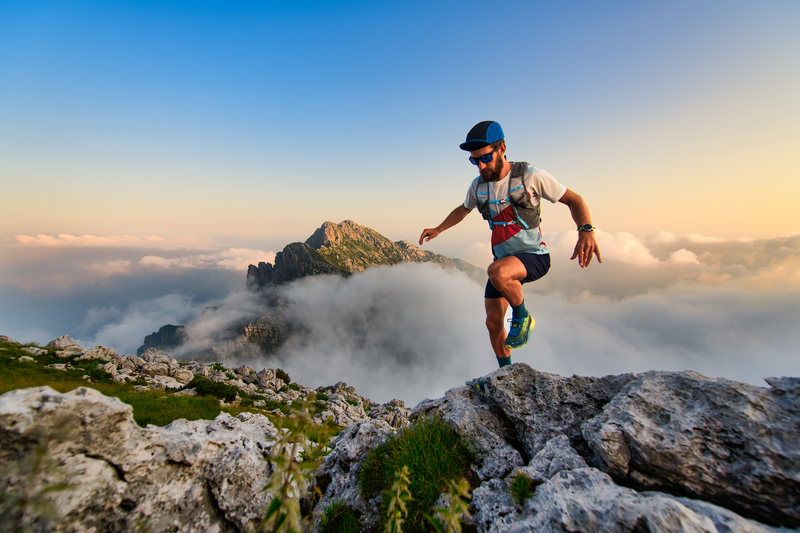
94% of researchers rate our articles as excellent or good
Learn more about the work of our research integrity team to safeguard the quality of each article we publish.
Find out more
ORIGINAL RESEARCH article
Front. Virtual Real. , 22 March 2021
Sec. Virtual Reality and Human Behaviour
Volume 2 - 2021 | https://doi.org/10.3389/frvir.2021.618381
Research and design of virtual reality technologies with mental-health focused applications has increased dramatically in recent years. However, the applications and psychological outcomes of augmented reality (AR) technologies still remain to be widely explored and evaluated. This is particularly true for the use of AR for the self-management of stress, anxiety, and mood. In the current study, we examined the impact of a brief open heart meditation AR experience on participants with moderate levels of anxiety and/or depression. Using a randomized between-group design subjects participated in the AR experience or the AR experience plus frontal gamma asymmetry neurofeedback integrated into the experience. Self-reported mood state and resting-state EEG were recorded before and after the AR intervention for both groups. Participants also reported on engagement and perceived use of the experience as a stress and coping tool. EEG activity was analyzed as a function of the frontal, midline, and parietal scalp regions, and with sLORETA current source density estimates of anterior cingulate and insular cortical regions of interest. Results demonstrated that both versions of the AR meditation significantly reduced negative mood and increased positive mood. The changes in resting state EEG were also comparable between groups, with some trending differences observed, in line with existing research on open heart and other loving-kindness and compassion-based meditations. Engagement was favorable for both versions of the AR experience, with higher levels of engagement reported with the addition of neurofeedback. These results provide early support for the therapeutic potential of AR-integrated meditations as a tool for the self-regulation of mood and emotion, and sets the stage for more research and development into health and wellness-promoting AR applications.
There has been a surge in the interest and application of home and mobile technologies to help deliver health and wellbeing programs and interventions. Developments over the last decade have allowed high quality virtual reality and augmented reality to be experienced with modern handheld smartphone devices, expanding the reach and potential impact of many health and wellness-promoting products and services. Some clinical applications, such as virtual reality-based exposure therapy for phobias (Bouchard et al., 2007) and PTSD (Motraghi et al., 2014), or for rehabilitation after stroke (e.g., Laver et al., 2015) are demonstrative of the unique and beneficial contribution of this technology. Although burgeoning, scientific research and design are still in a stage of relative infancy in terms of understanding and optimizing the use of digital reality in health psychology.
While a substantial amount of research and design has focused on virtual reality (VR) systems with mental health applications (Malbos et al., 2013; Maples-Keller et al., 2017), comparatively less exists in the way of augmented reality (AR) applications (Pallavicini and Bouchard, 2019). To compare and contrast, VR and AR exist at opposing ends of the reality-virtuality continuum, but can be combined to create a spectrum of mixed virtual and augmented reality experiences (Milgram and Kishino, 1994). While VR provides a 360-degree fully simulated virtual environment, AR is distinguished as any technological system that combines real and virtual objects (Azuma, 1997). When used as a mobile phone application, AR systems use the camera to view the real world and then superimposes in virtual elements, creating an augmented view of reality with additional sensory and perceptual features. AR provides a continuous view of the physical world with an additional digital overlay, whereas pure VR does not incorporate the real world resulting in a fully synthetic artificial environment for users. AR interventions for mental health may be particularly powerful as they allow users to interact with virtual objects placed in the real world in real time (Benyousef et al., 2017). This can improve the ecological validity of training or interventions, increase the user’s sense of presence and engagement with the intervention or experience (Waterworth and Riva, 2014), improve compliance and positive outcomes (Giglioli et al., 2015), and also potentially reduce some of the unwanted side effects associated with pure VR immersion, such as real-world environmental unawareness and cybersickness (Silva and Fernando, 2019; Saredakis et al., 2020).
AR technology has been adapted and applied across several fields, including medicine (De Buck et al., 2005), entertainment (Ozbek et al., 2004), and education (Arvanitis, et al., 2009; Kerawalla, et al., 2006). Its applications for mental health and wellness are less well known, but the existing research suggests promise. In their review, Riva et al. (2016) postulated that VR and AR technologies are capable of promoting personal growth and therapeutic changes for individuals suffering from psychiatric disorders by enhancing experiential learning, self-reflection, and self-efficacy. However, their review focused only on VR-based interventions. Giglioli et al. (2015) conducted a systematic review of AR for treating psychological disorders. Of the 13 included studies, the majority focused on small animal phobia treatment and concluded that AR techniques were effective for treating this specific disorder. However, there were not enough included studies to speak to AR’s efficacy for the treatment of other anxiety or mood disorders. Beyond its use in phobia treatments, relatively little research has been conducted on whether AR is a suitable tool for general mood or stress management (Baus and Bouchard, 2014). To date, there has been no research examining the use of AR as a cognitive and emotional self-regulation tool for those with significant generalized anxiety and/or depressive symptoms.
One intriguing avenue of VR and AR technology has been to create immersive and engaging environments in order to support meditation training, and the mental health and wellness benefits associated. A variety of web and mobile-based apps have been developed to provide guided meditation experiences in a convenient, self-help format. In general, these apps appear to be designed to increase mindfulness and reduce stress. In a recent study, Flett et al. (2019) compared the effectiveness of three of the most popular meditation-based phone apps on reducing psychological distress among college students. The average participant used the app 8 of the 10 days of the study. The results revealed that all of the apps led to improvements in depressive symptoms and in college adjustment. One app led to improvements in resilience, and another app led to improvements in mindfulness. In addition, the more the app was used over an extended 30-day period, the more improvement was maintained. Carissoli et al. (2015) compared an app-based mindful breathing meditation method to a relaxation/music listening control over a 3-week period. They found that both groups reported a reduction in stress. However, the apps from these studies were purely audio-based guided meditations.
These and other studies (e.g., Athanas et al., 2019) suggest the feasibility and promise of meditation training apps for supporting mental health and wellness in clinical and non-clinical populations. However, 30-day retention rates for traditional mindfulness-based apps are quite low (median 4.7%; Baumel, et al., 2019), suggesting that they may be of limited long-term value for most users. Seabrook et al. (2020) used a mixed method approach to examine how guided meditations supported by virtual environments may enhance the experience. Specifically, they noted that VR-based experiences may help address some of the difficulties experienced in meditation practice by creating a sense of presence and by “reducing the scope of the content in users’ mind-wandering.” Essentially, by providing specific visual cues in an immersive medium, it may be easier to enter and sustain specific meditative states. This is particularly relevant for beginners or individuals new to the practices of meditation, and who are interested in the widely reported wellness benefits associated with practiced meditation (Brown and Ryan, 2003; Sedlmeier et al., 2012; Goyal et al., 2014), or who are interested in pursuing a longer course of growth and self-actualization through longer-term training in contemplative traditions and practice. Virtual environments can also facilitate the practice of meditation in situations not highly conducive to practice, particularly for novice or first-time meditators who are not yet able to initiate or sustain meditative states in distracting or stressful environments; a utility that has become considerably more pertinent in the background of the global COVID-19 pandemic, where many people will continue to face extended periods of time stressfully confined at home or in hospital environments.
An important point to bear in mind is that meditation is not a homogenous or uniform set of self-regulatory practices. Meditation is an umbrella term which captures an expansive variety of contemplative traditions and practices, inclusive of many techniques and styles across secular and religious entities. The process, goals, and outcomes are different between training systems, between practices within training systems, and even between the same practices within the same meditator in some cases. In order to try to identify and coalesce meaningful differences across such diversity, one of the most prominently employed frameworks for the scientific study of meditation has been to classify various meditation techniques based on how attention is being deployed (Lutz et al., 2008; Dahl et al., 2015). Research suggests that these different categories of meditation are also associated with unique electrophysiological signatures (Cahn and Polich, 2006; Tarrant, 2017b). Based on this framework, Tarrant (2017a) developed a matching set of guided meditations that include focus, mindfulness, open heart, and quiet mind meditations, augmentable by the addition of EEG neurofeedback technology in order to facilitate the depth and consistency of these meditations.
