- 1SMART Infrastructure Facility, University of Wollongong, Wollongong, NSW, Australia
- 2Department of Computer Science and Information Technology, La Trobe University, Melbourne, VIC, Australia
- 3School of Psychology, University of Wollongong, Wollongong, NSW, Australia
- 4Coal Services Pty Ltd., Lake Macquarie, NSW, Australia
This paper discusses results from two successive rounds of virtual mines rescue training. The first round was conducted in a surround projection environment (360-VR), and the second round was conducted in desktop virtual reality (Desktop-VR). In the 360-VR condition, trainees participated as groups, making collective decisions. In the Desktop-VR condition, trainees could control their avatars individually. Overall, 372 participants took part in this study, including 284 mines rescuers who took part in 360-VR, and 243 in Desktop-VR. (155 rescuers experienced both.) Each rescuer who trained in 360-VR completed a battery of pre- and post-training questionnaires. Those who attended the Desktop-VR session only completed the post-training questionnaire. We performed principal components analysis on the questionnaire data, followed by a multiple regression analysis, the results of which suggest that the chief factor contributing to positive learning outcome was Learning Context, which extracted information about the quality of the learning content, the trainers, and their feedback. Subjective feedback from the Desktop-VR participants indicated that they preferred Desktop-VR to 360-VR for this training activity, which highlights the importance of choosing an appropriate platform for training applications, and links back to the importance of Learning Context. Overall, we conclude the following: 1) it is possible to train effectively using a variety of technologies but technology that is well-suited to the training task is more useful than technology that is “more advanced,” and 2) factors that have always been important in training, such as the quality of human trainers, remain critical for virtual reality training.
1 Introduction
Virtual reality (VR) has the potential to be a powerful tool for vocational training. This is especially true for so-called safety-critical industries, such as mining, aviation, and medicine [Blickensderfer et al. (2005); Tichon and Burgess-Limerick (2011); Graafland et al. (2012)]. In these industries, mistakes can result in injury or death, and traditional methods of training may themselves put trainees in danger. VR training can replicate many of the physical and psychological stimuli associated with the real-world training domain, while still offering the trainee a safe space in which to learn. That said, there remain many open questions regarding VR vocational training, including: Do some trainees benefit more than others from VR training, and, if so, why? What is the role of traditional trainers in such training? Which training activities are best suited to delivery in VR? Which VR technologies are best suited for their delivery?
In this article, we investigate these questions in the domain of mining rescue operations. We conducted and observed mines rescue training activities in both immersive and non-immersive contexts. Our results provide tentative answers to some of these questions. In particular, we did not observe any characteristics of trainees that led them to train differently in VR, but we did find that traditional trainers still have a very significant role to play in VR training. We also found that the immersiveness of the technology may matter less than whether that technology is well-suited to the training task at hand.
2 Previous Work
VR environments have been described as either immersive (e.g., 360-VR (Figure 1), Head Mounted Display) or non-immersive (e.g., Desktop) [Ai-Lim Lee et al. (2010)]. The immersion that VR generates can also be either active or passive [Nakatsu and Tosa (2000)]. In this second classification the key criteria is the lack or existence of interaction features; active immersion includes interacting with the environment and objects in it, whereas passive immersion provides no interaction. Desktop-VR, also known as “non-immersive VR” [Merchant et al. (2014)], enables a user to interact with 3-D simulations that are generated on a personal computer through keyboard, mouse or joystick, touch screen, headphones, shutter glasses, and data gloves [Gazit et al. (2006)]. Desktop-VR provides an alternative to immersive VR; despite being non-immersive, it can still be productively used for learning [Merchant et al. (2014); Merchant et al. (2012)].
There is growing interest in the assessment of training technologies and the prediction of learning outcomes. One objective measure is how much an operator’s performance in a real-world task is improved by their training using the technology [Tichon and Burgess-Limerick (2011)]. However, unlike operational training, where worker performance can be measured while they are engaged in their everyday work, the effectiveness of safety training can only be measured when accidents occur. Training for disaster response and rescue operations—where the situation being trained for may be extremely unpredictable—is also different to that for high stress environments where event progression can be more easily modeled, such as aviation [Barbosa Mendes et al. (2010); Chittaro et al. (2018)]. A commonly used evaluation technique is to assess the pre- and post-training change in the trainees’ rescue skills and knowledge following the training session [14]. Another evaluation approach is to analyze the number of incidents/accidents that occurred before and after the rescue training [Kowalski-Trakofler and Barrett (2003)]. However, the outcomes of such longitudinal studies are often influenced by hidden and independent factors. Thus, it is difficult to precisely determine how much of the worker/responder’s improvement is due to the actual training, as there are typically many other factors which could have impacted the rate of incidents [Tichon and Burgess-Limerick (2011)]. It is clear that different techniques are required for obtaining objective measures of trainee performance. It might not always be possible to measure the training outcome directly and therefore the focus might need to be on the training tools and processes instead.
A number of different frameworks have been proposed for evaluating the trainee’s learning in computer mediated environments. For example, Piccoli et al.’s framework focuses exclusively on the characteristics of the trainee, ignoring the role of learning processes that mediate the relationships between instructional design/technology dimensions and learning outcomes [Piccoli et al. (2001)]. In that study, they concluded that individuals who are comfortable with technology, have previous experience with VR, and have positive attitudes toward the technology, should thrive during computer mediated learning—due to low levels of anxiety and likely excitement. Hiltz reported that trainees who are mature and motivated learners appear to have better learning experiences and outcomes in VR than those who are less motivated and mature, who instead tend to suffer [Hiltz (1994)]. Pekrun also argued that enjoyment can result in higher motivation and a greater focus on the learning material [Pekrun (2006)]. Lee et al. found that the learners’ motivation, cognitive benefits, control, active learning, and reflective thinking can all impact their learning processes in VR learning environments [Ai-Lim Lee et al. (2010)]. It has also been found that self-efficacy, beliefs about the nature of learning, and the structure of knowledge, also influence a trainee’s ability to learn effectively in VR [Makransky and Lilleholt (2018); Makransky and Petersen (2019)]. These factors have been proposed as trainee factors which are important for successful training in VR learning environments.
