and approved by Cornell University Institutional Review Board. The participants provided their written informed consent to participate in this study.
Author Contributions
YS and ASW performed conceptualization, data curation, investigation, methodology, visualization, writing the original draft preparation and reviewing and editing. YS also performed data analysis. ASW also performed supervision.
Conflict of Interest
The authors declare that the research was conducted in the absence of any commercial or financial relationships that could be construed as a potential conflict of interest.
Publisher’s Note
All claims expressed in this article are solely those of the authors and do not necessarily represent those of their affiliated organizations, or those of the publisher, the editors and the reviewers. Any product that may be evaluated in this article, or claim that may be made by its manufacturer, is not guaranteed or endorsed by the publisher.
Acknowledgments
The authors would like to thank all the research assistants who helped to conduct the experiment and code the qualitative data in the study: Sarah Chekfa, Lilian Garrido, Seonghee Lee, T. Milos Kartalijia, Byungdoo Kim, Hye Jin Kwon, Kristi Lin, Swati Pandita, Juliana Silva, Rachel Song, Yutong Wu, Keun Hee Youk, Yutong Zhou, Joshua Zhu, and Colton Zuvich. We thank Meredith Keller for her help in the pilot study, and Katy Miller for her assistance in proofreading the manuscript. We appreciate Omar Shaikh’s work in implementing the software and programming for the original project. We also thank Stephen Parry from the Cornell Statistical Consulting Unit for providing statistical consulting, and Florio Arguillas, Jacob Grippin and Daniel Alexander from Cornell Center for Social Sciences for helping validate the code and archiving the data. We also thank Cornell University and the Ithaca community for participating in this experiment.
References
André, E., Klesen, M., Gebhard, P., Allen, S., Rist, T., et al. (2000). “Exploiting Models of Personality and Emotions to Control the Behavior of Animated Interactive Agents,” in Fourth International Conference on Autonomous Agents, Barcelona, Spain, June 3-7, 2000.
Astrid, M., Krämer, N. C., and Gratch, J. (2010). “How Our Personality Shapes Our Interactions with Virtual Characters-Implications for Research and Development,” in International Conference on Intelligent Virtual Agents, Philadelphia, PA, September 20-22, 2010 (Springer), 208–221.
Atkinson, A. P., Dittrich, W. H., Gemmell, A. J., and Young, A. W. (2004). Emotion Perception from Dynamic and Static Body Expressions in point-light and Full-Light Displays. Perception 33, 717–746. doi:10.1068/p5096 &
Bailenson, J. N., and Yee, N. (2005). Digital Chameleons: Automatic Assimilation of Nonverbal Gestures in Immersive Virtual Environments. Psychol. Sci. 16, 814–819. doi:10.1111/j.1467-9280.2005.01619.x &
Bailenson, J. N., Yee, N., Merget, D., and Schroeder, R. (2006). The Effect of Behavioral Realism and Form Realism of Real-Time Avatar Faces on Verbal Disclosure, Nonverbal Disclosure, Emotion Recognition, and Copresence in Dyadic Interaction. Presence Teleop.Virtual Environ. 15, 359–372. doi:10.1162/pres.15.4.359
Bailenson, J. (2018). Protecting Nonverbal Data Tracked in Virtual Reality. JAMA Pediatr. 172, 905–906. doi:10.1001/jamapediatrics.2018.1909 &