The open heart style of meditation which was selected for this study guides the meditator to engage in positive emotional states such as compassion, gratitude, or empathy and then direct those feelings toward the self or others (Tarrant, 2017a), similar to many other forms of loving-kindness and compassion meditation practices (Dahl et al., 2015). Research on this type of practice has demonstrated that even a brief training period can have significant results, including the reduction of pain, anxiety, anger, and mood difficulties (Carson et al., 2005; Gilbert and Proctor, 2006). As short as a 7-min open heart meditation has been shown to produce significant improvements in feelings of positivity (Hutcherson et al., 2008).
On this background, the current study examined the impact of a brief open heart meditation in AR on an adult sample of participants who had a moderate-to-severe range of depression and/or anxiety symptoms, with the aims of reducing negative mood states and increasing positive mood states in these volunteers. Additionally, we directly compared AR-guided meditation implemented with (AR+NF) and without (AR−NF) adjunctive neurofeedback influencing the virtual elements to evaluate whether a frontal gamma asymmetry neurofeedback protocol (Tarrant et al., 2018) would be ameliorative.
Our primary hypotheses were that both groups would show reductions in negative mood states with increased feelings of positive mood states, but that the addition of a neurofeedback input would increase these effects. Second, we hypothesized that there would be a larger change in cortical activity, as measured by pre-to-post resting-state EEG activity, for the AR+NF group, beyond that of the AR−NF group. Specifically, we expected to see significant shifts in gamma activity, given that the neurofeedback protocol was designed to promote frontal asymmetric activity patterns previously linked with positive mental health states (Tarrant et al., 2015) and based on previous research observing changes in gamma-related activity resulting from LK-C meditation practiced by expert meditators (Lutz et al., 2008). We also expected to see increased activity in the anterior cingulate cortex (ACC) and right insular cortex, regions that are known to support the experience of subjective feeling states, including empathy (Singer et al., 2004; Craig, 2009). Finally, we expected the EEG changes to correlate more strongly with mood changes for the AR+NF group compared to the AR−NF group. This finding would support the idea that the neurofeedback protocol helped to drive the underlying neural systems and states involved in the meditative experience, potentially facilitating the meditation experience and benefits thereof in the long term.
The study was performed at the NeuroMeditation Institute, LLC in Eugene, Oregon. It was approved by the Quorum Institutional Review Board, Seattle, WA. Participants were recruited through flyers and marketing on Facebook® and Craigslist. Interested participants completed an on-line screening (n = 78) or a phone screening (n = 28) which consisted of exclusion/inclusion criterion as well as completion of the Generalized Anxiety Disorder (GAD-7; Spitzer et al., 2006) and Patient Health Questionnaire (PHQ-9; Kroenke et al., 2001). Exclusion criteria included a history of head injury, seizure activity, or major mental health concerns (schizophrenia or bipolar disorder).
Inclusion criteria required participants be at least 18 years old, and report levels of generalized anxiety, and/or depression symptoms at or above the moderate cutoff point. Moderate levels of anxiety and depression were defined by cutoff scores of 8 or higher (M = 11.0 ± 3.2) on the GAD-7 (Spitzer et al., 2006) and scores of 10 or higher (M = 12.9 ± 3.9) on the PHQ-9 (Kroenke et al., 2001). Subjects passing the screening and agreeing to participate were randomly assigned to either the AR+NF or AR−NF condition. The final sample consisted of 22 participants in the AR+NF and 19 participants in the AR−NF group. The EEG data of one participant assigned to the AR+NF condition was contaminated by high levels of artifactual noise and removed from further analysis.
This questionnaire asked subjects to identify information related to their sex, age, race, education level, experience with meditative practices, and history of mental illness.
The Brunel Mood Scale (Soos et al., 2007) consists of 32 mood descriptors that are categorized into eight unipolar dimensions: anger, tension, depression, vigor, fatigue, confusion, happiness, and calmness. The anger and vigor subscales were not used in this study. Using a response frame of “how do you feel right now?” subjects indicated the extent of their experience of the mood descriptors on a 5-point scale (0 = not at all, 1 = a little, 2 = moderately, 3 = quite a bit, 4 = extremely).
In an attempt to examine subjective perceptions of the two experiences in regard to engagement and motivation, subjects were asked to respond to three statements at the conclusion of the experiment. The first item was “how engaged were you in this meditation experience?” (1 = not at all, 2 = a little, 3 = a moderate amount, 4 = a lot, 5 = a great deal). The second item “do you think this type of meditation application could help you manage stress or anxiety in the future?” was a simple yes or no response. The third item was “how likely are you to use this type of digital meditation in the future?” (1 = very unlikely, 2 = unlikely, 3 = somewhat unlikely, 4 = neither likely or unlikely, 5 = somewhat likely, 6 = likely, 7 = very likely).
The AR experience used an Apple iPhone with the Healium software. This software can be used with or without biometric inputs to control aspects of the AR experience with biometric data. A 4-min guided experience encouraged the user to activate feelings of calm and positivity to hatch virtual butterflies from a virtual chrysalis. The AR images viewed on the iPhone were superimposed on the environment viewed by the phone’s camera, giving the impression that the experience was happening in the physical environment (Figure 1). As the experience progressed, the user was coached into recalling times they felt happiness and appreciation which gradually resulted in the hatching of butterflies. The top of the screen had a counter to keep track of the number of butterflies hatched, and as the butterflies hatched they “flew around the room” and could be tracked by moving the phone, providing an interactive element.
FIGURE 1. Example of the equipment and AR visual experience. (A) Muse headband for neurofeedback and the Healium augmented reality headset. (B) The Healium neurofeedback setup as worn. (C) A screenshot of the AR-guided meditation with chrysalis hatching neurofeedback. Below is an enlarged image of the threshold line (solid) and “firefly” depicting gamma asymmetry ratios, with part of the meditation instruction shown in text.
The AR+NF group engaged in the butterfly experience driven by the brainwave inputs from the Muse headband. In this group, the EEG data was displayed in a simplified form at the bottom of the Healium app. Specifically, the ratio of gamma asymmetry between the two frontal electrodes was represented by a glowing ball that moved up and down in relation to changes in the ratio and across the screen in relation to time (Figure 1C, bottom). Higher numbers (ball moving up and changing to yellow glow) reflected a shift of gamma power to the left while lower values (ball moving down and changing to blue glow) reflected a shift to the right. The delay between signal analysis and feedback stimuli changes approximated 1 s. A solid reference or threshold line was displayed in the same space providing a target for the biometric information. The app threshold was adjustable to make the task easier or harder. However, for the current study, the threshold was adjusted to “easy” for all participants. When the gamma asymmetry rose and remained above the threshold for 6 s, it activated specific assets in the experience; in this case hatching butterflies. However, when the Muse-derived EEG signal data fell below the neurofeedback threshold for five consecutive seconds, the screen tinted red, the hatching paused, and the verbal guidance was re-activated, reminding the user of the intention behind the experience.
The AR−NF group engaged in the same butterfly experience for the same length of time, but without biometric inputs. In this case, the experience played forward with the butterflies automatically hatching at a rate similar to that experienced in the AR+NF group when criterion were consistently met.
The Muse headband was used to record and provide frontal gamma asymmetry feedback integrated with the AR experience. Specifically, the ratio of gamma activity (30–44 Hz) was calculated between the two frontal electrodes of the Muse headband at sites AF7 and AF8. It was operating as a closed system from the research EEG data collection process described below, as would be the case for its typical consumer use. The Muse headband has demonstrated itself as a valid neurofeedback device in studies examining mindfulness training (Bhayee et al., 2016), and to differentiate states of concentration and relaxation (Kovacevic et al., 2015). More recently, Tarrant and Cope (2018) published a proof of concept study indicating that the same gamma asymmetry protocol with the Muse headband can be used as an effective neurofeedback tool for shifting mood states.
The EEG data collected for statistical analysis were sampled with 19 electrodes in the standard 10–20 international placement referenced to linked ears using a BrainMaster Discovery amplifier (BrainMaster Technologies, Inc.). Electrode sites corresponded to Fp1, Fp2, F3, F4, F7, F8, Fz, C3, C4, Cz, P3, P4, Pz, T3, T4, T5, T6, O1, and O2. Five minutes of eyes-closed resting data was collected immediately before and after the intervention. Subjects were given brief instruction on relaxing the eyes and jaw to minimize artifact. Each raw EEG file was processed through a Standardized Artifact Rejection Algorithm (S.A.R.A; QEEG Professionals, The Netherlands). This procedure removes segments from an EEG recording that are likely due to other sources, such as eye blinks, muscle tension, etc. Using an automated process such as this ensures that each file is handled in the same manner and reduces the possibility of bias in the artifact removal process.
