- Department of Communication Sciences and Disorders, University of Kentucky, Lexington, KY, United States
Introduction: Deficits in the cognitive domains of attention and memory leave a large impact on everyday activities that are not easily captured in the clinical environment. Therefore, clinicians are compelled to utilize assessment tools that elicit everyday functioning that include real-world contexts and distractions. As a result, the use of computer-assisted assessment has emerged as a tool for capturing everyday functioning in a variety of environments. The purpose of this scoping review is to map how virtual reality, augmented reality, and computer-based programs have implemented distractions for clinical populations.
Methods: A scoping review of peer reviewed publications was conducted by searching Pubmed, PsychInfo, Web of Science, Rehabdata, and Scopus databases (1960-October 20, 2020). Authors completed hand-searches for additional published and unpublished studies.
Results: Of 616 titles screened, 23 articles met inclusion criteria to include in this review. Primary distraction display modalities included computer monitor displays (n = 12) and head mounted displays (HMD) (n = 7). While computer-assisted assessments included distractions, no systematic approach was utilized to implement them. Primary distractions included both auditory and visual stimuli that were relevant to the task and/or simulated environment. Additional distraction characteristics emerged including location, timing, and intensity that can contribute to overall noticeability.
Conclusion: From this review, the authors examined the literature on the implementation of distractions in simulated programming. The authors make recommendations regarding identification, measurement, and programming with suggestions that future studies examining metrics of attention to implement distraction in measurable and meaningful ways. Further, the authors propose that distraction does not universally impact performance negatively but can also enhance performance for clinical populations (e.g. additional sensory stimuli to support focused attention).
Introduction
Persons with attention and memory deficits (PAMDs) are susceptible to long-term problems in everyday functioning due to distractibility (Simmeborn Fleischer, 2012; Gelbar et al., 2014; Adreon and Durocher, 2016). PAMDs include clinical populations with diagnoses of attention deficit hyperactivity disorder (ADHD), autism, stroke, traumatic brain injury (TBI), Parkinson’s disease (PD), and cognitive decline with aging. A common challenge with assessing PAMDs is that they can score within normal limits on standardized assessments but report significant difficulty in everyday environments (Beaman, 2005; Knight et al., 2006). A promising new method of assessing these clinical populations is through computer-assisted assessments that simulate everyday environments with distractions.
Functional activities, such as attending to classroom activities, scheduling appointments, completing work tasks, and communication, require fluctuating attentional resources and management of distractions. However, PAMDs often experience difficulty maintaining focus during tasks (Newcorn et al., 2001; van Mourik et al., 2007) while inhibiting competing sensory stimuli (Koplowitz et al., 1997). Inhibition issues are also associated with breakdowns in working memory (Brewer, 1998; Trudel et al., 1998; Lutz, 1999; Hartnedy and Mozzoni, 2000; Brewer et al., 2002; Levin et al., 2004; Knight et al., 2006; Krawczyk et al., 2008; Levin et al., 2008; Couillet et al., 2010). Deficits in distraction management may impact several PAMDs life domains, including home, academic, social, and work. Because PAMDs attention and memory abilities can decrease in distracting environments, their performance in a clinical testing environment can differ significantly from everyday life.
Standardized neuropsychological assessment has evolved from detecting and identifying neuropathology (impairment-based) to making inferences regarding abilities to perform everyday tasks. Shifting from impairment detection to making predictions of everyday functioning has increased the need for ecologically validity assessments (Spooner and Pachana, 2006). Ecological validity has two distinct components, 1) surface validity, and 2) criterion validity that refer to the degree of relevance or similarity that a test or training has to the “real” world (Neisser, 1978). Surface validity includes linking of task demands and the demands in everyday environments (Spooner and Pachana, 2006). Criterion validity indicates how the results on an assessment instrument are associated to scores on other measures that make inferences about real-world performance (Franzen and Wilhelm, 1996). Impairment-based assessments are administered under optimal conditions for concentration; and therefore, are not sensitive to the distractions present in daily cognitive function (Sbordone, 1996).
The ecological validity in impairment-based assessments of attention and memory has been a point of concern for many years (Sbordone, 1996; Van Der Linden, 2008). Primarily, with their ability to capture “everyday” functioning (Sbordone, 1996; Van Der Linden, 2008; Marcotte and Grant, 2010). The issue is that impairment-based assessments are used to diagnose attention and memory deficits and to make predictions of everyday function (Spooner and Pachana, 2006). Research analyzing the relationship between standardized neuropsychological assessments and everyday functioning shows a “modest” or “moderate” (predicting job performance R2 = 0.64, predicting occupational status R2 = 0.57), correlation depending on the assessment type (Kibby et al., 1998), real-life performance criteria (Marcotte et al., 2010), injury (Chaytor and Schmitter-Edgecombe, 2003; Wood and Liossi, 2006; Silverberg et al., 2007), or cognitive and/or executive function target (Chaytor and Schmitter-Edgecombe, 2003). The development of functional assessments such as the Test of Everyday Attention (TEA; Robertson et al., 1994), the Behavioral Assessment of the Dysexecutive Syndrome (BADS; Wilson et al., 1996), the Rivermead Behavioral Memory Test (RBMT; Wilson et al., 1985), the Cambridge Test of Prospective Memory (CAMPROMPT; Wilson et al., 2004), Continuous Performance Test (Conners, 1995), STROOP Task (Stroop, 1935), and Wechsler Adult Intelligence Scale (WAIS; Wechsler, 2008) may better predict everyday function than impairment-based assessments. Such functional assessments have higher levels of ecological validity (surface and criterion validity), enabling inferences of everyday functioning to be made (Spooner and Pachana, 2006). Still, there continues to be a missing component of the inclusion of everyday distraction in the development of functional and impairment-based assessments. Accurate inferences about everyday performance should be made based on assessments that simulate everyday environments that include distractions. Furthermore, clinical assessments and interventions should identify specific distraction characteristics that impact attention and memory to predict environments in which strategies or accommodations are needed. Perhaps the absence of research focusing on the impact of specific elements of distraction is due to a lack of established distraction characteristics, dosing, and measurement parameters.
The term distraction has an inherent negative connotation implying it that it solely creates negative outcomes on attention and memory performance. However, previous ADHD literature suggests that distractions can enhance task performance by increasing arousal to meet task demands (Zentall and Meyer, 1987; Corkum et al., 1993; Abikoff et al., 1996; Sergeant et al., 1999; Leung et al., 2000; Sergeant, 2005). One explanation for this phenomenon is that when there is spare attentional capacity with no other information to attend to, attention may turn inwards resulting in internal distraction (i.e., mind wandering) (Kahneman, 1973; Forster and Lavie, 2007). Thus, findings about the impact of distraction on task performance remain unknown.
Distractions can be classified by modality, such as internal, auditory, visual, audiovisual, and tactile. Distractions can also be classified by relevance to the task at hand or by the relevance of stimuli to the task stimuli (Boll and Berti, 2009). Recent evidence supports the duplex-mechanism account of auditory distraction where sound can cause distraction by interfering with task processes (interference-by-process) or by steering attention away from the task despite the type of processing that task requires (attentional capture) (Huges, 2014). The challenge with managing distractions is that attention is required to focus on goal-relevant stimuli while also being simultaneously open to irrelevant-stimuli given the possibility that stimuli will become relevant. However, goal-irrelevant stimuli can interfere with the effectiveness of goal-driven behavior (Baddeley et al., 1974; Salame et al., 1982; Beaman et al., 1997; Jones et al., 1992; Huges and Jones, 2003a). Attention, motivation, and emotional state contribute to the filtering of environmental stimuli that assists in the judgment and selection of information that are the most important to our behavior, goals, and situation context (Tannock et al., 1995; Levin et al., 2005; Baars and Gage, 2010; Pillay et al., 2012). For this scoping review the term relevancy will be defined as relevance to the task at hand as well as relevance to the simulated environment. Although distraction has been described with characteristics such as relevancy, perhaps there are more characteristics that can be utilized for their implementation into simulation assessments. For the purposes of this study authors are interested in extrapolating distraction characteristics among simulation research either for task disruption or enhancement.
Recently, computer-assisted assessment approaches have been used to simulate everyday environments in order to analyze human performance in everyday life (Eliason et al., 1987; Rizzo et al., 2000; Lalonde et al., 2013; Bouchard and Rizzo, 2019; Mozgai et al., 2019; Saredakis et al., 2020). Computerized approaches can simulate functional everyday environments with greater fidelity as technology has improved. The simulation hardware includes computers, tablets, smartphones, as well as virtual reality (VR) and augmented reality (AR) programming. Visual displays have evolved from computer monitors to 3-dimentsional (3D) displays to head mounted displays (HMDs) with 360° views of the environment. VR and computer programs offer a variety of display options that increase the sense of “presence” within a program. Presence refers to the feeling that the user is in the simulated environment. It is hypothesized that the sense of presence will increase the likelihood the user will interact as they would in the real world. However, increasing the range of visual representations within simulation programming also comes with the risk of triggering simulation sickness or motion sickness in participants wearing HMDs. Although certain clinical and healthy populations may suffer from simulator sickness during or after HMD usage, improvements in updating visual displays, often 90 times per second, have decreased the prevalence of this issue (Bouchard and Rizzo, 2019). Saredakis et al. (2020) completed a systematic review and meta-analysis on the factors associated with VR sickness in HMDs. Results indicated that symptoms of dizziness, blurred vision, and difficulty focusing may be more likely to develop when using HMDs based on pooled Simulation Sickness Questionnaire ratings (SSQ) (Saredakis et al., 2020). Also, SSQ subscale scores were lower for studies with simulation exposure <10 min as compared to those that were equal or 10 min (Saredakis et al., 2020).