Banse, R., and Scherer, K. R. (1996). Acoustic Profiles in Vocal Emotion Expression. J. Personal. Soc. Psychol. 70, 614–636. doi:10.1037/0022-3514.70.3.614
Borkenau, P., Mauer, N., Riemann, R., Spinath, F. M., and Angleitner, A. (2004). Thin Slices of Behavior as Cues of Personality and Intelligence. J. Personal. Soc. Psychol. 86, 599–614. doi:10.1037/0022-3514.86.4.599
Campbell, A., and Rushton, J. P. (1978). Bodily Communication and Personality. Br. J. Soc. Clin. Psychol. 17, 31–36. doi:10.1111/j.2044-8260.1978.tb00893.x
Clarke, T. J., Bradshaw, M. F., Field, D. T., Hampson, S. E., and Rose, D. (2005). The Perception of Emotion from Body Movement in point-light Displays of Interpersonal Dialogue. Perception 34, 1171–1180. doi:10.1068/p5203 &
Cowie, R., Douglas-Cowie, E., Tsapatsoulis, N., Votsis, G., Kollias, S., Fellenz, W., et al. (2001). Emotion Recognition in Human-Computer Interaction. IEEE Signal. Process. Mag. 18, 32–80. doi:10.1109/79.911197
Dael, N., Goudbeek, M., and Scherer, K. R. (2013). Perceived Gesture Dynamics in Nonverbal Expression of Emotion. Perception 42, 642–657. doi:10.1068/p7364 &
Dael, N., Mortillaro, M., and Scherer, K. R. (2012). Emotion Expression in Body Action and Posture. Emotion 12, 1085. doi:10.1037/a0025737 &
De Gelder, B., and Vroomen, J. (2000). The Perception of Emotions by Ear and by Eye. Cogn. Emot. 14, 289–311. doi:10.1080/026999300378824
De Meijer, M. (1989). The Contribution of General Features of Body Movement to the Attribution of Emotions. J. Nonverbal Behav. 13, 247–268. doi:10.1007/bf00990296
Dodds, T., Mohler, B., and Bülthoff, H. (2010). “A Communication Task in Hmd Virtual Environments: Speaker and Listener Movement Improves Communication,” in 23rd Annual Conference on Computer Animation and Social Agents (CASA), Saint-Malo, France, May 31-June 2, 2010, 1–4.
Doyle, C. M., and Lindquist, K. A. (2017). “Language and Emotion: Hypotheses on the Constructed Nature of Emotion Perception,” in The Science of Facial Expression (Oxford University Press).
Ehrsson, H. H., Holmes, N. P., and Passingham, R. E. (2005). Touching a Rubber Hand: Feeling of Body Ownership Is Associated with Activity in Multisensory Brain Areas. J. Neurosci. 25, 10564–10573. doi:10.1523/jneurosci.0800-05.2005
Enea, V., and Iancu, S. (2016). Processing Emotional Body Expressions: State-Of-The-Art. Soc. Neurosci. 11, 495–506. doi:10.1080/17470919.2015.1114020 &
Facebook (2019). Facebook Spaces. Website. [Dataset]. Available at: https://www.facebook.com/spaces (Accessed September 19, 2019).
Fong, K., and Mar, R. A. (2015). What Does My Avatar Say about Me? Inferring Personality from Avatars. Personal. Soc. Psychol. Bull. 41, 237–249. doi:10.1177/0146167214562761
Gallaher, P. E. (1992). Individual Differences in Nonverbal Behavior: Dimensions of Style. J. Personal. Soc. Psychol. 63, 133. doi:10.1037/0022-3514.63.1.133
Garau, M., Slater, M., Vinayagamoorthy, V., Brogni, A., Steed, A., and Sasse, M. A. (2003). “The Impact of Avatar Realism and Eye Gaze Control on Perceived Quality of Communication in a Shared Immersive Virtual Environment,” in Proceedings of the SIGCHI conference on Human factors in computing systems (ACM), Ft. Lauderdale, FL, April 5-10, 2003, 529–536. doi:10.1145/642611.642703
Garau, M. (2003). “The Impact of Avatar Fidelity on Social Interaction in Virtual Environments,”. Technical Report (University College London).