This study implemented a randomized between-group design. Participants were blind to which condition they were assigned to. They were simply informed that the study was comparing two different augmented reality meditation experiences. Participating subjects were scheduled for a 75-min office visit. After a verbal description of the study procedures and completion of IRB information form and informed consent, participants completed a demographic form, and the Brunel Mood questionnaire. Subjects were then provided with a demonstration of the AR experience to orient them toward the process. Following this orientation, subjects were fitted with a 19-channel EEG electrocap (Electrocap International, United States). Each electrode was prepped using electrogel conductance paste (Electro-Cap International, Inc. United States). Impedances for all sites were assessed prior to each recording and kept below 10 kOhms. Subjects completed a 5-min, eyes-closed EEG baseline, recorded using a BrainMaster Discovery amplifier (BrainMaster Technologies, Inc., United States). AR+NF participants were then fitted with the Muse headband and provided with an iPhone with the Healium experience already loaded. AR−NF participants were simply given the iPhone with the standard experience. Immediately following the intervention, a second eyes-closed baseline was recorded for 5 min and subjects were asked to complete a second Brunel Mood questionnaire and the AR experience questions. Participants were given a $25 gift card upon study completion.
Global and regional activity was evaluated by averaging mean power across scalp electrodes, across the following selection of conventionally defined frequency bands: alpha (8–12 Hz), beta (12–30 Hz), and gamma (30–50 Hz). Electrodes were grouped into frontal (Fp1, Fp2, Fz, F3, F4, F7, F8), midline (Fz, Cz, Pz), and parietal (Pz, P3, P4) regions for analysis. Source-location analysis (sLORETA; Pascual-Marqui, 2002; Pascual-Marqui, 2007) was also conducted in order to calculate current source density estimations in the anterior ACC and insula cortical regions of interest. Follow-up from significant main effects, included evaluating left vs. right hemispheric differences, and evaluating alpha and beta were broken down into the following sub-band ranges; lower alpha (8–10 Hz), upper alpha (10–12 Hz), low beta (12–18), and high beta (18–30 Hz).
Independent t-tests were used to test for equivalency between groups prior to the AR experience, and on demographic and primary dependent measures. Assumptions of normality and equality of variance were evaluated with Levene’s tests (p < 0.05), Shapiro-Wilks tests (p < 0.05), as well as inspection of QQ plots, histograms, and skew and kurtosis values. The initial data set revealed significant violations of normality identified as being caused by significant outlier data points. This was dealt with as objectively and consistently as possible by employing the recommended method outlined by Hoaglin and Iglewicz (1987). Briefly, it involves calculating the difference between the third and the first quartile value for each measurement multiplied by a g coefficient value of 2.2. Data points below this value minus the first quartile, or above this value plus the third quartile value, are marked as highly significant outliers, and are removed. Cleaned data were analyzed by two-way repeated-measures ANOVAs to test within- and between-group effects, with group (AR+NF, AR−NF) and testing point (pre and post AR) factorials. Chi-squared likelihood ratios (G2) were used to test significance differences between groups on the AR experience survey. Family-wise error corrections for multiple comparisons were applied according to the Holm-Bonferroni method (Holm, 1979).
Initial evaluation of data indicated significant kurtosis, and severe violations of normality and equivalence of variance across a number of variables. Left uncorrected, this would have significantly affected the reliability and confidence of the parametric-based approaches used. A very small number of highly significant outlier EEG data points were evident upon visual inspection of histogram and stem-and-leaf plots, produced by a few select participants. The method outlined by Hoaglin and Iglewicz (1987) was used to objectively and consistently identify and remove outlier data from further analysis. Less than 2% of the dependent variable data were identified as outlying and removed. This data primarily sourced to two participants in the AR+NF group, created by channel noise or possible muscle tension contamination. Subsequent normality and variance tests were passed after the significant flagged outlier data was removed. Characteristics of the final sample are displayed in Table 1. There were no statistically significant differences between groups across demographic measures, with the exception of the higher number of meditation sessions per week in the AR−NF group (t39 = 2.31, p = 0.026). This is largely attributable to two participants endorsing meditation more than six times per week who were both part of the NF-AR group. However, the weekly time in meditation was not significantly different between groups (t39 = 2.31, p = 0.143).
No statistically significant interaction or between group main effects occurred. A significant main effect for time (pre, post) was found for each of the following subscales: happy (F1,37 = 12.9, p = 0.001, ηp2 = 0.258), calm (F1,37 = 28.4, p < 0.001, ηp2 = 0.43), depression (F1,37 = 27.3, p < 0.001, ηp2 = 0.42), tension (F1,37 = 41.4, p < 0.001, ηp2 = 0.53), and fatigue (F1,37 = 6.81, p = 0.013, ηp2 = 0.16). For both conditions, on average, happiness and calmness increased, while depression, tension, vigilance, and fatigue decreased. The decrease in vigilance was the only measure not to reach statistical significance (F1,38 = 2.92, p = 0.096, ηp2 = 0.07). Table 2 displays the pre and post group means for Brunel ratings. Figure 2 displays the average individual change in scores after the AR meditation for each group.
FIGURE 2. Mean difference of rating on questionnaire subscales pre-to-post AR meditation. Both groups demonstrated significant pre-to-post changes across subscales, with the exception of the vigilance subscale. No significant differences occurred between groups or as interaction effects. Mean of differences and standard errors of the mean are shown.
Changes in resting state brain activity (alpha, beta, gamma) before and after the AR meditation were analyzed by scalp region (frontal, midline, parietal), and with terms of current source density estimates in the ACC and insula regions calculated by the sLORETA method (Pascual-Marqui, 2002).
There were no significant interactions or between-group main effects. There were significant main effects for time (pre, post) found for alpha (F1,37 = 7.84, p = 0.008, ηp2 = 0.17) and gamma (F1,36 = 5.21, p = 0.029, ηp2 = 0.13), but not for beta (F1,38 = 0.001, p = 0.981, ηp2 < 0.01). On average both groups demonstrated increased alpha activity and decreased gamma activity. Follow-up analysis indicated that the alpha effect was driven largely by increased lower alpha activity (F1,36 = 6.53, p = 0.015, ηp2 = 0.15) as opposed to upper alpha activity (F1,38 = 3.15, p = 0.084, ηp2 = 0.08), with lower alpha effects more left (F1,36 = 8.69, p = 0.006, ηp2 = 0.19) than right lateralized (F1,36 = 4.66, p = 0.038, ηp2 = 0.12). This was also true of gamma changes, whereby left hemisphere activity (F1,36 = 8.69, p = 0.006, ηp2 = 0.17) decreased significantly for both groups, but not so for right frontal gamma activity (F1,36 = 0.916, p = 0.345, ηp2 = 0.03). Interestingly, the average right frontal gamma activity remained largely unchanged on average for the AR+NF group, whereas it decreased for the AR−NF group (means found in Table 3), although this trend did not reach statistically significant levels.
There were no significant interactions or between-group main effects. Significant main effects for time (pre, post) were found to be related to increased alpha (F1,38 = 9.91, p = 0.003, ηp2 = 0.21) and beta (F1,38 = 14.00, p = 0.001, ηp2 = 0.27) power (Figure 3B). Follow-up analysis indicated that lower alpha activity more prominently increased (F1,37 = 9.29, p = 0.004, ηp2 = 0.20) compared to upper alpha activity (F1,38 = 3.37, p = 0.074, ηp2 = 0.08). In contrast beta activity changes were underlined by broader band increases (low beta, F1,38 = 11.62, p = 0.002, ηp2 = 0.23; high beta, F1,37 = 10.87, p = 0.002, ηp2 = 0.23). Time (F1,35 = 3.10, p = 0.087, ηp2 = 0.08) and interaction (F1,35 = 3.37, p = 0.075, ηp2 = 0.09) effects approached significance for gamma midline activity, whereby sparse change occurred in the AR−NF group, but showed trending increases in the AR+NF group (Figure 3B, right).
FIGURE 3. Changes in resting state EEG after AR-guided meditation with and without neurofeedback. T* denotes significant main effect for pre-post change for both conditions. No significant between-group main effects or interactions effects occurred. Means and standard error of the means are shown. Units are mV2.
There were no significant interactions or between-group main effects. Significant main effects for time (Pre, Post) were found related to increased alpha (F1,38 = 8.14, p = 0.007, ηp2 = 0.18) and increased beta (F1,38 = 8.90, p = 0.005, ηp2 = 0.19) power (Figure 3C). The pattern of results was similar to those of the midline analyses, with changes in lower alpha (F1,37 = 8.25, p = 0.007, ηp2 = 0.18) more evident than changes in upper alpha (F1,38 = 2.11, p = 0.155, ηp2 = 0.05), and with beta changes were more evenly distributed (low beta, F1,38 = 6.14, p = 0.018, ηp2 = 0.14; high beta, F1,38 = 7.29, p = 0.010, ηp2 = 0.16). Gamma effects did not approach significance over the parietal lobe (F1,36 = 0.11, p = 0.747, ηp2 < 0.01).
No significant within, between, or interaction effects were observed with respect to ACC localized activity across any bands of interest.
