- 1Fruit Biology and Pathology Unit, University of Bordeaux, INRAE, Bordeaux, France
- 2Division of Virology, Department of Pathology, Addenbrookes Hospital, University of Cambridge, Cambridge, United Kingdom
- 3Istituto per la Protezione Sostenibile Delle Piante, CNR, Torino, Italy
- 4Institute for Experimental Virology, TWINCORE Centre for Experimental and Clinical Infection Research, a Joint Venture Between the Hannover Medical School (MHH) and the Helmholtz Centre for Infection Research (HZI), Hannover, Germany
- 5Department of Plant Pathology, University of California, Davis, Davis, CA, United States
- 6DOE Joint Genome Institute, Lawrence Berkeley National Laboratory, Berkeley, CA, United States
- 7Bioinformatics Group, Wageningen University, Wageningen, Netherlands
- 8Instituto de Patología Vegetal, Centro de Investigaciones Agropecuarias, Instituto Nacional de Tecnología Agropecuaria (IPAVE, CIAP, INTA), Córdoba, Argentina
- 9Unidad de Fitopatología y Modelización Agrícola, Consejo Nacional de Investigaciones Científicas y Técnicas, Córdoba, Argentina
- 10Department of Microbiology and Infection Control, Faculty of Medicine, Osaka Medical and Pharmaceutical University, Osaka, Japan
- 11University of Liège, Gembloux AgroBioTech - TERRA, Gembloux, Belgium
- 12Flanders Research Institute for Agriculture, Fisheries and Food, Plant Sciences Unit – Virology, Ghent, Belgium
- 13Department of Molecular Life Science, Tokai University School of Medicine, Isehara, Japan
- 14Department of Molecular Genetics, University of Toronto, Toronto, ON, Canada
- 15The Donnelly Centre for Cellular and Biomolecular Research, University of Toronto, Toronto, ON, Canada
- 16Institute of Technology, University of Tartu, Tartu, Estonia
- 17Ecology, Diagnostics and Genetic Resources of Agriculturally Important Viruses, Fungi and Phytoplasmas, Crop Research Institute, Prague, Czechia
- 18Department of Plant Protection, Faculty of Agrobiology, Food and Natural Resources Czech University of Life Sciences, Prague, Czechia
- 19Archaeal Virology Unit, Institut Pasteur, Université Paris Cité, CNRS, Paris, France
- 20Instituto de Biología Molecular y Celular de Plantas (IBMCP), Consejo Superior de Investigaciones Científicas (CSIC) - Universitat Politècnica de València (UPV), València, Spain
- 21ASTRE, CIRAD, INRAE, University of Montpellier, Montpellier, France
- 22Department of Electrical Engineering, City University of Hong Kong, Kowloon, Hong Kong SAR, China
- 23School of Agriculture, Meiji University, Kawasaki, Japan
- 24Research Centre for Bioscience and Nanoscience, Japan Agency for Marine-Earth Science and Technology (JAMSTEC), Yokosuka, Japan
- 25KU Leuven, Department of Microbiology, Immunology, and Transplantation, Rega Institute, Division of Clinical and Epidemiological Virology, Laboratory of Viral Metagenomics, Leuven, Belgium
- 26Department of Microbiology, Faculty of Agriculture and Forestry, University of Helsinki, Helsinki, Finland
- 27Department of Oceanography and Global Change, Centro Oceanográfico de Málaga (IEO-CSIC), Fuengirola, Spain
- 28Department of Microbiology and Immunology, University of Otago, Dunedin, New Zealand
- 29Department of Virology, Montpellier University Hospital, Montpellier, France
- 30Sydney Institute for Infectious Diseases, School of Medical Sciences, The University of Sydney, Sydney, NSW, Australia
- 31Molecular Virology Laboratory, ICAR-National Research Centre for Banana, Tiruchirappalli, Tamil Nadu, India
- 32College of Marine Science, University of South Florida, St Petersburg, FL, United States
- 33Bioinformatics Centre, SP Pune University, Pune, India
- 34High Performance Computing (HPC)-Medical & Bioinformatics Applications Group, Centre for Development of Advanced Computing, Pune, India
- 35Department of Botany and Plant Pathology, Oregon State University, Corvallis, OR, United States
- 36Paul G. Allen School for Global Health, Washington State University, Pullman, WA, United States
- 37Bioinformatics/High-Throughput Analysis, Faculty of Mathematics and Computer Science, Friedrich Schiller University Jena, Jena, Germany
- 38KwaZulu-Natal Research and Innovation Sequencing Platform (KRISP), UKZN, Durban, South Africa
- 39School of Laboratory Medicine and Medical Sciences, University of KwaZulu–Natal, Durban, South Africa
- 40Research Department, Flanders Marine Institute (VLIZ), InnovOcean Site, Ostend, Belgium
- 41Department of Microbiology and Biochemistry, Faculty of Sciences, Ghent University, Ghent, Belgium
- 42Laboratoire Ampère, École Centrale de Lyon, Écully, France
- 43Departments of Microbiology and Civil, Environmental, and Geodetic Engineering, and Center of Microbiome Science, Ohio State University, Columbus, OH, United States
- 44Centre for Pathogen Genomics, Department of Microbiology and Immunology, University of Melbourne, at the Doherty Institute for Infection and Immunity, Melbourne, VIC, Australia
- 45National Center for Biotechnology Information, National Library of Medicine, National Institutes of Health, Bethesda, MD, United States
- 46Latvian Biomedical Research and Study Centre, Riga, Latvia
- 47The Shmunis School of Biomedicine and Cancer Research, Tel Aviv University, Tel Aviv, Israel
Improved RNA virus understanding is critical to studying animal and plant health, and environmental processes. However, the continuous and rapid RNA virus evolution makes their identification and characterization challenging. While recent sequence-based advances have led to extensive RNA virus discovery, there is growing variation in how RNA viruses are identified, analyzed, characterized, and reported. To this end, an RdRp Summit was organized and a hybrid meeting took place in Valencia, Spain in May 2023 to convene leading experts with emphasis on early career researchers (ECRs) across diverse scientific communities. Here we synthesize key insights and recommendations and offer these as a first effort to establish a consensus framework for advancing RNA virus discovery. First, we need interoperability through standardized methodologies, data-sharing protocols, metadata provision and interdisciplinary collaborations and offer specific examples as starting points. Second, as an emergent field, we recognize the need to incorporate cutting-edge technologies and knowledge early and often to improve omic-based viral detection and annotation as novel capabilities reveal new biology. Third, we underscore the significance of ECRs in fostering international partnerships to promote inclusivity and equity in virus discovery efforts. The proposed consensus framework serves as a roadmap for the scientific community to collectively contribute to the tremendous challenge of unveiling the RNA virosphere.