Gosling, S. D., Rentfrow, P. J., and Swann, W. B. (2003). A Very Brief Measure of the Big-Five Personality Domains. J. Res. Personal. 37, 504–528. doi:10.1016/s0092-6566(03)00046-1
Gunes, H., and Piccardi, M. (2007). Bi-modal Emotion Recognition from Expressive Face and Body Gestures. J. Netw. Comp. Appl. 30, 1334–1345. doi:10.1016/j.jnca.2006.09.007
Hertenstein, M. J., Holmes, R., McCullough, M., and Keltner, D. (2009). The Communication of Emotion via Touch. Emotion 9, 566. doi:10.1037/a0016108 &
Johansson, G. (1973). Visual Perception of Biological Motion and a Model for its Analysis. Percept. psychophys. 14, 201–211. doi:10.3758/bf03212378
Kobylinski, P., Pochwatko, G., and Biele, C. (2019). “Vr Experience from Data Science point of View: How to Measure Inter-subject Dependence in Visual Attention and Spatial Behavior,” in International Conference on Intelligent Human Systems Integration, San Diego, February 7-10, 2019 (Springer), 393–399. doi:10.1007/978-3-030-11051-2_60
Koppensteiner, M. (2013). Motion Cues that Make an Impression: Predicting Perceived Personality by Minimal Motion Information. J. Exp. Soc. Psychol. 49, 1137–1143. doi:10.1016/j.jesp.2013.08.002
Kozlowski, L. T., and Cutting, J. E. (1977). Recognizing the Sex of a walker from a Dynamic point-light Display. Percept. psychophys. 21, 575–580. doi:10.3758/bf03198740
Latoschik, M. E., Roth, D., Gall, D., Achenbach, J., Waltemate, T., and Botsch, M. (2017). “The Effect of Avatar Realism in Immersive Social Virtual Realities,” in Proceedings of the 23rd ACM Symposium on Virtual Reality Software and Technology (ACM), 39. doi:10.1145/3139131.3139156
Li, C., Androulakaki, T., Gao, A. Y., Yang, F., Saikia, H., Peters, C., et al. (2018). “Effects of Posture and Embodiment on Social Distance in Human-Agent Interaction in Mixed Reality,” in Proceedings of the 18th International Conference on Intelligent Virtual Agents (ACM), 191–196. doi:10.1145/3267851.3267870
Lorey, B., Kaletsch, M., Pilgramm, S., Bischoff, M., Kindermann, S., Sauerbier, I., et al. (2012). Confidence in Emotion Perception in point-light Displays Varies with the Ability to Perceive Own Emotions. PLoS One 7, e42169. doi:10.1371/journal.pone.0042169 &
Lugrin, J.-L., Latt, J., and Latoschik, M. E. (2015). “Avatar Anthropomorphism and Illusion of Body Ownership in Vr,” in 2015 IEEE Virtual Reality (VR) (IEEE), France, March 23-27, 2015, 229–230. doi:10.1109/vr.2015.7223379
Matthews, G., Jones, D. M., and Chamberlain, A. G. (1990). Refining the Measurement of Mood: The Uwist Mood Adjective Checklist. Br. J. Psychol. 81, 17–42. doi:10.1111/j.2044-8295.1990.tb02343.x
McCleary, R., Hay, R. A., Meidinger, E. E., and McDowall, D. (1980). Applied Time Series Analysis for the Social Sciences. Beverly Hills, CA: Sage Publications.
McVeigh-Schultz, J., Márquez Segura, E., Merrill, N., and Isbister, K. (2018). “What’s it Mean to Be Social in Vr?: Mapping the Social Vr Design Ecology,” in Proceedings of the 2018 ACM Conference Companion Publication on Designing Interactive Systems (ACM), Hong Kong, China, June 9-13, 2018, 289–294.
Missana, M., Atkinson, A. P., and Grossmann, T. (2015). Tuning the Developing Brain to Emotional Body Expressions. Develop. Sci. 18, 243–253. doi:10.1111/desc.12209
Montagne, B., Kessels, R. P., De Haan, E. H., and Perrett, D. I. (2007). The Emotion Recognition Task: A Paradigm to Measure the Perception of Facial Emotional Expressions at Different Intensities. Perceptual Mot. skills 104, 589–598. doi:10.2466/pms.104.2.589-598
Mousas, C., Anastasiou, D., and Spantidi, O. (2018). The Effects of Appearance and Motion of Virtual Characters on Emotional Reactivity. Comput. Hum. Behav. 86, 99–108. doi:10.1016/j.chb.2018.04.036
Nowak, K. L., and Fox, J. (2018). Avatars and Computer-Mediated Communication: a Review of the Definitions, Uses, and Effects of Digital Representations. Rev. Commun. Res. 6, 30–53. doi:10.12840/issn.2255-4165.2018.06.01.015
Nowak, K. L., and Rauh, C. (2005). The Influence of the Avatar on Online Perceptions of Anthropomorphism, Androgyny, Credibility, Homophily, and Attraction. J. Comp.Mediated Commun. 11, 153–178. doi:10.1111/j.1083-6101.2006.tb00308.x
Pelachaud, C. (2009). Studies on Gesture Expressivity for a Virtual Agent. Speech Commun. 51, 630–639. doi:10.1016/j.specom.2008.04.009