Examination of how distractions have been incorporated into computerized simulations has been given little attention. However, attention and memory processes require adjustment to meet the demands of environmental distractions for successful task completion. Therefore, it is critical to understand what distraction characteristics impact attention and memory to improve the sensitivity and specificity of clinical tools as well as clinical recommendations for home, academic, social, and vocational independence. Further investigation into methods for distraction implementation, types of distractions, and their impact on attention and memory could provide useful information for both researchers and clinicians. The purpose of this scoping review is to map how distractions have been utilized in simulated environments (Tricco et al., 2016). Authors will address the following question:
RQ1: How and what type distractions have been implemented in simulation research to target clinical populations with attention and/or memory deficits?
Methods
Identifying Relevant Studies
Authors utilized PRISMA guidelines and protocols to complete this scoping review. We completed a systematic search of the literature on distractions utilized in computer-assisted assessment of attention and memory in five database searches including PsychInfo, PubMed, RehabData, Scopus, and Web of Science. The literature search focused on VR, AR, and computer-based programs that utilized distractions for populations with attention and memory disorders. A search strategy was developed in collaboration with a medical school librarian at the University of Kentucky. Articles were searched from any point in time until October 20, 2020. In addition to key word searches, a hand-search of citations for pertinent articles was conducted. The following search terms were used for the retrieval of relevant articles:
(((((virtual reality) OR computer-based) OR augmented reality) AND attention) OR memory) AND distractions.
((Virtual Reality OR Computer-Based) AND (Attention OR Memory) AND Distractions) NOT (Pain Management OR Surgery OR Exposure Therapy)
(TITLE-ABS-KEY (virtual AND reality) OR TITLE-ABS-KEY (computer-based) OR TITLE-ABS-KEY augmented AND reality) AND TITLE-ABS-KEY (attention) OR TITLE-ABS-KEY (memory) AND TITLE-ABS-KEY (distractions))
Study Selection
To meet inclusion criteria articles needed to be written or available in English, included and focused on clinical populations with attention and/or memory deficits, utilized distractions within a simulation (VR, AR, or Computer-Based), used any experimental design, and was written prior to literature search date. To ensure a comprehensive literature search authors included other scholarly work that provided descriptions of programs that included distractions.
Articles were excluded if they; 1) only utilized a healthy population, 2) included second language learners, and 3) if they did not assess attention or memory (i.e., assessed limb movement, pain management, balance, etc.). Pain management articles were excluded if they did not assess attention or memory and included distractions. Articles that targeted PTSD or other anxiety disorders were excluded if they did not target attention or memory, did not document distractions, and were not a simulation-based study.
A total of 661 articles were found from the five databases used (PsychInfo, PubMed, RehabData, Scopus, and Web of Science) and hand searching. Forty-six duplicates were removed using EndNote software this left the authors with 615 articles to be screened. Initial screening was done based on article title and abstract excluding irrelevant studies using the inclusion and exclusion criteria. After the initial screening, 61 articles were chosen to read the full text. After reading all 61 articles 38 articles were excluded with reasons including lack of clinical population, no assessment program, and no distractions measured or implemented. Additionally, authors did not find data from any unpublished articles that met the inclusion criteria for this review. In total 23 articles were identified as relevant. One independent author screened article titles and identified relevant articles. Two independent investigators extracted data from each article. Any discrepancies in data extraction were resolved between investigators. Authors discussed and identified pertinent distraction factors and characteristics from each article to be examined within this paper. The full screening process is visualized in Figure 1.
Outlining the Data
Twenty-three articles that satisfied the inclusion for this review. Data extracted included the following information: population, study design, purpose, distraction modality, distraction characteristics, distraction location, distraction timing, distraction location, target, measurement, and program. If the study did not provide details of distractions used, the authors were contacted via email. Supplementary Tables S1, S2 in the supplementary materials provides a complete overview of all data extracted for this scoping review.
Results
Range, Nature, and Extent of Included Studies
Twenty-three articles met inclusion criteria for this scoping review. Figure 1 provides a flow diagram of the selection process for included articles. The chosen articles included seven clinical populations: TBI (n = 7), ADHD (n = 6), autism spectrum (n = 3), stroke (n = 1), PD (n = 3), fibromyalgia (n = 1), and cognitive decline with aging (n = 2). The most common study design type was a non-randomized clinical control trial (n = 21) followed by a randomized controlled trial (n = 1) and one program description (n = 1). In the non-randomized clinical control trials, clinical populations were compared to healthy controls in conditions with and without distractions. In the randomized control trial participants were assigned to two groups who received traditional and VR based therapy. Table 1 includes a summary of the distraction modality, statistical analysis, and p-values of group performance differences in the presence of distraction for each article. A richer description of distraction characteristics and study outcomes are provided in the subsequent sections of this article. A more comprehensive table of distraction descriptions and additional relevant outcome measures are in Supplementary Tables S1, S2 of the supplementary material. Due to the variability in nature of the included article simulation programs, measurement outcomes, and clinical populations authors were unable to conduct a systematic review. The age ranges from selected articles included elementary school aged children to older adults. Control groups were present in 22 articles. Assessment measures used to examine attention and memory included standardized assessments (CPT, STROOP, WAIS). Additional tasks included cognitive and executive function targets to investigate attention, prospective, working, and long-term memory. Articles featured a range of research disciplines including psychology, neuropsychology, psychiatry, neurology, cognitive neuroscience, neuro-engineering, and computer science. Literature maps of distraction modality and distraction elements are depicted in Figure 2.
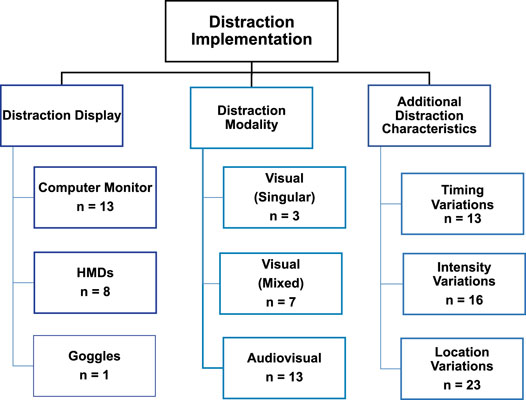
FIGURE 2. Literature map of distraction display, modality, and additional characteristics across all clinical populations.
Distractions
Distraction Display
The primary methods of display options for distractions were HMDs and computer monitors. Of the included articles head mounted displays (HMDs) were utilized in eight studies (8/23, 35%) (Parsons et al., 2007; Adams et al., 2009; Nolin et al., 2009; Diaz-Orueta et al., 2014; Parsons and Carlew, 2016; Ansado et al., 2018; Ouellet et al., 2018; Yeh et al., 2020). It is important to note that not all clinical populations are good candidates for HMDs due to increased susceptibility to simulator sickness and/or hypersensitivity to audiovisual stimuli. Computer monitor displays were utilized in thirteen studies (13/23, 57%) (Dockree et al., 2004; Knight et al., 2006; Pollak et al., 2009; Potvin et al., 2011; Schnabel and Kydd, 2012; Krch et al., 2013; Faria et al., 2016; Uitvlugt et al., 2016; Remington et al., 2019; Banire et al., 2020; Biss et al., 2020; Park et al., 2020; Pretus et al., 2020). One study (1/23, 4%) utilized a goggle system, the NordicNeuroLab goggle system for the presentation of distraction stimuli (Harrington et al., 2019). One study (1/23, 4%) monitored environmental distractions that naturally occurred in the participants’ environments (Kratz et al., 2019; Kratz et al., 2020). The included studies did not report on distraction caused by external distraction in the testing environment such as auditory or visual stimuli beyond the computer monitor display or outside of the HMD.
Internal Distraction
Baseline measures for internal distractions such as simulator sickness, pain, anxiety, and fatigue were completed for eight articles (7/23, 30%) (Dockree et al., 2004; Harrington et al., 2020; Adams et al., 2009; Potvin et al., 2011; Biss et al., 2020; Kratz et al., 2020; Park et al., 2020). In the Dockree et al. (2004) study statistically significant differences were detected in persons with TBI and healthy controls on the Cognitive Failures Questionnaire (CFQ) (Broadbent et al., 1982) but not on the Hospital Anxiety Depression Scale (HADS) (Zigmond and Snaith, 1983). Biss et al. (2020) measured anxiety and depression utilizing HADS, however differences in scores between aMCI and older healthy controls was not statistically significant. Potvin et al. (2011) measured anxiety by the Beck Anxiety Inventory (BAI) (Beck and Steer, 1993) and depression BDI-II questionnaires (Beck Depression Inventory second edition) (Beck et al., 1996). Results indicated a lack of correlation between outcome measures and questionnaires by persons with TBI, however participant results were associated with their relatives’ perception of their everyday prospective memory functioning. Park et al. (2020) assessed depression by the Japanese version of Geriatric Depression Scale (GDS-S-J) (Yesavage et al., 1986; Sugishita et al., 2009) and results were higher for the PD group than healthy control group however scores did not statistically correlate memory encoding dysfunction. Harrington et al., 2020 measured daytime sleepiness (Epworth Sleepiness Scale) and depression symptoms (Hamilton Depression Scale) at baseline but results did not differ between PD group and healthy controls. Kratz et al. (2020) baseline measures included the Patient-Reported Outcome Measures Information System (PROMIS) Pain intensity 3a Short Form; Patient Health Questionnaire-8 (PHQ-8), PROMIS fatigue item bank. Participants with fibromyalgia reported significantly worse scores on measures of depression, pain, fatigue, and objective cognitive function but differences with healthy controls was not significant (Kratz et al., 2020).