1 Introduction
RNA viruses (Orthornavirae) are genetic elements with RNA-based genomes that replicate using their encoded RNA-dependent RNA polymerase (RdRp) and by hijacking their host’s cellular machinery. Progeny viruses are then transmitted to new hosts either vertically, or horizontally most often in protein-based viral particles that can sometimes be surrounded by a lipid envelope. Viruses are widely diverse, infect all life forms (1), and include many human pathogens of medical and epidemiological importance (2), as well as various species with strong deleterious impact in agriculture (3). Additionally, by infecting unicellular eukaryotic and prokaryotic life forms, RNA viruses play a role in shaping microbial ecosystems, from the oceans to the human gut (4–8).
Historically, RNA virus discovery and characterization relied on direct cultivation or isolation of the infective agents via experiments that are often laborious and inherently restricted to viruses infecting hosts amenable to laboratory cultivation or propagation. The procedures typically involve the concentration of infectious particles from symptomatic or diseased host cells or tissues, followed by various identification techniques like microscopy (imaging), neutralization (antibody), hemadsorption, hemagglutination and plaque assays, and animal, plant, tissue or cell culture inoculation. In most cases, isolated concentrated particles would then undergo (viral) RNA extraction and purification, followed by reverse transcription into cDNA and subsequent sequencing enabling further genomic investigations (phylogeny, genotyping, etc).
1.1 Recent developments in omic-based RNA virus discovery: more, bigger, faster is the new pace
The advent of the genomic era has gradually expanded RNA virus discovery beyond experimental cultivation and isolation methodologies. The substantial decrease in costs associated with high-throughput nucleic acid sequencing, coupled with advances in computational capacities for big data storage and processing greatly facilitates the development of RNA virus discovery projects through large-scale sequencing (omic-based).Importantly, this genomic-data-driven exploration of the RNA virosphere using computational tools offers a unique opportunity to bypass many biases and limitations of traditional approaches, and goes hand in hand with the growing recognition of global viral diversity in ecological systems as a whole, including public health and one health [“pandemic preparedness”, surveillance - (9)].
With an expanded diversity of environments sampled as well as a growing re-assessment of publicly available sequencing data and the continuous development of tools and resources, this field has experienced massive growth in recent years, with no signs of deceleration in sight (Figure 1) (8, 10–29).
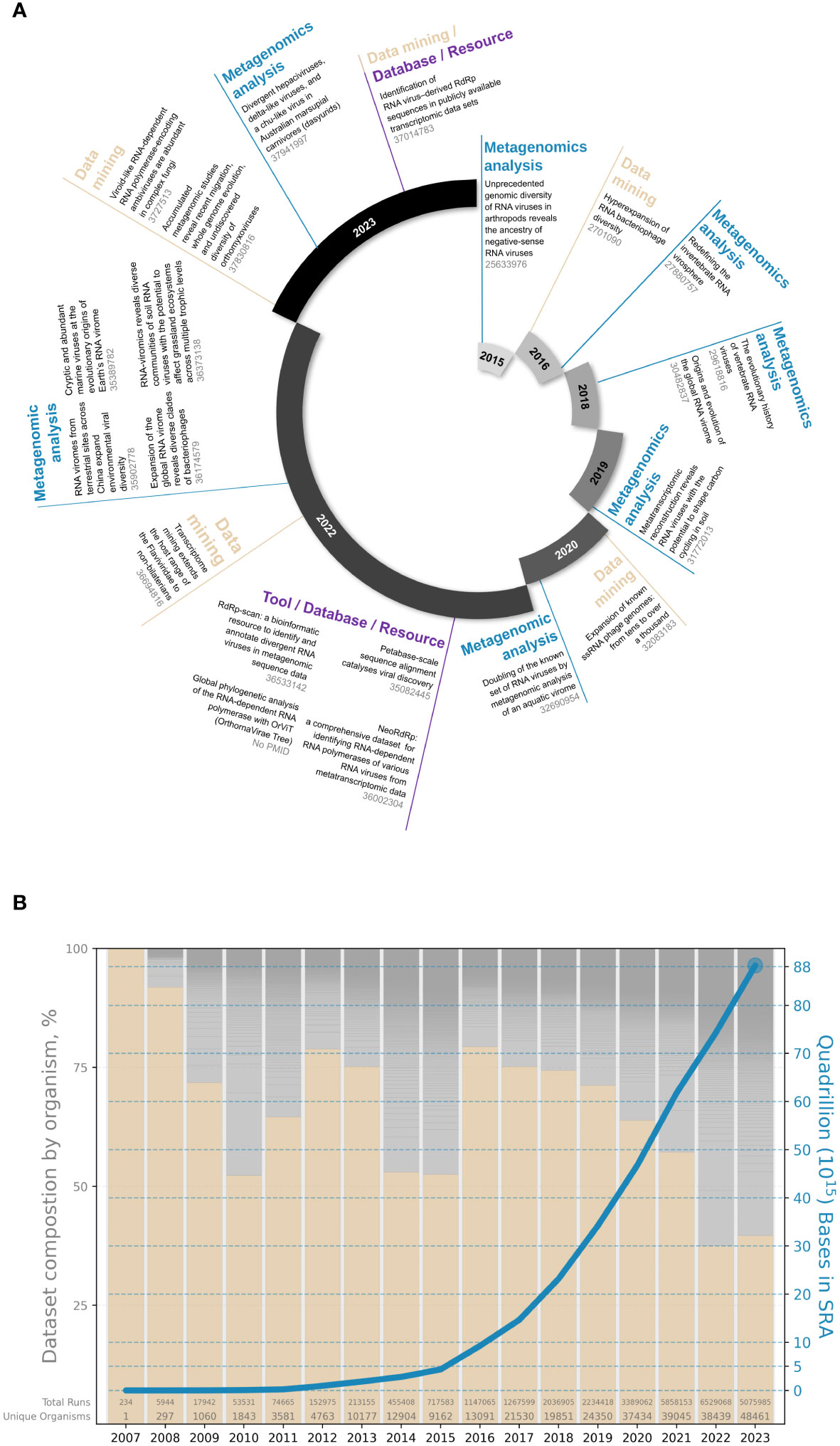
Figure 1 The recent expansion of the omic-based RNA virus discovery field. (A) Examples of RdRp-based viral metagenomic studies and tools (PMID are indicated in grey); (B) Multiplicity of publicly available sequences in the Sequence Read Archive based on human (pink bars) and other host (grey bars) composition (bar chart, left axis) and total cumulative number of bases (blue line, right axis). Data taken from SRA metadata available via BigQuery (nih-sra-datastore.sra.metadata).