There were no significant interactions or between-group main effects. Insular beta (F1,36 = 4.09, p = 0.053, ηp2 = 0.10) and gamma (F1,36 = 3.70, p = 0.062, ηp2 = 0.93) trended towards statistical cutoffs. Increased current source densities were found for each band. Significant main effects for time (Pre, Post) were found for alpha (F1,36 = 12.68, p = 0.001, ηp2 = 0.26) and beta (F1,36 = 8.31, p = 0.007, ηp2 = 0.19), but not for gamma (F1,36 = 3.42, p = 0.073, ηp2 = 0.09). Follow-up on lateralization, a significant main effect of time was found for left (F1,36 = 10.21, p = 0.003, ηp2 = 0.22) and right (F1,36 = 11.96, p = 0.001, ηp2 = 0.25) alpha activity, and for right but not left beta (right, F1,36 = 9.89, p = 0.003, ηp2 = 0.22; left, F1,36 = 0.83, p = 0.368, ηp2 = 0.03) and gamma activity (right, F1,36 = 5.71, p = 0.022, ηp2 = 0.04; left, F1,36 = 0.10, p = 0.753, ηp2 <0.01). Figure 4 illustrates the favoring increased activity in the right compared to left insula between groups. Notably, despite outlier removal, right insula gamma data remained significant for Levene’s test of equality of variance (p = 0.048) so this finding must be interpreted with due caution.
FIGURE 4. Lateralized activity differences in insular cortex activity after AR meditation. Mean and standard error of the mean are shown. Units are nA/mm3.
In terms of how engaging participants found the AR experiences, overall response distributions indicated some notable differences in response patterns distributions (G2 = 7.84, p = 0.05). While the majority of both groups indicated being engaged “a lot” or “a great deal”, more participants in the AR+NF group endorsed “a great deal” (G2 = 4.56, p = 0.033). Both groups responded similarly in their thinking that the AR experiences they received could help manage stress or anxiety (G2 = 0.40, p = 0.530), with 83% of the overall sample indicating “yes”. In terms of their predictive use of similar AR meditations in the future, again responses between the two groups were comparable (G2 = 5.39, p = 0.495), with 73% of the overall sample indicating that they were somewhat-to-very likely to use this type of experience in the future. Figure 5 displays the pattern of endorsement frequencies for the questions.
FIGURE 5. Between-group item endorsement patterns for AR experience questions. Percentage of response endorsements for each item for each group are displayed.
The correlational analyses of mood and EEG data revealed that post-to-pre changes in frontal gamma were negatively associated with changes in vigilance across groups (r = −0.50, p = 0.001), but this relationship was primarily driven by the AR+NF group (r = −0.70, p < 0.001; NF-AR, r = −0.11, p = 0.625). Changes in depression scores were negatively correlated with right frontal alpha for the AR+NF group (r = −0.44, p = 0.046) but not the AR−NF group (r = −0.14, p = 0.580). In contrast, changes in depression scores for the AR−NF group were negatively correlated with change in left frontal gamma activity (r = −0.53, p = 0.035), but not for the AR+NF group (r = 0.34 p = 0.127). No significant correlational relationships were observed between the frontal, ACC, or insular regions of interest as anticipated.
Other unexpected results between groups were found with exploratory analyses. Parietal alpha (r = −0.507, p = 0.016) and beta (r = −0.540, p = 0.017) were negatively correlated with changes in fatigue only for the AR−NF group (AR+NF Alpha, r = −0.126, p = 0.586; AR+NF Beta, r = −0.093, p = 0.681). Changes in happiness scores were negatively correlated with changes in midline high beta activity for the AR+NF (r = −0.44, p = 0.047) group, but not for the AR−NF group (r = 0.03 p = 0.900).
In terms of AR meditation questions, level of engagement predicted anticipated future use for stress and anxiety coping (r = 0.328, p = 0.036). Overall increased happiness (r = 0.314, p = 0.046) and vigilance were positively associated with engagement ratings of the AR experience (r = 0.334, p = 0.036). Future use was positively correlated with participants increases in calmness ratings (r = 0.311, p = 0.048). Changes in electrophysiological activity did not predict survey responses (p’s > 0.10).
In this study we investigated user experience, as well as mood and electrophysiological changes resulting from a guided loving-kindness and compassion-based meditation (open heart) in an AR environment, with or without adjunctive neurofeedback. We found some notable differences in user’s experiences between groups and in EEG activity at rest in the wake of the meditation experience. However, overall, the AR meditation produced highly similar effects on mood and EEG activity regardless of the inclusion of neurofeedback.
We are unaware of previous studies that have examined a combination of AR with neurofeedback for anxiety or mood concerns. Potts et al. (2019) completed a feasibility study combining AR (MagicLeap hardware) with a consumer grade EEG headband (Muse) as a meditative experience. The authors reported that participants using the application found it enjoyable and relaxing, but no formal examination of mood state was conducted. Cebolla et al. (2019) conducted a randomized controlled trial aimed to investigate the efficacy of a self-compassion meditation with and without VR augmentation on a non-clinical sample of young post-secondary students. The VR augmentation delivered a body swap simulation to increase mindful awareness and positive affect and self-care behaviors. After 2 weeks of regular practice, both conditions demonstrated an increase in self-care behaviors, but significantly more so in the VR meditation condition. Both groups demonstrated a similar frequency of keeping up with weekly meditation practice. In our study, the vast majority of participants in both groups reported finding value in the AR meditation experience as a useful resource to help manage stress and anxiety. Both groups reported high levels of engagement with the AR meditation, but the AR+NF group endorsed greater levels of engagement than the AR−NF group. The high levels of engagement reported by both groups seems in line with results of Potts et al. (2019) and Cebolla et al. (2019), although a long training regimen and follow-up measurements are needed to confirm that the high engagement reported by participants veritably translates into sustained practice habits.
In terms of mood and electrophysiological effects, we anticipated that there would be a significant increase in positive mood state and decreases in negative mood states for both groups, but that these changes would be larger and correlate more strongly with resting state EEG change resulting from the meditation experience. Our results showed equivalent change between the two groups across the mood subscales, to the effect that both groups showed significant increases in the ratings of happiness and calmness, and significant decreases in depression, tension, and fatigue. Our results align with that of an earlier study examining the effects of a brief VR-guided meditation, without neurofeedback, on anxiety and depression symptoms (Tarrant et al., 2018). For both experimental groups, the single session of AR meditation produced significant changes in electrophysiological resting state activity, consistent with physiological changes recorded after single brief meditation (Cahn and Polich, 2006; Tang et al., 2009; Amihai and Kozhevnikov, 2014) and neurofeedback sessions (Chow et al., 2016).
However, between group differences in EEG were not very evident. This is perhaps not a surprising finding given the briefness of the session. Gorini et al. (2010) directly compared the impact of using VR with heart rate biofeedback, VR without biofeedback, and a waitlist control intervention for persons diagnosed with generalized anxiety disorder. Both experimental groups demonstrated similarly improved clinical outcomes at the end of the eight-week treatment period. Additionally, the group with adjunctive biofeedback had slightly larger changes in physiological outcome measures including decreased heart rate and skin conductance. More practice over a longer timescale may be required for more robust and reliably divergent neurophysiological outcomes to emerge with respect to the addition of adjunctive neurofeedback (Marzbani et al., 2016). It is well known in the meditation literature that robust and lasting physiological and neural connectivity changes, associated with enhanced cognitive and emotional self-regulation, requires a substantial amount of training; minimal daily practice for several weeks is needed for early markers of enduring neurocognitive changes to be reliably observed (Nyklíc et al., 2013; Tang et al., 2015), but months to years of practice are needed to integrate, as an effortless trait, the more profound neurocognitive abilities and benefits associated with extensive meditative training (Grossman et al., 2004; Lutz et al., 2004; Eberth and Sedlmeier, 2012; Ferrarelli et al., 2013; Fox et al., 2014). Such changes emerge slowly from the cumulative effects of repeatedly entering and maintaining various meditative states in formal meditation sessions, but also include the practiced generalization of the skills and attitudes into daily life.
The spectral shifts observed across conventionally defined EEG bands and regions of interest demonstrated a high degree of similarity between groups in our study. To the previous point, these observations are presumed to reflect a temporary positive change in the participants’ psychological and physiological state (rather than trait) resulting from the AR meditation. Specifically, both groups showed increased alpha and reduced gamma frontally, and predominantly left lateralized. Midline and parietal regions showed significant increases in alpha and beta, with the alpha effect specifically being driven by increased lower frequency alpha activity. A trending divergence in gamma activity was observed, with the AR+NF group tending to show increased gamma activity in midline and source-localized insula regions of interest, with some lateralization, while the AR−NF group’s gamma activity remained largely unchanged in these same regions. As compelling and consistent with other literature as this is, these trending interactional patterns were not robust enough to be considered statistically reliable in our sample and require replication with larger sample sizes, or perhaps a longer period of training is required to produce and consolidate this emerging EEG difference (Marzbani et al., 2016).