In technology-mediated learning, trainees also interact with each other, and with other objects and features of their environment, as well as with the trainer. These interactions are often mediated or enabled by the technology. Researchers have suggested that the features of VR—such as its representational fidelity (realism), degree of immersion, the sense of presence (or “being there”) that it can generate, the immediacy of control, as well as the technology’s perceived usefulness and ease of use—can all significantly impact learning outcomes [Alavi and Leidner (2001); Benbunan-Fich and Hiltz (2003); Wan and Fang (2006); Ai-Lim Lee et al. (2010)]. Presence in VR also appears to be influenced by the trainee’s level of enjoyment [Sylaiou et al. (2010)] as well as their personality [Alsina-Jurnet and Gutiérrez-Maldonado (2010)]. Co-presence (the perceived sense of being there together) and social presence (the capability of the medium to create a sociable environment where users can interact with others and be with them) can positively affect learning experiences and outcomes [Pedram et al. (2020)]. A number of studies have shown that social presence is an important antecedent of learner satisfaction in technology mediated learning environments [Hostetter and Busch (2006); Cobb (2009)] and a significant factor that affects satisfaction and enjoyment in VR environments [Mansour et al. (2010); Zhang (2010)]. Makransky and Lilleholt also highlighted the importance of immersion in both the experience and outcomes of training in VR [Makransky and Lilleholt (2018)]. In a recent study, Grabowski and Jankowski found that reported training experiences and outcomes were better with a highly (as opposed to a moderately) immersive VR training session [Grabowski and Jankowski (2015)].
The use of VR-based training environments assumes that human-machine interaction stimulates learning processes leading to a more effective transfer of the learning outcomes into workplace environments [Chen et al. (2009)]. As stated by Meadows: “When I hear, I forget; when I see, I remember; when I do, I understand” [Meadows (2001)]. Fulton and colleagues argue that interactive models like flight simulators are designed to improve the trainee’s understanding of the consequences of decisional queues under limited resource availability (material, time, or energy) and uncertain or hazardous conditions (unintended consequences) [Fulton et al. (2011)]. Seymour and colleagues argue that the more realistic the experience is, the better the learning [Seymour et al. (2002)]. In situations where real life training opportunities are limited, hazardous, or impossible (like emergency responding), virtual reality simulators offer the opportunity to emulate many wide-ranging experiments and enable immediate feedback and broad accessibility in a safe environment [Seymour et al. (2002); Lovreglio et al. (2017); Smith and Ericson (2009); van Ginkel et al. (2019)].
Building on previously established frameworks, Pedram et al. recently developed a comprehensive causality framework, to measure the relationship between various factors. They reported that features of the VR technology (realism and co-presence), of the learning experience enabled by the VR scenario (flow, immersion, presence, co-presence and not feeling distress), of the usability of the VR system (usefulness and ease of use), and of the involvement of an expert trainer and their feedback, had direct and indirect impacts on learning outcomes [Pedram et al. (2020)].
Research has also shown that characteristics of the trainer, such as their availability and their level of engagement, technology experience, self-efficacy and the feedback that they provide, are important for technology-mediated learning [Piccoli et al. (2001); Benbunan-Fich and Hiltz (2003)]. Trainers can be seen as facilitators who help trainees to shape their learning experiences and promote their learning [Ornstein and Hunkins (1988)]. This is consistent with the notions of social constructivism and collective learning, which emphasize the important roles that teachers, peers and other community members can play in assisting in the trainee’s learning. Research suggests that the feedback the trainer provides during the learning is particularly important. During the process of learning, feedback can help the trainee select the relevant information, organize this information into a coherent mental representation, and then integrate this information with existing knowledge stored in long-term memory [Makransky and Petersen (2019)]. It has been reported that reliable feedback can motivate and encourage student learning, as well as increasing this learning [Stiggins et al. (2004)]. Feedback can either be: corrective, where the trainee will only be informed whether he/she was right or wrong; or explanatory, where an explanation is given about why he/she was right or wrong. Moreno and colleagues found that trainees who received explanatory feedback performed better in solving complex problems compared to another group who only received the corrective feedback [Moreno and Mayer (2002)]. Similarly, Johnson and Priest found that novice students learn better with explanatory feedback than with corrective feedback alone [Johnson and Priest (2014)]. Researchers have also investigated the importance of providing feedback in VR learning environments. For instance, Butler and Roediger reported that providing feedback helped students to perform better in a subsequent test [Butler and Roediger (2008)]. In a follow-up study, they found that feedback approximately doubled student performance in comparison with testing without feedback [Butler and Roediger (2008)]. Moreover, Kruglikova et al. conducted an experiment using VR for colonoscopy training and reported that the group which received feedback performed significantly better than the control group [Kruglikova et al. (2010)]. Additionally, Strandbygaard et al. reported that instructor feedback improves learning of surgery skills in a VR simulator [Strandbygaard et al. (2013)]. In a meta-analysis, Merchant et al. reported that unfortunately, few studies in games and virtual worlds provided information on the feedback provided during the VR based instruction [Merchant et al. (2014)]. Therefore, this could be an interesting area to explore for deeper insight into the design of virtual reality applications.
3 Materials and Methods
This paper discusses results from a study of two successive rounds of virtual mines rescue training. The first round was conducted in a surround projection environment (360-VR), and the second round was conducted in desktop virtual reality (Desktop-VR). This study was approved by the Social Sciences Human Research Ethics Committee of the University of Wollongong.
3.1 Technology-in-use
This research focused on a training program developed for mines rescue brigades. The training scenario was offered in two different VR platforms, 360-VR and Desktop-VR. Based on a definition provided by Moreno and Mayer [Moreno and Mayer (2002)], 360-VR is classified as a high immersion environment which consists of a 10 m diameter, 4 m high cylindrical screen (Figure 2). The large area within the environment enables a mixed reality experience with small groups of 5–7 trainees. In this environment, trainees can interact with props (e.g., virtual gas detectors) and each other in order to facilitate realistic responses, activities, and reflexes as part of the training experience.
We also investigated training using a non-immersive platform (Desktop-VR). In the Desktop-VR condition, groups of 6–7 participants were seated in a same room and experienced the scenario on individual laptop PCs which were equipped with gamepads for navigation and noise canceling headphones for communication with each other, in the virtual environment (See Figure 3). Prior to training, a guide sheet on how to use the gamepad was given to each participant.
3.2 Scenario
Training occurred in a unique whole-of-mine VR environment, which modeled 50 km of roadway and covered all regular underground mining activities. The training scenario used in the study was developed by Mines Rescue Pty Ltd.1 using Unity3D2, a multi-platform game engine.
In the specific scenario used in these training exercises, an accident involving an underground vehicle starts a fire at the bottom of the transport drift. The fire is uncontained and spreads to the coal, contaminating several galleries and roadways with toxic gases. The incident occurs during a night shift at 3:06 am on a Sunday. At the time of the incident seven workers are underground and three others are on the surface. Visibility in the galleries is reduced to approximately 50 m. The trainees are informed that one of the miners is missing and the others are safe. The task assigned to the trainees—all experienced mines rescue brigadesmen—is to undertake search and rescue for the missing miner. The trainer (Figure 4) guides the trainees (Figure 5) through each stage of this scenario, prompting them for the appropriate actions and responses.