1.2 Interdisciplinarity nature of the field limits data uniformity and standardization
RNA virus discovery is at the interface of various disciplines (i.e. virology, molecular, and structural biology, evolution, genomics, ecology, and computational sciences) and spans various fields within virology itself, each with its specific virus groups of interest, priorities, approaches, concepts, resources, etc. leading to apparent discrepancies throughout the scientific process. This heterogeneity manifests in both the experimental design, in the interpretation of the data and in the eventual conclusions and data sharing (novelty estimation, host inference, risk assessment, choice of data deposition location).
This growing lack of standardization directly and severely hampers interoperability - i.e. the ability to review, compare, reproduce, share, and build on each other’s efforts. For instance, what one study may consider as a new RNA virus group based on coat or movement protein sequence similarity, another study may consider part of an existing group using the RdRp comparison.
In recognition of these issues as detrimental to the advancement of the field, we recently held the first “RdRp summit” (https://rdrp.io/) - a discussion-centric event with the goals of fostering reproducibility, collaboration and interoperability in omics-derived RNA virus discovery. The event was attended by over 70 participants (60% in-person and 40% remotely), from 50 research institutions across the world. Most attendees were ECRs, with half of the participants listed as PhD students. To promote inclusion and exchanges between all participants, the meeting featured both open discussion sessions and traditional lectures, given by key bioinformaticians and experimentalists. Herein, we summarize the major insights and consensus that emerged from the workshop.
2 Current challenges in RNA virus discovery
2.1 Multiplicity of experimental and computational practices in RNA virus discovery workflows
The initial source and type of environment, the preservation, and preparation of RNA input have profound implications on the whole analysis and final RNA virus discovery. The input for RNA-virus metagenomic studies is often the total extractable RNA from an environmental, vector or host-associated sample (12, 17, 30). Alternatively, studies can focus on a size-selected fraction where host cells are excluded and virus-like-particles (VLPs) are enriched using filtration and/or centrifugation, otherwise known as viromics or virion-associated nucleic acids (VANA)-based sequencing (22, 31). Double-stranded RNA (dsRNA) purification can also be applied to total RNA samples to specifically target dsRNA virus genomes and replicative intermediate of single-stranded RNA (ssRNA) viruses instead of single-stranded transcripts and ribosomal RNAs (32). Beyond dsRNA enrichment, targeted approaches such as the Fragmented and primer-Ligated dsRNA Sequencing (FLDS) method also feature the ability to sequence both ends of the genome (33, 34), from which pairs of segmented or multipartite viral genomes can be searched (33). Deep sequencing of small RNAs (sRNAs) can also be advantageous to plant and mycovirus discovery, by using various sRNA size profiles depending on the organism for RNA genome assembly (35–40). On the other hand, untargeted total RNA extraction followed by RNA-seq better reflects the global sample complexity, including host and viral diversity, and can assist with host association, further answering ecological questions (41). However, the choice of the kit used for RNA extraction can substantially influence the downstream analysis (42). Prior to sequencing, classical treatments include genomic DNA digestion and either targeted ribosomal RNA (rRNA) depletion, or poly(A) enrichment steps prior to reverse transcription of RNA into cDNA. Along with the choice of sequencing platforms/technologies, those methods will directly impact the subsequent RNA virus findability and identification.
Current computational identification of RNA viruses from “-omic’’ data (mainly transcriptomic and metatranscriptomic sequencing, i.e. bulk RNA-seq of either a single organism or a community of organisms, respectively) is typically conducted via direct comparative approaches following quality control and filtering of raw reads and de novo assembly. The discovery of viruses through omics data primarily depends on identifying sequence similarities with existing RNA virus genomes, protein sequences, or protein sequence profiles. This is often accomplished using methods such as Hidden Markov Models (HMMs) or Position-Specific Scoring Matrices (PSSMs). Similarity is defined as a set of minimal statistical thresholds; typically established arbitrarily in each study and thus further stresses the need for standardized protocols.
Predominantly, the RdRp, which is the only protein shared by all known RNA viruses, is used as the marker gene for RNA virus identification (14). Virus RdRps share a right-hand-shape structure, typical of DNA/RNA polymerases, with a palm-based active site comprising several catalytic and structural motifs, which may require additional host factors to constitute a mature, complete replicase domain (43). Assignment of a query sequence as a potential viral RdRp usually requires the identification of at least the three “core” motifs, commonly referred to as A, B, and C, with the presence of any additional motifs increasing the reliability of the assignment and the presumed completeness of the analyzed sequence (44). The presence of these motifs (or roughly the region they occupy) is most often identified via sequence search engines (BLAST, DIAMOND, MMseqs, etc) (45–47) or profile-based approaches (HMM via HH-Suite or HMMER, or PSSM/PWM using PSI-BLAST or MEME) (48–50), often used in conjunction with public databases and repositories of RNA virus-derived RdRp e.g. subsetting NCBI nr, or custom databases like TSA-database derived RdRps (29), NeoRdRp (23), Palmscan (51), or RdRp-scan (18).
Furthermore, the enhancement of RNA extraction methods, sequencing technologies, and the rapid advancement in the development of new AI-based techniques, among other factors, is playing a crucial role in advancing RNA virus discovery. These advances facilitate the improved identification of potentially divergent and low-concentration viruses within overlooked environmental or host taxa. Nevertheless, these developments also reinforce the methodology gaps and heterogeneity between studies and severely limit interoperability.
2.2 Consequences of procedural inconsistency for comparative analyses across studies
Choices in both the experimental procedures and subsequent in silico analyses play a crucial role in how different studies handle, share, report, and reach conclusions regarding the suspected viral sequences in the corresponding data. Discussions held during this first RdRp summit pinpointed the global lack of procedure and good practice standards at every level (sampling, extraction, sequencing, read and contig processing, as well as data analysis, storage, submission, mining, survey, etc) (Figure 2). Ultimately, those differences in the computational and experimental aspects can constitute strong obstacles for the ability to adequately compare the results of different studies and it seemed important to first identify them.
Regarding RNA virus detection itself, there is a crucial lack of standard minimal alignment statistics (e-value, %ID, %coverage, etc). Also, the inconsistency of what is considered a genuine viral genome/viral hit/viral sequence versus a potential “false-positive” or contaminant poses a major risk of misinterpretation of results. The ability to identify and discriminate true replicative RNA virus signals from active or integrated viruses replicating via reverse-transcriptases (RT) (divergent other palm-like polymerases) (kingdom Pararnavirae), endogenous viral elements (EVEs), and non-viral hits or contaminants, is absolutely crucial in our field. However, there is currently no widely agreed-upon consensus about defining quality standards for viral sequences and how to ensure their identification as such. Compounding this issue is the lack of definition for real RNA-virus derived sequences that are either chimeric or misassembled, and thus are not likely to represent a functional infectious entity. Plus, expanding our knowledge of the RNA virosphere revealed an ever-increasing plurality of genome architectures and RdRp properties, which make it even harder to define one single rule for all of them. The recently-described divided RdRps confirmed and validated in silico (52–54), which are encoded by two distinct ORFs from separate genomic segments, constitute the best example of such unexpected plurality. Such challenges require continuous adaptation of standard practices and motivate to establish community-driven, up-to-date guidelines for RNA virus discovery.