Increases in parietal alpha activity is one of the most reliable meditation-induced EEG effects (particularly object-focused meditations; Cahn and Polich, 2006; Chow et al., 2016; Fell et al., 2010), which is thought to represent a mental and physiological state of calm-abiding alertness (Thompson and Thompson, 2003; Olbrich et al., 2011). While this appears in line with the mood and EEG effects, the directional shifts in gamma and beta are a little more difficult to interpret. It has been suggested that trait levels of depressed affects are associated with suppressed left frontal activity (Davidson, 1992), and that high frequency activity in the left frontal cortex are linked with positive mood states and approach behaviors whereas right lateralized frontal activity is linked with negative mood states and withdrawal behaviors (Sutton and Davidson, 1997). Multiple studies examining EEG frontal asymmetries and the induction of mood states have demonstrated greater right activation with induced positive mood and greater left activation with negative moods (Kop et al., 2011; Warden-Smith et al., 2017). Other studies have found no connection between frontal EEG asymmetry and mood reactivity (Gotlib et al., 1998; Dennis and Solomon, 2010), potentially because these patterns are moderated by individual factors such as personality traits (Gale et al., 2001) and individual differences in functional neural lateralization (Lezak et al., 2012). Given the conflicting research regarding frontal asymmetry’s relationship to mood, the current findings may be best understood through a different lens.
Turning to meditation literature, Engström and Söderfeldt (2010) conducted an imaging study on a Tibetan Buddhist with many years of compassion meditation practice. During meditation, fMRI imaging revealed activation of several brain regions involved in sustained attention and empathy, with the strongest findings related to activation of the left prefrontal cortex. In a study comparing eight long-term meditators with eight novice meditators, there were common activation patterns found in the adepts when engaged in a compassion form of meditation. Among the findings, expert meditators showed large increases in the proportion of frontal gamma brainwave activity during the meditation in several areas of the brain including the left prefrontal cortex (Lutz et al., 2004). On the other hand, in a sample of mindfulness meditation-trained participants Farb et al. (2007), mindfulness meditation resulted in a reduction of medial prefrontal cortex activity, and increased engagement of right lateralized regions including the lateral prefrontal cortex, insula, secondary somatosensory cortex, and inferior parietal lobule.
Notably, in our study the NF protocol reinforced left frontal gamma activation. Gamma has a demonstrably consistent relationship with frontal lobe glucose metabolism (Oakes et al., 2004). Consequently, gamma activity may provide the most direct measure of frontal network activation (Lutz et al., 2004). Consistent with this understanding, frontal gamma asymmetry has been shown to be a better predictor of motivation than alpha asymmetry (Ramsøy et al., 2018) as well as a reliable method for examining changes in emotional states (Bonnstetter et al., 2015). In a feasibility case series study (n = 4) conducted by Tarrant and Cope (2018), also including participants with elevated anxiety and depression, the left frontal gamma NF protocol led to alleviation of depression symptoms and increased positive mood states for some, but not all, participants (Tarrant and Cope, 2018).
On this background, we expected to find more evidence of between-group differences in relation to EEG changes after the AR meditation associated with the addition of neurofeedback. Specifically, we hypothesized that the AR+NF group would show stronger changes in frontal asymmetry, congruent with positive mental state changes, as well as stronger activation patterns in both the anterior cingulate cortex and the right insula. These are areas which are often recruited in meditation (Fox et al., 2014; Fox et al., 2016) and have been associated with empathic states and compassion generation (Lutz et al., 2004; Singer et al., 2009). However, our results did not emerge completely as expected.
Our finding of decreased frontal left gamma replaced by increased frontal low alpha seems largely at odds with what might be expected based on the meditation and affect and EEG asymmetry discussed in literature. One reasonable possibility is that this could be due to differences in the sample characteristics of this study (elevated clinical symptoms and mixed meditation experience) or the meditation itself (length, instructions, AR component). However, our pattern of results could also be interpreted by other accounts. For example, the decreased gamma in lieu of increased lower alpha could be the response of frontal attentional and executive neural regions deactivating post-meditation as a result of being in high demand during the meditation. Alternatively, it might be less of a passive rebounding effect, and more of an active continuation of the unique state of mind cultivated by the meditation; a state of quieting of the frontal nodes of the default mode network, a major functional network linked to self-referential processing and self-directed thought (Raichle et al., 2001; Northoff et al., 2006).
Increased power in the lower gamma band ranges has been linked to activity in the prefrontal node of the DMN (Mantini et al., 2007; Chen et al., 2008). Therefore, the decrease of gamma and increase of low alpha might be a reflection of a quieting of activity related to verbal narrative-based thinking, analysis, or other goal-oriented states (Lezak et al., 2012). Thus, the increased presence of low alpha in frontal and parietal regions may be in line with participants residing in a “being mode” of mind rather than a “doing mode” of mind, which has been closely aligned with mindfulness-based meditation (Williams, 2008; Kabat-Zinn, 2013; Segal et al., 2013). Increased central and parietal low alpha usually co-occurs with a reduction in beta when people enter more relaxed states as a result of natural waking rest or as induced by some (but not all, e.g.; Amihai and Kozhevnikov, 2014) types of meditation. The increased beta power alongside the lower alpha increases may reflect continued broad cortical excitability associated with the AR experience, or perhaps it is reflective of more specific networks involved in feelings and thoughts of compassion and gratitude (Laufs et al., 2003; Mantini et al., 2007; Chen et al., 2008). Taken together the complex and unique pattern of EEG results we observed, which are both similar and different to those observed in non-AR/VR inclusive meditations, might just be a reflection of the calm, positive, abiding state generated by the open heart AR mediation. This fits with the increased ratings of calmness and happiness indicated on the Brunel scales. These results will need replication and could be enhanced with the direct inclusion of self-compassion measures to validate this feeling state more precisely.
Unfortunately, the correlational analyses did not produce results in line with our hypotheses. Different correlational relationships were observed between changes in mood and changes in EEG between groups, against expectation. A result like this could emerge because neural or psychological mechanisms have been recruited differently between the groups, perhaps due to the presence or absence of NF. However, given the small sample size and exploratory nature of the correlational findings, all interpretations should be made with a high degree of caution, and require replication in higher powered studies.
The use of commercial industry vs. “in-house” technology and software is beneficial in terms of ecological validity; studying the effects of already widely sold and used consumer products is a merited scientific endeavor. However, a major limitation related to this approach is that key performance data were not made available to the authors by the NF hardware and software developer (Muse). Thus, we could not statistically evaluate or control for degree of learning between participants. This is a particularly critical issue because it has been documented that between 10–40% of the general population are brain-computer interface (BCI) non-learners (Guger et al., 2003; Blankertz et al., 2010; Zich et al., 2015). This is a wide ranging and not well understood phenomenon, but could be increasingly addressed by the availability of performance data for single study and meta-level analysis. This fuzzy non-learner issue has a direct impact on the power and planning of NF research, given that NF is a BCI technique, and further reduces the ability to understand the relationship between performance and outcomes in conducted research. Interestingly, some researchers have data to suggest that training in meditation may actually enhance NF/BCI learning, which further speaks to the importance of exploring these relationships as thoroughly as possible (Garrison et al., 2013; Davelaar et al., 2018). This will be an interesting and important area for future studies to address and explore, but one we were not able to approach in our study due to this limitation. We strongly recommend performance metrics be made available to researchers in industry collaborative studies in order to improve the quality and interpretability of findings. Translation of metrics into arbitrary units of performance may be one way to protect “trade secrets” and proprietary calculations while still providing usable performance data to move basic and applied science forward in important and necessary ways for all stakeholders.
Other limitations include the absence of an additional control comparison groups, the sample characteristics, the brief nature of the study, and small sample size. It cannot be ruled out that the observed changes in EEG and mood states were anything above and beyond taking the same amount of time for unoccupied rest. Individuals high in anxiety and depression, as were selected for in this study, are more prone to worry or rumination compared to individuals without these predispositions, might be expected to show worsened moods across a rest period (Zhou et al., 2020). However, while we did not experimentally include a rest condition (i.e., inactive control group) to directly address this, previous research with a similar sample suggests that a period of short rest can produce measurable changes in mood endorsement but does not result in EEG patterns as were observed in the present study (Tarrant, et al., 2018). The addition of a matched VR-based guided meditation group would have helped to better reveal comparative advantages and outcomes for one technology vs. the other. We have discussed our results in the context of separate research studies, but future research designs that directly compare AR with VR or traditional meditation practices will provide much more value and a clearer understanding of strengths and limitations of these applications.
In terms of sample limitations, our sample included a mix of individuals with and without previous meditation experience which may have resulted in non-representative (i.e., over-inflated) positivity in terms of the openness, engagement, and perceived value as a mental health and wellness coping tool. On average, the AR−NF group included individuals with more meditation experience and regular practice compared to the AR+NF group, in terms of the past six months of practice, but not weekly amounts. Research that examines longer term use of similar technology-assisted meditations, or that include and follow up measurements, will help to better understand whether existing familiarity or proficiency in meditation plays a role in the experience of meditation training with technological aids and what the enduring psychological and physiological outcomes are. That we evaluated the effects of a one-time brief meditation session is a limitation in some respects, but also provides promising evidence that even a rather brief meditation experience can lead to measurable mood changes. This is certainly a positive finding and bodes well for its quick and flexible application in real-world use as a potential mental health and mood regulation tool.
At least in terms of a brief single AR-guided meditation session, the addition of NF shows negligible differences improving self-reported mood and physiological states altering resting state brain activity. Both groups reported comparable improvements in mood. Additionally, both groups had similar patterns of change in brain activity states after the AR experience conducive to more relaxed, calm, and open psychological states, with parallel ratings of positive emotions related to the experience. We observed some trending differences in brain activity between groups, particularly with regards to gamma, in line with expectations given the NF protocol was gamma focused. Single meditation and neurofeedback sessions have been linked to acute transient changes in psychological and physiological states, but it is well known that longer lasting, trait level, psychological, and neural changes require substantial training efforts over longer periods of time than the participants were subjected to in this study.