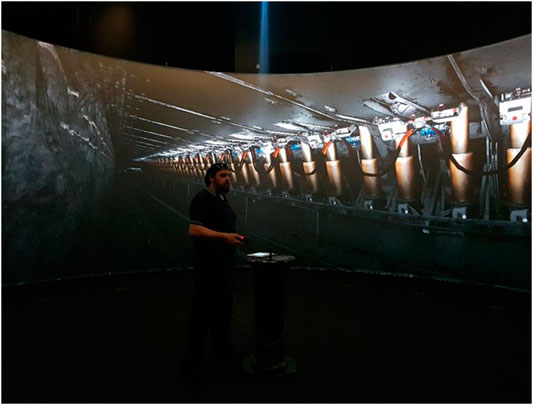
FIGURE 4. The trainer in the 360-VR environment, operating Longwall mining machinery in the background.
3.3 Participants
The data reported in this paper was obtained from two rounds of VR mines rescue training. The first round was conducted in 360-VR, and consisted of 45 separate training sessions. The second round was conducted in Desktop-VR, and consisted of 35 separate training sessions.
284 trainees (all male) took part in the 360-VR training sessions. The mean age of this sample was 40.2 years, and on average they had 9 years of experience in the field. Demographic information for this sample appears in Table 1.
243 trainees (again all male) took part in the Desktop-VR training sessions. The mean age of this sample was 38 years, and on average they had 9 years of field experience. Demographic information for this sample appears in Table 2.

TABLE 2. Demographic information for the Desktop-VR trainees (N = 243; all numbers represent years).
There was substantial—but not complete—overlap across these samples. 155 trainees participated in both training rounds, 129 participated only in the 360-VR sessions, and 88 participated only in the Desktop-VR sessions.
3.4 Instruments and Procedures
Before their training in VR, trainees a completed brief competency test (the skill test, Table 3), which assessed their knowledge about search and rescue protocols. The skill test was obtained from Coal Services Pty Ltd. subject matter experts.
For each question, participants received a score of either 1 (correct answer) or 0 (incorrect answer). Total scores therefore ranged from 0 to 4 (Trainees later repeated this skill test one month after their VR training to objectively evaluate the quality of their learning).
Trainees then completed the pre-training questionnaire which was designed to assess their background, previous experience with technology, and state of mind just prior to the VR training session. This measured seven different constructs: Gaming experience, enthusiasm, stress, motivation, competition, self-efficacy, and well-being. Various items were used to measure each construct, with each item being a 10-level Likert scale, where 0 was highly disagree, 10 was highly agree and 5, was neutral. Due to time constraints and the large number of factors we sought to measure, the items for this pre-training questionnaire were taken from the established questionnaires listed in Table 4. All factors returned a Cronbach’s alpha greater than 0.7.
Following the VR training, trainees then completed the post-training questionnaire which assessed their experience in VR and also measured their perceived learning (See Table 5). This questionnaire measured the following constructs: well-being, realism/representational fidelity, immersion, presence, co-presence, social presence, flow, stress/worry, ease of use, usefulness, plausibility, attitude toward use, attitude toward trainer, attitude toward feedback, and perceived learning. Each construct was again measured using various items and each item was a 10-level Likert scale where 0 was highly disagree, 10 was highly agree, and 5 was neutral. Again due to time constraints and the large number of factors we sought to measure, the items for this post-training questionnaire were taken from the established questionnaires listed in Table 5. As with the pre-training factors, all factors returned a Cronbach’s alpha greater than 0.7.
Trainees again responded to each question on a Likert scale of 0–10 where ratings from 0 to 4 indicate less successful, useful, and realistic, etc., ratings of 5 indicate neutrality, and ratings from 6 to 10 indicate progressively more successful, useful, and realistic, etc.
Trainees also completed a four-item subjective feedback questionnaire, as seen in Table 6.
Finally, to measure perceived learning, trainees responded to four questions on a Likert scale of 0–10 where 0
4 Results
Participants in the 360-VR round (N = 284) completed a pre-experience questionnaire, the descriptive results of which appear in Table 8.
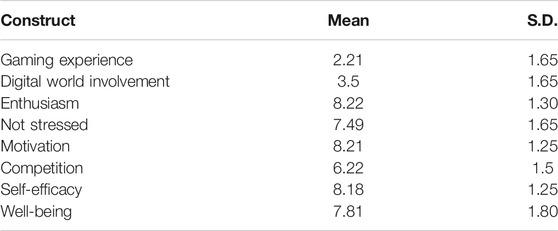
TABLE 8. Results from the pre-experience questionnaire (All constructs have values from 0 (lowest) to 10 (highest).).
Participants in both rounds (N (360-VR) = 284, N (Desktop-VR) = 243) completed a post-experience questionnaire, the descriptive results of which appear grouped by training round in Table 9.
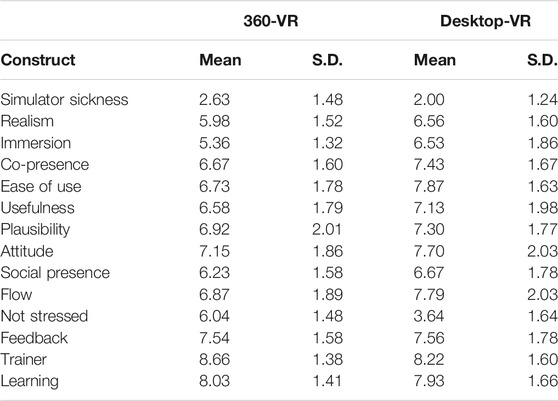
TABLE 9. Results from the post-experience questionnaires (All constructs have values from 0 (lowest) to 10 (highest)).
4.1 Data Analysis
One goal of this research program was to determine whether the trainees’ perceived learning—as measured by the questionnaire in Table 7—could be predicted or explained by any of these pre-training or post-training factors. The relatively small size of the sample (231 observations for 17 predictor variables) led us to implement a two stage modeling process, where stage 1 was a principal component analysis used to reduce the number of predictors, and stage 2 was the construction of a multiple regression model where these aggregated predictors were used to model perceived learning. Principal components analyses (PCA) were performed separately on the pre-training and post-training predictors, using SPSS with default settings.
4.1.1 Principal Component Analysis of Pre-training Factors
The first component of the PCA on the pre-training factors, which explained 34% of the variance, was characterized by five factors: “Enthusiasm,” “Motivation,” “Self-Efficacy,” “Well-being,” and “Competitiveness.” The second component, which explained 17% of the variance, was characterized by two strongly correlated factors: “Worry” and “No stress.” The third component, which explained 13% of the variance, was characterized by two strongly correlated factors: “Gaming Experience” and “Digital World Involvement.” Together these three components explained 64% of the total variance.
Based on the nature of the factors that contributed the most to each component we created the following three aggregate predictor variables: “Positive State of Mind” (Component 1), “Negative State of Mind” (Component 2) and “Technology Experience” (Component 3).