Standardizing RNA virus detection would strongly require a community-built consensus about performance evaluation pipelines (sensitivity, recall, F1, precision, algorithm resilience, etc.), similarly to the ongoing efforts in microbial and DNA virus metagenomics (55–58). Directly linked to this, unequivocal agreements on the plurality of operational taxonomic unit (OTU) definitions, clustering thresholds, and minimal procedure for genome completeness estimation of novel and divergent viruses will help set gold standards for the scientific community (Figure 2).
The aforementioned considerations would also dramatically decrease common inconsistencies regarding the multiplicity of repositories that host the data as well as metadata associated with viral metagenomics projects deposited in standard databases. Indeed, one could note the major confusion between host and sample source, arbitrary taxonomy assignment and gene, protein, and genome annotations, the lack of information relating to sample preparation, sequencing, and computational analysis, the inadequacy of current tools for uploading viruses with divided RdRps or segmented genomes, or sequences with alternative START codons, and the inability for external users to revise/re-assess/edit/annotate the deposited metadata. All of which leads to an absurd rate of unclassified/unannotated sequences when dealing with remote homology searches.
Considering the rising scale of viral metagenomic studies and the pace of virus taxonomy expansion, such misannotations or mis-assignments in reference databases can have dramatic consequences when propagated to new studies and data submission, and drastically limit the scope and efficiency of data mining projects, yet are increasingly essential in our field.
3 Solutions and future perspectives
While incredibly valuable, the interdisciplinarity in the RNA virus discovery field also requires concerted efforts from researchers to build connections between those communities, share, and adapt our respective practices, tools, vocabulary, terminologies, and standards to fit in with everyone’s domain language.
To tackle the first challenge consisting of establishing minimum standards for RNA virus genome (or viral RdRp) annotations, cut-off for parameters (alignment scores, e-values, query and reference lengths, etc.) need to be agreed upon when comparing candidate sequences to known valid RdRp sequence database vs. decoy - (RT)-like - databases and sets of unclassified/unannotated sequences. Annotation could then be automatically assigned based on these comparison scores (true complete RdRp vs RT-like hits vs unclassified/unknown). Discussions and lectures at the summit also highlighted the importance of integrating additional procedures into classical workflows such as placement within phylogenies, genomic context scan (untranslated regions, RNA structure, nucleotide and kmer composition among others), and structural homology assessment using cutting-edge AI-based prediction tools (59, 60), essential for distant homology detection or validation. While some quality criteria and cut-offs can easily be built in, some others may be very challenging. Defining boundaries for the RdRp gene (minimal length to describe a RdRp, structural attributes, minimal presence of the catalytic motifs, presence/absence of additional domains, such as Nidovirus RdRp associated nucleotidyl transferase domain - NiRAN) remains a complex task and requires expertise and extended knowledge of the viral strain (61–63).
In addition, the standardization effort should also promote the integration into the standard discovery pipelines of the most recent and state-of-the-art concepts in RNA virology such as the search for potential additional segments (64), the screen for divided RdRp (52–54), etc.
Another challenge consists of homogenization of manual and automatic clusterization procedures and taxonomic assignment of viral-like sequences. Formal virus classification by the International Committee on Taxonomy of Viruses (ICTV) plays a vital role in providing a reference language for scientists to communicate, collaborate, and share knowledge about viruses. By coupling the robust, updated, and standardized ICTV classification framework with the power of omic-based RNA virus discovery, we can collectively improve our description and global understanding of RNA virus diversity (65).
Concretely, all these proposals will be pursued by the RdRp summit community with different initiatives that are intended to be maintained and updated over time by the community members. In particular, the community will focus on:
I) Building a central infrastructure for the RNA virus discovery community, which would work as a central repository for data and knowledge.
II) Creating a curated database with automated quality scores for data deposition based on the information provided. The database should be community-driven and open to feedback from end users to aid further curation.
III) Consolidating state-of-the-art experimental and computational resources and knowledge to ensure the best up-to-date practices among the community (pipelines, scripts, protocols, glossary, guides, international journal club). Among others, this could consist of recommended workflows for the challenging identification of novel/remote RdRp, the Do’s and Don’ts of annotating new, uncultivated RNA virus genomes, and metadata recommendations for RNA viruses.
IV) In the same manner as the European Virus Bioinformatics Center (https://evbc.uni-jena.de/), particular effort will be put into enhancing the communication and uniting our expanding community into one single spot through a potential membership system, forums, round tables, workshops, online chat channels, etc.
To conclude, the major consensus that emerged from the rich discussions led during this first RdRp summit lies in the current lack of interoperability and reproducibility in our field but also the possible concrete solutions to tackle these obstacles (summarized in Figure 2).
The omic-based RNA virus discovery community, as an open science-to-society-oriented community, should be aware of its roles and responsibilities to make its scope as transparent and accessible as possible. Through the collective development of a user-friendly open platform, we aim to build a solid foundation for communicating, sharing, and performing comparable analyses using optimal and state-of-the-art tools across a wide array of biological contexts by reaching the broadest audience possible. With similar issues faced in microbial metagenomics and omic-based DNA virus discovery, we also intend to inspire from the emerging solutions and infrastructures being developed in these related fields and learn from their experiences in tackling these challenges (e.g. 66–68).
We believe these efforts will lay the groundwork to promote ECRs insertion into the community, best practices and repeatability, and ultimately ensure the best future for our exploration of the RNA virosphere.
Data availability statement
The original contributions presented in the study are included in the article/supplementary material. Further inquiries can be directed to the corresponding author.