One article displaying simulated environments by HMDs evaluated simulation sickness by the Simulation Sickness Questionnaire (Kennedy et al., 1992; SSQ; Parsons et al., 2007; Adams et al., 2009). Results indicated that ADHD participants reported no or minimal simulator sickness after completed the Virtual Classroom experience.
Auditory Distraction
None (0/23, 0%) of the included articles utilized singular or mixed auditory distractions without the additional presence of visual distraction.
Visual Distraction
Three articles implemented singular static visual distractions (3/23, 13%) (Dockree et al., 2004; Uitvlugt et al., 2016; Remington et al., 2019). Included relevant and irrelevant visual distractions ranged from simple shapes or letters to pictures and videos. Remington et al. (2019) added relevant and irrelevant visual images during a story listening task. During the irrelevant distraction condition, children with autism were able to recall both verbal information as well as the irrelevant information displayed (p > 0.4) (Remington et al., 2019). The results suggest distracting conditions may support more capacity for information processing.
Dockree et al. (2004) included relevant distractions (i.e., numbers) during a go-no-go task. Results indicated that persons with TBI were more susceptible to distraction when a distractor image appeared during a computerized task. Since the TBI group was more susceptible to distraction, their performance resulted in an increased amount of errors as compared to healthy controls (p = 0.026).
Uitvlugt et al. (2016) included relevant visual distractions (two yellow and three red dots) that were related to the assessment task (memorizing dot locations). The aim of Uitvlugt et al. (2016) study was to assess distractibility with persons diagnosed with PD on and off medication. Findings indicated that individuals on medication were more susceptible to distraction (p = 0.009). The “on” medication state enabled participants’ working memory system to update with sensory information including distraction as opposed to the “off” medication state, which did not update the working memory system, thus inhibiting detection of distraction.
Seven articles incorporated multiple visual distractions (7/23, 30%) (Pollak et al., 2009; Faria et al., 2016; Harrington et al., 2020; Banire et al., 2020; Biss et al., 2020; Park et al., 2020; Pretus et al., 2020). Distractions included: hand drawn animation, toys, shapes, words, faces, cars, streets, commercial buildings, and moving cars. Distractions were deemed as “multiple” because they co-occurred with one another.
Time estimation differences were tested between adults with ADHD and healthy controls in the Pretus et al. (2020) study. Irrelevant distraction images of animals were included in the time estimation task. The distractor trial analysis indicated enhanced time estimation performance in the ADHD group (p = 0.032). Additionally, fMRI imaging detected greater superior orbitofrontal activation in the ADHD group, suggesting distractors may recruit attentional resources for focus (Pretus et al., 2020). Pollak et al. (2009) investigated time estimation differences between adults with ADHD and healthy controls. Irrelevant visual distractions incorporated dynamic visual stimuli consisting of videos and hand-drawn animation. Results indicated that adults with ADHD demonstrated greater and more variable deviation in time estimation when distractions were present (p < 0.001). Biss et al. (2020) tested facial and name recall between older adults with mild cognitive impairment and healthy older adults. Distractions included irrelevant non-words, relevant incorrect names, and relevant pictures of faces. The group with mild cognitive impairment recalled significantly fewer names than healthy controls in the presence of distraction (face repetitions p = 0.007, immediate recall p = 0.048, delayed recall p = 0.29) (Biss et al., 2020).
Banire et al. (2020) included relevant visual distractions in a classroom setting and utilized eye tracking to measure eye fixation during the CPT between children with autism and healthy controls. Findings indicated that significant differences were detected in CPT scores and sum of fixation count between experimental groups (p < 0.05). Statistical differences were not detected between CPT scores and average fixation time, but the scores were correlated (Banire et al., 2020).
Faria et al. (2016) investigated differences between individuals with post stroke conditions receiving traditional therapy and individuals post stroke receiving therapy via the Rehab@City program. During the Rehab@City program participants with post stroke conditions virtually entered stores and completed cognitive and executive functioning tasks. When participants transitioned to a different store, relevant video distractions of people were played. Neuropsychological assessments included the Addenbrooke Cognitive Examination (ACE) (Mioshi et al., 2006) to assess global cognition, Trail Making Test A and B (TMT A and B) (Rietan, 1958) to assess attention and executive functioning, Picture Arrangement test from the Wechsler Adult Intelligence Scale III (WAIS III) (Ryan et al., 2001) to assess executive functions. Between group analysis revealed that the experimental group improved in global cognition (p = 0.014), attention (p = 0.018), memory (p = 0.018) and executive function (p = 0.026) as compared to the control group.
Harrington’s et al. (2019) study integrated relevant distractions of filled and unfilled shapes that were presented during a visuospatial working memory task for persons with PD and healthy controls. Distractions included varying colors, shapes, and sizes of boxes of all shapes. Working memory scores fell within the normal range for PD group, however fMRI images indicated that connectivity differences were detected (p < 0.01) (Harrington et al., 2019). In a long-term memory study Park et al. (2020) analyzed persons with PD’s ability to encode faces based on semantic, attractiveness, and form judgments. Relevant visual distractions included unfamiliar faces. Frontal lobe function and long-term memory was correlated with retrial of faces based on semantics and attractiveness. The healthy control group retrieved more faces encoded by semantic and attractiveness judgements as compared to the PD group (p < 0.01) (Park et al., 2020).
Audiovisual Distraction
Thirteen articles combined both auditory and visual distractions for attention and memory assessment (13/23, 57%) (Knight et al., 2006; Parsons et al., 2007; Adams et al., 2009; Nolin et al., 2009; Potvin et al., 2011; Schnabel and Kydd, 2012; Krch et al., 2013; Díaz-Orueta et al., 2014; Parsons and Carlew, 2016; Ansado et al., 2018; Ouellet et al., 2018; Kratz et al., 2020; Yeh et al., 2020). Auditory distractions included background music, broadcasted news, chatter, cars, horns, pencils dropping, and bells from a cash register. Visual distractions were comprised of shops, people, videos, photos, and advertising. Distraction locations ranged from stationary to moving in multiple locations. Simulated environments included a classroom, post office, supermarket, bank, pharmacy, and office.
The Virtual Street program by Knight et al. (2006) studied prospective memory performance in the presence of “low; and “high” areas of relevant and irrelevant distractions for persons with TBI. Low areas of distraction contained only sounds of footsteps and traffic noise while images were static. High areas of distraction included videos of actors creating the scene of a busy street, also sounds louder and included car horns, radio news, rock/rap music, and weather broadcasts. Results indicated that deficits were not necessarily due to an inability to complete prospective memory tasks. However, errors or delays in task completion were a direct consequence of distraction (p < 0.05). The TEMP program also assessed prospective memory performance during relevant and irrelevant distraction for persons with TBI (Potvin et al., 2011). The TEMP program plays a movie featuring driving to various shops within the community while completing prospective memory tasks (time and event-based conditions). A ceiling effect occurred for the healthy control group for event-based and time-based recall conditions. A statistically significant conditions scoring a 90% or more of the delayed intentions at the correct time. 21 participants in the TBI group detected 90% or more prospective cues and nine participants in the TBI group detected less than 80% of the prospective cues (seven who did not detect any). Statistically significances between groups also occurred for event-based task detection (p = 0.02) and deviation from the target time (p < 0.05). effect occurred for group (p < 0.05), and condition (p < 0.05) for actions correctly retrieved (Potvin et al., 2011). Krch et al. (2013) created the Assessim Office to assess executive function in persons with TBI and multiple sclerosis (MS) in an office setting with relevant and irrelevant distractions. The Assessim Office was able to distinguish between persons with TBI, MS, and healthy controls in the areas of selective and divided attention, problem solving, and prospective memory. Statistically significant differences were found between TBI and healthy control groups for correct real estate decisions (p = 0.007), and printing real estate offers (p = 0.011). Statistically significant differences were also detected between the MS group and healthy controls for correct real estate decisions (p = 0.030) and turning the projector light on (p = 0.010) (Krch et al., 2013). Schnabel and Kydd (2012) studied attention, working, and logical memory in persons with TBI and depression in the presence of irrelevant audiovisual distractions using the Assessim Office. The TBI group demonstrated a significant decline in scores during the distraction condition while performing the WAIS-IV DSF, WAIS-IV DST, and WMS-IV LM as compared to the healthy control group (p < 0.001). Interestingly, participants who were diagnosed with depression showed improvement during the distraction condition but also reported an increase in stress to an “unbearable” level (Schnabel and Kydd, 2012).
Ouellet et al. (2018) aimed to develop an ecologically valid VR environment and scenario that measured everyday memory in older adults (Virtual Shop). Participants completed a shopping task in the presence of irrelevant ambient noise and relevant visual distractions in the same semantic category. Groups were statistically different for less correct responses, response initiation for first selection, and time required for task completion (p < 0.05) (Ouellet et al., 2018).