Our results suggested that the addition of NF with AR provided a more engaging experience, which may have implications for maintaining training efforts and app use. With longer term, repeated use, or tightened NF parameters, conjunctive NF with AR may yield more divergent and lasting patterns of brain rhythm change, reflective of enduring changes in the underlying neural circuits and consolidation of the psychological benefits associated with long-term meditation practice. We believe that further scientific study of AR mental health promoting applications, with longer term training paradigms and exploration of different protocols, will help to uncover some of the neural and psychological mechanistic underpinnings from which these technological interactions can support and promote positive mental health outcomes. We hope that the results of this study and subsequent research along this vein will continue the advancement and optimization of AR/VR into mental health and wellness commercial and clinical settings.
The original contributions presented in the study are included in the article/Supplementary Material, further inquiries can be directed to the corresponding author.
The studies involving human participants were reviewed and approved by the Quorum Institutional Review Board, Seattle, WA. The patients/participants provided their written informed consent to participate in this study.
JT contributed to study conception, design, and data collection. JV performed statistical analysis, and results reporting. JV and JT co-wrote the manuscript. RJ organized the database, prepared tables, and assisted in manuscript organization. All authors contributed to manuscript revisions.
JT is contracted by StoryUp XR to assist in product development and assessment. Coauthor JV, who has no affiliation with StoryUp, was recruited as a third party consultant to conduct statistical analyses and assist in the interpretation and scientific publication of this data in order to reduce potential conflicts of interest.
The remaining author declares that the research was conducted in the absence of any commercial or financial relationships that could be construed as a potential conflict of interest.
Amihai, I., and Kozhevnikov, M. (2014). Arousal vs. relaxation: a comparison of the neurophysiological and cognitive correlates of Vajrayana and Theravada meditative practices. PLoS One 9 (7), e102990. doi:10.1371/journal.pone.0102990
Arvanitis, T. N., Petrou, A., Knight, J. F., Savas, S., Sotiriou, S., Gargalakos, M., et al. (2009). Human factors and qualitative pedagogical evaluation of a mobile augmented reality system for science education used by learners with physical disabilities. Pers. Ubiquitous Comput. 13 (3), 243–250. doi:10.1007/s00779-007-0187-7
Athanas, A. J., McCorrison, J. M., Smalley, S., Price, J., Grady, J., Campistron, J., et al. (2019). Association between improvement in baseline mood and long-term use of a mindfulness and meditation app: observational study. JMIR Ment. Health 6 (5), e12617. doi:10.2196/12617
Baumel, A., Muench, F., Edan, S., and Kane, J. M. (2019). Objective user engagement with mental health apps: systematic search and panel-based usage analysis. J. Med. Internet Res. 21 (9), e14567. doi:10.2196/14567
Baus, O., and Bouchard, S. (2014). Moving from virtual reality exposure-based therapy to augmented reality exposure-based therapy: a review. Front. Hum. Neurosci. 8, 112. doi:10.3389/fnhum.2014.00112
Benyousef, Y., Lesport, P., and Chassagnueux, A. (2017). The emergent role of virtual reality in the treatment of neuropsychiatric disease. Front. Neurosci. 11, 491. doi:10.3389/fnins.2017.00491
Bhayee, S., Tomaszewski, P., Lee, D. H., Moffat, G., Pino, L., Moreno, S., et al. (2016). Attentional and affective consequences of technology supported mindfulness training: a randomised, active control, efficacy trial. BMC Psychol. 4, 60. doi:10.1186/s40359-016-0168-6
Blankertz, B., Sannelli, C., Halder, S., Hammer, E. M., Kübler, A., Müller, K.-R., et al. (2010). Neurophysiological predictor of SMR-based BCI performance. Neuroimage 51 (4), 1303–1309. doi:10.1016/j.neuroimage.2010.03.022
Bonnstetter, R. J., Hebets, D., and Wigton, N. L. (2015). Frontal gamma asymmetry in response to soft skills stimuli: a pilot study. Neuroregulation 2 (2), 70–85. doi:10.15540/nr.2.2.70
Bouchard, S., Cote, S., and Richard, D. C. (2007). “Virtual reality applications for exposure,” in Handbook of exposure therapies. Editors D. C. Richard, and D. L. Lauterbach (San Diego, CA: Elsevier Inc.), 347–388.
Brown, K. W., and Ryan, R. M. (2003). The benefits of being present: mindfulness and its role in psychological well-being. J. Pers. Soc. Psychol. 84, 822–848. doi:10.1037/0022-3514.84.4.822
Cahn, B., and Polich, J. (2006). Meditation states and traits: EEG, ERP, and neuroimaging studies. Psychol. Bull. 132 (2), 180–211. doi:10.1037/0033-2909.132.2.180
Carissoli, C., Villani, D., and Riva, G. (2015). Does a meditation protocol supported by a mobile application help people reduce stress? suggestions from a controlled pragmatic trial. Cyberpsychol. Behav. Soc. Netw. 18 (1), 46–53. doi:10.1089/cyber.2014.0062
Carson, S. H., Peterson, J. B., and Higgins, D. M. (2005). Reliability, validity, and factor structure of the creative achievement questionnaire. Creativity Res. J. 17 (1), 37–50. doi:10.1207/s15326934crj1701_4
Cebolla, A., Herrero, R., Ventura, S., Miragall, M., Bellosta-Batalla, M., Llorens, R., et al. (2019). Putting oneself in the body of others: a pilot study on the efficacy of an embodied virtual reality system to generate self-compassion. Front. Psychol. 10, 1521. doi:10.3389/fpsyg.2019.01521
Chen, A. C., Feng, W., Zhao, H., Yin, Y., and Wang, P. (2008). EEG default mode network in the human brain: spectral regional field powers. Neuroimage 41, 561–574. doi:10.1016/j.neuroimage.2007.12.064
Chow, T., Javan, T., Ros, T., and Frewen, P. (2016). EEG dynamics of mindfulness meditation versus alpha neurofeedback: a sham-controlled study. Mindfulness 8 572–584. doi:10.1007/s12671-016-0631-8
Craig, A. D. B. (2009). How do you feel-now? the anterior insula and human awareness. Nat. Rev. Neurosci. 10 (1), 59–70. doi:10.1038/nrn2555
Dahl, C. J., Lutz, A., and Davidson, R. J. (2015). Reconstructing and deconstructing the self: cognitive mechanisms in meditation practice. Trends Cogn. Sci. 19 (9), 515–523. doi:10.1016/j.tics.2015.07.001
Davelaar, E. J., Barnby, J. M., Almasi, S., and Eatough, V. (2018). Differential subjective experiences in learners and non-learners in frontal alpha neurofeedback: piloting a mixed-method approach. Front. Hum. Neurosci. 12, 402–423. doi:10.3389/fnhum.2018.00402
Davidson, R. R. J. (1992). Anterior cerebral asymmetry and the nature of emotion. Brain Cogn. 20 (1), 125–151. doi:10.1016/0278-2626(92)90065-t
De Buck, S., Maes, F., Ector, J., Bogaert, J., Dymarkowski, S., Heidbüchel, H., et al. (2005). An augmented reality system for patient-specific guidance of cardiac catheter ablation procedures. IEEE Trans. Med. Imaging 24 (11), 1512–1524. doi:10.1109/TMI.2005.857661
Dennis, T. A., and Solomon, B. (2010). Frontal EEG and emotion regulation: electrocortical activity in response to emotional film clips is associated with reduced mood induction and attention interference effects. Biol. Psychol. 85, 456–464. doi:10.1016/j.biopsycho.2010.09.008
Eberth, J., and Sedlmeier, P. (2012). The Effects of mindfulness meditation: a meta-analysis. Mindfulness 3 (3), 174–189. doi:10.1007/s12671-012-0101-x
Engström, M., and Söderfeldt, B. (2010). Brain activation during compassion meditation: a case study. J. Altern. Complement. Med. 16 (5), 597–599. doi:10.1089/acm.2009.0309
Farb, N. A., Segal, Z. V., Mayberg, H., Bean, J., McKeon, D., Fatima, Z., et al. (2007). Attending to the present: mindfulness meditation reveals distinct neural modes of self-reference. Soc. Cogn. Affect. Neurosci. 2 (4), 313–322. doi:10.1093/scan/nsm030
Fell, J., Axmacher, N., and Haupt, S. (2010). From alpha to gamma: electrophysiological correlates of meditation-related states of consciousness. Med. Hypotheses 75 (2), 218–224. doi:10.1016/j.mehy.2010.02.025
Ferrarelli, F., Smith, R., Dentico, D., Riedner, B. A., Zennig, C., Benca, R. M., et al. (2013). Experienced mindfulness meditators exhibit higher parietal-occipital EEG gamma activity during NREM sleep. PLoS One 8 (8), e73417. doi:10.1371/journal.pone.0073417