4.1.2 Principal Component Analysis of Post-training Factors
The first component, which explained 56% of the variance, was characterized by eleven correlated variables: “Plausibility,” “Tool functionality,” “Usefulness,” “Ease of use,” “Attitude toward using the technology,” “Presence,” “Social presence,” “Co-presence,” “Flow,” “Immersion,” and “Realism.” The second component, which explained 9% of the variance, was characterized by three strongly correlated variables: “Task characteristics,” “Feedback” and “Trainer.” The third component, which explained 8% of the variance, was characterized by two strongly correlated variables: “No stress” and “Simulation sickness.” Together these three components explained 73% of the total variance.
Based on the nature of the factors that contributed the most to each component we created the following three aggregate variables: “Positive Learning Experience” (Component 1), “Learning Context” (Component 2), and “Negative Learning Experience” (Component 3).
4.1.3 Perceived Learning and Change in Skill Test Score Post-training
In general, the trainees ranked their perceived learning quite highly (M
There is evidence that subjective measures of learning outcomes might not be highly correlated with actual learning outcomes and the researchers are advised to be cautious in their use of subjective assessments of knowledge as indicators of learning [Makransky and Petersen (2019); Sitzmann et al. (2010)]. Sitzmann et al., based on their meta-analysis of 166 studies in the fields of education and workplace training, reported that subjective assessments of knowledge are only moderately related to learning outcomes [Sitzmann et al. (2010)].
In our study we performed scale conversion for “actual learning” in order for the actual score to be out of 10 and then we performed correlation between “perceived” and “actual learning” and as the result of our correlation indicates—
4.1.4 Multiple Regression Analysis
A stepwise linear regression was conducted to examined the relationship between perceived learning (PL) and the following six aggregated variables—three pre-training and three post-training: “Positive state of mind (PSM),” “Negative state of mind (NSM),” “Technology experience (TE),” “Positive learning experience (PLE),” “Negative learning experience (NLE),” and “Learning context (LC).” These variables are the results of the principal components analyses described in sections 4.1.1 and 4.1.2. The stepwise regression was performed on the entire data set, as a method of fitting a model to the data. In this method, at each step a factor is considered for addition to or subtraction from the set of explanatory variables based on its significance. At each step statistically insignificant variables are excluded from the model until all the remaining variables are statistically significant. As the result shows, three out of six aggregate variables were found to be significant predictors of perceived learning in this study. As the result shows, 72% of variance in data set was explained by the final model (Table 10). None of the pre-training aggregated variables—PSM, NSM, or TE—significantly predicted perceived learning, and so were omitted from the final model (Table 11).
These results show that trainees’ individual characteristics as measured on the pre-training questionnaire did not significantly influence the perceived learning of the trainees. However, the context of the training session and the (positive or negative) experiences during the session did significantly impact the perceived learning (Table 12). The final model equation is presented in Eq. 1.
4.1.5 Model Validation
In order to validate the model generated as described in Section 4.1.4, the data set was randomly split into a training sample group (75% of data set) and a validation sample group (25% of data set). We then performed stepwise regression analysis again on these two groups.
Here the multiple regression model explains 70% of the observed variance—as opposed to 72% with the full dataset—and the overall fit between observed and predicted values for Perceived Learning remained statistically significant (See Table 13.). All of the variables which were excluded from the original model (Table 14) were once again excluded in the model based on 75% of the data, and all of the included predictors were still included (See Table 15.). There was no significant decrease in
4.1.6 Subjective Results
In addition to the data discussed previously in this section, all trainees—both 360-VR and Desktop-VR—completed a short subjective feedback questionnaire (see Table 6). Most of the trainees (89%) clearly preferred the Desktop-VR to traditional training, but the same could not be said of the 360-VR as only 49% indicated a preference over traditional training (Table 6, Q1). Although the trainees considered Desktop-VR more useful than the 360-VR, most of the trainees—64% for 360-VR and 75% for Desktop-VR—were agreed to strongly agreed that the VR training is useful (Table 6, Q2). Perhaps surprisingly, the trainees were more likely to recommend the 360-VR as compared to the Desktop-VR. Overall, 89% of the trainees would recommend the 360-VR to others while 81% would do so for Desktop-VR (Table 6, Q3, Figure 6). This may suggest that the trainees enjoyed the immersive experience in the 360-VR more than the non-immersive Desktop-VR training. It may also indicate that while trainees acknowledged the usefulness of VR training platforms, as evidenced by the high level of recommendation, familiarity with traditional training methods may have led them to prefer such methods over VR. Approximately 17% of the trainees were neutral about the success of the 360-VR training. The same is true for the Desktop-VR. This decision about the success of the VR training is bound to occur when the trainees cannot make a clear judgment as to whether the derived benefits of the technology outweigh the observed costs or its practical limitations. However, in both the Desktop-VR and the 360-VR, less than 2.5% of the trainees opposed the idea that the training was successful in helping them achieve their training needs. In other words, most trainees—approximately 80%—were agreed to strongly agreed with the notion that the training was successful in helping them achieve their training needs, regardless of whether it was Desktop-VR or 360-VR (Table 6, Q4).
5 Discussion
5.1 Importance of Learning Context
While Negative Learning Experience, Positive Learning Experience, and Learning Context were all significant predictors of perceived learning, the strongest predictor—as confirmed by the standardized regression coefficients in Table 7—was Learning Context (Standardized regression coefficients indicate the change in the outcome variable in response to a one standard deviation change in the predictor variable). The Learning Context factor had more than twice as much influence on the trainee’s perceived learning as Positive Learning Experience factor (0.653 vs. 0.246) and more than seven times as much as influence as Negative Learning Experience factor (0.653 vs 0.090).
Recall that the Learning Context factor was composed of items relating to characteristics of the training activity itself, the trainees’ perception of their trainer, and the trainees’ perceptions and attitudes relating to their feedback. Here we discuss each of these in turn.
5.1.1 Importance of Learning Context: Trainers
The trainer’s acceptance of the technology, their demonstrable comfort with it, and their ability to use it effectively are essential contributors to effective training. Other researchers such as Martins and Kellermanns [Martins and Kellermanns (2004)] and Wan and Fang [Wan and Fang (2006)] have reported on the importance of trainers or instructors in technology-mediated learning environments. Our research has specifically shown the importance of trainers who are engaged and motivated to use the technology. We observed in this study that there were trainers who were reluctant to use VR as a training tool and their apathy was demotivating to the trainees. This is an important aspect of VR-based training that models developed by Salzman et al. [Salzman et al. (1999)] or Dishaw and Strong [Dishaw and Strong (1999)] have so far overlooked. Whenever trainers are 1) reluctant to use the technology, 2) uncomfortable with its use, or 3) doubtful about its added value, they are likely to transfer their negative attitude to trainees. Several trainees mentioned these consequences in their responses: “[the training] felt rushed,” “trainer rushed us through the scenario, didn’t have time to complete the task,” “trainer not familiar with the program and [I] found it confusing at times,”“willingness to participate is required.” This might be related to the observation reported by Vogel et al. that students performed better when they were in control of their navigation through the virtual learning environment compared to when the teacher controlled the learning environment [Vogel et al. (2006)].