The Virtual Classroom incorporated relevant and irrelevant audiovisual distractions including background chatter, environmental noises, new people entering the classroom, and various objects moving during the CPT. Six of the included articles utilized the Virtual Classroom for assessment purposes (Parsons et al., 2007; Adams et al., 2009; Nolin et al., 2009; Díaz-Orueta et al., 2014; Parsons and Carlew, 2016; Yeh et al., 2020). Results indicated that the Virtual Classroom was sensitive to detecting differences between students with ADHD, autism, TBI, and healthy controls.
Kratz et al. (2020) focused on “fibrofog” due to Fibromyalgia (FM), using a smart phone and wrist-watch accelerometer. Across eight days participants reported perceived cognitive function and environmental distraction five times per day and completed tests of processing speed and working memory. Statistically significant differences were detected between groups for distractions present during cognitive tasks (p < 0.001), number of distractions (p < 0.001), and physical location (p = 0.02). The FM group had a significantly higher reporting of distraction during cognitive tests and reported multiple simultaneous distractions. Yet, interactions between groups for subjective and objective measures of cognitive function were not significant (p > 0.70). Both groups reported auditory distractions as the most common cause of distraction (Kratz et al., 2020). However, the FM group reported more distractibility in their home environment as opposed to outdoors. This finding suggests that individuals with FM will perceive more stimuli because of perceptual amplification and hypersensitivity of (internal and external) distraction (McDermid et al., 1996).
Additional Characteristics of Distraction: Location, Timing, and Intensity
In addition to reporting distraction modalities and relevancy, data from additional elements of distraction was extracted. Additional elements of distraction include distraction location, timing, and intensity. Eighteen articles (18/23, 78%) incorporated distractions in multiple locations (Dockree et al., 2004; Knight et al., 2006; Parsons et al., 2007; Adams et al., 2009; Nolin et al., 2009; Pollak et al., 2009; Potvin et al., 2011; Krch et al., 2013; Díaz-Orueta et al., 2014; Faria et al., 2016; Parsons and Carlew, 2016; Uitvlugt et al., 2016; Ansado et al., 2018; Ouellet et al., 2018; Harrington et al., 2019; Banire et al., 2020; Kratz et al., 2020; Yeh et al., 2020). Primary locations included: right-front, left-front, center-front, and behind-right, behind-left, and behind-center. Five articles (5/23, 22%) utilized singular locations (Schnabel et al., 2012; Biss et al., 2020; Park et al., 2020; Pretus et al., 2020; Remmington et al., 2020). Since the target measures of the included studies were attention and memory the impact of distraction location was not measured specifically for except for Banire et al. (2020). Results indicated that children with ASD demonstrated more interest in irrelevant areas of interest which contributed to their distractibility and CPT scores.
Seven articles (7/23, 30%) included distractions at random intervals (Parsons et al., 2007; Díaz-Orueta et al., 2014; Parsons and Carlew, 2016; Uitvlugt et al., 2016; Biss et al., 2020; Kratz et al., 2020; Park et al., 2020), six (6/23, 26%) included distractions at specific intervals (Dockree et al., 2004; Pollak et al., 2009; Harrington et al., 2019; Remmington et al., 2019; Pretus et al., 2020; Yeh et al., 2020), and four (4/23, 17%) included distractions continuously (Potvin et al., 2011; Schnabel and Kydd, 2012; Ouellet et al., 2018; Banire et al., 2020). Seven articles (7/23, 30%) did not specifically report on the timing of distractions within their programs. Since the target measures of the included studies were attention and memory the impact of distraction timing was not measured.
Sixteen articles (16/23, 70%) identified or included information about distraction intensities (Adams et al., 2009; Ansado et al., 2018; Banire et al., 2020; Dockree et al., 2004; Faria et al., 2016; Harrington et al., 2019; Knight et al., 2006; Krch et al., 2013; Ouellet et al., 2018; Park et al., 2020; Parsons et al., 2007; Parsons et al., 2016; Potvin et al., 2011; Remington, 2019; Schnabel et al., 2012; Yeh et al., 2020. Although these articles did not define distraction intensity, variables emerged that could impact the level of distraction difficulty. Intensity variables included: predictability, threat, loudness, color, contrast, and the amount of distraction present at once.
Distraction Intent
Studies by Pretus et al. (2020) and Remmington et al. (2019) indicate that the presence of distraction may recruit more attentional resources in order to increase focus on the assigned task. Harrington et al. (2019) study indicated an increase in brain activation during the encoding (superior parietal, precuneus, occipital cortices, cerebellum, middle frontal gyrus, posterior parahippocampal cortex, putamen, globus pallidus) and retrieval (superior parietal cortex and precuneus) of information in the presence of distraction. Harrington’s findings suggest that more effort is required when distractions are present, thus recruiting more attentional resources and that distractions disrupt the encoding and retrieval of information. However, these results do not indicate if an increase in attentional resources due to distractions elicit a negative impact on performance task or enhancement of task performance. Current literature does not come to a singular conclusion or consensus on the impact of distraction. After analyzing the data, the authors propose that distraction can serve two different purposes. Distraction can be the following:
1. Any stimuli of any sensory modality that disrupts the encoding, storage, retrieval, and inhibition for task completion. Stimuli can either be auditory, visual, and/or internal.
2. Any stimuli of any sensory modality that enhances task performance by increasing attentional focus and decreasing internal states (i.e., mind wandering).
Tasks
Articles included in this scoping review primarily focused on the specificity of the program for assessment purposes. During data synthesis CPT, and prospective/working memory tasks emerged as the primary assessments that were used in the presence of distraction on 14 articles (14/23, 57%) (CPT) (Adams et al., 2009; Banire et al., 2020; Diaz-Orueta et al., 2014; Nolin et al., 2009; Parsons et al., 2007; Yeh et al., 2020) (prospective/memory) (Knight et al., 2006; Pollak et al., 2009; Potvin et al., 2011; Krch et al., 2013; Uitvlugt et al., 2016; Ansado et al., 2018; Ouellet et al., 2018; Harrington et al., 2019). Additional measures included: WAIS on two articles (2/23, 7%) (Schnabel et al., 2012; Faria et al., 2016), STROOP task on one article (1/23, 4%) (Parsons et al., 2016), immediate and delayed recall on one article (1/23, 4%) (Biss et al., 2020), delayed recall on two articles (2/23, 7%) (Remington et al., 2019; Park et al., 2020) dot task and symbol search (Kratz et al., 2020), time estimation (Pretus et al., 2020), and attention (go/no go) on one article (1/23, 4%) (Dockree et al., 2004).
Discussion
This scoping review is the first of its kind to describe the implementation of distractions in computer-assisted assessment of attention and memory. Twenty-three articles met inclusion criteria and were analyzed. Articles included clinical populations of TBI, ADHD, autism spectrum, stroke, PD, fibromyalgia, and cognitive decline with aging. The selection of distraction display options was based on characteristics of the clinical population being assessed. For example, Banire et al. (2020) utilized a desktop display because HMDs may induce dizziness or other sensations for clinical populations with Autism (Bellani et al., 2011). Primary measures of attention and memory in the presence of distraction included: CPT, STROOP, WAIS and measures targeting immediate, delayed, and working memory. The included articles did not include a standardized approach to the introduction of distractor elements. Distractions primarily included auditory and visual stimuli however, we found three supplementary elements that also contribute to the distraction identification, 1) distraction location, 2) distraction intensity, and 3) distraction timing. Measurements of performance in the presence of distraction included body movement, omission, commission, and timing errors, vigilance, time and event-based memory, immediate and long-term memory. Tasks included the CPT, STROOP, WAIS, face/name recall, dot memory, symbol search, go/no go, story recall, time estimation, and selecting objects in a store. Although measures distinguished differences in performance between healthy groups and clinical populations more research is needed to create measures to aid in making predictions of everyday behavior. For example, a task that is matched to the simulated environment that includes common distractions may be more difficult as compared to a task that is far removed from the environmental context.
Included articles baseline measures targeted internal distractions such as pain, fatigue, and anxiety that commonly occur in PAMDs (Moore et al., 2009; Sciberras et al., 2014). Although there is mixed evidence on the impact of anxiety and cognition, there are studies that have demonstrated a reliable relationship between anxiety and interference from distractors in search tasks (Bar-Haim et al., 2007; Moser et al., 2013). However only one article investigated internal distraction (anxiety) and its impact on memory encoding and did not discover a correlation. Since internal distraction, including simulation sickness can significantly impact attention, studies could include measures pre, during, and post simulation to correlate with attention and memory measures.
Programming Recommendations
The relationship between task demands and type, intensity, and amount of distraction may play a large role in eliciting cognitive targets to reflect everyday functioning. Without intentional measurement and dosing of all three components it is questionable if the implemented distractions are truly eliciting everyday function. Dosing distractions should be considered in relation to the simulated environment and task demands. Task demands should be considered in conjunction with distraction modality and intensity. If a task is too difficult or too easy the distraction may not serve its intended purpose. Similarly, if the distraction is too distracting or not distracting enough, ceiling or flooring effects can occur.
Although many of the included articles utilized baseline anxiety, depression, or pain measures prior to simulation experiences, these measures could be given after the simulation. Qualitative measures could provide insights into distraction experiences as well as recording unanticipated internal distractions within target environments. Implementing pre- and post-qualitative measures could be beneficial for improving the sense of presence within simulated environments. Measures also including simulation sickness, fit of the HMD, distractions beyond the computer monitor display, or outside of the HMD (experimenters voice) could improve user experiences and simulation methodology.