Flett, J. A. M., Hayne, H., Riordan, B. C., Thompson, L. M., and Conner, T. S. (2019). Mobile mindfulness meditation: a randomised controlled trial of the effect of two popular apps on mental health. Mindfulness 10, 863–876. doi:10.1007/s12671-018-1050-9
Fox, K. C. R., Dixon, M. L., Nijeboer, S., Girn, M., Floman, J. L., Lifshitz, M., et al. (2016). Functional neuroanatomy of meditation: a review and meta-analysis of 78 functional neuroimaging investigations. Neurosci. Biobehav. Rev. 65, 208–228. doi:10.1016/j.neubiorev.2016.03.021
Fox, K. C. R., Nijeboer, S., Dixon, M. L., Floman, J. L., Ellamil, M., Rumak, S. P., et al. (2014). Is meditation associated with altered brain structure? a systematic review and meta-analysis of morphometric neuroimaging in meditation practitioners. Neurosci. Biobehav. Rev. 43, 48–73. doi:10.1016/j.neubiorev.2014.03.016
Gale, A., Edwards, J., Morris, P., and Forrester, D. (2001). Extraversion-introversion, neuroticism-stability, and EEG indicators of positive and negative empathic mood. Pers. Individ. Differ. 30, 449–461. doi:10.1016/S0191-8869(00)00036-2
Garrison, K. A., Santoyo, J. F., Davis, J. H., Thornhill, T. A., Kerr, C. E., and Brewer, J. A. (2013). Effortless awareness: using real time neurofeedback to investigate correlates of posterior cingulate cortex activity in meditators' self-report. Front. Hum. Neurosci. 7, 440. doi:10.3389/fnhum.2013.00440
Giglioli, I. A., Pallavicini, F., Pedroli, E., Serino, S., and Riva, G. (2015). Augmented reality: a brand new challenge for the assessment and treatment of psychological disorders. Comput. Math. Methods Med. 2015, 862942. doi:10.1155/2015/862942
Gilbert, P., and Procter, S. (2006). Compassionate mind training for people with high shame and self-criticism: overview and pilot study of a group therapy approach. Clin. Psychol. Psychother. 13, 353–379. doi:10.1002/cpp.507
Gorini, A., Pallavicini, F., Algeri, D., Repetto, C., Gaggioli, A., and Riva, G. (2010). Virtual reality in the treatment of generalized anxiety disorders. Stud. Health Technol. Inform. 154, 39–43. doi:10.3233/978-1-60750-561-7-39
Gotlib, I. H., Ranganath, C., and Rosenfeld, J. P. (1998). Frontal EEG alpha asymmetry, depression, and cognitive functioning. Cogn. Emot. 12, 449–478. doi:10.1080/026999398379673
Goyal, M., Singh, S., Sibinga, E. M., Gould, N. F., Rowland-Seymour, A., Sharma, R., et al. (2014). Meditation programs for psychological stress and well-being: a systematic review and meta-analysis. JAMA Intern. Med. 174 (3), 357–368. doi:10.1001/jamainternmed.2013.13018
Grossman, P., Niemann, L., Schmidt, S., and Walach, H. (2004). Mindfulness-based stress reduction and health benefits. A meta-analysis. J. Psychosom. Res. 57 (1), 35–43. doi:10.1016/S0022-3999(03)00573-7
Guger, C., Edlinger, G., Harkam, W., Niedermayer, I., and Pfurtscheller, G. (2003). How many people are able to operate an EEG-based brain-computer interface (BCI)? IEEE Trans. Neural Syst. Rehabil. Eng. 11 (2), 145–147. doi:10.1109/TNSRE.2003.814481
Hoaglin, D. C., and Iglewicz, B. (1987). Fine tuning some resistant rules for outlier labeling. J. Am. Stat. Assoc. 82, 1147–1149. doi:10.2307/2289392
Holm, S. (1979). A simple sequentially rejective multiple test procedure. Scand. J. Stat. 6 (2), 65–70.
Hutcherson, C. A., Seppala, E. M., and Gross, J. J. (2008). Loving-kindness meditation increases social connectedness. Emotion 8 (5), 720–724. doi:10.1037/a0013237
Kabat-Zinn, J. (2013). Full catastrophe living: using the wisdom of your body and mind to face stress, pain, and illness. New York, NY: Bantam Books.
Kerawalla, L., Luckin, R., Seljeflot, S., and Woolard, A. (2006). “Making it real”: exploring the potential of augmented reality for teaching primary school science. Virtual Real. 10 (3), 163–174. doi:10.1007/s10055-006-0036-4
Kop, W. J., Synowski, S. J., Newell, M. E., Schmidt, L. A., Waldstein, S. R., and Fox, N. A. (2011). Autonomic nervous system reactivity to positive and negative mood induction: the role of acute psychological responses and frontal electrocortical activity. Biol. Psychol. 86, 230–238. doi:10.1016/j.biopsycho.2010.12.003
Kovacevic, N., Ritter, P., Tays, W., Moreno, S., and McIntosh, A. R. (2015). ‘My virtual dream’: collective neurofeedback in an immersive art environment. PLoS One 10 (7), e0130129. doi:10.1371/journal.pone.0130129
Kroenke, K., Spitzer, R., and Williams, J. (2001). The PHQ-9: validity of a brief depression severity measure. J. Gen. Intern. Med. 16 (9), 606–613. doi:10.1046/j.1525-1497.2001.016009606.x
Laufs, H., Krakow, K., Sterzer, P., Eger, E., Beyerle, A., Salek-Haddadi, A., et al. (2003). Electroencephalographic signatures of attentional and cognitive default modes in spontaneous brain activity fluctuations at rest. Proc. Natl. Acad. Sci. U.S.A. 100, 11053–11058. doi:10.1073/pnas.1831638100
Laver, K. E., George, S., Thomas, S., Deutsch, J. E., and Crotty, M. (2015). Virtual reality for stroke rehabilitation. Cochrane Database Syst. Rev. 2, CD008349. doi:10.1002/14651858.CD008349.pub3
Lezak, M. D., Howieson, D. B., Bigler, E. D., and Tranel, D. (2012). Neuropsychological assessment. 5th Edn. New York, NY: Oxford University Press.
Lutz, A., Brefczynski-Lewis, J., Johnstone, T., and Davidson, R. J. (2008). Regulation of the neural circuitry of emotion by compassion meditation: effects of meditative expertise. PLoS One 3 (3), e1897. doi:10.1371/journal.pone.0001897
Lutz, A., Greischar, L. L., Rawlings, N. B., Ricard, M., and Davidson, R. J. (2004). Gamma synchrony during mental practice. Proc. Natl. Acad. Sci. U.S.A. 101 (46), 16369–16373. doi:10.1073/pnas.0407401101
Lutz, A., Slagter, H. A., Dunne, J. D., and Davidson, R. J. (2008). Attention regulation and monitoring in meditation. Trends Cogn. Sci. 12 (4), 163–169. doi:10.1016/j.tics.2008.01.005
Malbos, E., Boyer, L., and Lançon, C. (2013). Virtual reality in the treatment of mental disorders. Presse Med. 42, 1442–1452. [in French, with English summary]. doi:10.1016/j.lpm.2013.01.065
Mantini, D., Perrucci, M. G., Del Gratta, C., Romani, G. L., and Corbetta, M. (2007). Electrophysiological signatures of resting state networks in the human brain. Proc. Natl. Acad. Sci. U.S.A. 104, 13170–13175. doi:10.1073/pnas.0700668104
Maples-Keller, J., Bunnell, B., Kim, S., and Rothbaum, B. (2017). The use of virtual reality technology in the treatment of anxiety and other psychiatric disorders. Harv. Rev. Psychiatry 25 (3), 103–113. doi:10.1097/HRP.0000000000000138
Marzbani, H., Marateb, H. R., and Mansourian, M. (2016). Neurofeedback: a comprehensive review on system design, methodology and clinical applications. Basic Clin. Neurosci. 7 (2), 143–158. doi:10.15412/J.BCN.03070208
Milgram, P., and Kishino, F. (1994). A taxonomy of mixed reality visual displays. IEICE Trans. Inf. Syst. 12, 1321–1329.
Motraghi, T., Seim, R., Meyer, E., and Morissette, S. (2014). Virtual reality exposure therapy for the treatment of posttraumatic stress disorder: a methodological review using CONSORT guidelines. J. Clin. Psychol. 70 (3), 197–208. doi:10.1002/jclp.22051
Northoff, G., Heinzel, A., de Greck, M., Bermpohl, F., Dobrowolny, H., and Panksepp, J. (2006). Self-referential processing in our brain–a meta-analysis of imaging studies on the self. Neuroimage 31, 440–457. doi:10.1016/j.neuroimage.2005.12.002
Nyklíc, I., Mommersteeg, P. M. C., Van Beugen, S., and Ramakers, C. (2013). Mindfulness-based stress reduction and physiological activity during acute stress: a randomized controlled trial, Health Psychol. 32 (10), 1110–1113. doi:10.1037/a0032200
Oakes, T. R., Pizzagalli, D. A., Hendrick, A. M., Horras, K. A., Larson, C. L., Abercrombie, H. C., et al. (2004). Functional coupling of simultaneous electrical and metabolic activity in the human brain. Hum. Brain Mapp. 21, 257–270. doi:10.1002/hbm.20004
Olbrich, S., Sander, C., Matschinger, H., Mergl, R., Trenner, M., Schönknecht, P., et al. (2011). Brain and body: associations between EEG-vigilance and the autonomous nervous system activity during rest. J. Psychophysiol. 25 (4), 190–200. doi:10.1027/0269-8803/a000061
Ozbek, C. S., Giesler, B., and Dillmann, R. (2004). “Jedi training: playful evaluation of head-mounted augmented reality display systems,” in SPIE 5291, Stereoscopic Displays and Virtual Reality Systems XI, SanDiego, Calif, USA, May 21, 2004.