Author contributions
JC: Writing – original draft, Writing – review & editing, Conceptualization, Project administration. IO: Writing – original draft, Writing – review & editing, Visualization. MF: Writing – original draft, Writing – review & editing. LC: Writing – original draft, Writing – review & editing, Visualization. LH: Writing – original draft, Writing – review & editing. SR: Writing – original draft, Writing – review & editing. AK: Writing – original draft, Writing – review & editing. HD: Writing – original draft, Writing – review & editing. SS: Writing – original draft, Writing – review & editing. RT: Writing – original draft, Writing – review & editing. SN: Writing – original draft, Writing – review & editing. ABa: Writing – original draft, Writing – review & editing. AA: Writing – review & editing. NB: Writing – review & editing. KB: Writing – review & editing. KBM: Writing – review & editing. ABu: Writing – review & editing. AC: Writing – review & editing. FC: Writing – review & editing. GC: Writing – review & editing. YC: Writing – review & editing. LDC: Writing – review & editing. TD: Writing – review & editing. GD: Writing – review & editing. JD: Writing – review & editing. SG: Writing – review & editing. EH: Writing – review & editing. FJ: Writing – review & editing. DK: Writing – review & editing. SL: Writing – review & editing. SMK: Writing – review & editing. JM: Writing – review & editing. YN: Writing – review & editing. AO: Writing – review & editing. MR: Writing – review & editing. RR: Writing – review & editing. SS: Writing – review & editing. SS: Writing – review & editing. JS: Writing – review & editing. HS: Writing – review & editing. ES: Writing – review & editing. MS: Writing – review & editing. YS: Writing – review & editing. MW: Writing – review & editing. YIW: Writing – review & editing. NZ: Writing – review & editing. UN: Writing – original draft, Writing – review & editing, Conceptualization, Supervision.
Funding
The author(s) declare financial support was received for the research, authorship, and/or publication of this article. JC was supported by the French National Research Agency (ANR-21-CE35-0009). UN was supported by the European Research Council (ERC-AdG 787514) and by a fellowship from the Edmond J. Safra Center for Bioinformatics at Tel Aviv University, and by fellowship from the Shmunis School of Biomedicine and Cancer Research. The work conducted by the U.S. Department of Energy Joint Genome Institute (S.R.) (https://ror.org/04xm1d337), a DOE Office of Science User Facility, is supported by the Office of Science of the U.S. Department of Energy operated under Contract No. DE-AC02-05CH11231. AB was supported by the postdoctoral fellowship from Fondation Recherche Médicale (FRM) and Canadian Institutes for Health Research (PJT-496709). ABu was supported by a postdoctoral fellowship from Fondation Recherche Médicale (FRM). KBM was supported by the Ministry of Agriculture of the Czech Republic, institutional support (MZE-RO0423). RT was funded by the Belgian Federal Public Service Health, Food Chain Safety and Environment through the contract (GenoPREDICT - RF 22/635). ES was supported by Marie Sklodowska-Curie fellowship “DIVOBIS” awarded by Horizon Europe 2022. SN was supported by KAKENHI Grant-in-Aid for Scientific Research on Innovative Areas 16H06429, 16K21723, 19H04843 and by JST CREST JPMJCR20H6. YIW was supported by intramural funds of the US Department of Health and Human Services (National Institutes of Health, National Library of Medicine). EH was supported by AIR@InnoHK administered by the Innovation and Technology Commision, Hong Kong Special Administrative Region, China. AO-B was supported by a National Health and Medical Research Council (NHMRC) Investigator Grant (GNT2017197) awarded to Prof. Edward C. Holmes. YS was supported by Hong Kong Research Grants Council (RGC) General Research Fund (GRF) (11206819, 11217521) and Hong Kong Innovation and Technology Fund (ITF) MRP/071/20X. RR was supported by funding to Verena (viralemergence.org) from the U.S. National Science Foundation, including NSF BII 2021909 and NSF BII 2213854 and by a Chan Zuckerberg Initiative (CZI) Global Voice Travel Award from CZI, Redwood City, California, USA. YC and YN were supported by JSPS KAKENHI Grant Number JP22H05714. YC was supported by JSPS KAKENHI Grant Number JP23KJ1995. TD was supported by the Research Council of Finland grant 330977 and the Kone Foundation grant. FM was supported by a Chan Zuckerberg Initiative (CZI) Global Voice Travel Award from CZI, Redwood City, California, USA. DK and AK were supported by the Graduate School Experimental Plant Sciences (project V-GENE), LDC was supported by the Research Foundation Flanders (11L1323N). IO and KB were supported by Wellcome Trust 220814/Z/20/Z awarded to Prof Andrew Firth. HS was supported by Flanders Marine Institute (VLIZ). AC was supported by Ministerio de Ciencia e Innovación of Spain and FEDER grant PID2020-116008GB-I00 awarded to Marcos de la Peña. JS was supported by the South African Medical Research Council (SAMRC) with funds received from the National Department of Health. MS was supported by the US National Science Foundation awards #OCE1829831 and ABI#2149505. FC and SG were supported by the French Direction Générale de l’Alimentation (grant C-2023-016). LH was supported by the U.S. Department of Energy (DOE), Office of Science, Office of Biological and Environmental Research (BER), Genomic Science Program, award number DE-SC0023127 grant to Joanne B. Emerson, grant PI Sydney Glassman. SS was supported by KAKENHI Grant-in-Aid for Scientific Research (22K14999).
Acknowledgments
We would like to thank Amy Heather Fitzpatrick, Anastasia Gulyaeva, Chien-Fu Wu, Heli Mönttinen, Liubov Chuprikova, Fabiana Neves, Nikolay Simankov, Noriko Cassman, Nuria Fontdevila Pareta, Rebecca Grimwood, Stephanie Waller, Zivile Buivydaite, Alexander Allman, Artem Baidaliuk, Benjamin Lee, Cadhla Firth, Chuan Cao, Clarence Le, Luca Nishimura, Dehan Cai, Manja Marz, Elizabeth Fahsbender, Gabriel Lencioni Lovate, Hassan Z. A. Ishag, Isa de Vries, Janelle Wierenga, Javier Rodriguez-Grille, Jordan Taylor, Katarina Bačnik, Katrina Kalantar, Lauren Lim, Mark Paul S. Rivarez, Nina A. H. Madsen, Nolwenn Dheilly, Olve Peersen, Robert Edgar, Sandra Triebel, Satyabrata Satapathy, Sung won Lim, Xubo Tang and Yuanyuan Zhang for their precious contribution to the RdRp summit and the fruitful exchanges. We are also thankful to the sponsors of the first RdRp summit, the Chan-Zuckerberg Initiative (CZI) and Frontiers in Virology, for their support.
Conflict of interest
The authors declare that the research was conducted in the absence of any commercial or financial relationships that could be construed as a potential conflict of interest.
The author(s) declared that they were an editorial board member of Frontiers, at the time of submission. This had no impact on the peer review process and the final decision.
Publisher’s note
All claims expressed in this article are solely those of the authors and do not necessarily represent those of their affiliated organizations, or those of the publisher, the editors and the reviewers. Any product that may be evaluated in this article, or claim that may be made by its manufacturer, is not guaranteed or endorsed by the publisher.