The majority of simulated environments included elementary classrooms or shopping settings. Since PAMDs come from different socioeconomic, racial, and cultural backgrounds, computer-assisted assessment should include diverse environments and contexts. PAMDs struggle with managing distractions in environments such as social, vocational, medical, and housing scenarios that have a distinct subset of rules and tasks to follow. When distractions disrupt inhibition and recall of rules/tasks, PAMDs are at risk for being mislabeled as non-compliant and are therefore not allowed to continue participation. This may be particularly useful for clinical populations that think concretely or have difficulty manipulating strategies across environments and contexts.
Studies included in this scoping review primarily studied PAMDs’ assessment outcomes in the presence of distraction. However, training programs are also needed in order to make inferences about everyday function. Training programs could aid in the education, practice, and generalization of recommended strategies to everyday contexts. Clinicians would also be equipped to observe patients’ use of recommended strategies in a simulated everyday environment. Observational assessment could enable clinicians to adjust strategies to be individualized to their patients’ performance across different environments and contexts. Computer-assisted training programs would allow training and practice of strategies in low consequence environments (i.e., clinic). Adaptive programs that increase or decrease the difficulty level (i.e., distraction intensity) based on performance could also aid in patient assessment and training. Since rehabilitation has shifted to include more technology-based applications, adaptive programs would help in participant engagement and motivation.
Measurement Recommendations
In addition to standardized measures, researchers should select cognitive and executive functioning targets/measures in conjunction with everyday tasks. Many standardized neuropsychological assessments can detect if an impairment is present and distinguish between disordered and healthy populations. Still, identifying distraction characteristics that impact attention would enable researchers to systematically increase the degree or level of distraction to reflect what PAMDs encounter daily. The systematic implementation of real-world distractions in these assessments has the potential to improve ecological validity, and therefore greater predictive validity than traditional pen-and-paper tasks. Therefore, tasks assigned in simulations should mimic everyday tasks in the presence of distraction to predict real-life functioning. Since variation in performance occurs for PAMDs across contexts, tasks, and environments all three variables should be chosen carefully.
Internal distraction is an underrepresented modality of distraction that holds key information for assessment and rehabilitative purposes. It is reasonable to assume that internal distractions can be continuous, discontinuous, or randomized while co-occurring with other modalities of distraction. Breakdowns in attention more than likely occur when internal distraction floods attentional allocation systems. Until the internal distraction is resolved it could overpower the attentional allocation system for an extended period of time. Researchers could include measurements of internal distraction (i.e., surveys, questionnaires, and interviews) before, during, and after simulation. Although the literature is mixed on the impact of internal distraction, dosing of the internal element could be a critical barrier (Davidson et al., 1990; Garrett and Maddock, 2001; Gross, 1998) to understanding attention. Also, investigating internal distraction could uncover hidden attentional biases that do not overtly present themselves. The degree of how internal distractions impact life domains for clinical populations is not yet known. Internal states such as PTSD, OCD, anxiety, depression, pain, and fatigue commonly occur in both healthy and clinical populations. Also, measuring unintentional distraction such as motion or simulator sickness would be beneficial in assessing the sense of presence within a VR program. Measuring internal states will have a twofold benefit, first, it would provide a measurement of a variable that cannot be easily seen/observed. Secondly, comparing pre- and post-measurements of internal states could provide insight into variability with task performance.
Results from this scoping review indicate that distractions can enhance or disrupt task performance. Therefore, this surprising discovery should be explored further. The included articles did not identify which characteristics of distraction enhanced task performance. However, promising research for PD populations are demonstrating a lack of strategies based on distraction characteristics such as semantic and attractiveness features (Park et al., 2020). Task enhancement distraction research would be beneficial to both healthy and clinical populations to identify environmental accommodations for optimal attentional capacity. For clinical populations, such as autism spectrum disorder and ADHD, background distractions actually enhanced attentional capacity (Remington et al., 2019; Pretus et al., 2020). Enhanced attention capacity occurred due to the increased amount of attentional resources when distractions were present as compared to distraction-free environments that promoted “mind wandering” (i.e., internal distraction). However, ceiling effects occurred for both experimental groups during the Remington et al. (2019) study, which suggests dosing parameters for distractions are needed to test attentional capacity thresholds.
Limitations
This scoping review has several limitations. First, there is a risk of publication bias for studies that were not published due to positive or no effect from distraction. Unpublished studies may have had an unanticipated enhancement of task performance or no effect at all. However, authors did not find data from any unpublished articles to be included in this review. Second, since computer-assisted assessment studies are relatively new, articles may have been published after the search dates for this article. Third, this article focuses on programs for clinical populations where there is inherent variability in the outcomes. Knowing that clinical populations vary in diagnosis, severity, and outcomes an overall effect size is not feasible to calculate. Finally, the search of databases was based on a predefined set of search terms. The search strategy adheres to established methodologies and procedures for the scoping review process. However, terminology within computer-assisted assessment research has varied across the past thirty years, future reviews could consider including the following search terms: mixed-reality, user-computer interface, computerized models, and computer-assisted instruction.
Conclusion
Computer-assisted assessments for attention and memory simulating everyday environments are becoming more commonplace. However, this review demonstrates that additional focus is needed to measure, and dose everyday distractions. Authors developed recommendations for both measurement and programming. Identification of distraction elements is a promising first step in the process of developing computer-assisted programs that elicit everyday functioning. Also, identification of dosing parameters for specific clinical populations is needed. The information from this scoping review supports prior findings that computer simulation can be an effective tool for the assessment and rehabilitation of clinical populations (Rizzo et al., 1997; Schultheis et al., 2002; Rose et al., 2005). It also supports the possibility to systematically present cognitive tasks that span beyond what is offered by traditional standardized measures (Lengenfelder et al., 2002; Barkley, 2004; Rose et al., 2005; Clancy et al., 2006). Since literature that combines both simulations and distraction is scarce, there are still several unanswered questions remaining specifically for distractions that enhance performance. Also, for examining how modalities, intensities, locations, and timing of distractions impact task performance for both PAMDs and healthy populations.
Author Contributions
DP contributed to the conceptualization, design, article search, data extraction and analysis, interpretation of results, and manuscript development. PM and IH contributed to the conceptualization and design of the project as well as the interpretation of results and manuscript edits. All authors agreed on the final manuscript.
Conflict of Interest
The authors declare that the research was conducted in the absence of any commercial or financial relationships that could be construed as a potential conflict of interest.
Acknowledgments
Authors would like to thank the reviewers for volunteering their time and expertize with reviewing this article. We would like to thank the University of Kentucky Medical School Librarians for assisting in the development of key word searches and search strategy for this review. We would also like to thank Vrushali Angadi, Maria Bane, Mohammed ALHarbi, and Hannah Douglas from the University of Kentucky for their ongoing support and help with refining design concepts for this scoping review. We would also like to thank Brooklyn Knight from the University of Kentucky for help with data extraction validation.
Supplementary Material
The Supplementary Material for this article can be found online at: https://www.frontiersin.org/articles/10.3389/frvir.2021.685921/full#supplementary-material
References
Abikoff, H., Courtney, M. E., Szeibel, P. J., and Koplewicz, H. S. (1996). The Effects of Auditory Stimulation on the Arithmetic Performance of Children with ADHD and Nondisabled Children. J. Learn. Disabil. 29, 238–246. doi:10.1177/002221949602900302
Adams, R., Finn, P., Moes, E., Flannery, K., and Rizzo, A. S. (2009). Distractibility in Attention/Deficit/Hyperactivity Disorder (ADHD): The Virtual Reality Classroom. Child. Neuropsychol. 15 (2), 120–135. doi:10.1080/09297040802169077
Adreon, D., and Durocher, J. S. (2016). Evaluating the College Transition Needs of Individuals with High-Functioning Autism Spectrum Disorders. Intervention Sch. clinic 42 (5), 271–279. doi:10.1177/10534512070420050201
Ansado, J., Brulé, J., Chasen, C., Northoff, G., and Bouchard, S. (2018). The Virtual Reality Working-Memory-Training Program (VR WORK M): Description of an Individualized, Integrated Program. Annu. Rev. CyberTherapy Telemed. 16, 101–117.
Baars, B. J., and Gage, N. M. (2010). Cognition, Brain, and Consciousness: Introduction to Cognitive Neuroscience. 2nd ed.. Oxford, UK: Elsevier.
Baddeley, A. D., and Hitch, G. (1974). “Working Memory,” in Psychology of Learning and Motivation (Elsevier), 47–89. doi:10.1016/s0079-7421(08)60452-1
Banire, B., Al Thani, D., Qaraqe, M., Mansoor, B., and Makki, M. (2020). Impact of Mainstream Classroom Setting on Attention of Children with Autism Spectrum Disorder: An Eye-Tracking Study. Univ Access Inf Soc. doi:10.1007/s10209-020-00749-0
Bar-Haim, Y., Lamy, D., Pergamin, L., Bakermans-Kranenburg, M. J., and van IJzendoorn, M. H. (2007). Threat-related Attentional Bias in Anxious and Nonanxious Individuals: A Meta-Analytic Study. Psychol. Bull. 133 (1), 1–24. doi:10.1037/0033-2909.133.1.1
Barkley, R. A., Koplowitz, S., Anderson, T., and McMurray, M. B. (1997). Sense of Time in Children with ADHD: Effects of Duration, Distraction, and Stimulant Medication. J. Int. Neuropsychol. Soc. 3 (4), 359–369.