Pallavicini, F., and Bouchard, S. (2019). Editorial: assessing the therapeutic uses and effectiveness of virtual reality, augmented reality and video games for emotion regulation and stress management. Front. Psychol. 10, 2763. doi:10.3389/fpsyg.2019.02763
Pascual-Marqui, R. D. (2007). Discrete, 3D distributed, linear imaging methods of electric neuronal activity. Part 1: exact, zero error localization. arXiv:0710.3341.
Pascual-Marqui, R. D. (2002). Standardized low-resolution brain electromagnetic tomography (sLORETA): technical details. Methods Find. Exp. Clin. Pharmacol. 24, 5–12.
Potts, D., Loveys, K., Ha, H., Huang, S., Billinghurst, M., and Broadbent, E. (2019). “Zeng: AR neurofeedback for meditative mixed reality,” in C&C ‘19 Proceedings of the 2019 on Creativity and Cognition, New York: ACM, 583–590. May 13, 2019.
Raichle, M. E., MacLeod, A. M., Snyder, A. Z., Powers, W. J., Gusnard, D. A., and Shulman, G. L. (2001). A default mode of brain function. Proc. Natl. Acad. Sci. U.S.A. 98 (2), 676–682. doi:10.1073/pnas.98.2.676
Ramsøy, T. Z., Skov, M., Christensen, M. K., and Stahlhut, C. (2018). Frontal brain asymmetry and willingness to pay. Front. Neurosci. 12, 138. doi:10.3389/fnins.2018.00138
Riva, G., Baños, R. M., Botella, C., Mantovani, F., and Gaggioli, A. (2016). Transforming experience: the potential of augmented reality and virtual reality for enhancing personal and clinical change. Front. Psychiatry 7, 164. doi:10.3389/fpsyt.2016.00164
Saredakis, D., Szpak, A., Birckhead, B., Keage, H. A. D., Rizzo, A., and Loetscher, T. (2020). Factors associated with virtual reality sickness in head-mounted displays: a systematic review and meta-analysis. Front. Hum. Neurosci. 14, 96. doi:10.3389/fnhum.2020.00096
Seabrook, E., Kelly, R., Foley, F., Theiler, S., Thomas, N., Wadley, G., et al. (2020). Understanding how virtual reality can support mindfulness practice: mixed methods study. J. Med. Internet Res. 22 (3), e16106. doi:10.2196/16106
Sedlmeier, P., Eberth, J., Schwarz, M., Zimmermann, D., Haarig, F., Jaeger, S., et al. (2012). The psychological effects of meditation: a meta-analysis. Psychol. Bull. 138, 1139–1171. doi:10.1037/a0028168
Segal, Z. V., Williams, M. J., and Teasdale, J. D. (2013). Mindfulness-based cognitive therapy for depression. 2nd Edn. New York, NY: Guilford Press.
Silva, B. M., and Fernando, P. (2019). “Early prediction of cybersickness in virtual, augmented & mixed reality applications: a review,” in 2019 IEEE 5th international conference for convergence in technology (I2CT). Editors J. Y. C. Chen, and G. Fragomeni, Bombay, India, 29–31 March 2019 (IEEE), 1–6. doi:10.1109/I2CT45611.2019.9033650
Singer, T., Critchley, H. D., and Preuschoff, K. (2009). A common role of insula in feelings, empathy and uncertainty. Trends Cognit. Sci. 13 (8), 334. doi:10.1016/j.tics.2009.05.001
Singer, T., Seymour, B., O’Doherty, J., Kaube, H., Dolan, R. J., and Frith, C. D. (2004). Empathy for pain involves the affective but not sensory components of pain. Science 303 (5661), 1157–1162. doi:10.1126/science.1093535
Soos, I., Lane, A., Leibinger, E., Karasai, I., and Hamar, P. (2007). Validity of the Brunel mood scale for use with UK, Italian, and Hungarian athletes. J. Sports Sci. Med. 11 (1), 119–130.
Spitzer, R. L., Kroenke, K., Williams, J. B., and Löwe, B. (2006). A brief measure for assessing generalized anxiety disorder: the GAD-7. Arch. Intern. Med. 166 (10), 1092–1097. doi:10.1001/archinte.166.10.1092
Sutton, S. K., and Davidson, R. J. (1997). Prefrontal brain asymmetry: a biological substrate of the behavioral approach and inhibition systems. Psychol. Sci. 8 (3), 204–210. doi:10.1111/j.1467-9280.1997.tb00413.x
Tang, V., Poon, W. S., and Kwan, P. (2015). Mindfulness-based therapy for drug-resistant epilepsy. Neurology 85, 1100–1107. doi:10.1212/WNL.0000000000001967
Tang, Y.-Y., Ma, Y., Fan, Y., Feng, H., Wang, J., Feng, S., et al. (2009). Central and autonomic nervous system interaction is altered by short-term meditation. Proc. Natl. Acad. Sci. U.S.A. 106 (22), 8865–8870. doi:10.1073/pnas.0904031106
Tarrant, J. (2017a). Meditation interventions to rewire the brain: integrating neuroscience strategies for ADHD, anxiety, depression and PTSD. Eau Claire, WI: PESI Publishing and Media.
Tarrant, J. (2017b). “NeuroMeditation: an introduction and overview,” in Clinician’s companion to QEEG and Neurofeedback (annotated and with an introduction by J. Kiffer). Editors T. F. Collura, and J. A. Frederick (New York: Taylor & Francis).
Tarrant, J., and Cope, H. (2018). Combining frontal gamma asymmetry neurofeedback with virtual reality: a proof-of-concept case study. Neuroregulation 5 (2), 57–66. doi:10.15540/nr.5.2.57
Tarrant, J., Viczko, J., and Cope, H. (2018). Virtual reality for anxiety reduction demonstrated by quantitative EEG: a pilot study. Front. Psychol. 9, 1280. doi:10.3389/fpsyg.2018.01280
Tarrant, J. M., Raines, N., and Blinne, W. (2015). “The impact of meditation on twin hearts on psychological functioning and quantitative EEG: a comparison of experienced and novice meditators,” in 1st Pranic healing research and development conference, Bogota, Columbia. September.
Thompson, M., and Thompson, L. (2003). The neurofeedback book. Wheat Ridge, CO: Association for Applied Psychophysiology and Biofeedback.
Warden-Smith, J., Laboni, P., Olukogbon, K., Bointon, E. S., Cole, R. H., John, S. R., et al. (2017). Light and smell stimulus protocol reduced negative frontal EEG asymmetry and improved mood. Open Life Sci. 12, 51–61. doi:10.1515/biol-2017-0006
Waterworth, J., and Riva, G. (2014). Feeling present in the physical world and in computer-mediated environments. Basingstoke, United Kingdom: Palgrave Macmillan. doi:10.1057/9781137431677
Williams, J. M. G. (2008). Mindfulness, depression and modes of mind. Cognit. Ther. Res. 32, 721–733. doi:10.1007/s10608-008-9204-z
Zhou, H. X., Chen, X., Shen, Y. Q., Li, L., Chen, N. X., Zhu, Z. C., et al. (2020). Rumination and the default mode network: meta-analysis of brain imaging studies and implications for depression. Neuroimage 206, 116287. doi:10.1016/j.neuroimage.2019.116287
Keywords: augmented reality, meditation, neurofeedback, loving kindness meditation, electroencephalogram, mental health, apps and smartphones
Citation: Viczko J, Tarrant J and Jackson R (2021) Effects on Mood and EEG States After Meditation in Augmented Reality With and Without Adjunctive Neurofeedback. Front. Virtual Real. 2:618381. doi: 10.3389/frvir.2021.618381
Received: 16 October 2020; Accepted: 25 January 2021;
Published: 22 March 2021.
Edited by:
Daniel Thalmann, École Polytechnique Fédérale de Lausanne, SwitzerlandReviewed by:
Hideyuki Ando, Osaka University of Arts, JapanCopyright © 2021 Viczko, Tarrant and Jackson. This is an open-access article distributed under the terms of the Creative Commons Attribution License (CC BY). The use, distribution or reproduction in other forums is permitted, provided the original author(s) and the copyright owner(s) are credited and that the original publication in this journal is cited, in accordance with accepted academic practice. No use, distribution or reproduction is permitted which does not comply with these terms.
*Correspondence: Jeff Tarrant, amVmZkBuZXVyb21lZGl0YXRpb25pbnN0aXR1dGUuY29t
Disclaimer: All claims expressed in this article are solely those of the authors and do not necessarily represent those of their affiliated organizations, or those of the publisher, the editors and the reviewers. Any product that may be evaluated in this article or claim that may be made by its manufacturer is not guaranteed or endorsed by the publisher.
Research integrity at Frontiers
Learn more about the work of our research integrity team to safeguard the quality of each article we publish.