5.1.2 Importance of Learning Context: Feedback
Compared to other training media, VR has a unique ability to provide the combination of realistic stimuli, low risk, and timely feedback. We observed that training sessions in 360-VR were particularly effective in their ability to create opportunities for discussion, decision making, and feedback. A typical pattern is as follows: Trainees observe a given situation and are prompted by the trainer to take action. Once a decision is made to act, the trainees observe the potential consequences of that action. The trainer then engages the group to elicit their immediate reactions, discuss alternate courses of action, and give corrective or explanatory feedback. Unlike onsite or classroom training, the scenario does not have to stop during this discussion phase; the trainer has the ability to “pause” the scenario, or to let it play out and then later return to an earlier state. This strength of VR-based training was mentioned by many trainees and trainers: “going back over an incident to correct yourself,” “trainers could stop or alter [an] exercise easily to facilitate learning and understanding of competencies.” Giving feedback to trainees is shown to be effective for the learning process [Wisniewski et al. (2020)] and for correcting mistakes and misconceptions [Dantas and Kemm (2008); Marcus et al. (2011)].
5.1.3 Importance of Learning Context: Training Activity
Trainees also generally had positive feelings about the usefulness and efficacy of the training session as a whole, which can be seen in their responses to the questions that made up this component. It can also be seen in their responses to the open-ended questions. For instance trainees mentioned the benefit of “seeing possible hazardous conditions without the real life exposure,” and that as a result they could get “some exposure to an incident that could not be simulated down a pit,” and “train in scenarios not encounter in normal mining operation, train for emergency conditions.” Moreover, “you can have an over view of the whole situation and not be in harm, it gives you the chance to stop pause, rewind,” and “cover a lot of hazards in a short period of time,” therefore you can “experience everything without real danger.” From these responses, it is clear that they considered the virtual training useful, especially because of its ability to simulate dangerous situations without putting the trainees in real danger.
5.2 Importance of Positive and Negative Learning Experiences
The second strongest predictor of perceived learning was Positive Learning Experience, which groups Plausibility, Tool Functionality, Usefulness, Ease of use, Attitude toward the technology, Presence, Social Presence, Co-presence, Flow, Immersion, and Realism. The fact that these are all grouped into a single component is interesting, as these are generally treated as separate constructs. Here, they all group together, on a sort of overall “goodness rating” of the virtual environment. This may indicate that our questionnaire generated a similar effect as Slater’s “colorfulness” questionnaire [Slater (2004)], where users who had an overall positive experience made use of the existing instrument as best they could to indicate that feeling.
Open-ended feedback does generally indicate that trainees were satisfied with the quality of the simulation and the training experience: “being able to simulate a real underground fire and change gas level in VR was great,” “very life like situation,” “simulated smoke,” “closest to real thing and can relate,” “getting a sense of real time working,” and “it felt real.” However, not all trainees shared this view: “moving around in VR room is not realistic,” “[it is] not realistic, cannot smell or feel or hear anything,” “reduced ability to orientate, not fully demanding physically or mentally,” and “can seem unrealistic at time.”
Negative learning experience was comprised of Simulator Sickness and Stress. Simulator sickness and feeing distress are important factors to be considered in evaluating the effectiveness of VR-based training [Pedram et al. (2020)]. In this study trainees did not report significant simulator sickness and discomfort and as a result it did not negatively impact their training experience. That being said, trainees who are uncomfortable in the training environment will be distracted from the training and will not be able to concentrate on content, possibly resulting in lower sense of presence and even leading them to withdraw from training and therefore, these factors are crucial to be considered [Pedram et al. (2020)].
5.3 (Lack of) Importance of Individual Characteristics
The pre-exposure surveys administered to trainees were designed to measure temporary and permanent characteristics of individual trainees. These characteristics were hypothesized to impact trainees’ learning. However, none of the aggregated variables derived from the pre-exposure surveys—Positive State of Mind, Negative State of Mind, and Technology Experience—were observed to have a significant effect on trainees’ perceived learning, as can be observed that none of these variables appear in Eq. 1. The literature is mixed regarding the effects of individual characteristics on learning. A meta-analysis conducted by Richardson et al. found significant, but mostly small, correlations between individual characteristics and university students’ academic performance [Richardson et al. (2012)] (Self-efficacy had the strongest correlation in that analysis; we did not observe it to have a significant effect in this study.) The positive effect of self-efficacy on learning was also observed in Makransky and Petersen’s study investigating learning in desktop VR [Makransky and Petersen (2019)]; again, it was not a significant factor in our model. One possible explanation is that both of those results came from a population of university undergraduate students, while the trainees in our study were experienced mining professionals. As such, one might reasonably expect to see less variance in motivation and capability among our sample population. Similarly, one might expect variables related to Negative State of Mind, such as worry and stress, to have little or no effect on a population of experienced professionals, while they might have more impact in a different population.
Regarding Technology Experience, on the other hand, the fact that this variable did not significantly influence our model is well-supported by the literature, including Parnell and Carraher [Parnell and Carraher (2003)] and Ju et al. [Ju et al. (2019)]. In these studies, previous gaming experience was not found to have a significant effect on any of perceived learning, satisfaction, or decision making.
5.4 Importance of Selecting an Appropriate Platform
In this study, participants interacted with a search and rescue training scenario using two different VR platforms: Immersive (360-VR) and non-immersive (Desktop-VR). In addition to the difference in immersion, these platforms also required participants to interact with the system differently: In 360-VR, one user controlled the movement of the entire group, so trainees discussed the issues together, reached a consensus, and then moved together. In Desktop-VR, each use controlled their own movement, so there was no need to reach consensus or act through an intermediary. For this task, which in reality requires users to act individually, Desktop-VR is likely a more suitable platform for the task than 360-VR.
Our results suggest that choosing an appropriate platform for the task is more important to trainees than improving immersion. Questionnaire responses suggest that trainees found Desktop-VR more useful (75–64%) and preferred Desktop-VR over traditional training to a greater degree (89–49%) than 360-VR. These findings are consistent with previous research from Taylor and Barnett [Taylor and Barnett (2011)] and Makransky et al. [Makransky et al. (2020)] who found that Immersive virtual reality increases liking but not learning and training outcomes (Similarly, we found that trainees were more likely to recommend 360-VR to others—39% of 360-VR trainees “strongly agree” compared to 31% of Desktop-VR trainees. This is also consistent with the results of Makransky et al. We speculate that trainees found 360-VR “cooler” or more novel or more fun, but less suited to effective training, when compared to Desktop-VR.)