Barkley, R. A. (2004). Adolescents with Attention-Deficit/hyperactivity Disorder: an Overview of Empirically Based Treatments. J. Psychiatr. Pract. 10 (1), 39–56. doi:10.1097/00131746-200401000-00005
Beaman, C. P. (2005). Auditory Distraction from Low-Intensity Noise: a Review of the Consequences for Learning and Workplace Environments. Appl. Cognit. Psychol. 19 (8), 1041–1064. doi:10.1002/acp.1134
Beaman, C. P., and Jones, D. M. (1997). Role of Serial Order in the Irrelevant Speech Effect: Tests of the Changing-State Hypothesis. J. Exp. Psychol. Learn. Mem. Cogn. 23 (2), 459–471. doi:10.1037/0278-7393.23.2.459
Beck, A. T., and Steer, R. A. (1993). Beck Anxiety Inventory. Toronto, ON: The Psychological Corporation.
Beck, A. T., Steer, R. A., and Brown, G. K. (1996). Beck Depression Inventory. 2nd ed.. Toronto, ON: The psychological corporation.
Bellani, M., Fornasari, L., Chittaro, L., and Brambilla, P. (2011). Virtual Reality in Autism: State of the Art. Epidemiol. Psychiatr. Sci. 20, 235–238. doi:10.1017/s2045796011000448
Biss, R. K., Rowe, G., Hasher, L., and Murphy, K. J. (2020). An Incidental Learning Method to Improve Face-Name Memory in Older Adults with Amnestic Mild Cognitive Impairment. J. Int. Neuropsychol. Soc. 26 (9), 851–859. doi:10.1017/s1355617720000429
Boll, S., and Berti, S. (2009). Distraction of Task-Relevant Information Processing by Irrelevant Changes in Auditory, Visual, and Bimodal Stimulus Features: a Behavioral and Event-Related Potential Study. Psychophysiology 46 (3), 645–654. doi:10.1111/j.1469-8986.2009.00803.x
Bouchard, Stéphane., and Rizzo, Albert. Skip. (2019). Virtual Reality for Psychological and Neurocognitive Interventions. New York, NY: Springer.
Brewer, T. L. (1998). “Attentional Impairment in Minor Brain Injury [ProQuest Information & Learning],” in Dissertation Abstracts International: Section B: The Sciences And Engineering, 0599.
Brewer, T. L., Metzger, B. L., and Therrien, B. (2002). Trajectories of Cognitive Recovery Following a Minor Brain Injury. Res. Nurs. Health 25 (4), 269–281. doi:10.1002/nur.10045
Broadbent, D. E., Cooper, P. F., FitzGerald, P., and Parkes, K. R. (1982). The Cognitive Failures Questionnaire (CFQ) and its Correlates. Br. J. Clin. Psychol. 21, 1–16. doi:10.1111/j.2044-8260.1982.tb01421.x
Chaytor, N., and Schmitter-Edgecombe, M. (2003). The Ecological Validity of Neuropsychological Tests: A Review of the Literature on Everyday Cognitive Skills. Neuropsychol. Rev. 13 (4), 181–197. doi:10.1023/B:NERV.0000009483.91468.fb
Clancy, T. A., Rucklidge, J. J., and Owen, D. (2006). Road-crossing Safety in Virtual Reality: A Comparison of Adolescents with and without ADHD. J. Clin. Child Adolesc. Psychol. 35 (2), 203–215. doi:10.1207/s15374424jccp3502_4
Conners, C. K. (1995). Conners Continuous Performance Test Computer Program. Toronto, Ontario: Multi-Health Systems.
Corkum, P. V., and Siegel, L. S. (1993). Is the Continuous Performance Task a Valuable Research Tool for Use with Children with Attention-Deficit-Hyperactivity Disorder?. J. Child. Psychol. Psychiat 34 (7), 1217–1239. doi:10.1111/j.1469-7610.1993.tb01784.x
Couillet, J., Soury, S., Lebornec, G., Asloun, S., Joseph, P.-A., Mazaux, J.-M., et al. (2010). Rehabilitation of Divided Attention after Severe Traumatic Brain Injury: A Randomised Trial. Neuropsychological Rehabil. 20 (3), 321–339. doi:10.1080/09602010903467746
Díaz-Orueta, U., Garcia-López, C., Crespo-Eguílaz, N., Sánchez-Carpintero, R., Climent, G., and Narbona, J. (2014). AULA Virtual Reality Test as an Attention Measure: Convergent Validity with Conners' Continuous Performance Test. Child. Neuropsychol. 20 (3), 328–342. doi:10.1080/09297049.2013.792332
Dockree, P. M., Kelly, S. P., Roche, R. A. P., Hogan, M. J., Reilly, R. B., and Robertson, I. H. (2004). Behavioural and Physiological Impairments of Sustained Attention after Traumatic Brain Injury. Cogn. Brain Res. 20 (3), 403–414. doi:10.1016/j.cogbrainres.2004.03.019
Eliason, M., and Richman, L. (1987). The Continuous Performance Test in Learning Disabled and Nondisabled Children. J. Learn. Disabilities 20 (Dec 87), 614–619. doi:10.1177/002221948702001007
Faria, A. L., Andrade, A., Soares, L., and I Badia, S. B. (2016). Benefits of Virtual Reality Based Cognitive Rehabilitation through Simulated Activities of Daily Living: a Randomized Controlled Trial with Stroke Patients. J. Neuroengineering Rehabil. 13 (1), 96. doi:10.1186/s12984-016-0204-z
Forster, S., and Lavie, N. (2007). High Perceptual Load Makes Everybody Equal. Psychol. Sci. 18 (5), 377–381. doi:10.1111/j.1467-9280.2007.01908.x
Franzen, M. D., and Wilhelm, K. L. (1996). “Conceptual Foundations of Ecological Validity in Neuropsychological Assessment,” in Ecological Validity of Neuropsychological Testing. Editors R. J. Sbordone, and C. J. Long (Boca Raton, FL: St Lucie Press), 91–112.
Gelbar, N. W., Smith, I., and Reichow, B. (2014). Systematic Review of Articles Describing Experience and Supports of Individuals with Autism Enrolled in College and University Programs. J. Autism Dev. Disord. 44 (10), 2593–2601. doi:10.1007/s10803-014-2135-5
Harrington, D. L., Shen, Q., Vincent Filoteo, J., Litvan, I., Huang, M., Castillo, G. N., et al. (2019). Abnormal Distraction and Load‐specific Connectivity during Working Memory in Cognitively normal Parkinson's Disease. Hum. Brain Mapp. 41 (5), 1195–1211. doi:10.1002/hbm.24868
Hartnedy, S., and Mozzoni, M. P. (2000). Managing Environmental Stimulation during Meal Time: Eating Problems in Children with Traumatic Brain Injury. Behav. Interventions 15 (3), 261–268. doi:10.1002/1099-078X(200007/09)15:3<261::AID-BIN60>3.0.CO;2-N
Hughes, R. W., and Jones, D. M. (2003). Indispensable Benefits and Unavoidable Costs of Unattended Sound for Cognitive Functioning. Noise Health 6, 63–76. https://www.noiseandhealth.org/text.asp?2003/6/21/63/31681
Hughes, R. W. (2014). Auditory Distraction: A Duplex-Mechanism Account. PsyCh J. 3 (1), 30–41. doi:10.1002/pchj.44
Jones, D. M., and Morris, N. (1992). “Irrelevant Speech and Cognition,” in Handbook of Human Performance, Vol. 1: The Physical Environment; Vol. 2: Health and Performance; Vol. 3: State and Trait. Editors A. P. Smith, and D. M. Jones (San Diego, CA: Academic Press), 29–53. doi:10.1016/b978-0-12-650351-7.50008-1
Kahneman, D. (1973). Attention and Effort. Englewood Cliffs, N.J.Englewood Cliffs, N.J.: Prentice-Hall.
Kennedy, R. S., Fowlkes, J. E., Berbaum, K. S., and Lilienthal, M. G. (1992). Use of a Motion Sickness History Questionnaire for Prediction of Simulator Sickness. Aviation, Space Environ. Med. 63, 588–593.