References
1. Dominguez-Huerta G, Wainaina JM, Zayed AA, Culley AI, Kuhn JH, Sullivan MB. The RNA virosphere: How big and diverse is it? Environ Microbiol. (2023) 25:209–15. doi: 10.1111/1462-2920.16312
2. Jones KE, Patel NG, Levy MA, Storeygard A, Balk D, Gittleman JL, et al. Global trends in emerging infectious diseases. Nature. (2008) 451:990–3. doi: 10.1038/nature06536
3. Savary S, Willocquet L, Pethybridge SJ, Esker P, McRoberts N, Nelson A. The global burden of pathogens and pests on major food crops. Nat Ecol Evol. (2019) 3:430–9. doi: 10.1038/s41559-018-0793-y
4. Lang AS, Rise ML, Culley AI, Steward GF. RNA viruses in the sea. FEMS Microbiol Rev. (2009) 33:295–323. doi: 10.1111/j.1574-6976.2008.00132.x
5. Steward GF, Culley AI, Mueller JA, Wood-Charlson EM, Belcaid M, Poisson G. Are we missing half of the viruses in the ocean? ISME J. (2013) 7:672–9. doi: 10.1038/ismej.2012.121
6. Liang G, Bushman FD. The human virome: assembly, composition and host interactions. Nat Rev Microbiol. (2021) 19:514–27. doi: 10.1038/s41579-021-00536-5
7. Dominguez-Huerta G, Wainaina JM, Zayed AA, Culley AI, Kuhn JH, Sullivan MB, et al. The RNA virosphere: How big and diverse is it? Environ Microbiol. (2022) 25:209–15. doi: 10.1111/1462-2920.16312
8. Neri U, Wolf YI, Roux S, Camargo AP, Lee B, Kazlauskas D, et al. Expansion of the global RNA virome reveals diverse clades of bacteriophages. Cell. (2022) 185:4023–37.e18. doi: 10.1016/j.cell.2022.08.023
9. Keusch GT, Amuasi JH, Anderson DE, Daszak P, Eckerle I, Field H, et al. Pandemic origins and a One Health approach to preparedness and prevention: Solutions based on SARS-CoV-2 and other RNA viruses. Proc Natl Acad Sci USA. (2022) 119:e2202871119. doi: 10.1073/pnas.2202871119
10. Li C, Shi M, Tian J, Lin X, Kang Y, Chen L, et al. Unprecedented genomic diversity of RNA viruses in arthropods reveals the ancestry of negative-sense RNA viruses. Elife. (2015):e05378. doi: 10.7554/eLife.05378
11. Krishnamurthy SR, Janowski AB, Zhao G, Barouch D, Wang D. Hyperexpansion of RNA bacteriophage diversity. PLoS Biol. (2016) 14:1–17. doi: 10.1371/journal.pbio.1002409
12. Shi M, Lin X-D, Tian J-H, Chen L-J, Chen X, Li C-X, et al. Redefining the invertebrate RNA virosphere. Nature. (2016) 540:539–43. doi: 10.1038/nature20167
13. Shi M, Lin X-D, Chen X, Tian J-H, Chen L-J, Li K, et al. The evolutionary history of vertebrate RNA viruses. Nature. (2018) 556:197–202. doi: 10.1038/s41586-018-0012-7
14. Wolf YI, Kazlauskas D, Iranzo J, Lucía-Sanz A, Kuhn JH, Krupovic M, et al. Origins and evolution of the global RNA virome. MBio. (2018) 9:e02329–18. doi: 10.1128/mBio.02329-18
15. Starr EP, Nuccio EE, Pett-Ridge J, Banfield JF, Firestone MK. Metatranscriptomic reconstruction reveals RNA viruses with the potential to shape carbon cycling in soil. Proc Natl Acad Sci U S A. (2019) 116:25900–8. doi: 10.1073/pnas.1908291116
16. Callanan J, Stockdale SR, Shkoporov A, Draper LA, Ross RP, Hill C. Expansion of known ssRNA phage genomes: From tens to over a thousand. Sci Adv. (2020) 6:eaay5981. doi: 10.1126/sciadv.aay5981
17. Wolf YI, Silas S, Wang Y, Wu S, Bocek M, Kazlauskas D, et al. Doubling of the known set of RNA viruses by metagenomic analysis of an aquatic virome. Nat Microbiol. (2020) 5:1262–70. doi: 10.1038/s41564-020-0755-4
18. Charon J, Buchmann JP, Sadiq S, Holmes EC. RdRp-scan: A bioinformatic resource to identify and annotate divergent RNA viruses in metagenomic sequence data. Virus Evol. (2022) 8:1–15. doi: 10.1093/ve/veac082
19. Chen YM, Sadiq S, Tian JH, Chen X, Lin XD, Shen JJ, et al. RNA viromes from terrestrial sites across China expand environmental viral diversity. Nat Microbiol. (2022) 7:1312–23. doi: 10.1038/s41564-022-01180-2
20. Cheng D-Q, Kolundžija S, Lauro FM. Global phylogenetic analysis of the RNA-dependent RNA polymerase with OrViT (OrthornaVirae Tree). Front Virol. (2022) 2:981177. doi: 10.3389/fviro.2022.981177
21. Edgar RC, Taylor J, Lin V, Altman T, Barbera P, Meleshko D, et al. Petabase-scale sequence alignment catalyses viral discovery. Nature. (2022) 602:142–7. doi: 10.1038/s41586-021-04332-2
22. Hillary LS, Adriaenssens EM, Jones DL, McDonald JE. RNA-viromics reveals diverse communities of soil RNA viruses with the potential to affect grassland ecosystems across multiple trophic levels. ISME Commun. (2022) 2:1–10. doi: 10.1038/s43705-022-00110-x
23. Sakaguchi S, Urayama SI, Takaki Y, Hirosuna K, Wu H, Suzuki Y, et al. NeoRdRp: A comprehensive dataset for identifying RNA-dependent RNA polymerases of various RNA viruses from metatranscriptomic data. Microbes Environ. (2022) 37:1–7. doi: 10.1264/JSME2.ME22001
24. Zayed AA, Wainaina JM, Dominguez-Huerta G, Pelletier E, Guo J, Mohssen M, et al. Cryptic and abundant marine viruses at the evolutionary origins of Earth’s RNA virome. Science. (2022) 376:156–62. doi: 10.1126/SCIENCE.ABM5847
25. Chong LC, Lauber C. Viroid-like RNA-dependent RNA polymerase-encoding ambiviruses are abundant in complex fungi. Front Microbiol. (2023) 14:1144003. doi: 10.3389/fmicb.2023.1144003
26. Dudas G, Batson J. Accumulated metagenomic studies reveal recent migration, whole genome evolution, and undiscovered diversity of orthomyxoviruses. J Virol. (2023) 97:1–15. doi: 10.1128/jvi.01056-23
27. Harvey E, Mifsud JCO, Holmes EC, Mahar JE. Divergent hepaciviruses, delta-like viruses, and a chu-like virus in Australian marsupial carnivores (dasyurids). Virus Evol. (2023) 9:1–14. doi: 10.1093/ve/vead061