6 Limitations
Unfortunately, due to the fact that these data were collected from real training sessions involving real practitioners, not all confounding variables could be well controlled. All participants who experienced both 360-VR and Desktop-VR experienced 360-VR first, so there may be order effects that were not controlled for. That said, we were able to split the Desktop-VR trainees and compare results for those who had previously experienced 360-VR and those who had not; there were no significant differences in any of the post-training variables. While this does not completely rule out the possibility of order effects, it does provide some evidence that both groups experienced Desktop-VR similarly.
In addition, because the skill test was performed when trainees returned for the Desktop-VR session, real-world scheduling constraints meant that we could only test actual learning one month after the 360-VR session.
On the initial skill test, 48% of trainees had a perfect score, and so any learning from the VR training could not be captured by this test. This is evidence of a ceiling effect. All trainees in this study were experienced mines rescuers with an average of 9 years of mining experience (see Tables 1 and 2); we would likely see different results with inexperienced trainees.
Finally, it is important to note that 360-VR and Desktop-VR differed in many ways, not just in the immersiveness of the display. The means by which users interacted with the simulation were also different. These have been referred to as display fidelity and interaction fidelity, respectively McMahan et al. (2012). Further study would be needed to determine whether the effects we observed were due to display fidelity, interaction fidelity, or a combination of the two.
7 Conclusion
The studies presented in this paper investigated the efficacy of VR, both immersive and non-immersive, for vocational training. A large number of mines rescuers took part in training exercises in 360-VR, Desktop-VR, or both. Across both conditions, participants generally reported that they felt the training was both successful and useful, and that they would recommend VR training to others. Based on these data, it would seem that VR training—whether immersive or not—can be productively employed in vocational training.
The 284 participants who trained in the 360-VR condition completed both pre- and post-experiment questionnaires. Principal component analysis of these data identified six factors: Positive State of Mind, Negative State of Mind, Technology Experience, Positive Learning Experience, Negative Learning Experience, and Learning Context. A subsequent multiple regression analysis found that, of these, Positive Learning Experience, Negative Learning Experience, and Learning Context were significant predictors of perceived learning. Furthermore, Learning Context was by far the strongest predictor of the three. This suggests that the role of the traditional teacher/trainer in VR training remains extremely important.
155 of these participants returned after one month and also trained in the Desktop-VR condition. Results from these participants indicate that their experience with Desktop-VR was significantly better than with 360-VR. Comments from participants suggest that this may be because they were able to act individually in Desktop-VR, while they were constrained by the technology to work as a group in 360-VR. This highlights the importance of platform appropriateness for VR training.
From these results, we see that more sophisticated technology is not necessarily better for learning or training. While all participant groups reported that the training was effective, Desktop-VR was preferred to 360-VR, likely because it enabled the virtual training activities to more accurately model the activities that would be undertaken in the real environment; and the single factor that had the greatest impact on learning outcome was the trainer. We conclude with the following advice for creators of training systems: Unless there is a specific task-informed need for investing in better training technology, you are likely to get superior results from investing in better trainers.
Data Availability Statement
The raw data supporting the conclusions of this article will be made available by the authors, without undue reservation.
Ethics Statement
The studies involving human participants were reviewed and approved by The Human Research Ethics–UOW. The patients/participants provided their written informed consent to participate in this study. Written informed consent was obtained from the individuals in the photos for the publication of any potentially identifiable images or data included in this article.
Author Contributions
SHP, STP, and PP conceptualised the method. SHP attended all the training sessions and collected data. SHP, STP and PP analysed the quantitative data. SHP, RS, MF, and STP wrote the manuscript draft. PP review the final manuscript. Farrelly provided technical guidance on the Virtual Reality technology. All the authors review the final manuscript for submission. All authors read and approved the final manuscript
Conflict of Interest
Author MF was employed by Coal services Pty. Ltd.
The remaining authors declare that the research was conducted in the absence of any commercial or financial relationships that could be construed as a potential conflict of interest.
Acknowledgments
The authors wish to thank trainees and trainers at Coal Services Pty Ltd for allowing access to their facility and participating in this study.
Footnotes
1https://www.coalservices.com.au/mining/mines-rescue/virtual-reality-technologies-vrt/
References
Ai-Lim Lee, E., Wong, K. W., and Fung, C. C. (2010). How does desktop virtual reality enhance learning outcomes? A structural equation modeling approach. Comput. Educ. 55, 1424–1442. doi:10.1016/j.compedu.2010.06.006
Alavi, M., and Leidner, D. E. (2001). Technology-mediated learning—a call for greater depth and breadth of research. Inf. Syst. Res. 12, 1–10. doi:10.1287/isre.12.1.1.9720
Alsina-Jurnet, I., and Gutiérrez-Maldonado, J. (2010). Influence of personality and individual abilities on the sense of presence experienced in anxiety triggering virtual environments. Int. J. Hum. Comput. Stud. 68, 788–801. doi:10.1016/j.ijhcs.2010.07.001
Barbosa Mendes, J., Henrique Caponetto, G., Paiva Lopes, G., and Carlos Brandão Ramos, A. (2010). “Low cost helicopter training simulator a case study from the Brazilian military police,” in 2010 IEEE international conference on virtual environments, human-computer interfaces and measurement systems, Taranto, Italy, September 6–8 2010 (Taranto, Italy: IEEE), 18–22.
Benbunan-Fich, R., and Hiltz, S. R. (2003). Mediators of the effectiveness of online courses. IEEE Trans. Prof. Commun. 46, 298–312. doi:10.1109/tpc.2003.819639
Blickensderfer, B., Liu, D., and Hernandez, A. (2005). Technical Report. Simulation-Based Training: applying lessons learned in aviation to surface transportation modes. Available at: https://www.academia.edu/14082454/ (Accessed June 30, 2005).
Butler, A. C., and Roediger, H. L. (2008). Feedback enhances the positive effects and reduces the negative effects of multiple-choice testing. Mem. Cognit. 36, 604–616. doi:10.3758/MC.36.3.604
Chen, I. Y., Chen, N.-S., and Kinshuk, (2009). Examining the factors influencing participants’ knowledge sharing behavior in virtual learning communities. J. Educ. Technol. Soc. 12, 134–148.