Kibby, M., Schmitter-Edgecombe, M., and Long, C. J. (1998). Ecological Validity of Neuropsychological Tests Focus on the California Verbal Learning Test and the Wisconsin Card Sorting Test. Arch. Clin. Neuropsychol. 13 (6), 523–534. doi:10.1016/S0887-6177(97)00038-3
Knight, R. G., Titov, N., and Crawford, M. (2006). The Effects of Distraction on Prospective Remembering Following Traumatic Brain Injury Assessed in a Simulated Naturalistic Environment. J. Int. Neuropsychol. Soc. 12 (1), 8–16. doi:10.1017/s1355617706060048
Kratz, A. L., Whibley, D., Kim, S., Sliwinski, M., Clauw, D., Williams, D. A., et al. (2019). Fibrofog in Daily Life: An Examination of Ambulatory Subjective and Objective Cognitive Function in Fibromyalgia. Arthritis Care Res. 72, 1669–1677. doi:10.1002/acr.24089
Kratz, A. L., Whibley, D., Kim, S., Williams, D. A., Clauw, D. J., and Sliwinski, M. (2020). The Role of Environmental Distractions in the Experience of Fibrofog in Real‐World Settings. ACR Open Rheuma 2 (4), 214–221. doi:10.1002/acr2.11130
Krawczyk, D. C., Morrison, R. G., Viskontas, I., Holyoak, K. J., Chow, T. W., Mendez, M. F., et al. (2008). Distraction during Relational Reasoning: The Role of Prefrontal Cortex in Interference Control. Neuropsychologia 46 (7), 2020–2032. doi:10.1016/j.neuropsychologia.2008.02.001
Krch, D., Nikelshpur, O., Lavrador, S., Chiaravalloti, N. D., Koenig, S., and Rizzo, A. (2013). “Pilot Results from a Virtual Reality Executive Function Task,” in 2013 International Conference on Virtual Rehabilitation (ICVR), Philadelphia, PA, USA, 26-29 Aug. 2013, (IEEE), 15–21. doi:10.1109/ICVR.2013.6662092
Lalonde, G., Henry, M., Drouin-Germain, A., Nolin, P., and Beauchamp, M. H. (2013). Assessment of Executive Function in Adolescence: A Comparison of Traditional and Virtual Reality Tools. J. Neurosci. Methods 219 (1), 76–82. doi:10.1016/j.jneumeth.2013.07.005
Lengenfelder, J., Schultheis, M. T., Al-Shihabi, T., Mourant, R., and Deluca, J. (2002). Divided Attention and Driving. J. Head Trauma Rehabil. 17 (1), 26–37. doi:10.1097/00001199-200202000-00005
Leung, J. P., Leung, P. W. L., and Tang, C. S. K. (2000). A Vigilance Study of ADHD and Control Children: Event Rate and Extra Task Stimulation. J. Dev. Phys. Disabilities 12, 187–201. doi:10.1002/9780470699034
Levin, H. S., and Hanten, G. (2005). Executive Functions after Traumatic Brain Injury in Children. Pediatr. Neurol. 33 (2), 79–93. Retrieved from http://ezproxy.uky.edu/login?url=http://search.ebscohost.com/login.aspx?direct=true&db=cmedm&AN=15876523&site=ehost-live&scope=site. doi:10.1016/j.pediatrneurol.2005.02.002
Levin, H. S., Hanten, G., Roberson, G., Li, X., Ewing-Cobbs, L., Dennis, M., et al. (2008). Prediction of Cognitive Sequelae Based on Abnormal Computed Tomography Findings in Children Following Mild Traumatic Brain Injury. Ped 1 (6), 461–470. Retrieved from http://ezproxy.uky.edu/login?url=http://search.ebscohost.com/login.aspx?direct=true&db=c8h&AN=105468394&site=ehost-live&scope=site. doi:10.3171/ped/2008/1/6/461
Levin, H. S., Hanten, G., Zhang, L., Swank, P. R., Ewing-Cobbs, L., Dennis, M., et al. (2004). Changes in Working Memory after Traumatic Brain Injury in Children. Neuropsychology 18 (2), 240–247. doi:10.1037/0894-4105.18.2.240
Lutz, R. L. (1999). Assessment of Attention in Adults Following Traumatic Brain Injury. Vocational Eval. Work Adjustment J. 32 (1), 47–53. doi:10.1111/1467-9361.00050 Retrieved from http://ezproxy.uky.edu/login?url=http://search.ebscohost.com/login.aspx?direct=true&db=psyh&AN=2001-00772-004&site=ehost-live&scope=site.
Marcotte, T. D., and Grant, I. (2010). Neuropsychology of Everyday Functioning. New York, NY: The Guilford Press.
Marcotte, T. D., Scott, J., Kamat, R., and Heaton, R. K. (2010). “Neuropsychology and the Prediction of Everyday Functioning,” in Neuropsychology of Everyday Functioning, 5–38.
McDermid, A. J., Rollman, G. B., and McCain, G. A. (1996). Generalized Hypervigilance in Fibromyalgia: Evidence of Perceptual Amplification. Pain 66 (2-3), 133–144. doi:10.1016/0304-3959(96)03059-x
Mioshi, E., Dawson, K., Mitchell, J., Arnold, R., and Hodges, J. R. (2006). The Addenbrooke's Cognitive Examination Revised (ACE-R): a Brief Cognitive Test Battery for Dementia Screening. Int. J. Geriat. Psychiatry 21 (11), 1078–1085. doi:10.1002/gps.1610
Moore, E. L., Terryberry-Spohr, L., and Hope, D. A. (2009). Mild Traumatic Brain Injury and Anxiety Sequelae: A Review of the Literature. Brain Inj. 20 (2), 117–132. doi:10.1080/02699050500443558
Moser, J. S., Moran, T. P., Schroder, H. S., Donnellan, M. B., and Yeung, N. (2013). On the Relationship between Anxiety and Error Monitoring: a Meta-Analysis and Conceptual Framework. Front. Hum. Neurosci. 7, 466. doi:10.3389/fnhum.2013.00466
Mozgai, S., Hartholt, A., and Rizzo, A. S. (2019). Systematic Representative Design and Clinical Virtual Reality. Psychol. Inq. 30 (4), 231–245. doi:10.1080/1047840X.2019.1693873
Neisser, U. (1978). “Memory: What Are the Important Questions?,” in Practical Aspects of Memory. Editors M. M. Gruneberg, P. E. Morris, and R. N. Sykes (London: Academic Press), 3–24.
Newcorn, J. H., Halperin, J. M., Jensen, P. S., Abikoff, H. B., Arnold, L. E., Cantwell, D. P., et al. (2001). Symptom Profiles in Children with ADHD: Effects of Comorbidity and Gender. J. Am. Acad. Child Adolesc. Psychiatry 40 (2), 137–146. doi:10.1097/00004583-200102000-00008
Nolin, P., Martin, C., and Bouchard, S. (2009). Assessment of Inhibition Deficits with the Virtual Classroom in Children with Traumatic Brain Injury: A Pilot-Study. Annu. Rev. CyberTherapy Telemed. 7, 240–242. doi:10.3233/978-1-60750-017-9-240 Retrieved from http://ezproxy.uky.edu/login?url=http://search.ebscohost.com/login.aspx?direct=true&db=psyh&AN=2009-17266-055&site=ehost-live&scope=site.
Ouellet, É., Boller, B., Corriveau-Lecavalier, N., Cloutier, S., and Belleville, S. (2018). The Virtual Shop: A New Immersive Virtual Reality Environment and Scenario for the Assessment of Everyday Memory. J. Neurosci. Methods 303, 126–135. doi:10.1016/j.jneumeth.2018.03.010
Park, P., Yamakado, H., Takahashi, R., Dote, S., Ubukata, S., Murai, T., et al. (2020). Reduced Enhancement of Memory for Faces Encoded by Semantic and Socioemotional Processes in Patients with Parkinson's Disease. J. Int. Neuropsychol. Soc. 26 (4), 418–429. doi:10.1017/s1355617719001280
Parsons, T. D., Bowerly, T., Buckwalter, J. G., and Rizzo, A. A. (2007). A Controlled Clinical Comparison of Attention Performance in Children with ADHD in a Virtual Reality Classroom Compared to Standard Neuropsychological Methods. Child. Neuropsychol. 13 (4), 363–381. doi:10.1080/13825580600943473
Parsons, T. D., and Carlew, A. R. (2016). Bimodal Virtual Reality Stroop for Assessing Distractor Inhibition in Autism Spectrum Disorders. J. Autism Dev. Disord. 46 (4), 1255–1267. doi:10.1007/s10803-015-2663-7
Pillay, Y., and Bhat, C. S. (2012). Facilitating Support for Students with Asperger's Syndrome. J. Coll. Student Psychotherapy 26 (2), 140–154. doi:10.1080/87568225.2012.659161
Pollak, Y., Kroyzer, N., Yakir, A., and Friedler, M. (2009). Testing Possible Mechanisms of Deficient Supra-second Time Estimation in Adults with Attention-Deficit/hyperactivity Disorder. Neuropsychology 23 (5), 679–686. doi:10.1037/a0016281
Potvin, M.-J., Rouleau, I., Audy, J., Charbonneau, S., and Giguère, J.-F. (2011). Ecological Prospective Memory Assessment in Patients with Traumatic Brain Injury. Brain Inj. 25 (2), 192–205. doi:10.3109/02699052.2010.541896
Pretus, C., Picado, M., Ramos-Quiroga, A., Carmona, S., Richarte, V., Fauquet, J., et al. (2020). Presence of Distractor Improves Time Estimation Performance in an Adult ADHD Sample. J. Atten Disord. 24 (11), 1530–1537. doi:10.1177/1087054716648776
Reitan, R. M. (1958). Validity of the Trail Making Test as an Indicator of Organic Brain Damage. Percept Mot. Skills 8 (3), 271–276. doi:10.2466/pms.1958.8.3.271
Remington, A., Hanley, M., O’Brien, S., Riby, D. M., and Swettenham, J. (2019). Implications of Capacity in the Classroom: Simplifying Tasks for Autistic Children May Not Be the Answer. Res. Dev. Disabilities 85, 197–204. doi:10.1016/j.ridd.2018.12.006
Rizzo, A. A., Buckwalter, J. G., Bowerly, T., Van Der Zaag, C., Humphrey, L., Neumann, U., et al. (2000). The Virtual Classroom: A Virtual Reality Environment for the Assessment and Rehabilitation of Attention Deficits. CyberPsychology Behav. 3 (3), 483–499. doi:10.1089/10949310050078940
Rizzo, A. A., Buckwalter, J. G., and Neumann, U. (1997). Virtual Reality and Cognitive Rehabilitation: A Brief Review of the Future. J. Head Trauma Rehabil. 12 (6), 1–15. doi:10.1097/00001199-199712000-00002
Robertson, I. H., Ward, T., Ridgeway, V., and Nimmo-Smith, I. (1994). The Test of Everyday Attention. Bury St. Edmunds, England: Thames Valley Test Company.