28. Mifsud JCO, Costa VA, Petrone ME, Marzinelli EM, Holmes EC, Harvey E. Transcriptome mining extends the host range of the Flaviviridae to non-bilaterians. Virus Evol. (2023) 9:1–17. doi: 10.1093/ve/veac124
29. Olendraite I, Brown K, Firth AE. Identification of RNA virus-derived RdRp sequences in publicly available transcriptomic data sets. Mol Biol Evol. (2023) 40:1–24. doi: 10.1093/molbev/msad060
30. Ni XB, Cui XM, Liu JY, Ye RZ, Wu YQ, Jiang JF, et al. Metavirome of 31 tick species provides a compendium of 1,801 RNA virus genomes. Nat Microbiol. (2023) 8:162–73. doi: 10.1038/s41564-022-01275-w
31. Ma Y, Marais A, Lefebvre M, Theil S, Svanella-Dumas L, Faure C, et al. Phytovirome analysis of wild plant populations: comparison of double-stranded RNA and virion-associated nucleic acid metagenomic approaches. J Virol. (2019) 94:e01462–19. doi: 10.1128/jvi.01462-19
32. Decker CJ, Steiner HR, Hoon-Hanks LL, Morrison JH, Haist KC, Stabell AC, et al. DsRNA-seq: Identification of viral infection by purifying and sequencing dsRNA. Viruses. (2019) 11:1–21. doi: 10.3390/v11100943
33. Urayama S, Takaki Y, Nunoura T. FLDS: A comprehensive dsRNA sequencing method for intracellular RNA virus surveillance. Microbes Environ. (2016) 31:33–40. doi: 10.1264/jsme2.me15171
34. Hirai M, Takaki Y, Kondo F, Horie M, Urayama SI, Nunoura T. RNA viral metagenome analysis of subnanogram dsRNA using fragmented and primer ligated dsRNA sequencing (FLDS). Microbes Environ. (2021) 36:1–6. doi: 10.1264/jsme2.ME20152
35. Kreuze JF, Perez A, Untiveros M, Quispe D, Fuentes S, Barker I, et al. Complete viral genome sequence and discovery of novel viruses by deep sequencing of small RNAs: A generic method for diagnosis, discovery and sequencing of viruses. Virology. (2009) 388:1–7. doi: 10.1016/j.virol.2009.03.024
36. Vainio EJ, Jurvansuu J, Streng J, Rajamäki ML, Hantula J, Valkonen JPT. Diagnosis and discovery of fungal viruses using deep sequencing of small RNAs. J Gen Virol. (2015) 96:714–25. doi: 10.1099/jgv.0.000003
37. Nerva L, Ciuffo M, Vallino M, Margaria P, Varese GC, Gnavi G, et al. Multiple approaches for the detection and characterization of viral and plasmid symbionts from a collection of marine fungi. Virus Res. (2016) 219:22–38. doi: 10.1016/j.virusres.2015.10.028
38. Zheng Y, Gao S, Padmanabhan C, Li R, Galvez M, Gutierrez D, et al. VirusDetect: An automated pipeline for efficient virus discovery using deep sequencing of small RNAs. Virology. (2017) 500:130–8. doi: 10.1016/j.virol.2016.10.017
39. Adalia EJM, Diez JJ, Fernández MM, Vainio JHEJ. Characterization of small RNAs originating from mitoviruses infecting the conifer pathogen Fusarium circinatum. Arch Virol. (2018) 163:1009–18. doi: 10.1007/s00705-018-3712-2
40. Raco M, Vainio EJ, Sutela S, Eichmeier A, Hakalová E, Jung T, et al. High diversity of novel viruses in the tree pathogen Phytophthora castaneae revealed by high-throughput sequencing of total and small RNA. Front Microbiol. (2022) 13:911474. doi: 10.3389/fmicb.2022.911474
41. Coclet C, Sorensen PO, Karaoz U, Wang S, Brodie EL, Eloe-Fadrosh EA, et al. Virus diversity and activity is driven by snowmelt and host dynamics in a high-altitude watershed soil ecosystem. Microbiome. (2023) 11:1–19. doi: 10.1186/s40168-023-01666-z
42. Hjelmsø MH, Hellmér M, Fernandez-Cassi X, Timoneda N, Lukjancenko O, Seidel M, et al. Evaluation of methods for the concentration and extraction of viruses from sewage in the context of metagenomic sequencing. PLoS One. (2017) 12:1–17. doi: 10.1371/journal.pone.0170199
43. Te Velthuis AJW. Common and unique features of viral RNA-dependent polymerases. Cell Mol Life Sci. (2014) 71:4403–20. doi: 10.1007/s00018-014-1695-z
44. Poch O, Sauvaget I, Delarue M, Tordo N. Identification of four conserved motifs among the RNA-dependent polymerase encoding elements. EMBO J. (1989) 8:3867. doi: 10.1002/embj.1989.8.issue-12
45. Camacho C, Coulouris G, Avagyan V, Ma N, Papadopoulos J, Bealer K, et al. BLAST+: architecture and applications. BMC Bioinf. (2009) 10:1–9. doi: 10.1186/1471-2105-10-421
46. Buchfink B, Xie C, Huson DH. Fast and sensitive protein alignment using DIAMOND. Nat Methods. (2015) 12:59–60. doi: 10.1038/nmeth.3176
47. Steinegger M, Söding J. MMseqs2 enables sensitive protein sequence searching for the analysis of massive data sets. Nat Biotechnol. (2017) 35:1026–8. doi: 10.1038/nbt.3988
48. Altschul SF, Madden TL, Schäffer AA, Zhang J, Zhang Z, Miller W, et al. Gapped BLAST and PSI-BLAST: a new generation of protein database search programs. Nucleic Acids Res. (1997) 25:3389–402. doi: 10.1093/genetics/156.4.1997
49. Eddy SR. Accelerated profile HMM searches. PloS Comput Biol. (2011) 7:e1002195. doi: 10.1371/journal.pcbi.1002195
50. Steinegger M, Meier M, Mirdita M, Vöhringer H, Haunsberger SJ, Söding J. HH-suite3 for fast remote homology detection and deep protein annotation. BMC Bioinf. (2019) 20:1–15. doi: 10.1186/s12859-019-3019-7
51. Babaian A, Edgar R. Ribovirus classification by a polymerase barcode sequence. PeerJ. (2022) 10:e14055. doi: 10.7717/PEERJ.14055
52. Sutela S, Forgia M, Vainio EJ, Chiapello M, Daghino S, Vallino M, et al. The virome from a collection of endomycorrhizal fungi reveals new viral taxa with unprecedented genome organization. Virus Evol. (2020) 6:veaa076. doi: 10.1093/VE/VEAA076