Pertaub, D.-P., Slater, M., and Barker, C. (2002). An experiment on Public Speaking Anxiety in Response to Three Different Types of Virtual Audience. Presence Teleop. Virtual Environ. 11, 68–78. doi:10.1162/105474602317343668
Roth, D., Lugrin, J.-L., Galakhov, D., Hofmann, A., Bente, G., Latoschik, M. E., et al. (2016). “Avatar Realism and Social Interaction Quality in Virtual Reality,” in 2016 IEEE Virtual Reality (VR) (IEEE), Greenville, SC, March 19-23, 2016, 277–278. doi:10.1109/vr.2016.7504761
Schirmer, A., and Adolphs, R. (2017). Emotion Perception from Face, Voice, and Touch: Comparisons and Convergence. Trends Cogn. Sci. 21, 216–228. doi:10.1016/j.tics.2017.01.001 &
Shaikh, O., Sun, Y., and Won, A. S. (2018). “Movement Visualizer for Networked Virtual Reality Platforms,” in 2018 IEEE Conference on Virtual Reality and 3D User Interfaces (VR) (IEEE), Tuebingen/Reutlingen, Germany, March 18-22, 2018, 681–682. doi:10.1109/vr.2018.8446398
Shin, M., Kim, S. J., and Biocca, F. (2019). The Uncanny valley: No Need for Any Further Judgments when an Avatar Looks Eerie. Comput. Hum. Behav. 94, 100–109. doi:10.1016/j.chb.2019.01.016
Sun, Y., Shaikh, O., and Won, A. S. (2019). Nonverbal Synchrony in Virtual Reality. PloS one 14, e0221803. doi:10.1371/journal.pone.0221803 &
Tarr, B., Slater, M., and Cohen, E. (2018). Synchrony and Social Connection in Immersive Virtual Reality. Scientific Rep. 8, 1–8. doi:10.1038/s41598-018-21765-4
Thomas, J., Azmandian, M., Grunwald, S., Le, D., Krum, D. M., Kang, S.-H., et al. (2017). “Effects of Personalized Avatar Texture Fidelity on Identity Recognition in Virtual Reality,” in ICAT-EGVE, Adelaide, Australia, November 22-24, 2017, 97–100.
Tickle-Degnen, L., and Rosenthal, R. (1987). “Group Rapport and Nonverbal Behavior,” in Group Processes and Intergroup Relations (Sage Publications, Inc.).
Tolani, D., Goswami, A., and Badler, N. I. (2000). Real-time Inverse Kinematics Techniques for Anthropomorphic Limbs. Graph. Models 62, 353–388. doi:10.1006/gmod.2000.0528 &
Waltemate, T., Gall, D., Roth, D., Botsch, M., and Latoschik, M. E. (2018). The Impact of Avatar Personalization and Immersion on Virtual Body Ownership, Presence, and Emotional Response. IEEE Trans. Vis. Comput. Graph. 24, 1643–1652. doi:10.1109/tvcg.2018.2794629 &
Wei, W. W. (2006). “Time Series Analysis,” in The Oxford Handbook of Quantitative Methods in Psychology, 2.
Witmer, B. G., Jerome, C. J., and Singer, M. J. (2005). The Factor Structure of the Presence Questionnaire. Presence Teleop. Virtual Environ. 14, 298–312. doi:10.1162/105474605323384654
Witmer, B. G., and Singer, M. J. (1998). Measuring Presence in Virtual Environments: A Presence Questionnaire. Presence 7, 225–240. doi:10.1162/105474698565686
Won, A. S., Bailenson, J., Lee, J., and Lanier, J. (2015). Homuncular Flexibility in Virtual Reality. J. Comput. Mediated Commun. 20, 241–259. doi:10.1111/jcc4.12107
Won, A. S., Shriram, K., and Tamir, D. I. (2018). Social Distance Increases Perceived Physical Distance. Soc. Psychol. Personal. Sci. 9, 372–380. doi:10.1177/1948550617707017
Yee, N., and Bailenson, J. (2007). The proteus Effect: The Effect of Transformed Self-Representation on Behavior. Hum. Commun. Res. 33, 271–290. doi:10.1111/j.1468-2958.2007.00299.x
Yee, N., Ducheneaut, N., and Ellis, J. (2008). The Tyranny of Embodiment. Artifact: J. Des. Pract. 2, 88–93. doi:10.1080/17493460903020398
Yee, N., Harris, H., Jabon, M., and Bailenson, J. N. (2011). The Expression of Personality in Virtual Worlds. Soc. Psychol. Personal. Sci. 2, 5–12. doi:10.1177/1948550610379056
Zibrek, K., Kokkinara, E., and McDonnell, R. (2018). The Effect of Realistic Appearance of Virtual Characters in Immersive Environments-Does the Character’s Personality Play a Role? IEEE Trans. Vis. Comput. Graph. 24, 1681–1690. doi:10.1109/tvcg.2018.2794638 &
Appendix a: emotional difference scores
We replicated the results in section 3.1 with the emotional difference score, which was generated by taking the difference of the participants’ ratings of their partners and their partners’ rating of themselves, indicating that participants were able to recognize each other’s emotional states at a rate significantly different than chance across both conditions (M = 0.827, SD = 0.230) using a one sample t-test comparing the result with 0 (t (71) = 30.441, p < 0.001).