Chittaro, L., Corbett, C. L., McLean, G. A., and Zangrando, N. (2018). Safety knowledge transfer through mobile virtual reality: a study of aviation life preserver donning. Saf. Sci. 102, 159–168. doi:10.1016/j.ssci.2017.10.012
Cobb, S. C. (2009). Social presence and online learning: a current view from a research perspective. J. Interact. Online Learn. 8, 241–254. doi:10.3402/rlt.v22.19710
Dantas, A. M., and Kemm, R. E. (2008). A blended approach to active learning in a physiology laboratory-based subject facilitated by an e-learning component. Adv. Physiol. Educ. 32, 65–75. doi:10.1152/advan.00006.2007.PMID:18334571
Davis, F. D., Bagozzi, R. P., and Warshaw, P. R. (1989). User acceptance of computer technology: a comparison of two theoretical models. Manag. Sci. 35, 982–1003. doi:10.1287/mnsc.35.8.982
Dishaw, M. T., and Strong, D. M. (1999). Extending the technology acceptance model with task–technology fit constructs. Inf. Manag. 36, 9–21. doi:10.1016/S0378-7206(98)00101-3
Fulton, E. A., Jones, T., Boschetti, F., Sporcic, M., De la Mare, W., Syme, G. J., et al. (2011). A multi-model approach to stakeholder engagement in complex environmental problems. Environ. Sci. Pol. 48, 44–56. doi:10.1016/j.envsci.2014.12.006
Gazit, E., Yair, Y., and Chen, D. (2006). The gain and pain in taking the pilot seat: learning dynamics in a non immersive virtual solar system. Virtual Real. 10, 271–282. doi:10.1007/s10055-006-0053-3
Graafland, M., Schraagen, J. M. C., and Schijven, M. P. (2012). Systematic review of validity of serious games for medical education and surgical skills training. Br. J. Surg. 99, 1322–1330. doi:10.1002/bjs.8819
Grabowski, A., and Jankowski, J. (2015). Virtual reality-based pilot training for underground coal miners. Saf. Sci. 72, 310–314. doi:10.1016/j.ssci.2014.09.017
Hiltz, S. R. (1994). The virtual classroom: learning without limits via computer networks. Intellect Books, 384.
Hostetter, C., and Busch, M. (2006). Measuring up online: the relationship between social presence and student learning satisfaction. J. Scholarsh. Teach. Learn. 6, 1–12.
Johnson, C. I., and Priest, H. A. (2014). “The feedback principle in multimedia learning,” in The Cambridge handbook of multimedia learning. Editor R. E. Mayer (New York: Cambridge University Press), Chap. 19, 449–463.
Ju, U., Kang, J., and Wallraven, C. (2019). To brake or not to brake? Personality traits predict decision-making in an accident situation. Front. Psychol. 10, 134. doi:10.3389/fpsyg.2019.00134
Kowalski-Trakofler, K. M., and Barrett, E. A. (2003). The concept of degraded images applied to hazard recognition training in mining for reduction of lost-time injuries. J. Saf. Res. 34, 515–525. doi:10.1016/j.jsr.2003.05.004
Kruglikova, I., Grantcharov, T. P., Drewes, A. M., and Funch-Jensen, P. (2010). The impact of constructive feedback on training in gastrointestinal endoscopy using high-fidelity virtual-reality simulation: a randomised controlled trial. Gut 59, 181–185. doi:10.1136/gut.2009.191825
Lovreglio, R., Gonzalez, V., Amor, R., Spearpoint, M., Thomas, J., Trotter, M., et al. (2017). “The need for enhancing earthquake evacuee safety by using virtual reality serious games,” in Lean and computing in construction congress, Heraklion, Crete, July 4–7, 2017 (Edinburgh United Kingdom: Heriot-Watt University).
Makransky, G., Andreasen, N. K., Baceviciute, S., and Mayer, R. E. (2020). Immersive virtual reality increases liking but not learning with a science simulation and generative learning strategies promote learning in immersive virtual reality. J. Educ. Psychol. doi:10.1037/edu0000473
Makransky, G., and Lilleholt, L. (2018). A structural equation modeling investigation of the emotional value of immersive virtual reality in education. Educ. Technol. Res. Dev. 66, 1141–1164. doi:10.1007/s11423-018-9581-2
Makransky, G., and Petersen, G. B. (2019). Investigating the process of learning with desktop virtual reality: a structural equation modeling approach. Comput. Educ. 134, 15–30. doi:10.1016/j.compedu.2019.02.002
Mansour, S., El-Said, M., and Bennett, L. (2010). “Does the use of second life affect students’ feeling of social presence in e-learning,” in 8th Education and information systems, technologies and applications, Orlando, Florida, June–July 29–2, 2010 (EISTA).
Marcus, N., Ben-Naim, D., and Bain, M. (2011). “Instructional support for teachers and guided feedback for students in an adaptive elearning environment,” in 2011 18th International conference on information technology: new generations, Las Vegas, Nevada, April 11–14, 2011 (Las Vegas, NV: Spinger), 626–631.
Martins, L. L., and Kellermanns, F. W. (2004). A model of business school students’ acceptance of a web-based course management system. Acad. Manag. Learn. Educ. 3, 7–26. doi:10.5465/amle.2004.12436815
Matthews, G., Joyner, L., Gilliland, K., Campbell, S., Falconer, S., and Huggins, J. (1999). Validation of a comprehensive stress state questionnaire: towards a state big three. Pers. Psychol. Eur. 7, 335–350. doi:10.1177/154193120404801107
McAuley, E., and Tammen, V. V. (1989). The effects of subjective and objective competitive outcomes on intrinsic motivation. J. Sport Exerc. Psychol. 11, 84–93. doi:10.1123/jsep.11.1.84
McMahan, R. P., Bowman, D. A., Zielinski, D. J., and Brady, R. B. (2012). Evaluating display fidelity and interaction fidelity in a virtual reality game. IEEE Trans. Visual. Comput. Graph. 18, 626–633. doi:10.1109/TVCG.2012.43
Meadows, D. L. (2001). Tools for understanding the limits to growth: comparing a simulation and a game. Simulat. Gaming 32, 522–536. doi:10.1177/104687810103200408
Merchant, Z., Goetz, E. T., Cifuentes, L., Keeney-Kennicutt, W., and Davis, T. J. (2014). Effectiveness of virtual reality-based instruction on students’ learning outcomes in k-12 and higher education: a meta-analysis. Comput. Educ. 70, 29–40. doi:10.1016/j.compedu.2013.07.033
Merchant, Z., Goetz, E. T., Keeney-Kennicutt, W., man Kwok, O., Cifuentes, L., and Davis, T. J. (2012). The learner characteristics, features of desktop 3D virtual reality environments, and college chemistry instruction: a structural equation modeling analysis. Comput. Educ. 59, 551–568. doi:10.1016/j.compedu.2012.02.004
Moreno, R., and Mayer, R. E. (2002). Learning science in virtual reality multimedia environments: role of methods and media. J. Educ. Psychol. 94, 598–610. doi:10.1037/0022-0663.94.3.598
Nakatsu, R., and Tosa, N. (2000). “Active immersion: the goal of communications with interactive agentsKES’2000,” in Fourth international conference on knowledge-based intelligent engineering systems and allied technologies. Proceedings, Salt Lake City, UT, October 9–10, 2000 (Salt Lake City: IEEE), 1, 85–89.