53. Chiba Y, Oiki S, Yaguchi T, Urayama SI, Hagiwara D. Discovery of divided RdRp sequences and a hitherto unknown genomic complexity in fungal viruses. Virus Evol. (2021) 7:1–11. doi: 10.1093/ve/veaa101
54. Chiba Y, Oiki S, Zhao Y, Nagano Y, Urayama SI, Hagiwara D. Splitting of RNA-dependent RNA polymerase is common in Narnaviridae: Identification of a type II divided RdRp from deep-sea fungal isolates. Virus Evol. (2021) 7:1–9. doi: 10.1093/ve/veab095
55. Sczyrba A, Hofmann P, Belmann P, Koslicki D, Janssen S, Dröge J, et al. Critical Assessment of Metagenome Interpretation—a benchmark of metagenomics software. Nat Methods. (2017) 14:1063–71. doi: 10.1038/nmeth.4458
56. Meyer F, Hofmann P, Belmann P, Garrido-Oter R, Fritz A, Sczyrba A, et al. AMBER: assessment of metagenome BinnERs. Gigascience. (2018) 7:1–8. doi: 10.1093/gigascience/giy069
57. Pratama AA, Bolduc B, Zayed AA, Zhong Z, Guo J, Vik DR, et al. Expanding standards in viromics: in silico evaluation of dsDNA viral genome identification, classification, and auxiliary metabolic gene curation. PeerJ. (2021) 9:e11447. doi: 10.7717/peerj.11447
58. Ho SFS, Wheeler NE, Millard AD, van Schaik W. Gauge your phage: benchmarking of bacteriophage identification tools in metagenomic sequencing data. Microbiome. (2023) 11:1–15. doi: 10.1186/s40168-023-01533-x
59. Jumper J, Evans R, Pritzel A, Green T, Figurnov M, Ronneberger O, et al. Highly accurate protein structure prediction with AlphaFold. Nature. (2021) 596:583–9. doi: 10.1038/s41586-021-03819-2
60. Lin Z, Akin H, Rao R, Hie B, Zhu Z, Lu W, et al. Evolutionary-scale prediction of atomic-level protein structure with a language model. Science. (2023) 379:1123–30. doi: 10.1126/science.ade2574
61. Lehmann KC, Gulyaeva A, Zevenhoven-Dobbe JC, Janssen GMC, Ruben M, Overkleeft HS, et al. Discovery of an essential nucleotidylating activity associated with a newly delineated conserved domain in the RNA polymerase-containing protein of all nidoviruses. Nucleic Acids Res. (2015) 43:8416–34. doi: 10.1093/nar/gkv838
62. Slanina H, Madhugiri R, Bylapudi G, Schultheiß K, Karl N, Gulyaeva A, et al. Coronavirus replication-transcription complex: Vital and selective NMPylation of a conserved site in nsp9 by the NiRAN-RdRp subunit. Proc Natl Acad Sci U S A. (2021) 118:2–13. doi: 10.1073/pnas.2022310118
63. Malone B, Urakova N, Snijder EJ, Campbell EA. Structures and functions of coronavirus replication–transcription complexes and their relevance for SARS-CoV-2 drug design. Nat Rev Mol Cell Biol. (2022) 23:21–39. doi: 10.1038/s41580-021-00432-z
64. Candresse T, Svanella-Dumas L, Marais A, Depasse F, Faure C, Lefebvre M. Identification of seven additional genome segments of grapevine-associated Jivivirus 1. Viruses. (2023) 15:39. doi: 10.3390/v15010039
65. Adriaenssens EM, Roux S, Brister JR, Karsch-Mizrachi I, Kuhn JH, Varsani A, et al. Guidelines for public database submission of uncultivated virus genome sequences for taxonomic classification. Nat Biotechnol. (2023) 41:898–902. doi: 10.1038/s41587-023-01844-2
66. Arita M, Karsch-Mizrachi I, Cochrane G. The international nucleotide sequence database collaboration. Nucleic Acids Res. (2021) 49:D121–4. doi: 10.1093/nar/gkaa967
67. Eloe-Fadrosh EA, Ahmed F, Anubhav, Babinski M, Baumes J, Borkum M, et al. The National Microbiome Data Collaborative Data Portal: An integrated multi-omics microbiome data resource. Nucleic Acids Res. (2022) 50:D828–36. doi: 10.1093/nar/gkab990
Keywords: RNA virus discovery, viral metagenomics, RNA-dependent RNA polymerase, viral genome annotation, metagenomic metadata standards, virus evolution and diversity
Citation: Charon J, Olendraite I, Forgia M, Chong LC, Hillary LS, Roux S, Kupczok A, Debat H, Sakaguchi S, Tahzima R, Nakagawa S, Babaian A, Abroi A, Bejerman N, Ben Mansour K, Brown K, Butkovic A, Cervera A, Charriat F, Chen G, Chiba Y, De Coninck L, Demina T, Dominguez-Huerta G, Dubrulle J, Gutierrez S, Harvey E, Jayaraj Mallika FR, Karapliafis D, Lim SJ, Kasibhatla SM, Mifsud JCO, Nishimura Y, Ortiz-Baez AS, Raco M, Rivero R, Sadiq S, Saghaei S, San JE, Shaikh HM, Sieradzki ET, Sullivan MB, Sun Y, Wille M, Wolf YI, Zrelovs N and Neri U (2024) Consensus statement from the first RdRp Summit: advancing RNA virus discovery at scale across communities. Front. Virol. 4:1371958. doi: 10.3389/fviro.2024.1371958
Received: 17 January 2024; Accepted: 15 February 2024;
Published: 04 April 2024.
Edited by:
Jie Cui, Fudan University, ChinaReviewed by:
Akio Adachi, Tokushima University, JapanCopyright © 2024 Charon, Olendraite, Forgia, Chong, Hillary, Roux, Kupczok, Debat, Sakaguchi, Tahzima, Nakagawa, Babaian, Abroi, Bejerman, Ben Mansour, Brown, Butkovic, Cervera, Charriat, Chen, Chiba, De Coninck, Demina, Dominguez-Huerta, Dubrulle, Gutierrez, Harvey, Jayaraj Mallika, Karapliafis, Lim, Kasibhatla, Mifsud, Nishimura, Ortiz-Baez, Raco, Rivero, Sadiq, Saghaei, San, Shaikh, Sieradzki, Sullivan, Sun, Wille, Wolf, Zrelovs and Neri. This is an open-access article distributed under the terms of the Creative Commons Attribution License (CC BY). The use, distribution or reproduction in other forums is permitted, provided the original author(s) and the copyright owner(s) are credited and that the original publication in this journal is cited, in accordance with accepted academic practice. No use, distribution or reproduction is permitted which does not comply with these terms.
*Correspondence: Uri Neri, dXJpLm5lcmlAZ21haWwuY29t