Since the emotional consensus score was not normally distributed (W = 0.960, p = 0.022) using a Shapiro-Wilk normality test, we used a Wilcoxon rank sum test, finding that there were no significant differences in the emotion consensus scores (W = 716, p = 0.415) whether participants were represented by humanoid avatars or cubes. In other words, we did not see a difference between conditions in participants’ ability to recognize their partners’ emotional states.
Appendix b: trait recognition and avatar appearance
Similar to emotion recognition, we explored whether there was a difference in how personality traits were perceived by participants depending on the appearance of the avatar used during in the interaction. In other words, did participants who saw their partners represented by cubes rate their partner’s personality as being more open, conscientious, extroverted, agreeable or neurotic compared to participants who saw their partners represented by humanoid avatars?
Using a linear mixed-effect model, we tested whether the avatar’s appearance is a predictor of every participant’s ratings to their conversational partners’ personality traits. We found no significant difference in participants’ ratings of their conversational partners’ characteristics of openness to new experiences (F = 0.087, p = 0.769), conscientiousness (F = 2.121, p = 0.147), extroversion (F = 0.319, p = 0.574), agreeableness (F = 2.300, p = 0.132), emotional stability (F = 1.177, p = 0.282), regardless of the avatar appearance of their conversational partners.
Secondly, we explored whether participants who saw their partners in a humanoid avatar could perceive their partners’ personality traits more accurately compared to those who saw their partners represented by an animated cube. We tested whether there was a significant difference in the trait consensus scores and trait perception scores when participants were represented by different avatar appearances.
The traits consensus score, which was generated by averaging the correlation between participants’ trait ratings of their partners and their partner’s rating of themselves, indicated that participants were able to recognize each other’s traits at a rate significantly different than chance across both conditions (M = 0.394, SD = 0.265) using a one sample t-test comparing the result with 0 (t (68) = 12.339, p < 0.001).
Since the traits consensus score is not normally distributed (W = 0.939, p = 0.002) using a Shapiro-Wilk normality test, we used a Wilcoxon rank sum test, finding that there were no significant differences in the traits consensus scores (W = 675, p = 0.253) whether participants were represented by humanoid avatars or cubes. In other words, we did not see a difference between conditions in participants’ ability to recognize their partners’ traits.
Keywords: virtual reality, emotion perception, nonverbal communication, personality traits, emotional states, expansiveness of gesture, avatars, proximity
Citation: Sun Y and Won AS (2021) Despite Appearances: Comparing Emotion Recognition in Abstract and Humanoid Avatars Using Nonverbal Behavior in Social Virtual Reality. Front. Virtual Real. 2:694453. doi: 10.3389/frvir.2021.694453
Received: 13 April 2021; Accepted: 10 August 2021;
Published: 27 September 2021.
Edited by:
Missie Smith, Independent researcher, Detroit, MI, United StatesReviewed by:
Richard Skarbez, La Trobe University, AustraliaBenjamin J. Li, Nanyang Technological University, Singapore
Copyright © 2021 Sun and Won. This is an open-access article distributed under the terms of the Creative Commons Attribution License (CC BY). The use, distribution or reproduction in other forums is permitted, provided the original author(s) and the copyright owner(s) are credited and that the original publication in this journal is cited, in accordance with accepted academic practice. No use, distribution or reproduction is permitted which does not comply with these terms.
*Correspondence: Andrea Stevenson Won, a.s.won@cornell.edu