Ornstein, A. C., and Hunkins, F. P. (1988). Curriculum: foundations, principles, and issues. Englewood Cliffs, NJ: Prentice–Hall, 348.
Parnell, J. A., and Carraher, S. (2003). The management education by internet readiness (MEBIR) scale: developing a scale to assess personal readiness for internet-mediated management education. J. Manag. Educ. 27, 431–446. doi:10.1177/1052562903252506
Pedram, S., Palmisano, S., Skarbez, R., Perez, P., and Farrelly, M. (2020). Investigating the process of mine rescuers’ safety training with immersive virtual reality: a structural equation modelling approach. Comput. Educ. 153, 1038911–1103891. doi:10.1016/j.compedu.2020.103891
Pekrun, R. (2006). The control-value theory of achievement emotions: assumptions, corollaries, and implications for educational research and practice. Educ. Psychol. Rev. 18, 315–341. doi:10.1007/s10648-006-9029-9
Piccoli, G., Ahmad, R., and Ives, B. (2001). Web-based virtual learning environments: a research framework and a preliminary assessment of effectiveness in basic IT skills training. MIS Quarterly, 401–426. doi:10.2307/3250989
Richardson, M., Abraham, C., and Bond, R. (2012). Psychological correlates of university students’ academic performance: a systematic review and meta-analysis. Psychol. Bull. 138, 353–387. doi:10.1037/a0026838
Salzman, M. C., Dede, C., Loftin, R. B., and Chen, J. (1999). A model for understanding how virtual reality aids complex conceptual learning. Presence 8, 293–316. doi:10.1162/105474699566242
Seymour, N. E., Gallagher, A. G., Roman, S. A., O’brien, M. K., Bansal, V. K., Andersen, D. K., et al. (2002). Virtual reality training improves operating room performance: results of a randomized, double-blinded study. Ann. Surg. 236, 458–464. doi:10.1097/01.SLA.0000028969.51489.B4
Sitzmann, T., Ely, K., Brown, K. G., and Bauer, K. N. (2010). Self-assessment of knowledge: a cognitive learning or affective measure?. Acad. Manag. Learn. Educ. 9, 169–191. doi:10.5465/amle.9.2.zqr169
Slater, M. (2004). How colorful was your day? Why questionnaires cannot assess presence in virtual environments. Presence 13, 484–493. doi:10.1162/1054746041944849
Smith, S., and Ericson, E. (2009). Using immersive game-based virtual reality to teach fire-safety skills to children. Virtual Reality 13, 87–99. doi:10.1007/s10055-009-0113-6
Stiggins, R. J., Arter, J. A., Chappuis, J., and Chappuis, S. (2004). Classroom assessment for student learning: doing it right, using it well. Assessment Training Institute, 460.
Strandbygaard, J., Bjerrum, F., Maagaard, M., Winkel, P., Larsen, C. R., Ringsted, C., et al. (2013). Instructor feedback versus no instructor feedback on performance in a laparoscopic virtual reality simulator: a randomized trial. Ann. Surg. 257, 839–844. doi:10.1097/SLA.0b013e31827eee6e
Sylaiou, S., Mania, K., Karoulis, A., and White, M. (2010). Exploring the relationship between presence and enjoyment in a virtual museum. Int. J. Hum. Comput. Stud. 68, 243–253. doi:10.1016/j.ijhcs.2009.11.002
Taylor, G. S., and Barnett, J. S. (2011). Technical Report 1299. Training capabilities of wearable and desktop simulator interfaces. Available at: https://apps.dtic.mil/sti/citations/ADA552441 (Accessed November 1, 2011).
Tichon, J., and Burgess-Limerick, R. (2011). A review of virtual reality as a medium for safety related training in mining. J. Health Serv. Res. Pract. 3, 33–40.
van Ginkel, S., Gulikers, J., Biemans, H., Noroozi, O., Roozen, M., Bos, T., et al. (2019). Fostering oral presentation competence through a virtual reality-based task for delivering feedback. Comput. Educ. 134, 78–97. doi:10.1016/j.compedu.2019.02.006
Vogel, J. J., Vogel, D. S., Cannon-Bowers, J., Bowers, C. A., Muse, K., and Wright, M. (2006). Computer gaming and interactive simulations for learning: a meta-analysis. J. Educ. Comput. Res. 34, 229–243. doi:10.2190/FLHV-K4WA-WPVQ-H0YM
Wan, Z., and Fang, Y. (2006). “The role of information technology in technology-mediated learning: a review of the past for the future,” in Connecting the Americas. 12th Americas conference on information systems, AMCIS 2006, Acapulco, México, August 4–6, 2006 (AMCIS), 2036–2043.
Wisniewski, B., Zierer, K., and Hattie, J. (2020). The power of feedback revisited: a meta-analysis of educational feedback research. Front. Psychol. 10, 3087. doi:10.3389/fpsyg.2019.03087
Witmer, B. G., and Singer, M. J. (1998). Measuring presence in virtual environments: a presence questionnaire. Presence 7, 225–240. doi:10.1162/105474698565686
Zhang, C. (2010). “Using virtual world learning environment as a course component in both distance learning and traditional classroom: implications for technology choice in course delivery,” in SAIS 2010 Proceedings, Washington, DC, December 5, 2010. Available at: https://aisel.aisnet.org/sais2010/35 (Accessed July 17, 2010) 35, 196–200.
Keywords: Virtual reality, Immersive technology, Training, High risk industry, Mining industry, Regression modelling, Prediction
Citation: Pedram S, Skarbez R, Palmisano S, Farrelly M and Perez P (2021) Lessons Learned From Immersive and Desktop VR Training of Mines Rescuers. Front. Virtual Real. 2:627333. doi: 10.3389/frvir.2021.627333
Received: 09 November 2020; Accepted: 18 January 2021;
Published: 26 February 2021.
Edited by:
Liwei Chan, National Chiao Tung University, TaiwanReviewed by:
Meredith Carroll, Florida Institute of Technology, United StatesSalam Daher, New Jersey Institute of Technology, United States
Copyright © 2021 Pedram, Skarbez, Palmisano, Farrelly and Perez. This is an open-access article distributed under the terms of the Creative Commons Attribution License (CC BY). The use, distribution or reproduction in other forums is permitted, provided the original author(s) and the copyright owner(s) are credited and that the original publication in this journal is cited, in accordance with accepted academic practice. No use, distribution or reproduction is permitted which does not comply with these terms.
*Correspondence: Shiva Pedram, c3BlZHJhbUB1b3cuZWR1LmF1