Rose, F. D., Brooks, B. M., and Rizzo, A. A. (2005). Virtual Reality in Brain Damage Rehabilitation: Review. CyberPsychology Behav. 8 (3), 241–262. doi:10.1089/cpb.2005.8.241
Ryan, J. J., and Lopez, S. J. (2001). “Wechsler Adult Intelligence Scale-III,” in Understanding Psychological Assessment. Perspectives on Individual Differences. Editors W. I. Dorfman, and M. Hersen (Boston, MA: Springer), 19–42. doi:10.1007/978-1-4615-1185-4_2
Salame, P., and Baddeley, A. (1982). Disruption of Short-Term Memory by Unattended Speech: Implications for the Structure of Working Memory. J. Verbal Learn. Verbal Behav. 21, 150–164. doi:10.1016/S0022-5371(82)90521-7
Saredakis, D., Szpak, A., Birckhead, B., Keage, H. A. D., Rizzo, A., and Loetscher, T. (2020). Factors Associated with Virtual Reality Sickness in Head-Mounted Displays: A Systematic Review and Meta-Analysis. Front. Hum. Neurosci. 14, 96. doi:10.3389/fnhum.2020.00096
Sbordone, R. (1996). “Hazards of Blind Analysis of Neuropsychological Test Data in Assessing Cognitive Disability: the Role of Confounding Factors,” in Ecological Validity of Neuropsychological Testing. Editors R. J. Sbordone, and C. J. Long (Delray Beach, FL: Gr Press/St Lucie Press, Inc), 15–26. doi:10.1016/1053-8135(96)00172-2
Schnabel, R., and Kydd, R. (2012). Neuropsychological Assessment of Distractibility in Mild Traumatic Brain Injury and Depression. The Clin. Neuropsychologist 26 (5), 769–789. doi:10.1080/13854046.2012.693541
Schultheis, M. T., Himelstein, J., and Rizzo, A. A. (2002). Virtual Reality and Neuropsychology. J. Head Trauma Rehabil. 17 (5), 378–394. doi:10.1097/00001199-200210000-00002
Sciberras, E., Lycett, K., Efron, D., Mensah, F., Gerner, B., and Hiscock, H. (2014). Anxiety in Children with Attention-Deficit/hyperactivity Disorder. Pediatrics 133 (5), 801–808. doi:10.1542/peds.2013-3686
Sergeant, J. A. (2005). Modeling Attention-Deficit/hyperactivity Disorder: A Critical Appraisal of the Cognitive-Energetic Model. Biol. Psychiatry 57, 1248–1255. doi:10.1016/j.biopsych.2004.09.010
Sergeant, J. A., Oosterlaan, J., and van der Meere, J. (1999). “Information Processing and Energetic Factors in Attention Deficit/hyperactivity Disorder,” in Handbook of Disruptive Behavior Disorders. Editors H. C. Quay, and A. E. Hogan (New York, NY: Kluwer Academic).
Silverberg, N. D., Hanks, R. A., and McKay, C. (2007). Cognitive Estimation in Traumatic Brain Injury. J. Inter. Neuropsych. Soc. 13 (5), 898–902. doi:10.1017/S1355617707071135
Simmeborn Fleischer, A. (2012). Alienation and Struggle: Everyday Student-Life of Three Male Students with Asperger Syndrome. Scand. J. Disabil. Res. 14 (2), 177–194. doi:10.1080/15017419.2011.558236
Spooner, D., and Pachana, N. (2006). Ecological Validity in Neuropsychological Assessment: A Case for Greater Consideration in Research with Neurologically Intact Populations. Arch. Clin. Neuropsychol. 21, 327–337. doi:10.1016/j.acn.2006.04.004
Stroop, J. R. (1935). Studies of Interference in Serial Verbal Reactions. J. Exp. Psychol. 18, 643–662. doi:10.1037/h0054651
Sugishita&Asada, M. T. (2009). About Making of a Depressed Linear Measure Reduction Edition for Senior Citizens – a Japanese Edition (Geriatric Depression Scale-Short Version- Japanese, GDS-S-J). Jpn. J. Cogn. Neurosci. 11 (1), 87–90. doi:10.11253/ninchishinkeikagaku.11.87
Tannock, R., Ickowicz, A., and Schachar, R. (1995). Differential Effects of Methylphenidate on Working Memory in ADHD Children with and without Comorbid Anxiety. J. Am. Acad. Child Adolesc. Psychiatry 34 (7), 886–896. doi:10.1097/00004583-199507000-00012
Tricco, A. C., Lillie, E., Zarin, W., O’Brien, K., Colquhoun, H., Kastner, M., et al. (2016). A Scoping Review on the Conduct and Reporting of Scoping Reviews. BMC Med. Res. Methodol. 16 (15), 15. doi:10.1186/s12874-016-0116-4
Trudel, T. M., Tryon, W. W., and Purdum, C. M. (1998). Awareness of Disability and Long-Term Outcome after Traumatic Brain Injury. Rehabil. Psychol. 43 (4), 267–281. doi:10.1037/0090-5550.43.4.267
Uitvlugt, M. G., Pleskac, T. J., and Ravizza, S. M. (2016). The Nature of Working Memory Gating in Parkinson's Disease: A Multi-Domain Signal Detection Examination. Cogn. Affect Behav. Neurosci. 16 (2), 289–301. doi:10.3758/s13415-015-0389-9
Van Der Linden, W. J. (2008). Some New Developments in Adaptive Testing Technology. Z. für Psychol./J. Psychol. 216 (1), 3–11. doi:10.1027/0044-3409.216.1.3
van Mourik, R., Oosterlaan, J., Heslenfeld, D. J., Konig, C. E., and Sergeant, J. A. (2007). When Distraction Is Not Distracting: A Behavioral and ERP Study on Distraction in ADHD. Clin. Neurophysiol. 118 (8), 1855–1865. doi:10.1016/j.clinph.2007.05.007
Wechsler, D. (2008). Wechsler Adult Intelligence Scale, Fourth Edition (WAIS-IV) [Database Record]. San Antonia, TX: Pearson. doi:10.1037/t15169-000
Wilson, B. A., Alderman, N., Burgess, P. W., Emslie, H., and Evans, J. J. (1996). Behavioral Assessment of the Dysexecutive Syndrome. Test Manual. Edmunds England: Thames Valley Test Company.
Wilson, B. A., Cockburn, J. M., and Baddeley, A. D. (1985). The Rivermead Behavioral Memory Test. Reading, England/Gaylord, MI: Thames Valley Test Company/National Rehabilitation Services.
Wilson, B. A., Shiel, A., Foley, J., Emslie, H., Groot, Y., Hawkins, K., et al. (2004). Cambridge Test of Prospective Memory. Bury St. Edmunds, England: Thames Valley Test Company.
Wood, R., and Liossi, C. (2006). The Ecological Validity of Executive Tests in a Severely Brain Injured Sample. Arch. Clin. Neuropsychol. 21 (5), 429–437. doi:10.1016/j.acn.2005.06.014
Yeh, S.-C., Lin, S.-Y., Wu, E. H.-K., Zhang, K.-F., Xiu, X., Rizzo, A., et al. (2020). A Virtual-Reality System Integrated with Neuro-Behavior Sensing for Attention-Deficit/Hyperactivity Disorder Intelligent Assessment. IEEE Trans. Neural Syst. Rehabil. Eng. 28 (9), 1899–1907. doi:10.1109/TNSRE.2020.3004545
Yesavage, J. A., and Sheikh, J. I. (1986). 9/geriatric Depression Scale (GDS). Clin. Gerontologist 5 (1–2), 165–173. doi:10.1300/J018v05n01_09
Zentall, S. S., and Meyer, M. J. (1987). Self-regulation of Stimulation for ADD-H Children during reading and Vigilance Task Performance. J. Abnorm Child. Psychol. 15, 519–536. doi:10.1007/bf00917238
Keywords: attention, memory, scoping review, distraction, computer assisted
Citation: Pinnow D, Hubbard HI and Meulenbroek PA (2021) Computer- Assessment of Attention and Memory Utilizing Ecologically Valid Distractions: A Scoping Review. Front. Virtual Real. 2:685921. doi: 10.3389/frvir.2021.685921
Received: 26 March 2021; Accepted: 30 June 2021;
Published: 14 July 2021.
Edited by:
Andrea Stevenson Won, Cornell University, United StatesReviewed by:
Michael J. Roy, F. Edward Hebert School of Medicine, United StatesHongchuan Yu, Bournemouth University, United Kingdom
Copyright © 2021 Pinnow, Hubbard and Meulenbroek. This is an open-access article distributed under the terms of the Creative Commons Attribution License (CC BY). The use, distribution or reproduction in other forums is permitted, provided the original author(s) and the copyright owner(s) are credited and that the original publication in this journal is cited, in accordance with accepted academic practice. No use, distribution or reproduction is permitted which does not comply with these terms.
*Correspondence: DeAnna Pinnow, deannapinnow@uky.edu